1. INTRODUCTION
Alzheimer’s Disease (AD) is the most common type of neurodegenerative dementia; it disturbs the interactions between neuron cells involved in the brain functions, isolating them [1], [2].
Communication and cognitive skills are affected in AD patients [3]. For instance, the production of language, coherent sentences, and capabilities to structure conversations are compromised [4], [5].
Language production, both spoken and written, shows deficient speech that usually contains a high number of words and verbal utterances with no coherent information, low density of ideas, and poor grammar [6], [7]. Conversation structuring is undermined by the scarcity of declarative sentences such as propositions. Additionally, patients use pronouns more frequently and have a hard time finding the right words for a sentence [8].
The process to diagnose AD is difficult and time-consuming. However, speech and language processing can help, and its first step is the automatic classification of AD patients and healthy controls (HC). For that reason, the interest of the research community in contributing to the AD detection process has increased in recent years.
In [9], the authors classified transcripts from 99 AD patients and 99 HC subjects from the Dementia-Bank dataset [10].
They extracted syntactic, lexical, and n-gram-based features for the classification [11]. The syntactic features included the number of occurrences of coordinated, subordinated, and reduced sentences per patient, number of predicates, and average number of predicates. The lexical features comprised the total number of utterances, average length of utterances, and number of unique words and function words, among others. The features were classified using a Support Vector Machine (SVM). The models were validated using a Leave-Pair-Out-Cross-Validation strategy.
They reported accuracy values of up to 93 % with the proposed features. The same database was used in [12], where the authors classified AD and HC participants using a model based on word embeddings. The word-embedding technique they used was based on the Global Vectors (GloVe) model, which considers the context of neighbor words and the word occurrence in a document [13]. Said authors considered a pre-trained model with the Common Crawl dataset, whose vocabulary size exceeds the 2 million and contains 840 billion words.
A logistic regression classifier and a Convolutional Neural Network with Long Short-Term Memory Units (CNN-LSTM) were implemented for the classification. The models were validated with a 10-Fold-Cross-Validation strategy, and the authors reported accuracies of up to 75.6 %.
In [14], AD patients in the Dementia-Bank dataset were classified using a Bag-of-Words (BoW) representation and a classifier based on neural networks. The parameters of the classifier were optimized following a Leave-One-Out cross validation strategy (LOO), and an accuracy of 91 % was reported. In [15], the authors used a hybrid model composed of Word2Vec (W2V) word-embeddings, Term Frequency-Inverse Document Frequency (TF-IDF) features, and Latent Dirichlet Allocation (LDA) topic probabilities [16], [17], [18]. In that case, the Dementia-Bank dataset was used along with the 2011 survey of the Wisconsin Longitudinal Study (WLS) [19].
They considered an SVM classifier with a linear kernel whose complexity parameter was optimized following a 5-Fold-Cross-Validation strategy. The authors reported an accuracy of 77.5 % and established that the most accurate features were those based on TF-IDF combined with the W2V model.
This study considers word-embedding features extracted from a W2V model trained with the latest data dump from Wikipedia (February 2019), along with TF-IDF features and grammatical features, in order to classify AD patients and HC subjects based on transcripts in the Dementia-Bank dataset. The results show that the W2V model, along with an early fusion of the three feature sets, is appropriate to model the cognitive impairments of the patients. Additionally, the results indicate that the grammatical features are suitable to identify HC subjects and AD patients. To the best of our knowledge, this is the first study that considers grammatical features to model language deficiencies exhibited by AD patients.
2. METHODS
2.1 Word Embeddings
The global coherence of the spontaneous speech of AD patients shows semantic, comprehension, and memory loss impairments. Semantic impairments include errors when naming objects or actions [6]. Contextual impairments result in incorrect categorical names for entities and incoherent information in sentences [20].
Memory impairments are reflected in the restricted vocabulary of AD patients and their difficulties to find appropriate words for sentences [6]. W2V considers the contextual relations between words and their co-occurrences in a transcript. In this study, we aim to detect the impairments mentioned above in AD patients using word embeddings extracted from a W2V model.
The words in the selected transcripts of the dataset are mapped into vectors that are positioned in a n-dimensional space according to their context. On the one hand, the closer the word vectors, the more related the words are in that context. On the other hand, the further the vectors are from each other, the less the words are related in that context. Such relationships are illustrated in (Fig. 1).
