1. Introduction
In Brazil, about 159 million hectares of agricultural land is occupied by pastures, and the country has established in most of these areas the largest herd of cattle in the world with 221.81 million head [1].
However, high stocking rates, soil compaction and lack of management for nutrient replacement negatively affect the quality of pastures and soil, becoming precursors to degradation [26]. Thus, the use of new technologies for the correct management of these areas is important to prevent the degradation of new areas, since the use of pastures represents a practical alternative to feed ruminants and, concomitantly, to produce meat and milk [12].
Therefore, degradation in pasture areas can also be a consequence of overgrazing, so animal trampling, with the high intensity of vegetation removal by grazing, decreases the infiltration rate, increases soil density and reduces root growth of plants. Thus, the impact of trampling and high stocking rates can cause soil compaction and biological degradation of pastures. However, these possible phenomena are mainly dependent on the soil class, the soil's edaphoclimatic conditions, its moisture content, the animal stocking rate, the available forage mass and the forage species used in the system [15,26].
Orbital satellite images following the study of soil physical properties have been used to determine degraded pasture areas [23,25]. Therefore, through the vegetation indices that can be obtained in digital images by specific software, it is possible to monitor and document variables that cause degradation in areas with grazing as well as areas that are arid or without vegetation, etc., in large or small fields and at different time scales [19,21].
Digital image processing with the application of the Surface Energy Balance Algorithm for Land (SEBAL), for example, allows obtaining thematic maps of the energy balance components, with analyses in space and time practically and efficiently with low operational cost, determining biophysical parameters such as the vegetation indices Soil Adjusted Vegetation Index (SAVI) and Leaf Area Index (LAI). Changes in the cover and soil use can be observed directly by the reduction of the SAVI and LAI indices [5,18,21].
Given this context and given the need of the environmental monitoring of the vegetation cover of pasture in semiarid soil, the objective was to verify possible modifications of the soil structure and the pattern of the spectral response of pasture vegetation cover in the function of animal trampling. This was done with the assistance of multivariate factorial analysis of soil physical-hydric attributes the and use of the geoprocessing in the application of remote sensing techniques as well as satellite images to determine biophysical parameters, such as the vegetation indices, before and after grazing in a Brazilian semiarid region.
2. Material and methods
The study area is located in the municipality of Capoeiras (Fig. 1), which is in the Agreste region. This region is in the dairy basin. The sites are in the State of Pernambuco, which has Brazilian semiarid climate, and is located between the parallels of 08°37'10.27'' S (UTM: 9046392 m) and 08°37'11.82'' S (UTM: 9046344 m), and between the meridians of 36°36'46.50'' W (UTM: 762715 m) and 36°36'47.98'' W (UTM: 762670 m), with an altitude of 800 m, being west of the meridian of Greenwich. According to the climatic classification of Köppen, the climate of the region is characterized as semiarid (Bsh), and the average annual precipitation of the region is 588 mm. The soil of the study area was classified as Planosol according to the Agroecological Zoning of Pernambuco [31]. Soil characterization was performed according to the methods described by [6] in Table 1.
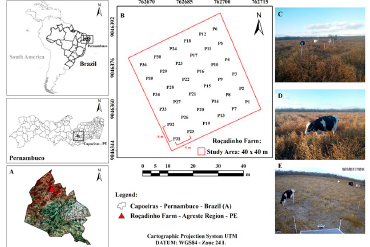
Source: The Authors.
Figure 1 Spatial location map of the municipality of Capoeiras-PE (A), study area (B), grazing field before (C), during (D) and after (E) the grazing period. The image was georeferenced by the Universal Transverse Mercator (UTM), with a spatial resolution of 10 m.
Table 1 Physical characterization of the study area, a commercial property of dairy cattle in the municipality of Capoeiras-PE.
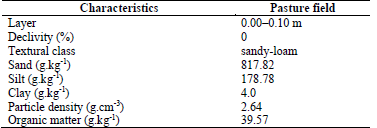
Source: The Authors.
The research was conducted in the spring season, from 16/10 to 05/11/2016, totalling 21 consecutive days. The grazing field had a size of 40 × 40 m (Fig. 1) and an enclosure for the animals, measuring 8 × 10 m, containing a drinking fountain and a shaded area of approximately 16 m². The soil of the study area was prepared with a harrow before planting of the grass; however, no fertilization or soil corrections were used. The area also had negligible slope. Management was adopted in a continuous grazing system, in soil with grazing of Brachiaria decumbens, under rainfed conditions. Three Girolando heifers with an average weight of 300 kg were used, which started the grazing period with a mean forage height of 0.90 m and it was suspended when a mean height of 0.23 m was reached, as indicated by [11].
In the grazing area, 36 points were defined in a 6 × 6 m regular mesh (Fig. 1), where resistance to penetration, moisture, bulk density, total porosity and soil particle density were determined at depths of 0.00-0.10 m in addition to the hydraulic conductivity in saturated soil and the organic matter in the soil surface. An Uhland-type sampler was used for the undeformed collection of soil.
