1. INTRODUCTION
Firms are facing environments that are increasingly competitive, which has forced them to develop innovations. However, when they do not have all the resources to innovate themselves, they find it necessary to associate with third parties so that these third parties can provide them with knowledge (Xie et al., 2013). In order to carry out the desired innovations, firms seek to develop market networks that are made up of many parties, including their customers, suppliers, competitors, consultants, and headquarters. At the same time, they also interact with institutional networks, whose actors are universities and research centers.
The connection between market networks and technological innovation has been studied from a variety of different angles, each leading to its own conclusions. Liu and Atuahene-Gima (2018) indicate that connections with customers encourage firms to promote product innovation. In a similar way, firms that connect with suppliers of technology are in a better state to develop process innovations (Najafi- Tavani et al., 2018), and Ozdemir et al. (2017) argue that firms seeking alliances with their competitors manage to deepen their knowledge and to improve their new product development processes; at the same time, they are abler to explore new market opportunities. Furthermore, when the headquarters becomes involved in the process of innovation that is carried out in the subsidiaries, these subsidiaries acquire the skills necessary to develop innovations (Ciabuschi et al., 2015). External consultants are also considered favorable for the development of firms’ innovation capacity (Back et al., 2014). Firms that develop the ability to connect to collaboration networks are more likely to develop product innovations (Ghauri et al., 2016).
Meanwhile, research on institutional networks and their relationship with technological innovation has interested scholars. Kim et al. (2018) investigated Korean manufacturing firms and found that firms’ alliances with universities and research institutes allow those firms to have access to external sources of knowledge that enhance their capacity for innovation. Additionally,, Seo et al. (2017), analyzing the information from the 2012 Korean innovation survey, verified how obstacles can influence the innovation capacity of low-tech firms, finding that there may be differences that are conditioned by the presence of a strong or weak appropriability regime. The obstacles firms face when they evaluate whether or not to carry out innovations fall mainly into four categories: knowledge, market, cost, and finances, as well as other factors (INEI, 2015).
This study contributes to the literature on innovation carried out by low-tech firms in an emerging economy (Del Carpio & Miralles, 2018). Manufacturing firms are classified according to an indicator that is calculated by dividing the research and development expenses made by firms by their sales, according to the OECD (Galindo-Rueda & Verger, 2016). Low-tech firms, according to Martínez et al. (2017), are characterized by the search for practical knowledge, and they acquire mature technologies and adapt them to their context. They depend on the acquisition of machinery and external knowledge.
On the one hand, low-tech firms are forced to apply the open innovation approach, which, according to Bayona-Saez et al. (2017), is based on the search for external knowledge to improve their innovation capacity. On the other hand, Zouaghi et al. (2018) points out that low-tech firms that are highly dependent on external knowledge are always in search of connections with customers, suppliers, competitors, and universities, which will help improve their processes of innovation and overcome resource constraints.
It should be noted that there is little research on innovation in Latin American countries, especially Peru (Tello, 2017). Most research on this topic is carried out in the most developed countries (Hervas-Olivere et al., 2011), and it is clear that the results obtained from this research cannot be extrapolated to Latin American emerging economies (Crespi et al., 2019) due to the fact that they are in a different context, one that includes factors such as the presence of high levels of corruption; economies with a sizable informal sector, which discourage formal firms from developing innovations (Pérez, 2015); and the lack of financial resources destined for the development of innovations (Fernández, 2017).
In recent years, interest in research on innovation has been raised in manufacturing firms in Peru. Thus, Huang et al. (2019) point out that these firms face, among others, the following obstacles: little access to advanced technology, scarce financial resources, poor resource management and few qualified workers. Pérez et al. (2019) propose a theoretical model, which allows one to analyze what factors favor the development of the innovation capability of Peruvian manufacturing firms. Also, Cabrera & González (2019) specify that manufacturing firms interact with external sources of knowledge to improve their capacity for innovation; meanwhile, Heredia et al. (2019) sustains that the managers of manufacturing firms develop strategies that are oriented towards reducing costs. Likewise, Ortigueira-Sánchez et al. (2020) investigate what factors favor the development of the innovation capacity of Peruvian manufacturing firms. Meanwhile, Seclen-Luna, Regalado & Cordova (2020) indicate that the Peruvian government is financing innovative projects that improve the innovation capability of Peruvian manufacturing firms.
