Introduction
Chronic or long-term illnesses worsen over time, often creating a need for treatment and long-term care 1. According to the Pan-American Health Organization, chronic diseases kill 41 million people each year, which is equivalent to 71% of the deaths that occur in the world. In the Region of the Americas, there are 5.5 million deaths from these each year 1. Moreover, it is alarming that the Americas region witnesses approximately 1.6 million deaths from cardiovascular diseases each year. Currently, diabetes and hypertension are the diseases with the highest prevalence among the population. These conditions give rise to significant complications, particularly affecting the vascular system, since they cause serious damage to organs such as kidneys, heart, brain, and eyes 2. In the case of the eye, there is a significant risk of damage to the retinal vasculature due to increased pressure, which can eventually lead to the development of retinopathy. Table 1 shows a brief description of the main parts of the eye and the health consequences due to diabetic and hypertensive retinopathy respectively. Many of these alterations occur in different areas or parts of the eye, often represented as the quadrants. These areas are a representation of the eye divided by two lines; a vertical line which separates into a nasal half and a temporal half and a horizontal line which separates the upper and lower part of the eye 3.
Table 1 Description of the main parts of the eye, and their effects due to diabetic and hypertensive retinopathy. Symptoms that are related to diabetic retinopathy in italics, the symptoms of hypertensive retinopathy in an underline and those that apply to both in bold.
Organ | Definition | Retinopathy Impact |
---|---|---|
Cornea | Complex, fibrous, transparent, and thin structure, refracts light and cooperates with the lens to produce a reduced inverted image 4. | Morphological and functional changes of the cornea 5 |
Hyaloid Canal | It is a clear central area of the eye that crosses the vitreous from the papilla or optic disc to the lens 4. | In the proliferative stage, there is greater tension in this part due to macular degeneration6. Reactive sclerosis because of an accumulation of hyaline material 7. |
Retina | It is a light-sensitive layer that lines the inside of the back of the eyeball 8. Made of the papilla or optic disc, the macular, and the retinal blood vessels 9. | In proliferative stage, increased homocysteine levels were demonstrated in retina10. Diffuse or focal vasoconstriction, extravasation due to increased vascular permeability 7. |
Macula | While the rest of the retina provides peripheral vision, the macula allows the human eye to see in detail 9. | Hemorrhages occur, producing exudation with retinal edema or hard exudates 7. The early Non-Proliferative is characterized by increased vascular permeability, and in PDR diabetic macular edema10. |
Optic Nerve | It is the first section of the pathway that goes from the eye to the brain. It is an approximately circular area in the center of the retina 9. | In the early stage of Diabetes with morphologically unaffected suggesting optic nerve degeneration11. Pale disk, blurring of the papillary and cavernous margins 7. |
Blood Vessels | They are in the upper layers and oversee nourishing the retina together with the choroidal blood vessels 9. | These alterations in the vessels can lead to ischemia of the retinal tissue 12. |
Currently, retinal fundus images are being used to detect and evaluate eye diseases, such as glaucoma and retinopathy; some authors consider it the best technique for studying the interior of the eye and includes the retina, optic nerve, macula, and posterior pole 13. In Figure 1, a photograph depicts the retina of a healthy patient, highlighting three distinct ocular structures: the papilla or optic disc, the macula, and the retinal blood vessels 9.
Ophthalmologists observe and take images of the fundus to examine possible lesions or abnormalities 9. To diagnose both diabetic and hypertensive retinopathy, the ophthalmologist conducts an initial examination that involves a comprehensive evaluation of the eyes 13.
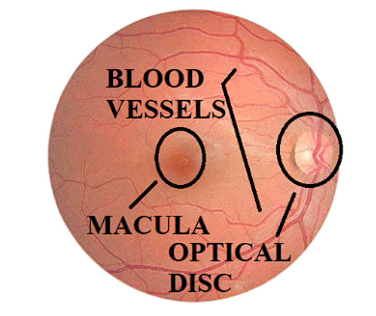
Figure 1 The retinal image of the right eye of a healthy patient highlights the optical disc, macula, and blood vessels, principal elements for the diagnosis of retinopathy.
Diabetic retinopathy (DR)
This disease is considered like a serious and stands as the primary contributor to visual impairment. It represents the prevalent microvascular complication of diabetes mellitus, potentially resulting in severe harm to the retina, ultimately resulting in a loss of vision 14. Diabetic Retinopathy (DR) causes damage to the blood vessels in the retina, the light-sensitive tissue at the fundus of the eye. This damage appears in two stages known as Non-Proliferative Diabetic Retinopathy (NPDR) and Proliferative Diabetic Retinopathy (PDR). In the PDR stage, there are serious alterations in the thickness of the retina and in the macular area, causing an edema. In NPDR it is common the swellings in the retina, when there is much bleeding, also it can develop and present macular edema, reaching the proliferative stage 15. Table 2 describes the key characteristics of this disease and their classes:
Table 2 Main characteristics of diabetic retinopathy in the different classes, accompanied by the images of this classification, the main characteristics of each level are enclosed in a yellow circle.