The undeformed soil samples were weighed to determine the wet soil mass and then oven-dried at 105 °C for 24 h to obtain the dry soil mass. Soil density (SD) was calculated from the weight of the oven-dried soil and the total volume of the soil cores. Gravimetric water content (w) at sampling was then calculated as the difference between the wet weight of each soil core at sampling and then after oven drying. The particle density (PD) was determined by the volumetric flask method. The total soil porosity (TP) was quantified using the ratio of SD to PD. The soil organic matter content (OM) was measured via oxidation with potassium dichromate in sulfuric acid, and the fractions of sand, silt and clay were determined by the hydrometer method. All procedures were performed according to [6].
An impact penetrometer, model IAA/Planalsucar/Stolf, was used to determine soil penetration resistance (PR), with the number of impacts dm-1 transformed into dynamic resistance (MPa) utilizing eq. (1) as proposed by [24].
where: PR-Soil penetration resistance, kgf.cm-2 (kgf.cm-2 × 0.098 = MPa); M-Mass of piston, 1.6 kg; m-Mass of machine without piston, 1.5 kg; h-Height of piston fall, 54 cm; X-Penetration of machine stick, cm by impact; A-Area of cone, 1.35 cm2.
To characterize soil water infiltration, the Beerkan method was used, based mainly on simplified infiltration tests and soil particle size distribution analysis [14]. For the determination of the hydraulic conductivity of the saturated soil, the methodology developed by [4] according to eq. (2).
where: K0 soil water infiltration, the Beerkan method Hydraulic conductivity in saturated soil, mm.s-1; b-Slope of an equation linearized in the function of cumulative infiltration over time; r-Ring radius, 75 mm; α-adopted 0.012, for practices in permeameters and infiltrometers in soils that range from thick sand to compacted clay.
Remote sensing techniques were introduced to aid and consolidate terrestrial information using two images of the Sentinel-2 satellite (processing level 2A) Multispectral Instrument (MSI) sensor and with a spatial resolution of 10 m. The mapping was carried out of the pasture field of the study area. The images were made available by the European Space Agency (ESA) via the EOS/Land Viewer (Earth Observing System) platform, dated 08/10/2016 (before grazing) and 07/11/2016 (after grazing). The digital and semi-automated processing of the images was performed using the ERDAS IMAGINE® 9.1 Software, in which some steps of the SEBAL (Surface Energy Balance Algorithm for Land) algorithm, responsible for the mathematical operations of image processing, was implemented (2002). The algorithm is based on the satellite's multispectral bands, and is used to obtain thematic maps of the terrestrial surface [5,2]. From the level 2A product of reflectance (corrected atmospheric product, including cloud tracking and adjacency/slope effects correction), the multispectral bands of the Sentinel-2A images are a baseline for the geophysical parameters [7,9,10]. The images were georeferenced by the Universal Transverse Mercator (UTM) Map Projection System, DATUM: WGS84-Zone 24 L.
From the multispectral reflective bands of satellite images, the vegetation indexes were determined: SAVI (Soil Adjusted Vegetation Index) and LAI (Leaf Area Index). The SAVI was determined according to eq. (3) [3,10,21].
where: SAVI-Soil Adjusted Vegetation Index; B08 e B04-near-infrared multispectral bands (NIR) and red of the Sentinel-2A satellite respectively [9]. L is the soil adjustment factor, which varies between 0 and 1, according to the vegetation cover density of the study area. An adjustment factor of 0.5 was used, which indicates an area with intermediate vegetation cover [16,2]. The chosen factor refers to the study area, which is located in the Brazilian semiarid and has Caatinga vegetation [22,21].
With the determination of the Soil Adjusted Vegetation Index (SAVI), the Leaf Area Index (LAI, m2 m-2) was determined as an indicator of the biomass amount according to eq. (4) [2, 19].
The results of the measured soil attributes were subjected to a multivariate factorial analysis, an exploration that makes it possible to ascertain the greatest amount of original information contained in the variables, and projects the attributes that better explain the impact of animal trampling under the soil during the study period.
The results of the SAVI and LAI vegetation indexes were analysed by descriptive statistics, central tendency (mean) and dispersion (minimum, maximum, standard deviation-SD and coefficient of variation-CV). The SAVI and LAI thematic maps were evaluated for spatial variability, by CV values (%), according to [28] criteria, which were being of low variability-CV<12%; mean variability-12%<CV<60% and high variability-CV>60%.
3.. Results and discussion
The soil physical attributes promoted patterns of important responses to the pasture field, based on multivariate statistical analyses, which allowed observing results over the level of soil compaction and the vulnerability to degradation due to grazing area management over time.
The spatial monitoring of the pasture vegetation management through the SAVI and LAI vegetation indices was possible by the development of thematic maps of the terrestrial surface, which showed different patterns of responses over the 21-day study period, emphasizing the changing conditions in the pasture through of the SAVI and IAF indices before and after the experiment.
The multivariate factorial analysis explained 59.21% of the total information in Factor 1 and Factor 2 (Fig. 2A) for the time before grazing, and, 57.61% of the two main factors explained the system for the period after grazing. [29] who evaluated the impact of pasture degradation on soil carbon and nitrogen fractions, through multivariate analysis and observed values of total variance greater than 50%, similarly to the present study.