The structure of this study is as follows: after this introduction, the theoretical framework is presented, and the hypotheses are formulated. The third section deals with methodology and gives a description of the data, the definitions of the variables, and an explanation of the statistical procedures that were conducted. The fourth section shows the results so that the fifth section can present the discussion of the results. Finally, the conclusions, limitations of the study, and future issues to research are stated.
2. THEORETICAL FRAMEWORK AND HYPOTHESES
Low-tech firms carrying out their activities in Latin American economies face financial resource limitations that hinder them from carrying out innovation activities (Fernandez, 2017). For this reason, these firms often implement the open innovation approach, which forces them to develop stronger connections with customers, suppliers, competitors, and universities (Bayona-Saez et al., 2017).
On the other hand, Sarpong and Teirlinck (2018) conducted an analysis of small businesses in Belgian firms that were facing enormous obstacles. They found that these firms are more likely to seek out connections with their market networks, that is, with customers, suppliers, or competitors, as well as with their institutional networks, made up of universities and research institutes.
2.1 The relationship between market networks and product innovation in low-tech firms
The literature includes diverse studies that show how firms connect with their customers, suppliers, competitors, consultants, and headquarters and how they carry out product innovations. Estrada et al. (2016) analyzed Flemish firms that collaborated with competitors and found that this helped them improve their ability to innovate products, when knowledge protection mechanisms were assured. Additionally, Xie and Li (2017) found that Chinese vehicle manufacturers that export and face a competitive domestic market are more likely to carry out product innovations, and Najafi-Tavani et al. (2018) found that, for Iranian manufacturing firms, collaborative networks improve product innovation capacity, especially when the firm has developed absorptive capacity.
On the other hand, Sheng et al. (2015), who analyzed Asian firms, developed a theoretical framework to show the positive relationship between the subsidiary firm’s tacit knowledge and the ability of the headquarters to develop product innovations. The hiring of external consultants allows firms to improve their innovation capability, even when they do not invest much in research and development, which is one of the characteristics of low-tech firms (Bianchi et al., 2016).
Furthermore, Jajja et al. (2017) researched how firms, through the development of very close relationships with their suppliers, were thus able to generate more knowledge; this had a strong impact on their ability to develop product innovations. Niammuad et al. (2014) show that product innovation is a fundamental activity for recently-formed firms that have had the help of an incubator, which exposes incubated firms to market networks to be able to develop product innovations. Finally, Ghauri et al. (2016) make the case that if firms develop the ability to connect to networks, it is easier for them to develop product innovations.
Latin American firms also link up with market networks to develop technological innovations. In that line, Solleiro & Castañón (2005) analyzing the competitiveness of the Mexican economy, found that firms develop innovations through vertical relationships with customers and suppliers. Also, Goedhuys & Veugelers (2012), studying Brazilian manufacturing firms, found that firms that develop technological innovation establish collaborative links with clients, suppliers, or universities.
Regarding innovation studies in Peruvian manufacturing firms, it has been identified that Nuruzzaman & Singh (2019) analyzing the information of firms from several South American countries, including Peru, that the interaction between firms and their customers increases quality of product innovations. On the other hand, Córdova-Aguirre & Ramón-Jerónimo (2021) analyzing Arequipa firms dedicated to the plastics industry, confirmed findings that these firms develop product innovations to meet the needs of their customers.
As can be seen, manufacturing firms that collaborate with their customers, suppliers, competitors, consultants, and headquarters will be enabled to develop product innovations. These arguments allow the following hypothesis to be proposed:
H1: There will be a positive association between market networks and product innovation in low-tech firms.
2.2 The relationship between institutional networks and product innovation in low-tech firms
Institutional networks made up of universities, public research institutes, and private research institutes promote the development of product innovation. The literature includes many studies that demonstrate this relationship. For example, Vega-Jurado et al. (2017) analyzed how the relationships between firms and universities favor the firms’ acquisition of knowledge, which leads to a focus on open innovation. This generated knowledge encourages firms to develop product innovations.
Additionally, Tang et al. (2019), analyzing a sample of 166 Chinese manufacturing firms, found that firms that partner with universities are more likely to develop either radical or incremental product innovations. Physical proximity of the firms to the universities is a fundamental aspect in said collaboration, as is these universities’ willingness to partner with the firms. More directly, Kobarg et al. (2018) examined the relationships established between firms and universities and noted that universities, through their technology transfer ofices, conduct research whose results favor the development of product innovations in firms. Also, Medda (2020) found that interactions between firms and universities favored the development not only of product innovations, but also of process innovations.