Class 0 ![]() |
Class 1![]() |
Class 2 ![]() |
Class 3 ![]() |
Class 4 ![]() |
---|---|---|---|---|
No abnormality is evident. Less than a 1% chance of developing PDR 16. | Mild NPDR, microaneurysms are the only abnormality found 16. | Moderate NPDR contains punctate hemorrhages or microaneurysms 16. | Severe NPDR. 20 or more hemorrhages over the four quadrants 16. | PDR More advanced stage. New fragile and abnormal blood vessels grow 16. |
Hypertensive retinopathy (HR)
Hypertensive retinopathy (HR) is retinal vascular damage, which is caused by hypertension, especially in the mild level patients. The examination of fundus image allows to Identify these morphological changes, such as microaneurysms, yellow hard exudates, soft exudates (cotton wool spot), hemorrhages, macula, optic disk, optic nerve head, and an elevation in the number of blood vessels such as arteriolar constriction or sclerosis 17. Table 3 describes the key characteristics of this disease and their classes:
Table 3 Main characteristics of hypertensive retinopathy in the different classes, accompanied by the images of this classification. The main characteristics of each level are enclosed in a yellow circle.
Class 0 ![]() |
Class 1 ![]() |
Class 2 ![]() |
Class 3 ![]() |
Class 4 ![]() |
---|---|---|---|---|
No visible signs of hypertensive retinopathy 18. | Benign hypertension. Sclerosis at a moderate level of the retinal arterioles 19. | Sclerosis at a moderate level of the retinal arterioles. Higher level of blood pressure compared to class 1 19. | Cottony spots and bleeding. Also symptoms of spastic lesions of the arterioles 19. | Malignant hypertension. All the previous are present in addition to macular edema 19. |
Related Work
Over the years, researchers have conducted numerous studies on diabetic retinopathy; however, there has been relatively limited research on hypertensive retinopathy 20. Nevertheless, in the field of hypertensive retinopathy, most studies have focused on the segmentation of blood vessels. Chala et al. propose the use of deep CNNs for retinal blood vessel segmentation, inspired by popular architectures like FCN, U-net because they are the most popular used architectures in medical imaging, with a multi-encoder-decoder structure and three main units: RGB encoder, green encoder, and decoder 21. While the first method employs a UNet, Zhang et al. employ TransUNet because combines the strengths of convolution and multi-head attention mechanisms to capture local features and global dependencies. It incorporates self-supervised anatomical landmark extraction through contrastive learning to emphasize retinal vessel morphology learning 22. In contrast, NA et al. employs a modified version of the popular U-NET architecture along with proposed pre-processing algorithms in order to segment the input images; the model works on extracted patches of images obtained after the pre-processing stage 23.
Some of the networks used in the classification of retinopathy include: Abbast et al. proposed the use of the DenseNet network to detect five stages of HR based on semantic- and instance-based dense layer architecture and a pre-training strategy 18. Moreover, Nguyen et al. employs a convolutional neural network (CNN) EOPSO-CNN model ensemble based on orthogonal learning particle swarm optimization (OPSO) algorithm to perform detection and classification of diabetic retinopathy 24. Also, Takahashi et al. employed a modified fully randomly initialized GoogLeNet deep learning neural network, using a retinal area that is not usually visualized on fundoscopy and another AI that directly suggests treatments and determines prognoses for DR classification 25. Moreover, Dutta et al. propose an automated knowledge model to identify the key antecedents of DR. The model has been trained with three types, back propagation NN, Deep Neural Network (DNN) and Convolutional Neural Network (CNN) 26.
Datasets
Data sets consist of different sources that are available from Kaggle, Drive, ChaseDB1, and Mendeley. Performing a preprocessing on the data set to improve it, as the images had different formats, and some contained a thick black frame. Afterward, using various computer vision and deep learning techniques to perform segmentation and classification of the different stages of the diseases.
Table 4 shows the different sources of information, including the total amount, division into training and validation sets, format, and file type associated with each set, along with their corresponding labeling. There are two types of labels used in the dataset. The first type is a CSV file employed in the Kaggle dataset, which provides information about the disease class and specifies whether it pertains to the right or left eye. In the case of the Odir dataset, also include patient's age, and gender. The second type of label represents the vascular vessels in an image format, specifically PNG.
Table 4 Description of the data set from the different sources used in the research, highlighting the quantity, the division into the different sets and the format of the images.
In order to face the inherent set imbalances, particularly the diminished representation of hypertensive retinopathy images and an uneven distribution among classes, with a pronounced bias towards healthy data, it was necesary implement a careful selection process. This involved both a reduction in the number of diabetes images and the application of data augmentation, especially in the advanced disease classes. Table 5 shows the refined dataset.