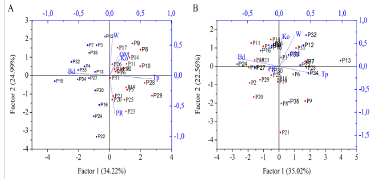
Source: The Authors.
Figure 2 Multivariate factorial analysis of soil physical-hydric attributes, before (A) and after (B) grazing.
After grazing, the variables measured in the study exposed changes (Fig. 2B), mainly those related to the porous space and the hydraulic conductivity of the soil, driven by positive relationships between soil density and resistance to soil penetration, which occupied the opposite direction the total porosity, caused by the pressure exerted on the hooves of the animals, that is, this finding indicates that the trampling of heifers in the pasture area compacted the soil at a depth of 0.00-0.10 m (Figs. 2A and 2B). These changes may favour the occurrence of pasture degradation [13,27].
Similar results were observed by [17], who reported that after animal trampling, the soil's physical-hydraulic properties underwent some type of modification, mainly with increased density and reduced infiltration rate, the authors also pointed out that clear signs of vegetation degradation were observed, manifested by a loss in species diversity and vegetation cover.
The vegetation cover of pasture highlighted over time a high variation at the end of the experiment, confirmed as well by monitoring grazing in the 21 uninterrupted days by remote sensing of the vegetation indices SAVI and LAI (Figs. 3 and 4).
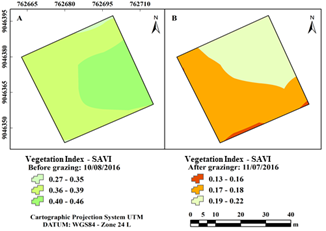
Source: The Authors.
Figure 3 Spatial distribution of the SAVI vegetation index in the pasture field, before (A) and after grazing (B).
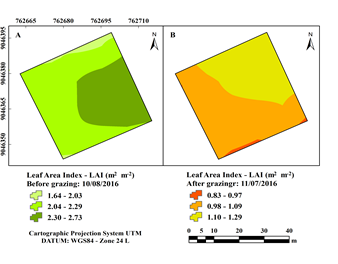
Source: The Authors.
Figure 4 Spatial distribution of the LAI vegetation index in the pasture field, before (A) and after grazing (B).
Fig. 3 illustrates the behaviour pattern of the SAVI index through thematic maps of the terrestrial surface in the study area.
Fig. 4 also illustrates the behaviour pattern of the Leaf Area Index (LAI) through thematic maps of the terrestrial surface in the study area. The LAI confirms the results of the response patterns found by the SAVI index, highlighting the main behaviour pattern of the vegetation cover of the pasture.
The monitoring of the pasture area by satellite images via remote sensing confirmed the decrease in vegetation, that is, the reduction of the leaf canopy and the amount of biomass in the study area. After grazing (Figs. 3B and 4B), it is possible to observe with both the SAVI and the LAI, the reduction of the minimum values, mainly next to zero, evidencing areas of exposed soil.
It is interesting to observe the conditions of changes in grazing, which were well supported quantitatively by the variation of the minimum and maximum values and in the averages of the spatial analysis. Thematic maps of the SAVI and LAI indices showed a pattern of the spatial distribution of low variability, with CV ranging between 10.48 and 11.87% (Table 2).
Table 2 Statistical parameters and quantitative variability of the SAVI and LAI vegetation indices in the study area, before and after grazing.
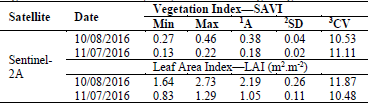
1Average, ²Standard deviation, ³Coefficient of variation (%).
Source: The Authors.
The minimum values of the SAVI and LAI decreased after grazing as well as the maximum values being significantly reduced. It can be seen that the average values followed the same reductive tendency, showing areas of exposed soil after grazing that may favour the processes of environmental degradation of the soil in the semiarid region over time (Table 2).
The SAVI was a good indicator of the conditions of the pasture in the study. [31,26], highlight that the use of NDVI is a good indicator of pasture conditions, showing values below 0.6 and 0.5, respectively, as the beginning of pasture degradation. As SAVI is an improved NDVI index, it is a better indicator for identifying degraded areas, with values below 0.4 describing a possible degradation of pasture in the present study.
Because of this aspect, the application of remote sensing techniques to identify pasture degradation areas proved a promising tool, combined with vegetation indexes, which in turn, expressed the conditions of the study area [8,20].
4. Conclusion
The intensive management of the grazing, with the bovine trampling pressure altered the soil structure, thus increasing soil density and soil penetration resistance, favouring soil compaction and, consequently, hindering regrowth and renewal of the pasture and reducing the productivity.
The SAVI and LAI vegetation indices presented thematic maps of low variability and were sensitive to changes in the vegetation cover of pasture before and after grazing.
Remote orbital sensing through vegetation indexes was efficient in determining the response pattern of pasture vegetation in the semiarid region.