Diabetes mellitus type II is frequently associated with lipid metabolic alteration, hypertension, being overweight/obese, which leads to metabolic syndrome and, later, to cardiovascular disease and mortality 1-4. There is evidence indicating that the prevalence of the metabolic syndrome increases with age, being 24% at 20 years of age, 30% or more in those over 50 years and over 40% in those 60 years or older 5. Unless urgent public health measures are taken, drastic decreases in life expectancy and increasing expenditure for health systems might generate a negative impact on developing economies, such as Colombia and other similar countries.
The Colombian Observatorio Nacional de Salud reported a 15% mortality due to diabetes mellitus, while hypertension accounted for 14% 5. Another two main contributors to mortality were ischemic heart disease with 53.6% followed by cerebrovascular disease with 20.6% 6. From this national health report, it is evident that non-communicable diseases, among them the metabolic syndrome, have a huge impact on the Colombian population. Such epidemiological situation prompted our question regarding the risk factors associated with the metabolic syndrome among inhabitants from Cali, Colombia. In this context, we conducted a case-control study aimed at determining the social, biological, and behavioral factors associated with the metabolic syndrome.
Materials and methods
We collected the data for 2013 using a random and representative population survey in Cali conducted by the Centro para el desarrollo y evaluación de políticas y tecnología en salud pública (CEDETES) at Universidad del Valle with the support of Cali’s public health office.
Case definition
Any man with waist circumference (abdominal obesity) of ≥94 cm or any woman with ≥88 cm plus two of the factors reported in table 1.
Control definition
Any man with waist circumference of <94 cm or any woman with <88 cm plus one or none of the other criteria established for MS diagnosis.
Population and sample
We calculated the sample size for an urban population of 1,476,264 people over 15 years of age in Cali according to the most recent national population census (2005). We randomly selected 4,000 households and we visited 9,000 families 7, and we included the subjects that fullfilled the case definition, as well as a random subsample of controls.
We applied a multiple logistic regression model to identify the factors associated with the metabolic syndrome. We reported the OR and its 95% confidence interval (CI) for each of the variables that significantly contributed to the metabolic syndrome.
Subjects’ age was tested as a potential confounder factor or having a modification effect (interaction), and we assessed its degree of uncertainty. Given that the study subjects came from a random sample in exposed and non-exposed subpopulations, we assumed that the sampling distribution of the data resulted from two binomial distributions. Based on this assumption, the marginal total was fixed by design and the sample was divided into several strata, which were homogenous internally with respect to the confounding factors. The strata analysis demonstrated that the OR systematically increased with age. We run a homogeneity test along the strata and, based on its result, a chi-square test for the trend.
Usually, the simple dichotomization of a risk variable in a 2 by 2 table will mask the ample rank of the association between exposure risk and disease. However, more valuable information is obtained if the values from a quantitative variable like age are grouped into ordered levels to reveal a dose-response gradient that could be critical to address the therapeutical interventions among those people already affected with metabolic syndrome and, on the other hand, to promote healthy life-styles among still healthy groups. A logistic conditional regression model was applied to determine the relationship between having a metabolic syndrome (case) or not (control), and the different social, biological, and behavioral risk factors.
Results
Overall metabolic syndrome prevalence was 30.2%: 33.6% in women and 25.6% in men.
Table 2 shows the characteristics of cases and controls: Controls were younger than the cases with an average age of 47 (SD=17.23) and cases’ average age was 53.67 (SD=14.36) the difference being significant (p<0.01). Controls also had a lower body mass index (BMI), 25.18 kg/m2 (SD=4.66), whiletheBMIforcaseswas28.68kg/m2(SD=5.37;p<0.01).Fruitconsumption per day was higher in the control group along with the combinedintake of both fruits and vegetables (p<0.01). Vegetable consumption was also higher in the control group, but it was not statistically significant. Additionally, the frequency of physical activity was higher in controls: 4.23 days per week (SD=2.43) as compared to cases with 3.48 days per week (SD=2.60;p<0.01).