Table 5 Description of data distribution used in the different sets after the selection and increase of the images to balance the total number of images to be used.
Set | Quantity | Diabetes | Hypertension |
---|---|---|---|
Training | 23.900 | 11.902 | 11.998 |
Validation | 5.996 | 2.998 | 2.998 |
Testing | 7.672 | 3.836 | 3.836 |
Total | 37.568 | 18.736 | 18.832 |
Image preprocessing
The image preprocessing consists of the process to reduce noise and improve the shape of the images, for that reason it performed different techniques to improve the datasets like Contrast Limited Adaptive Histogram Equalization (CLAHE), which stands for is a variant of Adaptive Histogram Equalization (AHE) 32. It operates in small regions of the image, called tiles, rather than on the entire image 32. An additional edge trimming was also implemented and improvement in the rounding of some because they presented a more elongated format. Additionally, performing resize in different square sizes to evaluate which of these sizes enabled more effective feature extraction in the convolutional neural networks used.
Methodology
In the realm of medical image analysis, the fusion of advanced deep learning architectures has proven to be a powerful strategy for comprehensive disease diagnosis 33. This study leverages the synergy between a U-Net for precise segmentation of eye blood vessels and a Convolutional Neural Network (ConvNet) featuring the efficient and effective architecture of EfficientNet. The objective is to concurrently address diabetic and hypertensive retinopathy, distinguishing between their four distinct severity levels. This integrated approach offers several advantages. Firstly, the segmentation accuracy achieved by the U-Net enhances the precision of feature extraction, allowing the ConvNet to make more informed and context-aware classifications. Secondly, the efficient architecture of EfficientNet optimizes computational resources, enabling faster and more resource-efficient training and inference. Finally, the results are generated in a CSV file where the image to be evaluated is related to the level of the disease, with 0 to 4 being the levels corresponding to diabetic retinopathy and 5 to 9 being hypertensive, Figure 2 shows the fusion process of the two networks and the integration of the morphological masks.
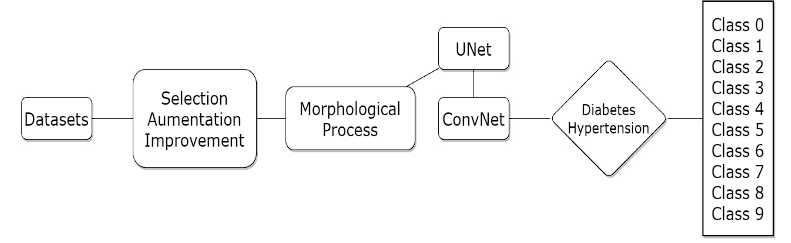
Figure 2 The diagram illustrates a sequential process for classifying diabetic and hypertensive retinopathy. It commences with image enhancement, followed by morphological mask creation and blood vessel segmentation using the U-Net model. These segmented outputs.
UNet
This network has an encoder-decoder structure in the sense that it is made up of two parts 34. The difference with the traditional encoder-decoder structure is that there are connections that copy the data that is being processed in the encoder to the decoder to facilitate the generation of the mask 34. Figure 3 present the UNet architecture proposed by Ronneberger et al. 35 for blood vessel.
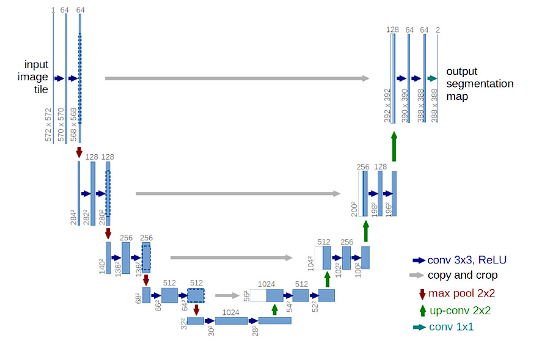
Figure 3 U-Net architecture employed by Nikhil Tomar for the segmentation of blood vessels in hypertensive retinopathy.
The red arrow pointing down is the max pooling process which halves downsize of image. The green arrow is a transposed convolution, an upsampling technic that expands the size of images. Each blue box corresponds to a multi-channel feature map. The number of channels is denoted on top of the box. The x-y-size is provided at the lower left edge of the box. White boxes represent copied feature maps. The arrows denote the different operations 35.
The investigation was also based on the code develop by Nikhil Tomar 36. The first part of this development in the preprocessing stage, using an increase in data with the open source “Albumentations” library, the second stage of this network is made up of four encoder layers. A bottleneck layer, this contains few nodes compared to the previous layers. The idea is that this compressed view only contains the “useful” information to be able to reconstruct the vessels 37. Finally, the four decoder layers rebuild the original image, doubling the size between each layer. The validation of the model is done with the Jaccard index, f1 score, recall, precision, and accuracy functions.
