In 2020, the World Health Organization (WHO) declared the severe acute respiratory syndrome coronavirus type 2 (SARS-CoV-2) outbreak, originally reported on December 31, 2019, in Wuhan of the Hubei province in China, as a public health emergency 1. Until today, the preventive measures against SARS-CoV-2 have focused globally in the homogeneous implementation of mitigation measures such as the interruption of non-essential services for prolonged periods, generating social and economic costs 2, or suppression measures such as social distancing that includes the restriction of mobility and the establishment of remote work. On March 17, 2020, the Colombian government issued Decree 417 of 2020, declaring the State of Economic, Social and Ecological Emergency throughout the national territory 3. However, differences (heterogeneities) between urban and rural areas were not considered and, therefore, the rural population had difficulties adapting to mobility restriction measures and implementing remote work, suffering negative economic consequences as a result 2.
Colombia is one of the Latin American countries with the largest rural population 4. According to the Departamento Administrativo Nacional de Estadística (DANE), the estimate of inhabitants in rural areas in the country is 22.9% of the total estimated population of 48,258,494 5. Although Colombia, like most countries in the world, has undergone an important urbanization process, only 0.3% of the entire Colombian territory corresponds to urban areas 6 and 53% of the population is concentrated in rural territories or in the urban-rural interface 7. Although it has heterogeneous conditions, and despite the more than 130,000 deaths reported associated to the SARS-CoV-2 pandemic, and more than six million reported cases 8. preventive measures continue to be implemented homogeneously, without considering the virus dynamics in rural food-producing communities and in the urban-rural interface.
Based on the impossibility of implementing suppression (i.e., mobility restriction and remote work) or mitigation (i.e., interruption of activities) measures in rural food-producing communities, this work aims to model real areas in Colombia that have an intrinsic SARS-CoV-2 transmission dynamics in these populations. We characterize rural-urban interaction by means of different scenarios that allows us to identify interactions capable of preventing SARS-CoV-2 transmission in rural food-producing communities.