In the same vein, Robin & Schubert (2013) analyzed data from a community innovation survey in Germany and France and found that the relationship between firms and public research institutions promoted the development of product innovations. More recently, Szucs (2018) argued that the academic quality of the university is a determining factor when a firm seeks to partner with it in order to improve its innovation capability.
If the emerging economies are considered, it can be found that Bustamante (2018) analyzing a sample of more than 5000 Chilean firms that participated in the national innovation survey, found that firms that are linked to universities are more likely to innovate. Also, Guerrero, Urbano & Herrera (2019) analyzing the alliances between Mexican universities and firms, found that these collaborations favor the development of product and process innovations.
When Peruvian manufacturing companies are analyzed, mixed results were found. Thus, López-Mendoza & Mauricio (2020) analyzed how universities seek to interact with firms to carry out technology transfer projects, allowing them to improve the satisfaction of the firm’s customers, by receiving better products. While Carvache-Franco et al. (2020), analyzing Peruvian firms, found a contradictory result, that is, firms that interact with their consultants are less likely to develop product innovations.
Therefore, the following hypothesis can be presented:
H2: There will be a positive association between institutional networks and product innovation in low-tech firms.
2.3 The relationship between market networks and process innovation in low-tech firms
Market networks, which include customers, suppliers, and competitors, promote the development of process innovation. Many studies show how market networks are related to process innovation, including a study by Najafi-Tavani et al. (2018), who researched Iranian manufacturing firms and found that collaborations in innovation networks, especially those connections between firms and their customers, suppliers, and competitors, favor the development of process innovations. In that same line, Aliasghar and Chetty (2019) analyzed the importance of process innovations for a manufacturing firm, pointing out that its development allows the firm to improve product quality, reduce costs, and improve the long-term competitiveness of the firm. Furthermore, Tomlinson and Fai (2016) evaluated the impact of the firms’ connections with their suppliers, finding that these interactions favor the development of process innovations. Likewise, Ayoub et al. (2017), analyzing the interactions between the members of a supply chain, found that these interactions favor firms’ process innovation. Customers and suppliers can contribute ideas and knowledge, and, in this way, the firms can improve their product design, their production process, and product delivery. The long-term development of interactions between the firm and its customers and suppliers favors the development not only of product innovation but also process innovation.
Meanwhile, Ciabuschi et al. (2015) argue that when the headquarters is involved in the innovation process that is being carried out at the subsidiaries, the subsidiaries acquire the competencies necessary to develop innovations, while Back et al. (2014) state that, in an emerging market, external consultants are important to helping generate innovation initiatives. Simon et al. (2012) state that innovation helps a firm satisfy its customers; this customer satisfaction provides all the more motive for firms to develop process, organizational, and marketing innovation. Finally, Un and Asakawa (2015) analyzed how cooperating with suppliers in research and development made for a greater impact when firms carried out their process innovations.
Collaboration between market networks and manufacturing firms improve its innovation capability. In that line, Corredor, Forero, & Somaya (2015), analyzing the Colombian manufacturing companies that participated in the innovation survey year 20024, found that companies that are linked with customers, suppliers and competitors are more likely to develop innovation. On the other hand, Pereira, Borini, & Oliveira Jr (2019) investigated how Brazilian firms link with suppliers, customers, and competitors to improve their capacity for innovation in processes, especially with suppliers, to exploit new technologies.
When Peruvian manufacturing firms are analyzed, mixed results were found. Thus, Carvache-Franco et al. (2020), analyzing Peruvian firms, found that firms that interact with their customers, suppliers and competitors are more likely to develop innovations in process. And, on the other hand, Nolazco (2020) studied Peruvian manufacturing firms, finding that interactions with customers, suppliers and competitors favor process innovations.
Based on the aforementioned, the following hypothesis can be proposed:
H3: There will be a positive association between market networks and process innovation in low-tech firms.
2.4 The relationship between institutional networks and process innovation in low-tech firms
Institutional networks made up of universities and public and private research institutes promote the development of process innovation. Institutional networks have captured the attention of scholars, including Ueasangkomsate and Jangkot (2017), who analyzed small and medium-sized food manufacturing firms in Thailand and found that when universities collaborated with the firms, carrying out technology transfers or joint research and development projects, or offering training to the firm’s workers, it benefited the firm by improving its capacity for product and process innovation. Furthermore, Un and Asakawa (2015) argue that when universities collaborate with firms to develop product innovations, they can also develop process innovations along the way, especially when the firms are commissioned by the universities to research a technology that will allow those firms to improve their production processes.