In addition, the validation of the process is conducted using the functions “Dice Loss” this approach effectively addresses the imbalance between the foreground and background 38. “Dice BCE Loss” calculates probabilities and compares each actual class output based of Bernoulli distribution loss, it compared each input and output with predicted probabilities, which can be either 0 or 1 39.
Convolutional Neural Network for Visual Recognition ConvNet
Over the decade, the field of visual recognition successfully transitioned from engineering functions to architecture design (ConvNet) 40. Representative ConvNets such as VGGNet, Inceptions, ResNe(X)t, DenseNet, MobileNet, EfficientNet, and RegNet focused on different aspects of accuracy, and performance, and popularized many useful design principles 40. ConvNets have several built-in inductive biases that make them appropriate to a wide variety of computer vision applications 40.
Figure 4 show the convolutional network propose by Person to execute the classification of diabetic retinopathy 41.
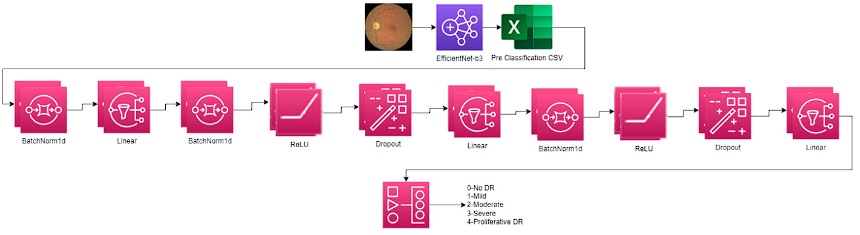
Figure 4 Architecture of convolutional networks for the classification of diabetic retinopathy proposed by Aladdin Person Image source: own elaboration from original architecture in 41.
Network architecture composed of EfficientNet learning transfer model because it is more efficient than most of the other CNNs models 42. There are eight models of this architecture, each one with seven blocks. These blocks also have a variable number of sub-blocks whose number increases from EfficientNetB0 to EfficientNetB7. The total number of layers in EfficientNet-B0 is 237 in EfficientNet-B7 it is 813 43. The model proposed by Aladdin Person uses the model EfficientNet-B3 this utilizes a composite scaling method that creates different models in the convolution neural network 44. In most of the exploration, better levels of accuracy are observed when using the superior models EfficientNetB7; however, for the research, the best results were obtained when using the B0 model that includes 4,049,564 parameters 45. Table 6 presents the layers, channels, and architecture of the EfficientNet-B3 and B0 model, where IRC represents the inverted residual connection used by the MBConv block. In traditional convolutional neural networks (CNNs), the number of channels typically increases as the network gets deeper. However, this can lead to a significant increase in computation and memory requirements 46. To address this issue, the IRC block is introduced in EfficientNet. By utilizing the IRC block, EfficientNet achieves a balance between model depth, width, and resolution, optimizing the trade-off between model size and accuracy. The skip connection within the IRC block enhances the gradient flow and helps in training deeper networks more effectively. An Inverted Residual Block, sometimes called an MBConv Block, is a type of residual block used for image models that uses an inverted structure for efficiency reasons.
It uses the same MBConv1 and MBConv6 modules as EffeicienNetB0 47. It was originally proposed. for the MobileNetV2 CNN architecture 48. The stage represents the typical design in a ConvNet network, where the layers are often divided into several stages 49.
Table 6 Description of the EfficientNet-B3 and B0 Architecture highlighting the layers, the resolution, and the number of channels 47.
EfficientNetB3 | EfficientNetB0 | |||||||
---|---|---|---|---|---|---|---|---|
Stage i | Operator 𝐹𝑖 | Resolution 𝐻𝑖 x 𝑊𝑖 | #Channels 𝐶𝑖 | #Layers 𝐿𝑖 | Operator 𝐹𝑖 | Resolution 𝐻𝑖 x 𝑊𝑖 | #Channels 𝐶𝑖 | #Layers 𝐿𝑖 |
1 | Conv3x3+BN | 300 x 300 | 40 | 1 | Conv3x3 | 224 x 224 | 32 | 1 |
2 | MBConv1, k3x3 | 112 x 112 | 24 | 2 | MBConv1, k3x3 | 112 x 112 | 16 | 1 |
3 | MBConv6, k3x3 | 112 x 112 | 32 | 1 | MBConv6, k3x3 | 112 x 112 | 24 | 2 |
4 | MBConv6, k3x3, IRC | 112 x 112 | 32 | 2 | MBConv6, k5x5 | 56 x 56 | 40 | 2 |
5 | MBConv6, k5x5 | 56 x 56 | 48 | 1 | MBConv6, k3x3 | 28 x 28 | 80 | 3 |
6 | MBConv6, k5x5, IRC | 28 x 28 | 48 | 2 | MBConv6, k5x5 | 14 x 14 | 112 | 3 |
7 | MBConv6, k3x3 | 28 x 28 | 96 | 1 | MBConv6, k5x5 | 14 x 14 | 192 | 4 |
8 | MBConv6, k3x3, IRC | 14 x 14 | 96 | 4 | MBConv6, k3x3 | 7 x 7 | 320 | 1 |
9 | MBConv6, k5x5 | 14 x 14 | 136 | 1 | Conv1x1 & Pooling & FC | 7 x 7 | 1280 | 1 |
10 | MBConv6, k5x5, IRC | 14 x 14 | 136 | 3 | ||||
11 | MBConv6, k5x5 | 7 x 7 | 332 | 1 | ||||
12 | MBConv6, k5x5, IRC | 7 x 7 | 332 | 4 | ||||
13 | MBConv6, k3x3 | 7 x 7 | 384 | 2 | ||||
14 | Conv1x1 & Pooling & FC | 7 x 7 | 1536 | 1 |
After the use of the EfficientNet model the use of a “Linear” layer or fully connected helps to change the dimensionality of the output of the previous layer so that the model can easily define the relationship between the data values on which the model works 50. The linear layer is the central building block of almost all modern neural network models, even convolutional networks typically feed one or more linear layers after processing by convolutions 51.