Materials and methods
Study area
Colombia has a population of approximately 50 million people, distributed in 32 administrative units called departments, with Bogotá, D.C., as the capital district. In this work, five departments were considered: Boyacá, Caquetá and Cundinamarca (dairy producer), Santander (poultry producer) and Sucre (fish producer) (table 1). In each department, the main urban center was considered, as well as the main neighboring rural municipalities associated with each production system (figure 1).
Table 1 Departments and municipalities considered as case studies.
Departament | Municipality | Population | Cases | Deaths | Departament | Municipality | Population | Cases | Deaths |
---|---|---|---|---|---|---|---|---|---|
Boyacá | Tunja | 179,263 | 8,473 | 110 (1.3%) | Cundinamarca | Zipaquirá | 146,352 | 5,268 | 126 (2.4%) |
Belén | 7,532 | 63 | 2 (3.2%) | Cajicá | 92,967 | 3,632 | 43 (1.2%) | ||
Boyacá | 5,118 | 26 | 0 (0.0%) | Cogua | 24,434 | 680 | 31 (4.6%) | ||
Chiquiza | 5,484 | 34 | 3 (8.8%) | Guasca | 16,934 | 113 | 2 (1.8%) | ||
Chivatá | 2,834 | 13 | 0 (0.0%) | La Calera | 32,917 | 594 | 12 (2.0%) | ||
Cómbita | 13,280 | 465 | 5 (1.1%) | Nemocón | 14,532 | 208 | 3 (1.4%) | ||
Cucaita | 3,787 | 32 | 1 (3.1%) | Pacho | 25,803 | 296 | 9 (3.0%) | ||
Duitama | 126,670 | 4,769 | 89 (1.9%) | Sopó | 28,999 | 630 | 16 (2.5%) | ||
Motavita | 5,703 | 65 | 4 (6.1%) | Subachoque | 16,743 | 166 | 5 (3.0%) | ||
Oicatá | 2,890 | 28 | 0 (0.0%) | Tabio | 24,206 | 489 | 8 (1.6%) | ||
Paipa | 34,679 | 855 | 16 (1.9%) | Tocancipá | 45,714 | 1177 | 18 (1.5%) | ||
Samacá | 18,818 | 444 | 12 (2.7%) Santander | Santander | Bucaramanga | 607,428 | 28,680 | 998 (3.5%) | |
Sora | 3,077 | 16 | 1 (6.3%) | Charta | 2,888 | 6 | 0 (0.0%) | ||
Soracá | 6,068 | 49 | 3 (6.1%) | El Playón | 14,038 | 110 | 11 (10.0%) | ||
Sotaquirá | 8,305 | 30 | 0 (0.0%) | Floridablanca | 307,896 | 10,352 | 421 (4.1%) | ||
Ventaquemada | 16,093 | 102 | 3 (2.9%) | Girón | 171,904 | 5,206 | 184 (3.5%) | ||
Caquetá | Florencia | 173,011 | 9,481 | 330 (3.5%) | Lebrija | 44,169 | 363 | 20 (5.5%) | |
Albania | 4,396 | 194 | 4 (2.1%) | Los Santos | 14,787 | 49 | 6 (12.2%) | ||