Tsinopouloset et al. (2018) analyzed community surveys carried out in the United Kingdom between 2004 and 2010 and found that although it is true that universities’ main economic activity is the training of future professionals, it can also be stated that when universities interact with firms, they are more likely to discover innovations in process. Moreover, Aliasghar et al. (2019), analyzing the Iranian auto industry, found that universities can help firms develop process innovations through the transfer of knowledge.
González-Pernía et al. (2015) indicate that the universities’ partnering role with firms is not clear but that this relationship stimulates product and process innovation. In this same sense, Maietta (2015) states that firms in the food and beverage industry seek out partnerships with universities and public research institutes in order to receive transfers of experience and knowledge that then allow them to improve their process innovation capability.
Relations between firms and universities are not very frequent in emerging economies. Those being studied by Araneda-Guirriman, Pedraja-Rejas & Rodríguez Ponce (2015) analyzing Chilean firms, conclude that universities play an important role in the training of qualified personnel, and generation of knowledge, that helps firms improve their capacity for innovation in products and processes. Thus, as also by de Moraes Silva, Furtado & Vonortas (2018), investigating Brazilian firms, identified that firms that carry out innovations in the process are more likely to link with universities and research institutes to develop projects that solve problems of a technological nature.
With regard to Peruvian firms, it is important to point out that universities are generators of technological knowledge that helps firms develop innovations (Arenas & González, 2019). While Carvache-Franco et al. (2020) analyzing Peruvian firms found a contradictory result, that is, firms that interact with their consultants are less likely to develop process innovations.
According to the aforementioned, the following hypothesis can be proposed:
H4: There will be a positive association between institutional networks and process innovation in low-tech firms.
2.5 Obstacles and their relationship to technological innovation in low-tech firms
Community innovation surveys of the manufacturing industry have always asked questions regarding the level of importance of the obstacles that firms face while attempting to carry out innovations (Leoncini, 2016). Ng and Kanagasundaram (2017), analyzing Malaysian furniture manufacturing firms, found that these firms face obstacles such as excessive risks, the excessive expense of carrying out innovation activities, lack of qualified personnel, uncertainty regarding demand, lack of infrastructure, and more. These factors discourage firms from developing technological innovations.
Blanchard et al. (2013) analyzed the fourth French community innovation survey; they focused on financial and non-financial obstacles in order to evaluate their impact on the propensity for firms to innovate, and they found that non-financial obstacles dissuaded firms from innovating while financial obstacles inhibited firms from implementing their innovations.
As can be seen, obstacles to innovation and their relationship with firms’ innovation capability have attracted attention. Indeed, Pellegrino and Savona (2017) studied how firms in the UK face a series of obstacles, including those of a financial nature, the lack of qualified personnel, and market entry barriers, which make it more dificult for firms to carry out activities that allow them to introduce product or process innovations. Research along these lines has been carried out in emerging economies, too, including a study by Karahan and Karhan (2013), who researched obstacles that impeded Turkish firms from carrying out innovations. Also, Santiago et al. (2016) analyzed Mexican firms to determine which financial and non-financial obstacles limited these firms’ innovation capability and suggested that policymakers focus on those factors that affected firms’ interest in innovating.
Manufacturing firms in Latin America also face obstacles to making innovations, in this vein, Vega-Jurado, Juliao-Esparragoza, Paternina-Arboleda & Vélez (2015) analyzing a case study of a Colombian agroindustrial company, found that its employees faced obstacles, such as the lack of resources and qualified personnel, when they carry out innovations. Moreover, Pérez, Geldes, Kunc & Flores (2019) made a study of the innovative behavior of manufacturing firms in Chile and Peru, finding that Chilean firms faced greater financial barriers to make innovations than Peruvian firms. In relation to Peruvian firms, we will mention that Huang et al. (2019) that Peruvian manufacturing firms face, among others, the following obstacles: little access to advanced technology, scarce financial resources, poor resource management, and few qualified workers. Meanwhile, Tello (2021) maintains that Peruvian manufacturing firms face some obstacles, which are complementary to each other, affecting the firm´s innovation capability.
Keeping in mind the aforementioned, the following hypothesis is posited:
H5: There is a significant relationship between the obstacles (that firms face when making innovations) and technological innovation in low-tech firms.
The Figure 1 shows the proposed theoretical model and the causal relationships among the variables.
3. METHODOLOGY
Next, the methodology used in this study is given. It consists of the description of the dataset; the definition of the dependent, independent, and control variables; and, finally, the methods used to analyze the data.