The function of the second convolutional network is to update the weights of the final layers of the pre-trained model of the first network, this architecture includes an additional 10 layers, with one specifically designed for normalization called “BatchNorm1d”. The primary function of BatchNorm1d is to estimate and normalize the mean and variance for each small batch of inputs processed by the layer. By performing this normalization, it helps ensure the stability and consistency of the input throughout the network. The advantage of using it is that it stabilizes the learning process and reduces the number of times that are necessary for training 52. After each convolutional layer, it is usual to apply a nonlinear activation layer. The most popular layer in convolutional networks is ReLU, compared to the more traditional sigmoid or Tanh since it is computationally more efficient 42. The dropout layer adds random zeros to some elements of the input tensor using samples from a Bernoulli distribution. This type of network has proven to be an effective way to regularize and prevent the adaptation of neurons 53.
Network Training
To execute the segmentation network Unet, the following transformations were used on the images:
After this process, the Unet is executed, training the information, and creating the mask that the ConvNet is going to use in the process of classification.
Changes to the original networks
Many segmentation methods are currently used to extract the region of interest, such as thresholding, region-based, soft computing, histogram, and neural network 55. The process used for the generation of the initial extraction of the tree of blood vessels is presented in Figure 5, in the same way an example of these steps is also presented in the Figure 9. Step 00 represents the initial image after undergoing the necessary preprocessing steps. Step 01 involves splitting the image into three RGB channels. The green channel is specifically selected for further processing due to its superior vessel-background contrast compared to the other two-color channels in retinal images 56. The step 02 “CLAHE” contrast in the green channel image, is a technique used to enhance the contrast of an image while limiting the amplification of noise 32. Using a contrast adjustment with a limit of two and a kernel of 11x11. The goal in this step is to improve the contrast and visibility of blood vessels. Step 03 subsequent step in vessel segmentation involved the application of morphological techniques, specifically erosion and dilation, as the fundamental operations 57. Starting with a dilation operation to preserve the finer details, using a structuring element of elliptical shape with a size of 5x5 pixels. A first opening operation, it helps remove small noise regions and smoothens the image, with a structuring element of 5x5, follow by a closed operation using a structuring element of size 7x7 pixels. Morphological closing is a dilation operation followed by an erosion operation 57. After this another opening operation but using a larger structuring element of size 9x9 pixels. The next operation is another closed, this time using an 11x11 pixel structuring element. It helps to close larger gaps and smoothens the image even more. Step 04 subtract from the image generated at the end of the morphology process with the image improved in the green channel. Step 05 applying CLAHE again to the previous result in order to improve the details in the blood vessels. Step 06 using “fastNIMeansDenoising” to clean the noise. The Fast Non-Local Means Algorithm (FNLM) algorithm under consideration encompasses multiple parameters. Within these parameters, the smoothing factor plays a pivotal role in defining the extent of filtering. It's important to note that this filtering extent directly affects the sharpness and clarity of images 58. Finally, Step 07 perform image thresholding on the denoised image using the "THRESH BINARY" and “THRESH_OTSU” technique. It converts the image to a binary image by assigning pixel values below a certain threshold to 0 and values above the threshold to 255.
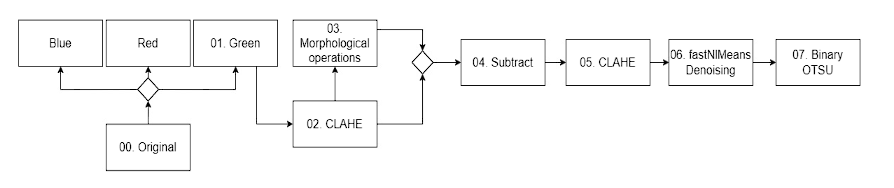
Figure 5 Description of the steps to extract the characteristics of the vascular tree utilizing various computer vision techniques, which are then employed to create masks in the UNet network.