The vocabulary size is v. The Y words of the context {Y0,Y1, …, Yc} are the one-hot encoded inputs of the {X0, X1, …, Xv} neurons at the input layer. The hidden layer has h n neurons, where n is the dimension of the W2V model. The output layer has O v neurons. The values {O0, O1, …, Ov} are used to predict the most probable word for the input context word W. This process is shown in (Fig. 2). Said model was trained with the latest Wikipedia data dump (February 2019).
The vocabulary size of the model is over 2 million and it has over 2 billion words. The Gensim topic modeling toolkit was used to develop the W2V model [21]. Default parameters were used unless specified. The feature extraction process consists of four steps: 1) The stop words are removed from the documents using the English stop words dictionary available in the Natural Language Toolkit (NLTK) [22]. 2) The W2V model is trained with the processed text corpus, with 300 hidden units, and a context of 10 words. 3) The word vectors are extracted from all the selected documents in the Dementia-Bank dataset. 4) Four statistical functionals are computed for the word vectors extracted from each transcript: average, standard deviation, skewness, and kurtosis. Thus, a 1200-dimensional feature vector was formed per transcript.
2.2 Term Frequency-Inverse Document Frequency
The language production impairments exhibited by AD patients also include a high number of non-coherent repetitions and sentences [6]. TF-IDF features represent the relevance of each word in a document, averaged by its global importance in the whole dataset [17]. The objective of TF-IDF features is to model the vocabulary of the patients and the relevance of each word in their transcripts.
On the one hand, Term Frequency (TF) features of each word in a document are obtained as the ratio between the number of times that the word appears in a document and the total amount of words in said document, according to (1). On the other hand, Inverse Document Frequency (IDF) features of each word are calculated as the logarithm of the total quantity of documents divided by the number of documents that contain that word, according to Expression (2). In (1), TF(ω,t) is the TF feature associated with word ω in the transcript t, and f (ω,t) is the frequency of ω in t. In (2), IDF ω is the IDF feature of each word ω, T is the total number of transcripts, and T ω is the total number of transcripts where ω is present.
The TF-IDF feature of each word ω is given by (3), and it is the result of computing the product of (1) and (2); this was done for each word ω in the transcripts. A 1408-dimensional feature vector was calculated per transcript; such dimensions were given by the vocabulary size of the Dementia-Bank dataset.
2.3 Grammar features
The feature sets studied in this work are inspired by clinical evaluations to assess the neurological state of AD patients. Additionally, we propose grammar features to model the sentence structuring capabilities of AD patients, who show deficits in using nouns and verbs[23]. Moreover, AD patients have problems to use verbs when arguments are involved [24]. The goal of such grammar features is to assess the sentence structuring capabilities of AD patients by counting the elements involved in the structuring of sentences and the number of grammatical elements (such as verbs and nouns) contained in their transcripts.
Eight grammar features were used with their corresponding equations: Readability of the transcript calculated with the Flesch reading score (FR) (4), Flesch-Kincaid grade level (FG) (5), propositional density (PD) (6), and content density (CD) of the transcript (7). The FR score indicates the educational attainment a person needs to easily read a portion of text, ranging from 1 to 100. A score between 70 to 100 means the text is easily readable by a person without specialized education. A score below 30 indicates that a text requires effort and a higher education to be read [21]. The FG measures writing skills, and ranges from 0 to 18. A score of 18 means a very complex and well-structured text. FG scores below 6 indicate a barely elaborated text [21]. PD measures the overall quality of propositions in a text.
In turn, CD quantifies the amount of useful information in a transcript. The constants in (4) and (5) are defined as standard for the English language. The feature set is completed with Part-Of-Speech (POS) counts: Noun to Verb Ratio (NVR), Noun Ratio (NR), Pronoun Ratio (PR) and Subordinated to Coordinated Conjunctions Ratio (SCCR) (8), (9), (10) and (11) [25].
The selected POS counts measure the quality of the syntactical abilities of AD patients when structuring sentences.
2.4 Data
The clinical Pitt Corpus from the Dementia-Bank dataset was used in this study [10]. The data are the result of a longitudinal study on AD conducted by the University of Pittsburgh School of Medicine. It contains the transcripts of spontaneous speech from HC subjects as well as individuals who possibly and probably have AD. The acquisition of such data involved annual interviews with participants, who described the situations occurring in the Cookie Theft picture (Fig. 3), which is part of the Boston Diagnostic Aphasia Examination.