3.1 Data
The population in this study is comprised of low-tech Peruvian manufacturing firms. The units of analysis were the Peruvian manufacturing firms that participated in the 2015 National Survey of Innovation in the Manufacturing Industry. This survey was carried out by the National Institute of Statistics and Informatics (INEI). The fieldwork produced1,452 surveys carried out over a period of three consecutive years (from 2012 to 2014). The analysis used information that belongs to year 2014. In the survey, 720 firms are classified as low-tech firms (See Table 1). It was considered a sample of 705 manufacturing firms, where the remains present missing values. These firms were considered low-tech, which means that they invested less than 1.0 percent of their sales revenues in research and development (Organization for Economic Cooperation and Development, OECD, 2007). These firms belong to the following industries: Manufacturing, recycling; wood, pulp, paper, paper products, printing and publishing, food products, beverages, and tobacco, textiles, textile products, leather, and footwear. Table 1 presents the descriptive statistics of the firms included in the sample. They have been classified according to their age and size.
Table 1 Number of firms according to their technological intensity.
Technological Intensity | Number | Relative frequency |
High | 54 | 3.7% |
Medium-high | 271 | 18.7% |
Medium-low | 407 | 28% |
Low | 720 | 49.6% |
Total | 1452 | 100% |
Firm age | ||
Old (≥ 44 years old) | 63 | 9% |
Young ( 25 years old) | 552 | 78% |
Intermediate (from 26 to 43 years old) | 90 | 13% |
Total | 705 | 100% |
Firm size | ||
Small (50 employees) | 245 | 35% |
Medium (51 to 250 employees) | 271 | 38% |
Large (≥251 employees) | 189 | 27% |
Total | 705 | 100% |
Source: INEI, 2015
3.2 Variables
There are two dependent variables: product innovation and process innovation. Product innovation is a continuous variable and occurs when a firm declares that it has introduced completely new goods or services or those with important modifications. Applying factor analysis two variables were obtained. The first one for the following items: (1) new good, (2) new service. And, for the second variable, the following items: (3) significantly improved good, and (4) significantly improved service. Applying factor analysis this variable is obtained.
Process innovation is also a continuous variable. It occurs when the firm indicates that it has introduced some significant modifications to the process of production, marketing, logistics, or distribution. Applying factor analysis two variables were obtained. The first one for the following items: (1) new production method for goods or services; (2) new method for the logistics, distribution, or delivery of supplies, goods, or services; (3) new activity that supports production, like maintenance, acquisition, accounting, or IT systems. And, for the second variable, the following items: (4) significantly improved production method for goods or services; (5) significantly improved method for the logistics, distribution, or delivery of supplies, goods, or services; and (6) significantly improved activity that supports production, like maintenance, acquisition, accounting, or IT systems.
Next, the independent variables are listed, which are market networks, institutional networks, and obstacles. To operationalize market and institutional networks, the criteria established by Laursen and Salter (2004, 2006) are followed; these researchers based their operational variables on a communitarian innovation survey in the United Kingdom. Regarding the obstacles, the criteria established for the questionnaire of the 2015 innovation survey of the Peruvian manufacturing industry were followed; this questionnaire analyzed 7 obstacles that had been grouped into four categories: obstacles related to knowledge, to the market, to expenses and finances, and to other factors (INEI, 2015). For both market and institutional networks, dichotomous variables consisting of 5 items and 3 items, respectively, were used. On the other hand, obstacles were measured on a Likert scale according to degree of importance by using the following scale: 1=none, 2=low, 3=medium, 4=high.
The control variables used are firm size and age. The size and age of the firm can influence how product and process innovation are carried out. Firm size (expressed as a logarithm) is measured by the number of employees each firm has (Schoenmakers & Duysters, 2006; Laursen & Salter, 2006), and firm age (Thornhill, 2006) is measured as the number of years (expressed as a logarithm) that have passed from the firm’s foundation up until the year the data was collected from the firm (2014). See table 8 in the annexes.
3.3. Analysis methods
SmartPLS 3 estimates the process of the structural equation model (SEM) through the two-step PLS technique, according to Chin et al. (2003). First, the measurement model is estimated when the relationship between the indicators and the latent construct are determined. Second, the estimation of the structural model is carried out, and the relationships between the constructs are obtained through the path coeficients and the level of significance. The application of structural equations is justified, according to Hair et al. (2019), for one main reason: because the information comes from a secondary database.
4. RESULTS
The results are presented in three stages: first, the results of the factorial analysis; then, the results of the measurement model; and finally, the results of the structural model.