Belén de Andaquíes | 11,181 | 200 | 4 (2.0%) | Matanza | 5,035 | 31 | 1 (3.2%) | ||
El Doncello | 19,284 | 368 | 15 (4.1%) | Rionegro | 27,062 | 243 | 13 (5.3%) | ||
El Paujil | 18,464 | 261 | 10 (3.8%) Sucre | Sucre | Sincelejo | 293,951 | 11,085 | 414 (3.7%) | |
La Montañita | 14,692 | 205 | 3 (1.5%) | Corozal | 70,853 | 1,109 | 54 (4.9%) | ||
Milán | 9,952 | 107 | 4 (3.7%) | Coveñas | 19,516 | 506 | 6 (1.1%) | ||
Morelia | 3,747 | 77 | 6 (7.8%) | Morroa | 15,858 | 179 | 2 (1.3%) | ||
Puerto Rico | 26,282 | 496 | 15 (3.0%) | Palmito | 15,056 | 77 | 1 (5.8%) | ||
San Vicente del Caguán | 52,593 | 999 | 42 (4.2%) | Sampués | 48,819 | 468 | 27 (3.7%) | ||
Solita | 3,815 | 49 | 1 (2.0%) | San Onofre | 51,109 | 241 | 9 (1.5%) | ||
Valparaiso | 7,048 | 75 | 4 (5.3%) | Tolú | 34,117 | 329 | 14 (4.2%) |
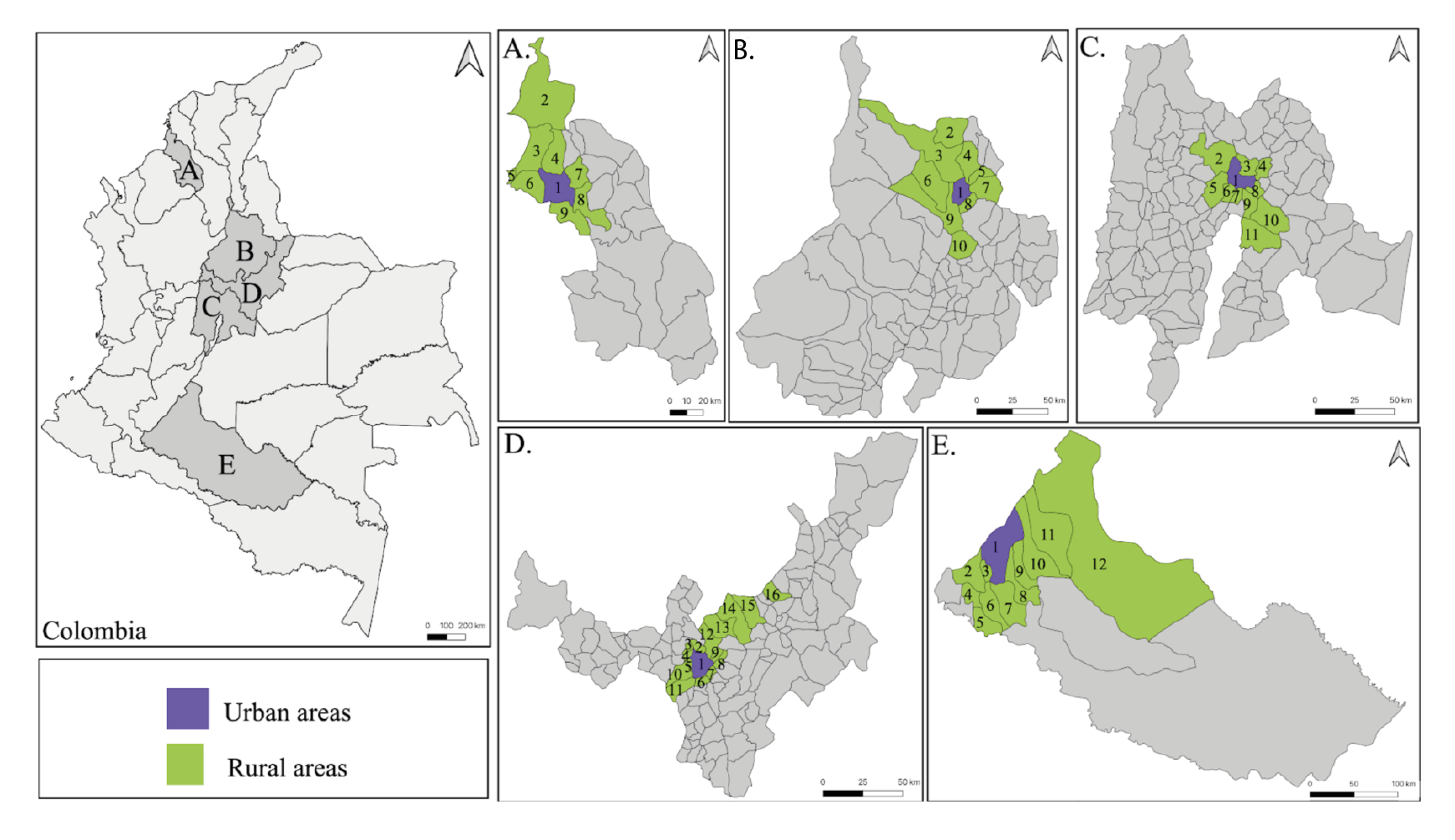
Figure 1 Study area: A) Sucre: 1-Sincelejo, 2-San Onofre, 3-Tolú, 4-Toluviejo, 5-Coveñas, 6-Palmito, 7-Morroa, 8-Corozal, 9-Sampués; B) Santander: 1-Bucaramanga, 2-Playón, 3-Rionegro, 4-Matanza, 5-Charta, 6-Lebrija, 7-Tona, 8-Floridablanca, 9- Girón, 10-Santos; C) Cundinamarca: 1-Zipaquirá, 2-Pacho, 3-Cogua, 4-Nemocón, 5-Subachoque, 6-Tabio, 7-Cajicá, 8-Tocancipá, 9-Sopó, 10-Guasca, 11-Calera; D) Boyacá: 1-Tunja, 2-Motavita, 3-Chiquiza, 4-Sora, 5-Cucaita, 6-Boyacá, 7-Soracá, 8-Chivatá, 9-Oicatá, 10-Samacá, 11-Ventaquemada, 12-Cómbita, 13-Sotaquirá, 14-Paipa, 15-Duitama, 16-Belén; E) Caquetá: 1-Florencia, 2-Belén, 3-Morelia, 4-Albania, 5-Solita, 6-Valparaíso, 7-Milán, 8-Montañita, 9-Paujil, 10-Doncello, 11-Puerto Rico, 12- San Vicente del Caguán.
Data
The data were obtained from the public reports of mortality due to SARS-CoV-2 reported by the Instituto Nacional de Salud of Colombia 8 from March 16, 2020, to December 31, 2020 8. As by date, a total of 42,909 deaths were reported, with Boyacá with 28,268 cases (232.20 per 100,000 inhabitants) and 609 deaths (lethality: 2.15%); 14,936 cases (371.68 per 100,000 inhabitants) and 529 deaths (lethality: 3.54%) in Caqueta; 66,254 cases (226.9 per 100,000 inhabitants) and 1,684 deaths (lethality: 2.54%) in Cundinamarca; 66,566 cases (304.7 per 100,000 inhabitants) and 2,361 deaths (lethality: 3.55%) in Santander; and 17,438 cases (192.8 per 100 thousand inhabitants) and 677 deaths (fatality: 3.88%) in Sucre.
