In recent times, mental health concerns have escalated,*1particularly due to the COVID-19 pandemic, profoundly impacting well-being (Losada-Baltar et al., 2021; Sandín et al., 2020). Responses to this crisis across nations and age groups have led to various levels of psychological distress, including fear, anger, depression, anxiety, and stress (Lathabhavan, 2021). A systematic review of global psychological status during the pandemic revealed an increased prevalence of psychiatric symptoms such as depression, anxiety, and stress (Xiong et al., 2020).
Notably, data from various countries underscores increased levels of depression and anxiety symptoms compared to the pre-pandemic period. A recent meta-analysis indicated a statistically significant rise in the prevalence of anxiety (from 8.90% pre-pandemic to 22.60% during the pandemic) and depressive symptoms (from 8.70% to 18.30%) (Schafer et al., 2022). In Spain, during the pandemic’s health alert phase, González-Sanguino et al. (2020) reported potential anxiety diagnoses in 21.60% of their sample and probable depression diagnoses in 18.70%.
Demographic factors, especially gender and age, are frequently cited predictors of mental health disorders. Throughout the pandemic, studies consistently show that women are more prone to depression, anxiety, and stress symptoms than men (Fountoulakis et al., 2022; González-Sanguino et al., 2020; Xiong et al., 2020). However, consensus on the impact of age is lacking, with some studies suggesting increased risk for those under 40 years of age (Xiong et al., 2020), while others found no age-related differences (Schafer et al., 2022).
On the other hand, there are modifiable factors such as emotional intelligence (EI) and resilience that can impact emotional health. EI comprises self-emotional appraisal, other’s emotional appraisal, regulation of emotion and use of emotion (Mayer & Salovey, 1997). In more recent updates of the model (Mayer et al., 2016), EI has been positioned in its relation to other intelligences, such as personal and social intelligence. Robust evidence suggests that effective emotion management acts as a protective factor against psychopathology (Delgado-Gómez et al., 2019; Delhom et al., 2018; Navarro-Bravo et al., 2019). Adequate EI skills correlate negatively with depression and perceived stress (Bermejo-Martins et al., 2021; Extremera et al., 2006; Fernández-Berrocal & Extremera, 2016; Mao et al., 2021). Conversely, low emotional clarity and repair are linked to higher risks of anxiety disorders and depression (Bardeen & Stevens, 2015; Bhat & Farooq, 2017; Okasha et al., 2022). Research during the COVID-19 pandemic demonstrated that enhancing EI could alleviate anxious and depressive symptoms in the general population (Persich et al., 2021).
The association between EI and psychopathology is influenced by factors such as gender and age, with varying relevance across studies (Navarro-Bravo et al., 2019; Salguero et al., 2012). Gender differences in emotional regulation patterns, influenced by cultural norms, impact the relationship between EI and psychopathology. Measurement instrument choice also influences gender’s role; for example, using performance testing, low EI is associated with greater depression in men but not in women (Salguero et al., 2012). Conversely, using a self-report instrument, gender is not significant, showing lower perceived EI associated with greater depressive symptoms in both men and women (Salguero et al., 2015). Similar results were obtained in the research conducted by Sergi et al. (2021).
Investigating age’s impact on the relationship between EI and psychopathology reveals significant moderating effects in some age groups. For instance, the association between depression and EI is significant among individuals 60 years of age or younger (Navarro-Bravo et al., 2019). Additionally, resilience emerges as a relevant protective factor against mental health disorders. Studies confirm a close relationship between EI and resilience, suggesting that better emotional regulation enhances resilience, resulting in improved affective balance and lower distress (Armstrong et al., 2011; Kimhi et al., 2020; Liu et al., 2013). An EI training programme has been shown to enhance EI skills and resilience while reducing perceived stress in a group of nurses (Mao et al., 2021). In other studies (Peláez-Fernández et al., 2021), it was demonstrated how resilience and EI were important predictors of depressive symptoms in situations of job loss or interruption, similar to those experienced during the pandemic.