4.1 Factorial Model
SPSS software was used for the analysis of the three independent variables: market networks, institutional networks, and obstacles. An Exploratory Factor Analysis (EFA) was used to determine which items measure each factor using certain statistical criteria, like the Kaiser-Meyer-Olkin (KMO) test for sampling adequacy and Bartlett’s test of sphericity, obtaining the factorial structure for an easy and significant interpretation (Pando et al., 2016). The results are shown in Table 2. According to Jiménez et al. (2016), to verify reliability and validity, the percentages of the total variance explained are given: 51.17%, 55.70%, and 52.05% for institutional networks, market networks, and obstacles, respectively.
4.2 Measurement model
The research data were analyzed using indicators of internal consistency reliability, including Cronbach’s alpha (CA) and the composite reliability (CR) index, whose values should be greater than 0.7; convergent validity through the average variance extracted (AVE), whose value should be greater than 0.5; the analysis of the multicollinearity measured by the variance inflation factor (VIF), whose values should be under 5; and the coeficient of determination (R2).
Table 3 shows the values of the reliability and convergent validity indicators, the multicollinearity analysis, and the coeficient of determination for the model with product innovation as the dependent variable. According to Wong (2016), when the focus of the partial structural equations is applied, the composite reliability index is a better indicator than Cronbach’s alpha. For convergent validity, the indicators possess reasonable values. For the multicollinearity analysis, the variance inflation factor exceeds 5 for none of the variables. The coeficients of determination are 0.33 (product innovation) and 0.31 (process innovation) which show a moderate relationship between the dependent and independent variables.
Table 3 Reliability and validity indicators
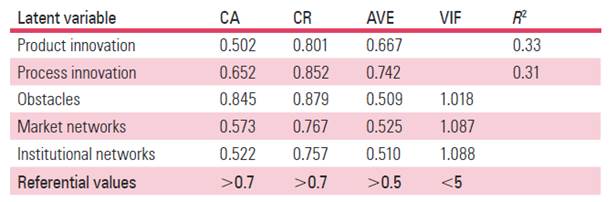
CA, Cronbach’s alpha; CR, Composite reliability; AVE, Average variance extracted; VIF, Variance inflation factor. Source: Smart PLS 3 Software.
Regarding discriminant validity, the Fornell-Larcker criterion (1981) establishes that the values of the square root of the variance extracted (AVE), shown in the diagonals of Table 4 in bold, should be greater than the correlations between the latent variables. Both in the product innovation model (Table 5) and in the process innovation model (Table 6), it can be appreciated that the requirements for discriminant validity are met.
4.3. Structural model
After evaluating measurement methods, a structural model was estimated for product and process innovation. Tables 5 and 6 show the coeficient values and the p-values of the models for product and process innovation, respectively, which helped to determine the hypotheses’ statistical significance, according to Hair et al. (2014). The bootstrapping method was used, with 2000 resamples.
Table 5 shows the results of the structural model of product innovation. The relationship between market networks, institutional networks, obstacles, and product innovation can be appreciated. The coeficients are positive and statistically significant.
Table 5 Results of the product innovation structural model
Hypothesis | Coefficient | P-value | Is the hypothesis confirmed |
H1: MN->PRODI | 0.211*** | 0.001 | Yes |
H2: IN->PRODI | 0.098** | 0.032 | Yes |
H5: OBS->PRODI | 0.109** | 0.003 | Yes |
Note: MN: Market Networks; IN: Institutional Networks; OBS: Obstacles; PRODI: Product Innova- tion. Note: *p<=0.1; **p<=0.05; ***p<=0.001
Source: Smart PLS 3 Software.
Table 6 shows the results for the structural model of process innovation. For all three relationships between market networks, institutional networks, obstacles, and process innovations, the coeficients are positive and statistically significant.
Table 6 Results of the process innovation structural model
Hypothesis | Coefficient | P-value | confirmed |
H3: MN->PROCI | 0.198*** | 0.001 | Yes |
H4: IN->PROCI | 0.194*** | 0.001 | Yes |
H5: OBS->PROCI | 0.085** | 0.017 | Yes |
Note: MN: Market Networks; IN: Institutional Networks; OBS: Obstacles; PROCI: Process Innova- tion. Note: *p<=0.1; **p<=0.05; ***p<=0.001. Source: Smart PLS 3 Software.