Model
To understand the dynamics of SARS-CoV-2 infection in selected rural food-producing communities, an epidemiological model was considered, classifying individuals according to their economic activity (rural - R: development of activities exclusively in the rural area), urban - U: development of activities exclusively in the urban area) or urban-rural - UR: development of activities in the urban-rural interface) and their epidemiological status (susceptible - S: at risk of developing the disease); exposed - E: infected but not infectious); infectious - I: capable of transmitting the disease; recovered -R: recovered from the disease; or deceased - F: death due to the disease 9.
According to this classification, susceptible individuals residing in rural areas could be exposed to SARS-CoV-2 infection by developing activities at the urban-rural interface through contact with infected individuals residing in urban areas. Likewise, these individuals residing in urban areas could be exposed to the infection through contact with other infected individuals who also reside in urban areas or when carrying out activities in the urban-rural interface. Due to the low contact rate reported in rural areas 2, the model does not consider transmission of SARS-CoV-2 among people residing in rural areas. As shown in table 2, this model can be described by twelve different transitions (reactions) from the ten different states and transitions (figure 2).
Table 2 Events, reactions, and parameters of the SEIRF epidemiological model
Event | Reaction | Parameter |
---|---|---|
Exposure of a susceptible individual living in an urban area by contact with infectious individuals in an urban area. | Su → Eu | ϵ1 |
Exposure of a susceptible individual living in an urban area by contact with infectious individuals in the rural area at the urban-rural interface. | Su → Eu | ϵ 2 |
Exposure of a susceptible individual residing in a rural area by contact with infectious individuals in the urban area at the urban-rural interface. | Sr → Er | ϵ 3 |
Infection of an exposed individual living in an urban area. | Eu → Iu | β1 |