Understanding the intricate interplay of EI, resilience, and socio-demographic characteristics is crucial for targeted interventions to prevent or overcome mental disorders such as depression, anxiety, and stress. Therefore, this study aims to analyse the predictive power of age, gender, emotional intelligence, and resilience with regard to anxiety, depression, and stress-related symptoms in a Spanish population sample. Linear and non-linear methodologies are used in this study to investigate the relationship between the variables analysed in much greater depth. Additionally, qualitative comparative analysis (QCA) models allow observing the different paths or combinations that lead to a given result, providing great value to the present study. Based on findings from previous literature reports, the proposed hypothesis states that younger women in the sample, especially those with poor EI skills and lower resilience levels, will have a higher risk of developing some form of psychopathology.
Methodo
Participants
A study involving 427 Spanish participants (78.50% female), between 18 and 83 years of age (M = 35.81, SD = 11.18), was conducted. Marital status varied, with 33.50% single, 28.60% married, 4.70% divorced, and 0.70% widowed. Employment status included 60.40% employed, 10.50% self-employed, 23.70% unemployed, 1.60% retired, and 1.90% students. Education levels showed 82.40% with university studies, 15.80% with secondary education, and 1.60% with primary education. All participants were of Spanish nationality. The inclusion criteria were completing all questionnaires, signing the informed consent, and not suffering from severe mental disorders (schizophrenia or bipolar disorder). The study was endorsed by the Committee on Ethics in Human Research (CEISH) of the Valencian International University and complied with the Helsinki Declaration.
Instruments
- Socio-demographic variables: their analysis required the development of an ad hoc questionnaire that included gender, age, marital status, academic background, employment status and physical/mental health history.
- Emotional Intelligence: Trait Meta Mood Scale - 24 (TMMS-24; Salovey et al., 1995), adapted to Spanish (Fernández-Berrocal et al., 2004). The TMMS-24 is a test used for the evaluation of emotional attention, emotional clarity, and emotional repair. The scale has 24 items with a range of responses from 1 “strongly disagree” to 5 “strongly agree”. In this study, good reliability levels were found for emotional attention (a = .88), emotional clarity (a = .89) and emotional repair (a = .86). This test has shown good psychometric properties in previous studies, including good convergent validity with other tests aimed at exploring this dimension (Górriz et al., 2021).
- Resilience: Brief Resilience Coping Scale (BRCS; Sinclair & Wallston, 2004), adapted to Spanish (Moret-Tatay et al., 2015). The scale has four items that explore a person’s ability to cope with life’s adversities. These items have a Likert- type structure, with responses ranging from 1 “strongly disagree” to 5 “strongly agree”. For this study, an internal consistency level of a = .69 was found, which is in line with that of previous studies (Limonero et al., 2014). The test demonstrates adequate convergent validity with other tests aimed at assessing resilience, both in the youth (Limonero et al., 2014) and in older adults (Tomás et al., 2012).
- Depression, anxiety, and stress: Depression, Anxiety and Stress Scales (DASS-21; Lovibond & Lovibond, 1995), adapted to Spanish (Fonseca-Pedrero et al., 2010). The DASS-21 explores depression, anxiety, and stress symptoms. It has 24 items with a Likert-type format ranging from 0 “never happened to me” to 3 “happened to me frequently”. In this research, good levels of reliability were found for depression (a = .91), anxiety (a = .88) and stress (a = .88); replicating that found in previous research (Gurrola et al., 2006). This test has demonstrated satisfactory convergent validity indices with other instruments for exploring depression, anxiety, and stress (Lee, 2019).
Procedure
All participants read and signed the informed consent form and accessed the assessment tests via Google Forms. The test battery was designed specifically for this study and took an average of 15 minutes to complete. An online methodology was used to avoid the communication barriers resulting from the COVID-19 health crisis. The test battery was shared by way of social media platforms (Twitter, Instagram, and Facebook), as well as via email. The resulting database was converted to a .sav format for statistical analysis, removing any identifiable subject data. Sampling was non-probabilistic, using a snowball approach. The guidelines were developed according to the ethical and deontological requirements of the Declaration of Helsinki (World Medical Association, 2013). In addition, the study was approved by the ethics committee of the Valencian International University (CEID2021_15).
Data analysis
Descriptive analyses, Student’s t-tests for independent means, as well as effect size calculations using Cohen’s d (d ≥ .20 (small), d ≥ .50 (medium), and d ≥ .80 (large).), Pearson correlations, and hierarchical regression models were conducted using SPSS v.26.