In addition to transferring the learning model, expanding the classification to include 10 distinct categories. This involved categorizing the labeled data into specific groups: levels 0-4 representing diabetic retinopathy, and levels 5-9 representing hypertensive retinopathy.
Results
The first results correspond to the preprocessing phase, encompassing image enhancement, morphological mask creation. These foundational steps lay the groundwork for subsequent disease segmentation and classification.
Figure 6 depicts the transformation of the images following the application of the trim and roundness enhancement process. The image on the left corresponds to the original image sourced from the Kaggle dataset, while the image on the right represents the result after trimming the edges and resizing. Notably, the modified image maintains the relative proportionality of the rounds when compared to the other images.
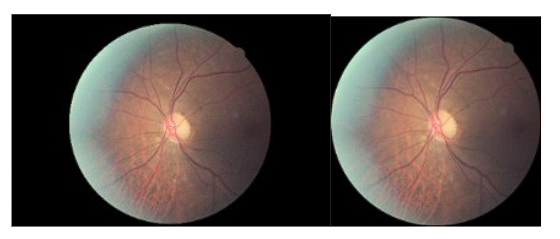
Figure 6 The image on the left is original from the Kaggle dataset, and the image on the right is after cropping extra edges and resizing without altering the fundus radius.
Figure 7, shows some examples of the results obtained after applying the transformations of the images that allow the data set to be increased, including the transformations of “HorizontalFlip”, “ElasticTransform”, “Grid Distortion”, “Optical Distortion” and “Rotate”.



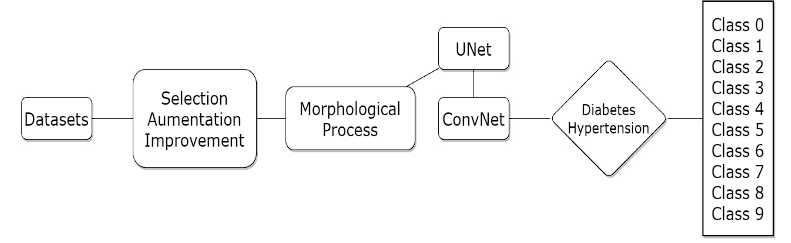

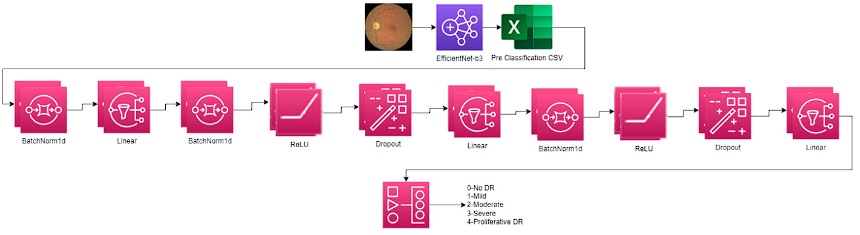
Figure 7 Result of the increase of images used in the UNet network, the first image corresponds to the original, and the others correspond to the increases through the Albumation library.
Figure 8 shows the result obtained by increasing the data in the ConvNet network. It observed an increase in the number of generated images, where some exhibit horizontal and vertical rotations, like the results obtained from the previous network. Additionally, the “ColorJitter” function introduces variations in color across the images. The last images display the utilization of CLAHE. Moreover, the application of the “Sharpen” function contributes to more pronounced edges, resulting in enhanced image details.

Figure 8 Result shown in a set of images of the magnification generated in the first part ConvNet network of several images selected by the algorithm when performing the training process.
The results shown below correspond to the process of creating a morphological mask and segmenting the blood vessels. After performing the morphological operation, the masked images were used to train the UNet Network. These masks were generated because the diabetes data sets did not have this information. Figure 9 exhibit an example of the creation of the vascular tree masks, and the Unet process, which will be used in the classification of the images in the different classes and levels of the disease.