Infection of an exposed individual residing in a rural area. | Er → Ir | β 2 |
Recovery of an infectious individual living in an urban area. | Iu → Ru | Y1 |
Recovery of infectious individual residing in rural area. | Ir → Rr | Y2 |
Death of an infectious individual living in an urban area. | Iu → Fu | ð1 |
Death of an infectious individual residing in a rural area. | Ir → Fr | ð 2 |
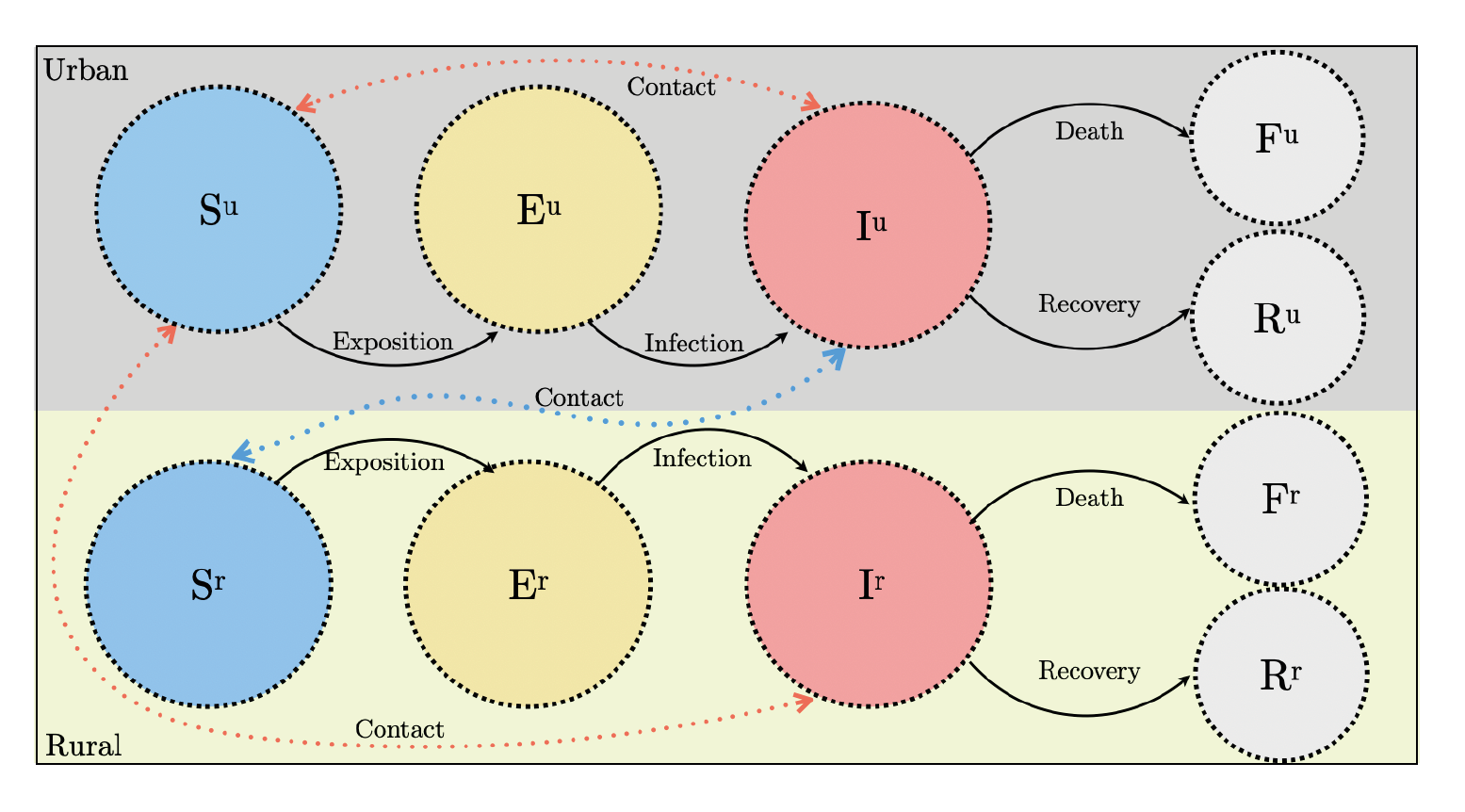
Su: Urban susceptibles; Sr: Rural susceptibles; Eu: Urban exposed; Er: Rural exposed; lu: Urban infected; Ir: Rural infected; Fu: Urban deaths; Fr: Rural deaths; Ru: Recovered urban; Recovered rural
Figure 2 Epidemiological SEIRF model considered for the different case studies. The states correspond to the circles and the transitions between the states are represented by arrows
Model fitting and scenario simulations
The epidemiological model was fitted to the number of deaths reported by the INS through the adjustment of the model parameters using a maximum likelihood function 9-11. For this adjustment, the number of initial susceptible individuals in each urban or rural municipality was considered according to the population size reported by the DANE 5. In this work, we used the maximum likelihood optimizer through the function "mle2" of the package "bbmle" of the computational language R (Bolker, 2020) to estimate this method for the model parameter 0 of each department.
In order to evaluate the impact of preventive measures focused on the urban-rural interface on rural areas, four scenarios associated with variations in the contact rate were considered 12,13 (ϵ = 70%, ϵ = 40%, ϵ = 20%, ϵ = 0%) between the individuals on this interface.
Results
Figure 3 shows the adjustment of the model to accumulated deaths in urban and rural areas reported in each department. This fit demonstrates the ability of the model to reproduce and predict the epidemiological profile of SARS-CoV-2 infection in urban and rural human populations. The list of parameters obtained because of the maximum likelihood function for each department considered in the case studies are found in table supplementary 1 of the supplementary material.