Finally, a fuzzy-set qualitative comparative analysis was conducted using the fsQCA software (Claude, 2014). This involved transforming raw data into fuzzy sets, removing missing data and recalibrating the constructs (Woodside, 2013). Necessity and sufficiency tests were conducted to test for the effects of age, gender, EI and resilience on depression, anxiety, and stress. To identify the necessary conditions, the fsQCA analysis uses an algorithm that converts all the data into a truth Table showing all the logical combinations of conditional causes and outcomes for each potential configuration. Finally, the software generates three possible solutions: complex, parsimonious, and intermediate. The latter is the most recommended and selected for this study (Ragin, 2008). The sufficiency analysis considers that the coverage solution refers to the explained variance (number of observations that can be explained by a specific combination of conditions), while the consistency solution refers to the model’s reliability.
Results
Descriptive statistics and relationship between variables Descriptive and comparative analysis
Moderate scores were observed for emotional attention, clarity, and repair. Resilience reached medium-high levels. Depression and stress scores were low, whereas anxiety scores were moderate (Table 1).
Table 1 Descriptive analysis (N = 427)
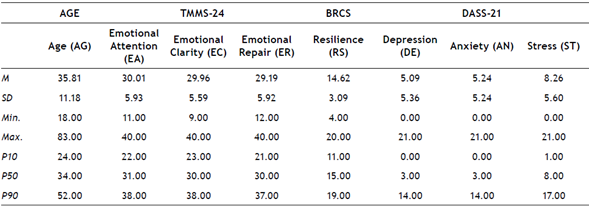
Note: Age (AG), Emotional Attention (EA), Emotional Clarity (EC), Emotional Repair (ER), Resilience (RS), Depression (DE), Anxiety (AN) and Stress (ST)
Women were found to have significantly higher levels of emotional attention, anxiety, and stress than men. In addition, moderate effect sizes were found for differences in emotional attention and stress. Anxiety reached a small effect in Cohen’s d test (Table 2).
Table 2 Comparative analysis of dependent variables according to gender
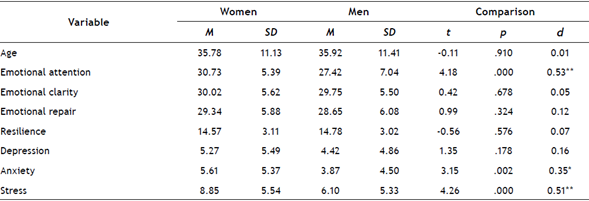
Note. Size effect (Cohen’s d): * p ≤ .05; ** p ≤ .01 *** p ≤ .001
When psychological symptom levels were examined according to the cut-off points of the DASS-21 questionnaire, most participants were found to have medium-low levels of stress, anxiety, and depression (Figure 1).
Correlational analysis
The correlational analysis revealed several associations among the variables studied. Age demonstrated links with EI and resilience. Emotional attention did not show significant associations with other EI factors but exhibited a negative correlation with resilience. Emotional clarity and emotional repair exhibited a moderate positive relationship, and both correlated positively with resilience. Additionally, age demonstrated significant correlations with psychological symptoms, and EI showed relevant covariation with these symptoms. Similar patterns were observed for emotional repair. Lastly, resilience displayed negative correlations with depression, anxiety, and stress (Table 3).
Hierarchical regression models vs. QCA
Hierarchical regression models
The study employed a HRM to analyse the predictive capacity of variables. Depression, anxiety, and stress served as criterion variables, and gender, age, EI, and resilience were designated as predictive dimensions. The model comprised three steps: the first step included gender and age, the second step incorporated EI, and the final step introduced resilience. Regarding the third step (which includes all the variables in the model), significant standard beta coefficients were found in emotional attention, emotional clarity and emotional repair with depression as criterion variable. For anxiety, data were obtained for gender (women), emotional attention, emotional clarity, and emotional repair. Finally, on stress, significant standard beta coefficients were found for gender (women), emotional attention, emotional clarity, and emotional repair. Overall, the final model explained 28% of the variance for depression, 25% of the variance for anxiety and 23% for stress (Table 4).
Fuzzy-set qualitative comparative analysis (fsQCA)
Analysis of Necessity First, the main descriptors and calibration values of the study variables are presented (Table 5). According to the results obtained, there were no necessary conditions for high and low levels of depression, anxiety, and stress, as the consistency was below .90 for all cases (Ragin, 2008).