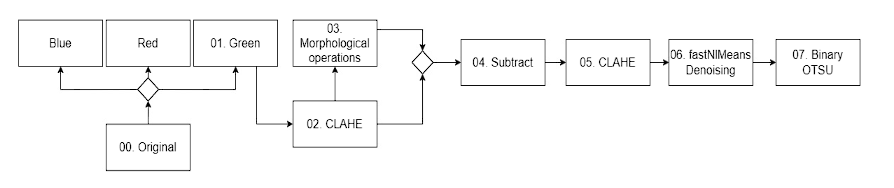



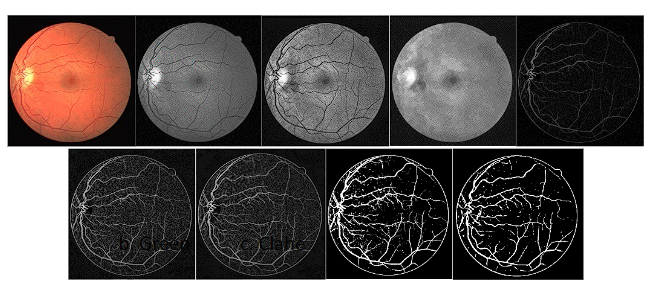
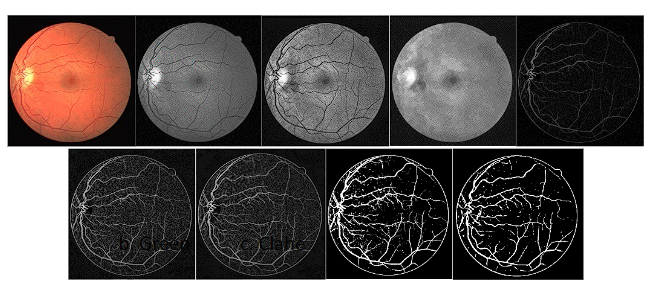
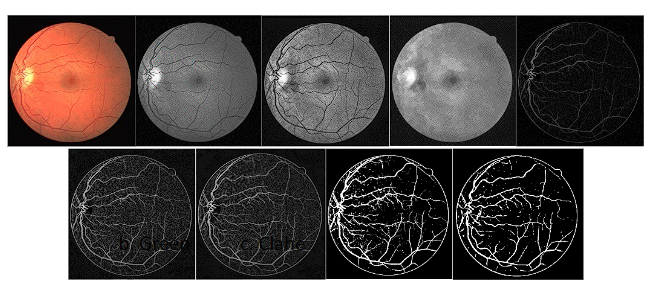
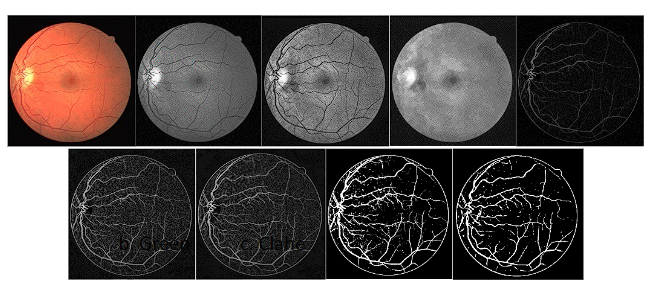
Figure 9 Segmentation result process, image a. corresponds to the original retina background. The images b to h are the result of applying the mask generation process. The image i. displays the mask generated with the UNet network.
To evaluate the degree of correspondence between the generated masks and the originals, a validation process became essential. This procedure entailed a thorough comparison between the original masks and those produced using the previously described morphological method. The findings demonstrated approximate accuracy rates of 90% and precision of 80% respectively. To gain a more comprehensive understanding of this similarity, we employed a confusion matrix to precisely quantify the number of pixels displaying matching correspondence within the two sets of masks. Figure 10 shows the comparison between the created mask and the original, as well as the number of pixels that were correctly selected.

Figure 10 Example of the comparison of the masks created by the morphological process and the original masks from the hypertension data sets. The image on the left is the created mask, the image in the center is the original mask, and the image on the right shows.
To assess the performance of machine learning algorithms, analysts commonly utilize the metric of precision or “accuracy”. This metric is favored due to its ability to evaluate the classifiers' generalization capabilities effectively 59. Jaccard Index, also known as Intersection-Over-Union (IoU), is a statistic used to measure the similarity or even diversity between sets 60. This Jaccard index approach has also been used in computer vision models and object recognition to measure the similarity between two images, usually, the predicted image and its ground truth image 60.
Additionally results obtained in the UNet network with the morphological images generated masks are Jaccard of 74%, F1 of 85% and Accuracy of 96%. Also, the results obtained in the ConvNet network for the classification of the images in the two diseases and in the different levels of the disease is 80% accuracy. Furthermore, rather than opting for commonly used datasets such as DRIVE or CHASEDB1, it was selected also a diabetic dataset that deviates from the conventional approach by lacking pre-existing masks. Finally, the selection over the use of UNet unlike others was because this is specially adapted for the segmentation of biomedical objects, specially is justified by the efficiency in training with limited data sets, which is essential in our context 61.
Finally, the results obtained in the classification process are presented. Figure 11 shows the confusion matrix and the table information with the distribution of the image for the validation set. It shows how each of the classes was identified in relation to the real and predicted label. In the matrix, it can be seen how most of the time a good classification was achieved, and where there was the greatest error was in the limit of one class or another. This test is performed using the EfficientNet-B0 learning transfer model, and the ConvNet network, with the previously mentioned increments and preprocess to the images. The difference in colors is not taken in comparison with all the classes but with the same class because there are classes with fewer images in total, as is the case with the most advanced classes of the disease. Table 7
Table 7 information from the confusion matrix total number of images according to the class, along with the variables for validation of results in the 10 classes created.