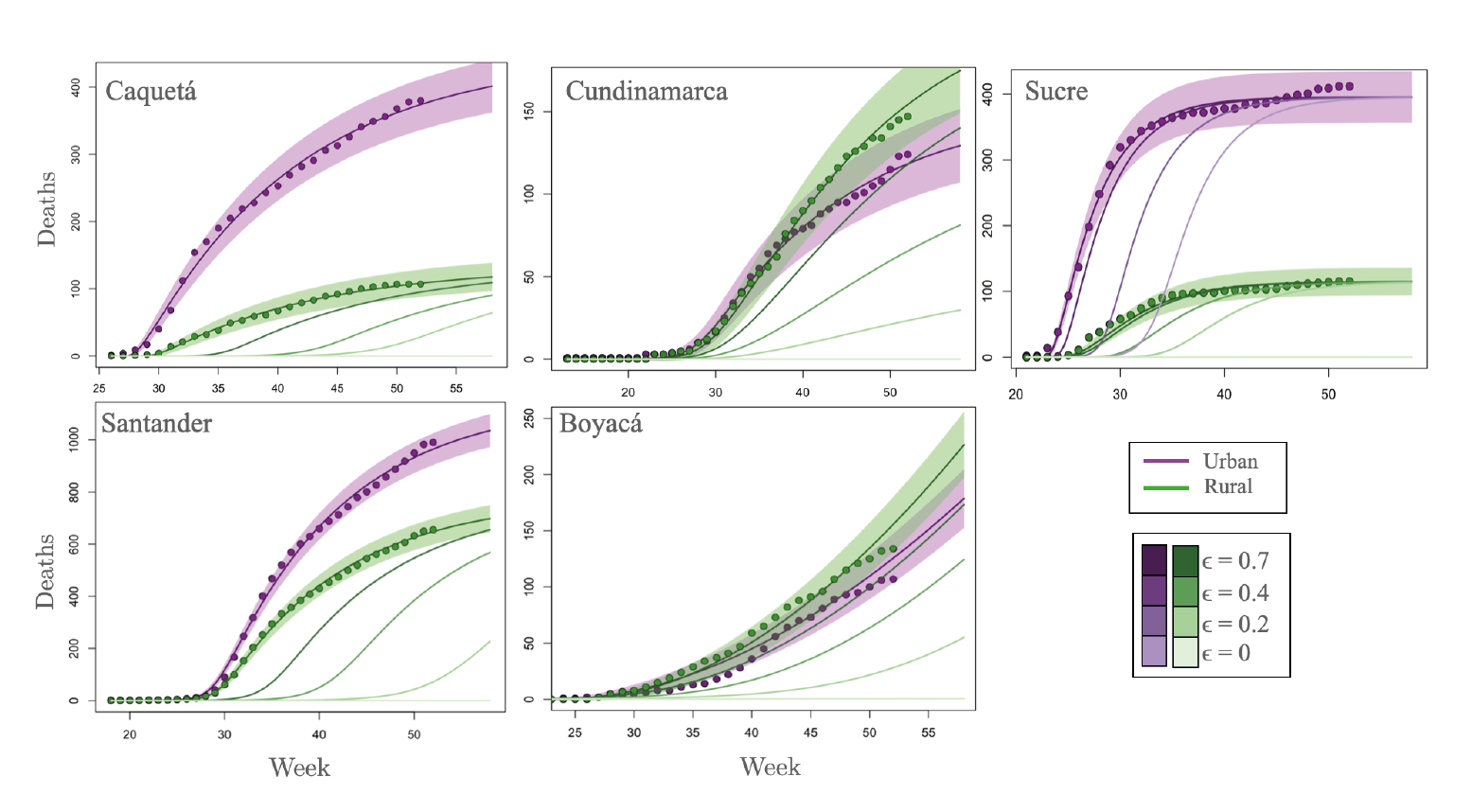
Figure 3 Model fitting to the number of deaths in each of the case study departments considering the selected municipalities of urban and rural areas.
Purple and green dots in figure 3 describe the cumulative number of deaths reported in urban and rural food-producing communities, respectively, while the lines indicate the it of the model to the actual data. There is a clear difference in the epidemiological profile of the pandemic in the different municipalities. Specifically, the irst epidemiological week, defined as the initial week in which the first infections were detected, it is different for each department, as can be observed in the x-axis of figure 3. Within the departments of Sucre and Caquetá, a difference in the first epidemiological week can also be observed for urban and rural populations. However, in Cundinamarca and Boyacá, departments with a high interaction between urban and rural communities, deaths occur simultaneously in rural and urban populations.
There is also a marked difference between the curves describing the accumulated deaths in urban and rural areas in Caquetá, Sucre, and Santander. However, in the departments of Cundinamarca and Boyacá, there is a very similar accumulated number of deaths for rural and urban areas. This difference in the case study departments is mainly because the coefficient that simulates the interaction in the urban-rural interface is markedly higher in Cundinamarca and Boyacá (table supplementary 1).
The importance of the interaction in the urban-rural interface becomes explicit when we consider its variation throughout four scenarios, namely: ϵ = 70%, ϵ = 40%, e = 20%, ϵ = 0%. The modulation of the parameter e counts for the implementation of different preventive measures focused on the urban-rural interface. As observed in figure 3, the preventive measures focused on the urban-rural interface impact the number of deaths in rural areas of all the considered departments, so it can be assumed that the dynamics of the disease in rural areas depend on connectivity (contact rate) at the urban-rural interface. Additionally, as shown in the case of Sucre, preventive measures focused on the urban-rural interface can delay the epidemic peak in urban areas.