The model also met the goodness of fit index (GoF) (Tenenhaus et al., 2005). This index varies between the values of 0 and 1. Although there is no minimum threshold, a value greater than 0.36 is recommended (Wetzels et al., 2009). The GoF index reaches a value of 0.48 for the proposed theoretical model, which is greater than the minimum recommended to guarantee the goodness of fit for the model studied. Moreover, the model fits through the standardized root mean square residual (SRMR) (Hu & Bentler, 1998, 1999). A model is considered to have a good fit when values are less than 0.08. Therefore, an SRMR value of 0 would indicate a perfect fit, but a recent simulation study shows that a specified correct model would have SRMR values greater than 0.06 (Henseler et al., 2016). The structural model has adequate SRMR values of 0.076.
Table 7 shows the coeficients of the control variables for each dependent variable. In the case of product innovation, both coeficients are not statically significant, whereas for process innovation, firm size has a positive and statistically significant coeficient. In other words, larger firms have more resources to carry out process innovations.
4.4. Analysis of the common method variance
According to Podsakof (2003), the criterion to evaluate the common method variance is Harman’s single-factor test, which is shown in Table 9. If the percentage of variance is less than 25%, it shows that the skew of the common method variance does not affect the model (Ylitalo, 2009). Therefore, in the study the items could be grouped to create the latent variables in the analysis. The variance explained is 17.48%, showing that the common method variance does not affect the model. The calculations were carried out using IBM SPSS Statistics software, and the results are shown in Table N˚ 9 the annex.
DISCUSSION
This research examines technological innovation capability in low-tech firms in emerging countries. The perspective of this study focuses on the role that market and institutional networks can have on technological innovation capability. With a SEM model the data of 705 Peruvian firms have been analyzed to verify if there is statistical support for the five hypotheses. The SEM model hypotheses relate firms’ technological innovation capability, divided into two types: product and process innovation, (the dependent variables) to the connections that firms have with market and institutional networks and, additionally, to the possible obstacles that the firms could encounter when trying to carry out innovation activities (independent variables). The study also uses control variables, like firm age and number of employees.
This study seeks mainly to analyze the influence that market and institutional networks have on product and process innovation in low-tech firms. The results obtained regarding the relationship between market and institutional networks and product innovation are similar. On one hand, market networks have a positive and statistically significant (Hypothesis H1, coeficient 0.212, p?0.001) relationship with product innovation. These results coincide with those of previous studies (Ghauri et al., 2016), which show that firms that forge connections with market networks: suppliers, customers, competitors, etc., are able to develop product innovations. On the other hand, this study provides support for the relationship between institutional networks and product innovation in low-tech firms (Hypothesis H2). The hypothesis is accepted. This last result is consistent with the results of Robert and Schubert (2013), who found that firms that form relationships with universities and public research institutions are more likely to develop product innovations.
For process innovation it can be confirmed, with statistical significance, that higher levels of involvement in market networks are related to more process innovation activity, in accordance with Hypothesis H3 (coeficient 0.199, p?0.001), and, in the same way, higher levels of involvement in institutional networks are related to more process innovation, in accordance with Hypothesis H4 (coeficient 0.195, p?0.001). These results agree with those obtained by Un and Asakawa (2015), who found that firms with connections to suppliers (market networks) are able to implement process innovations. Moreover, the connection of low-tech firms with universities and public or private research institutes (institutional networks) is related to process innovation, in accordance with Maietta’s (2015) results.
Furthermore, there is broad interest in determining how firms have been able to innovate despite obstacles to commercial activity (Karahan & Karhan, 2013). This present study shows that obstacles can be related both to product innovation and to process innovation (Hypothesis H5 and H6), and, therefore, low-tech firms are capable of overcoming these obstacles. These results are in agreement with those obtained by Blanchard et al. (2013), who analyzed communitarian innovation surveys in France, and Santiago et al. (2016) and Pellegrino and Savona (2017), who analyzed the situation in emerging countries; the aforementioned researchers all found that overcoming financial obstacles allowed for innovation capability improvement.
Finally, the results of this study of the influence of the control variables on process innovation coincide with the results of previous studies (Huang & Rice, 2012). The analysis shows that there is a positive and statistically significant relationship between firm size and process innovation; this coincides with the results of Huang and Rice (2012), aligning with evidence that large firms have more resources to carry out innovation activities.