The participants’ verbal utterances in English language were recorded and transcribed.
This study considers data from 98 individuals from the AD group and 98 from the HC group. Participants mentioned their age during the first interview.
(Table 1) shows the demographic and clinical information of AD patients and HC. (Fig. 4) is a histogram of their Mini-Mental-State-Examination (MMSE) scores with the corresponding probability density distribution of both groups. (Fig. 5) presents the box-plot, histogram, and the probability density distribution of the age of both groups.
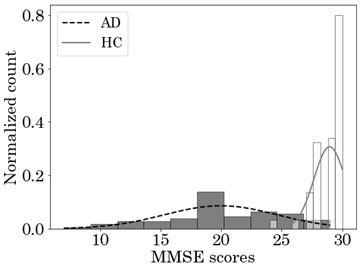
Source: Created by the authors.
Fig. 4 Histogram of MMSE scores and probability density function of the AD and HC groups
3. EXPERIMENTS, RESULTS, AND DISCUSSION
3.1 Classification
The feature sets were classified in four experiments using three different methods: SVM, Random Forest (RF), and K-Nearest Neighbors (KNN). Scikit-learn was used to classify the proposed models [26]. Default parameters were used unless specified.
A Leave-One-Out Cross-Validation strategy was followed, and the hyper-parameter optimization was performed via exhaustive grid-search, according to the accuracy obtained in the development set. The objective of such validation strategy is to compare our results with previous studies, such as [14]. The range of the hyper-parameters evaluated in the training process is shown in (Table 2). One speaker was used for testing; the rest were divided into 9 groups. Eight groups were used for training, and one group was employed for the hyper-parameter optimization.
3.2 Experiments and Results
Four experiments were conducted. The results of the three classifiers are reported for each experiment. The accuracy (ACC), sensitivity (SEN), specificity (SPEC), and the area under the Receiver Operating Characteristic curve (AUC) of the three classifiers are listed in Table 3.
Table. 3 Results obtained with different feature sets and classifiers to identify AD patients and HC subjects
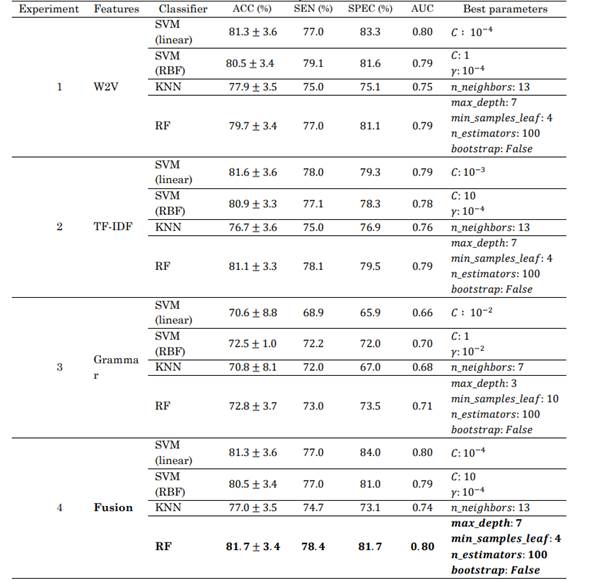
Source: Created by the authors.
Table. 4 Comparison of p-values (obtained with the Mcnemar test) between the best results of experiments 1-3 and experiment 4
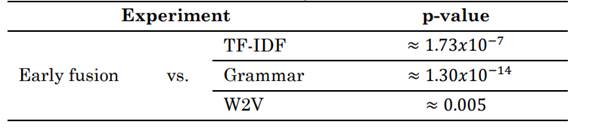
Source: Created by the authors.
Experiment 1: W2V features were considered. The best classifier in this experiment was SVM with a linear kernel (ACC = 81.3 %). The results of the Mcnemar test show a significant difference between the best result and those obtained with other classifiers (p<<0.02).
Experiment 2: TF-IDF features were considered. In this case, the best classifier was the SVM with linear kernel (ACC = 81.6 %), and the results of the Mcnemar test also show a significant difference between the best result and those obtained with the other classifiers (p<<0.001).