Discussion
Our findings confirm that information about human mobility and connectivity should be a starting point for modeling important dynamic processes in human and animal epidemiology, population ecology, biology, and evolution 14-17. The urban-rural interface is essential for the development of economic activities associated with sustainability and food security 18, so it is essential to establish strategic and differentiated preventive measures for these communities. Preventive measures focused on the urban-rural interface may include: mandatory and appropriate use of personal protection items such as face masks 12,19,20; implementation of transportation systems/ schedules to avoid crowds, including movement restrictions for all family members 20-23; trade products exclusively in open places that social distancing and low contact rates with implementation of sinks and cleaning items that allow constant handwashing 24,25. These recommendations focused on the urban-rural interface will potentially impact the dynamics of the infection, reducing the number of deaths in rural communities.
In the context of a global health emergency, the contributions of epidemiological models are essential to expand the knowledge regarding transmission dynamics, identify patterns and individuals with greater susceptibility, and propose strategic measures that minimize the inevitable adverse health, economic, and social effects 26,27. The findings of the proposed model warn that highly connected areas, such as the urban-rural interface, are vulnerable to infectious outbreaks and can hardly be adapted to the preventive measures proposed worldwide. Measures focused on reducing connectivity or guaranteeing safe interactions in the urban-rural interface can be an effective mitigation strategy to avoid the geographical spread of diseases to rural areas.
Considering the little importance given to the interaction between the rural population, the implementation of preventive measures focused on rural areas is not of fundamental significance in terms of reducing the transmission of SARS-CoV-2. Therefore, people associated exclusively with food production systems do not need to be subjected to the preventive measures implemented by the national government, such as mobility restrictions. Therefore, these areas are exposed to SARS-CoV-2 when trading their products and conducting activities at the urban-rural interface. Thus, in order to reduce exposure to the virus infection, and prevent itstransmission to rural communities in Colombia, measures should focus on places with high transmissibility and risk for rural communities, such as the urban-rural interface.
Supplementary material
Table supplementary 1 List of parameters obtained because of the maximum likelihood function for each department considered in the case studies.
Departament | Parameter | Definition | Unity | Value |
---|---|---|---|---|
Sucre | ϵu | Exposure rate of individuals residing in urban areas. | día-1 | 1.4e-4 |
ϵr | Exposure rate of individuals residing in rural areas. | día-1 | 1.2e-5 | |
β | Infection rate. | día-1 | 0.27 | |
ρ | Recovery rate. | día-1 | 0.66 | |
μ | Death rate | día-1 | 8.9e-4 | |
Caquetá | ϵu | Exposure rate of individuals residing in urban areas. | día-1 | 2.1e-21 |
ϵr | Exposure rate of individuals residing in rural areas. | día-1 | 6.2e-4 | |
β | Infection rate. | día-1 | 7.6e-2 | |
ρ | Recovery rate. | día-1 | 0.99 | |
μ | Death rate | día-1 | 2.5e-3 | |
Santander | ϵu | Exposure rate of individuals residing in urban areas. | día-1 | 2.2e-12 |
ϵr | Exposure rate of individuals residing in rural areas. | día-1 | 5.1e-5 | |
β | Infection rate. | día-1 | 7.8e-2 | |
ρ | Recovery rate. | día-1 | 0.99 | |
μ | Death rate | día-1 | 1.9e-3 | |
Boyacá | ϵu | Exposure rate of individuals residing in urban areas. | día-1 | 6.8e-3 |
ϵr | Exposure rate of individuals residing in rural areas. | día-1 | 9.3e-3 | |
β | Infection rate. | día-1 | 4.5e-4 | |
ρ | Recovery rate. | día-1 | 0.79 | |
μ | Death rate | día-1 | 6.8e-2 | |
Cundinamarca | ϵu | Exposure rate of individuals residing in urban areas. | día-1 | 1.3e-4 |
ϵr | Exposure rate of individuals residing in rural areas. | día-1 | 4.2e-5 | |
β | Infection rate. | día-1 | 6.1e-2 | |
ρ | Recovery rate. | día-1 | 0.98 | |
μ | Death rate | día-1 | 1.0e-3 |