Table 2 Demographics, nutritional habits, and biological measures in the metabolic syndrome cases and in the controls
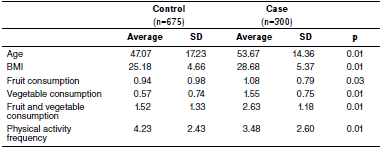
BMI: Body Mass Index
All results were significant.
Table 3 shows the comparison between cases and controls. Being female and a lack of education were the main factors associated with the metabolic syndrome, while ethnicity, civil status, occupation, and type of health insurance appeared not to be related. We also present here the antecedents of behavioral risk factors with their respective percentages categorized in a dichotomous way: Does consume or does not consume, except for the variable “Oil consumption” which included the different types of oil used in the region. This analysis indicated that there was a difference in the alcohol consumption variable: Chi- squared test (1, N=975) =7.97, p<0.05. This difference also occurred in the sugar consumption variable: chi-squared test (2, N=975) =18.5, p<0.01. On the other hand, salt consumption showed a chi-square value for trend (2, N=975) =7.42, p<0.06, while the other variables did not reveal significant differences.
Table 3 Demographics, educational level, occupation, race, and other risk factors associated with the metabolic syndrome
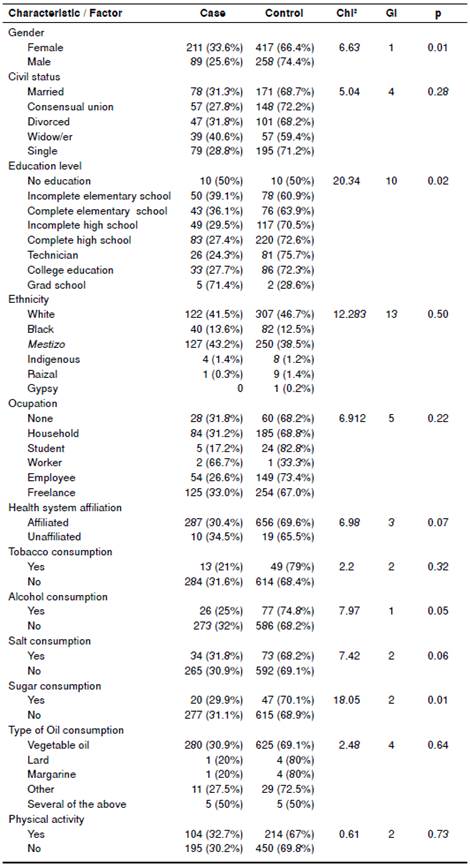
Table 4 shows the results of multiple logistic regression analyses. The strongest risk factor associated with the metabolic syndrome was obesity (OR=7.52; 95% CI 4.79-11.80). Age showed a linear trend with the risk of developing metabolic syndrome. The 40 to 49-year-old group yielded an OR of 4.24 (95% CI 2.20-8.16), the 50 to 59-year-old group one of 4.63 (95% CI 2.40- 8.93), and those older than 80 years, one of 5.32 (95% CI 1.92-14.71). Other important risk factors associated with the metabolic syndrome were being overweight (OR=3.52; 95% CI 2.38-5.20), being female (OR=1.70; 95% CI 1.17-2.47), and being mestizo (OR=1.55; 95%CI 1.10-2.19).
Discussion
Our study aimed at identifying the social, biological, and behavioral factors associated with the metabolic syndrome in Cali in 2013.
The overall metabolic syndrome prevalence was 30.2%: 33.6% in women and 25.6% in men. Other studies have reported similar findings8. A Peruvian study conducted by Soto and Vergara reported a global prevalence of 28.3% according to the Adult Treatment Panel III criteria (ATPIII) 9. The global prevalence in our study was also similar to that reported by Aguilar and Rojas, 25%10.For Asian populations, Mohan Deep a reported a metabolic syndrome prevalence of 13% in China and 30% in Iran 11. In a later study he informed that the prevalence of metabolic syndrome was lower among poor people and in rural areas compared to middle class and urban populations, possibly because the rural population is more engaged in physical activity and consume less processed food. Industrialized food is more related to the urban life style, it is consumed at any time of the day, and is always hypercaloric 12.