5. CONCLUSIONS, LIMITATIONS, AND FUTURE RESEARCH
One of the main challenges faced by emerging economies is their firms’ innovation capacity. Although this is a problem that has been thoroughly studied in relation to high-tech firms, there have not been studies on low-tech firms’ innovation behavior. Low-tech firms make up an important percentage of the gross domestic product of emerging countries and, therefore, their innovation capacity has an important influence on the wealth of those emerging economies. This study contributes by providing evidence of the relationship between market and institutional networks and product and process innovation in emerging economies like Peru. These results should help policymakers promote legislation to increase the competitiveness of low-tech firms in Peru and in other emerging economies.
In this sense, the academic results of our work may confirm that for these group of firms, low-tech in emerging countries, the connection between the low-tech firm and market and institutional networks aids in the development of product and process innovations. These results contribute to better understanding of how low-tech firms can afford technological innovation. This is peculiar to these firms because they are not able to develop strong R&D units and deploy R&D plans. Higher-tech firms in developed countries are more used to these initiatives than the others. And most of the literature on implementing innovation initiatives comes from different contexts. This, in turn, means that specific studies are needed to help stimulate and promote innovation in these firms from the new perspective of being part of a set of networks in an open setting, and an innovative ecosystem.
This study tries to contribute to the literature of management of low-tech firms from emerging economies. As it has been said, most of the studies on innovation have focused on high-tech firms in developed countries. This study starts a new line of research in this group of firms. Low-tech firms are not inclined to develop technological innovation activities. This study provides a specific action line to start innovation activities in low-tech firms in emerging countries. This action line can provide new insights to managers and policymakers to promote innovation activities in this segment of firms. On the one hand, low-tech firms are not considered pioneers in the development of technological innovations, and they try to replicate what innovation leaders do. This study gives some clues as to how low-tech firms can start acquiring a better position in the innovation scene. Firstly, low-tech firms can start forming their market and institutional networks to get new sources for innovation opportunities. Secondly, it is not necessary to have strong R&D departments to innovate. Innovation opportunities can be obtained from the networks that come with each firm. On the other hand, a set of specific cultural traits are necessary to manage an open innovation approach. Firstly, an open innovation approach needs a fluent relationship with the members of the firm’s network, but also an attitude to accept what comes from outside the firm. This implies establishing a new cultural setting within the firm. Secondly, open innovation is a not a new R&D department, as it requires a comprehensive vision within the strategic settings of the firm.
This study allows for some managerial implications to be identified. First, managers of low-tech firms should foment connections with customers, suppliers, and competitors and also with universities and research institutes in order to develop the greatest number of technological innovations. Second, this cannot be an isolated initiative, building connections should be related to changing the cultural settings within the firm. That means, at least, two different things. On the one hand, to create a culture of innovation, on the other hand, to prepare all members of the firm to participate in an open context where innovation is going to be part of the activities to develop new products and services. Also, managers of low-tech firms should evaluate the obstacles they face and, in this way, develop technological innovations.
How is this approach connected to the current situation of the pandemics? As far as we understand the trends behind the results of this work have been emphasized by the pandemics. Interacting with the members of firm’s networks and being an active part of an open innovation ecosystem is needed in order to have a sound infrastructural support form telecommunications networks and software applications. The current pandemics have accelerated the deployment and the use of this infrastructure. In this sense, to remain competitive, firms should be able to adapt to these new features to enhance their innovation efforts.
Most of the countries in the Latin American region have a similar economic structure. At the same time, all these countries differ from developed countries. Although this study has been based on a sample of firms in Peru, the trends behind the results obtained could be expected to have some replicability in other countries. In any case, further research should be necessary to better understand how these results can be used in other
This study is not exempt from limitations, which were as follows: the first limitation has to do with the fact that the study used information obtained from the national innovation survey of the Peruvian manufacturing industry in 2015. In other words, it is a cross-sectional study that faces two problems: the bias generated by the fact that each questionnaire was answered by one single person and the fact that this type of study does not allow for the establishment of a causal relationship between constructs (Rindfleisch et al., 2008). It is suggested that a longitudinal study or comparative studies using innovation surveys from other Latin American countries be carried out.
The second limitation has to do with how the constructs referred to as “market networks” and “institutional networks” have been measured; these constructs reflect connections to customers, suppliers, or competitors and to universities and public or private research institutes, respectively. It is suggested that research be carried out that identifies specific connections, for example, those with customers, with suppliers, or with universities, and that shows how these individual relationships favor the development of product and process innovations.
A final limitation has to do with how obstacles to innovation were measured. Four categories were formed: obstacles related to knowledge, obstacles related to the market, obstacles related to expenses and finances, and obstacles related to other factors, but then a single score was obtained. It is suggested that the way each category of obstacles is related to the development of technological innovations be examined individually.