Table 5 Main descriptions and calibration values
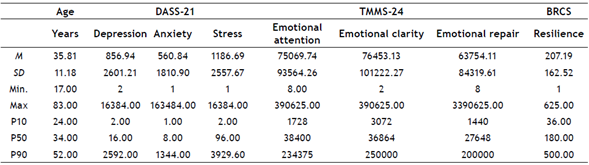
Note. M: mean; SD: standard deviation; min: minimum; max: maximum; P10 =:10th percentile; = P50 = 50th percentile; P90 =: 90th percentile
Analysis of Sufficiency For the analysis of sufficiency, the combination of conditions leading to high and low levels of depression, anxiety and stress was calculated (Table 3). Given that in the fsQCA, a model is informative when the consistency is around or above .74 (Eng & Woodside, 2012), all models obtained were consistent.
In predicting high depression levels, four pathways explained 23% of the high levels (Overall consistency = .85; Overall coverage = .23). The most relevant pathway was the interaction between being of a young age, having high resilience and emotional attention and clarity, and low emotional regulation and clarity (Raw coverage = .22; Consistency = .86). In predicting low depression levels, five combinations explained 82.00% of low levels (Overall consistency = .80; Overall coverage = .82). The most relevant pathway explaining most of the variance was the presence of good emotional regulation, the second pathway was good emotional clarity, and the third pathway was the interaction between high resilience and older age. The first pathway explained 60 % of cases (Raw coverage = .60; Consistency = .79).
As for the analyses conducted for the anxiety dependent variable, the intermediate solution indicated five causal combinations that explained high anxiety levels and accounted for 46% of the cases (Overall consistency = .79; Overall coverage = .46). In this prediction, the most relevant pathway was the result of the interaction between being female, young, with low emotional regulation and clarity and high emotional attention (Raw coverage = .34; Consistency = .82). Five pathways were observed in predicting low anxiety levels that explained 88.00 % of the cases with low levels (Overall consistency = .75; Overall coverage = .88). The most important predictor of low anxiety was the presence of resilience which explained 59.00% of cases (Raw coverage = .59; Consistency = .80). The second predictor was the presence of emotional clarity, and the third predictor was the presence of emotional regulation.
In predicting high levels of negative stress, twelve pathways explained 79% of high levels (Overall consistency = .77; Overall coverage = .79). The most relevant pathway was the interaction between being female, lacking resilience and emotional regulation, and having high emotional attention (Raw coverage = .41; Consistency = .79). For low stress levels, thirteen pathways explained 79.00% of the high levels (Overall consistency = .77; Overall coverage = .79). The most relevant pathway was the interaction between resilience and the absence of emotional attention (Raw coverage = .46; Consistency = .74).
Conclusions
In recent years, particularly following the COVID-19 pandemic, mental health issues have garnered increased attention among researchers and healthcare professionals due to a rise in psychopathological distress among individuals (Sandín et al., 2020; Schafer et al., 2022; Xiong et al., 2020). Consequently, there is growing interest in studying protective psychological factors such as EI (Delgado-Gómez et al., 2019; Delhom et al., 2018; Navarro-Bravo et al., 2019) and resilience (Kimhi et al., 2020; Riehm et al., 2021) that contribute to mitigating mental disorder symptoms. Additionally, socio-demographic variables such as age and gender have been associated with an increased risk of mental health disorders (Fountoulakis et al., 2022; González-Sanguino et al., 2020; Xiong et al., 2020), necessitating consideration in preventive intervention planning for specific groups. (Table 6)
Table 6 Summary of the main sufficient conditions for the intermediate solution of depression, anxiety and stress
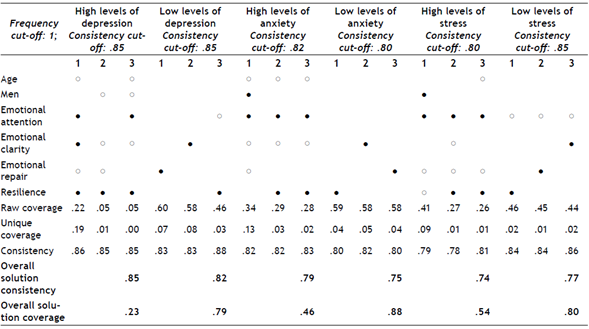
● = presence of condition. ○ = absence of condition.