Aging is a definitive risk factor for the metabolic syndrome 13,14. This finding may be due to the accumulative effect of the etiological factors along the course of life, the metabolic changes associated with becoming older or the sum of both. It was also relevant in our study showing a linear trend as regarded the risk of developing the metabolic syndrome with a 4.24 OR for the 40-49-year-old-group, 4.63 OR for the 50-59-year-old group, and 5.32 OR for those older than 80 years. This finding supports our hypothesis that increasing age has a modifying effect as demonstrated by the increasing magnitude of the OR through time (table 2 and 4). The homogeneity test showed a significant result (chi2=77.2; DF=4; p<0.001), which means that the odds ratios across the age strata were not uniform. The result of the trend gave a chi2 of 189.7, a DF of 1, and a p-value of <0.000, which happens to be significant suggesting a modifying effect of age in relation to the metabolic syndrome.
A study carried out in Medellin, Colombia, reported sedentarismas the most prevalent risk factor (prevalence, 73.1%) 15. In addition, obesity, smoking, high blood pressure, and a history of coronary disease showed high relevance.
Having dark skin (mestizo or brown skin) showed an association with the metabolic syndrome (OR=1.55; 95% CI 1.10-2.19), similar to the findings reported in Hawaii for multiracial individuals. The association was higher in Hawaiian-Asian natives (OR=1.45; 95% CI 1.27-1.65) than in native populations without mixed ancestry 16. Ethnicity has been associated with cultural and lifestyle habits that predispose to alcohol abuse, smoking, bad eating habits, and less physical activity and sports 17-19.
When analyzing the social factors that may be associated with the metabolic syndrome, we found that the occupation (housekeeper) (OR=0.59; 95% CI 0.40-0.87) was a protective factor. This unexpected result is opposite to what has been reported in the literature. It is possible to infer that in Cali women who are engaged in household activities are less sedentary and, therefore, less likely to develop a metabolic syndrome.
Regarding nutrition, fruit consumption was another protective factor (OR=0.83; 95% CI 0.69-1.00) against the metabolic syndrome, which was also reported in South Korea 20 where healthy eating patterns were found to be protective both for men and women (p=0.005). In contrast, traditional bad eating patterns had a positive relationship with high blood pressure (p=0.0328), low HDL levels (p=0.0007), and higher metabolic syndrome prevalence. Nutritional education could reduce the metabolic syndrome prevalence at the population level 20.
It is worth noting the lack of significant associations with alcohol, sugar, salt intake, and tobacco consumption in our study while in other studies, alcohol intake and smoking seemed to be important risk factors for the occurrence of the metabolic syndrome 21. A study conducted in Brazil showed that taking alcohol with meals had an inverse relationship with the metabolic syndrome: ≤4 drinks a week: OR=0.85; 95% IC 0.74-0.97, and 4 to 7 drinks a week: OR=0.75; 95% IC 0.61-0.92. In contrast, alcohol consuming out of meals showed a direct relationship (7-14 drinks a week: OR=1.32; 95% IC 1.11-1.57; ≥14 drinks a week, OR=1.60; 95% IC: 1.29-1.98) 21. In our study, alcohol consumption was not a factor associated with the metabolic syndrome.
On the other hand, obesity was associated with the metabolic syndrome (OR=7.52; 95% IC 4.79-11.80), as well as being overweight (OR=3.52; 95% IC 2.38- 5.20) (table 4). In a randomized study conducted by Martin, et al. 22, they reported that a high BMI correlated with a higher prevalence of abdominal obesity and with metabolic syndrome 23,24.
In conclusion, this study determined the factors positively associated with the metabolic syndrome, such as being overweight, obesity, being older than 40 years, female and mestizo, while fruit consumption and housekeeping were inversely related. Risk factors are considered important for the development of public health interventions to promote protective behaviors and healthy lifestyles in the population.