Experiment 3: Grammar features were considered. The best classifier for this feature set was RF (ACC = 72.8 %). The results of the Mcnemar test show a significant difference between the best result and those obtained with the other classifiers (p<<0.01).
Experiment 4: The early fusion of the feature sets was considered. In this case, the best classifier was RF (ACC = 81.7 %). Once more, there is a significant difference between the best results and those obtained with the other classifiers (p-value approx. 10(-12)).
A statistical comparison between the best results obtained with the early fusion strategy and those obtained with each feature set reveals significant differences. Table 4 shows the p-values obtained in the experiments.
3.3 Discussion
According to the results, the model based on the combination of the three feature sets and the RF classifier is the most accurate to classify AD patients and HC subjects. The values obtained with the linear SVM indicate that most of the extracted features are linearly separable. The accuracy obtained with this classifier ranges from 78.3 % to 85.1 %. The TF-IDF and W2V models exhibited similar results in general. According to the high and balanced values of specificity and sensitivity, and in spite of the high values of the MMSE scores of several AD patients, the proposed approach seems to be accurate and robust. Additionally, the grammar features are highly accurate (72.8 %) and effective. The reduced number of features in the grammar set indicates that this approach is suitable and promising.
It is important to highlight the results of the statistical information of all the experiments. There is a weak statistical relationship between the predictions of all the classifiers in the experiments, which means that the errors and correct predictions of each classifier were different. In experiment 4, the classifiers exhibit the weakest statistical relationship as a result of the early fusion of the feature sets. The accuracy values obtained using the early fusion strategy show improvements in the RF classifier, which is the most benefited with the combination of feature sets. SVM and KNN classifiers showed no significant improvement in performance compared with experiments 1 and 2.
The results of this study can be directly compared to those in [9] and [14], since we adopted the same cross-validation strategy. These results, are slightly lower than those in related studies, however, those reported there could be optimistic, since the features extracted in the BOW model and n-gram models were computed with information of the vocabulary of the test set. In a more realistic clinical environment, a more general feature set, such as W2V, is preferred. TF-IDF features showed an important role in modeling the difficulties of AD patients to find appropriate words. In addition, grammar features proved to be an alternative to detect, without a complex feature extraction process, AD patients’ impairments to structure sentences with useful information.
4. CONCLUSIONS
This study used to word-embed features (i.e., statistical functional, TF-IDF features, and grammar features) to classify AD patients and HC subjects in the Pitt Corpus of the Dementia-Bank dataset employing different classifiers. A total of 1200 word-embedding features, 1408 TF-IDF features, and 8 grammar features were computed based on the transcripts in the dataset. Each feature set was classified separately. An early fusion strategy of the three feature sets was also considered.
The language impairments of AD patients were successfully modeled using the proposed methods. TF-IDF features modeled the deteriorating vocabulary and low word relevance in the transcripts of AD patients. Semantic, comprehension, and memory loss impairments of AD patients were modeled with W2V features.
The sentence structuring capabilities of AD patients were modeled with grammar features. All the models achieved high accuracies in the automatic discrimination between AD patients and HC subjects. The models obtained from the W2V feature set and the TF-IDF feature set showed a similar performance, although the early fusion strategy contributed to a better model. The early fusion achieved accuracies of up to 81.7 %. When only grammar features were considered, the proposed approach exhibited accuracies of up to 72.8 %. The features based on the W2V model showed a significant importance as the embeddings were extracted from a non-specialized knowledge database rather than the classification dataset. TF-IDF features were extracted directly from the transcripts used in this work, and they have a higher dimensionality than the W2V model. Grammar features were found to be important and produced promising results without the need of complex calculations in the extraction process.
We believe that further experiments can be designed to identify the most suitable features for clinical evaluations.
Statistical differences between the classifiers were found in all the experiments. This suggests that the experiments that use an ensemble or stacking techniques could produce better results. Experiments with deep learning techniques, a bigger dataset to retrieve TF-IDF features, a larger word vector dimension, and a considerably larger set of grammar features are needed in future work. Additionally, word embeddings of novel language models based on more sophisticated neural network architectures, such as BERT and XLNET, could lead to better results because such models have achieved state-of-the-art performance in numerous NLP tasks [27], [28], [29]. Further research is required with the aim of finding possible clinical interpretations to the results based on these kinds of models.