Expected vector for high levels of depression, anxiety and stress: 0.0.1.0.0.0.0 (0: absent; 1: present)
Expected vector for low levels of depression, anxiety and stress 1.1.0.1.1.1.1 using the format of (Fiss, 2011)
However, most studies evaluating the predictive value of these variables on psychopathological distress have relied solely on linear methodologies (Delhom et al., 2018; Gao et al., 2020; Kimhi et al., 2020). Thus, this study aimed to provide evidence of the predictive power of the EI, age, gender, and resilience dimensions on psychopathological distress (depression, anxiety, and stress) using a combination of linear and non-linear methodologies, facilitating the comparison of results and exploration of variable relationships.
Results from hierarchical regression models (HRM) indicate that the three dimensions of EI were measured using the TMMS-24 (attention, clarity, and repair), but not age and resilience, explain the three emotional problems analysed (depression, anxiety, and stress). Moreover, gender also plays a significant role in explaining variance in anxiety and stress levels. These findings align with previous research demonstrating the importance of EI for psychopathological distress (Bardeen & Stevens, 2015; Bhat & Farooq, 2017; Delgado-Gómez et al., 2019; Delhom et al., 2018; Fernández-Berrocal & Extremera, 2016; Navarro-Bravo et al., 2019; Okasha et al., 2022), highlighting its role in effective emotion management and its association with better mental health and adjustment.
QCA model results reveal that being male and younger, possessing high levels of resilience and emotional attention, and low levels of emotional clarity and repair explain high depression levels, expanding upon other associations found in previous studies (Salguero et al., 2012). Conversely, low depression levels are associated with emotional clarity, understanding, and regulation, particularly among older individuals with high resilience levels. These findings are consistent with those of previous studies (Armstrong et al., 2011; Kimhi et al., 2020; Liu et al., 2013) and support the notion that emotionally intelligent individuals exhibit moderate or low emotional attention and high clarity and repair, while those with excessive attention and inadequate regulation tend to experience negative emotional states (Bermejo- Martins et al., 2021; Extremera et al., 2006; Fernández- Berrocal & Extremera, 2016; Mao et al., 2021).
Regarding the predictive effect of gender on depression, QCA model results contradict prevailing literature and hypotheses (Fountoulakis et al., 2022; González-Sanguino et al., 2020; Xiong et al., 2020), indicating that gender did not predict depression in the HRM. This discrepancy suggests that QCA models offer nuanced insights into complex relationships not captured by other methodologies. Unexpectedly, high levels of resilience explained both high and low depression levels, underscoring the multifaceted nature of resilience’s influence on mental health outcomes. This is an interesting finding whose scope will need to be addressed in future research.
QCA model results for anxiety highlight the interplay of gender, age, emotional clarity, repair, and attention in predicting anxiety levels. Conversely, low anxiety levels are associated with emotional clarity, repair, and resilience. These findings support existing evidence that women tend to experience higher anxiety levels, especially among younger age groups, and underscore the protective role of EI skills and resilience against anxiety (Sergi et al., 2021).
Similarly, QCA model results for stress identify gender, resilience, and emotional attention as key predictors of high stress levels, whereas emotional clarity and repair mitigate stress. These findings reaffirm the gender differences in stress susceptibility (Kogar & Uslu, 2021) and emphasise the importance of effective emotion management in stress reduction.
While the study provides valuable insights, limitations include a predominantly female and highly educated sample, warranting broader demographic representation in future research. Additionally, enhancing sample size which is small in our study, specifically considering the age range collected, and gender balance could improve data generalisability to the Spanish population. Challenges associated with data collection methods, particularly in ensuring participant identity and response accuracy, should also be addressed. It would also be important to implement probabilistic sampling to reduce potential methodological biases. Furthermore, potential limitations associated with self-reported EI assessments should be acknowledged, given evolving construct definitions. In the future, it would be important to control for other potentially confounding variables that could not be considered in this study, such as the reception of previous interventions or established coping mechanisms in the participants.
Nevertheless, the study’s findings shed light on the distinct roles of socio-demographic characteristics and psychological strengths in psychopathological distress, informing targeted intervention design to enhance mental health outcomes. Through the findings of this study, the interaction between fundamental variables for intervention design is expanded using sophisticated statistical strategies, emphasising key therapeutic targets for the success of various intervention approaches. To our knowledge, this study is the first to compare two statistical methodologies in examining this subject.