Class | Precision | Recall | F1 Score | # Images |
---|---|---|---|---|
0 | 0.89 | 0.95 | 0.92 | 980 |
1 | 0.74 | 0.85 | 0.79 | 772 |
2 | 0.65 | 0.61 | 0.63 | 502 |
3 | 0.77 | 0.69 | 0.73 | 526 |
4 | 0.70 | 0.50 | 0.58 | 218 |
5 | 0.90 | 0.95 | 0.92 | 980 |
6 | 0.78 | 0.86 | 0.82 | 772 |
7 | 0.67 | 0.64 | 0.66 | 502 |
8 | 0.86 | 0.71 | 0.78 | 526 |
9 | 1.00 | 0.73 | 0.84 | 218 |
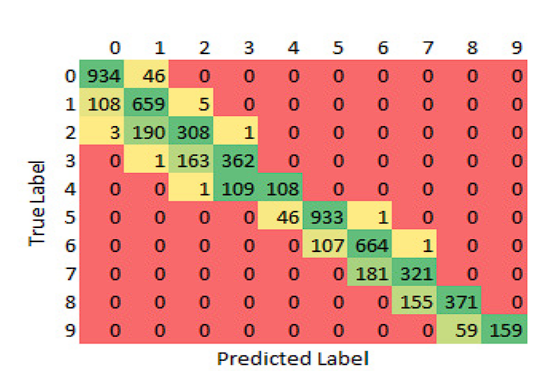
Figure 11 confusion matrix of the ConvNet results for the 9 classes of retinopathy, with levels 0 to 4 for diabetes and levels 5 to 9 for hypertension, using de model EfficientNet-B0. The Y axis shows the actual labels and the X axis those predicted by the network.
The results of the learning models were compared, obtaining better results when using the B0 model as the first step. Table 8 shows the differences in precision and loss at the end of executing the pretrained model and the second convolutional network. The number of successes column represents the number of classes correctly classified over the total number of images taken.
Discussion
Two distinct approaches were employed in the implementation of this study, encompassing both segmentation and classification. These two approaches were integrated to facilitate the comprehensive segmentation and classification of diabetic and hypertensive retinopathy images. For the segmentation process, we harnessed a Unet architecture, which involved an array of image processing steps, including vital morphological operations. This approach allowed for the segmentation of datasets that lacked masks as labels, enhancing the versatility of the model.
In the realm of hypertensive retinopathy research, segmentation typically takes precedence, often utilizing datasets furnished with masks as labels. However, in contrast to the approach taken by Xu 62, that primarily addresses the segmentation of retinal blood vessels in well-established datasets such as DRIVE and STARE. In contrast, in this research implement the segmentation of retinal images from a broader range of datasets, including a dataset for diabetic retinopathy. Crucially, both the diabetic retinopathy dataset and the Odir hypertensive dataset presented a challenge as they did not include initial masks, necessitating the application of a preprocessing step involving critical morphological operations.
Turning to the results, we achieved a Jaccard index of approximately 74% in the segmentation process. It's worth noting that further improvements in segmentation are plausible by refining the mask generation process, particularly as many images exhibit variations in tonal characteristics, necessitating consideration of the gray channel as an alternative to the green channel and enhancing noise reduction techniques. In terms of classification, we achieved an accuracy of approximately 80%.
The observed performance disparities in Table 6, particularly for classes 1, 2, 6, 7, and 8, are acknowledged and warrant a closer examination. These challenges highlight the complexity of real-world scenarios and the need for further refinement in the proposed approach. Looking ahead to future research endeavors, we propose a refinement of the classification process by working with a reduced set of categories, specifically 9 categories starting from 0, excluding the presence of the disease, and the four levels of the two diseases. However, one challenge we encountered, particularly in the case of hypertensive retinopathy, is the scarcity of data. To address this limitation, we intend to explore potential collaborations with medical centers to expand the dataset. For enhanced robustness and reliability, it recommends incorporating cross-validation techniques. Employing cross-validation ensures a more thorough evaluation of the model's performance by assessing its generalizability across different subsets of the dataset. This approach provides a comprehensive validation process, contributing to the overall reliability and effectiveness of the proposed methodology.
Conclusions
In this study, was presented an approach that used the power of deep learning and traditional convolutional neural networks to address the challenging task of diabetic and hypertensive retinopathy image segmentation and classification. The key contributions and findings can be summarized as follows:
Unet and ConvNet Integration: Introducing a fusion of Unet and ConvNet architectures, combining the strengths of both for the segmentation and classification of diabetic and hypertensive retinopathy images.
The creation of morphological masks specifically for the diabetic data set, because these did not have the initial labels, is different from the hypertension sets that have been widely used in another research. The characteristics of diabetic retinopathy images can vary, and the use of custom masks ensures that the segmentation process adapts to the unique characteristics present in these images.
Utilization of EfficientNet Pretrained Model: To enhance the efficiency and accuracy of our model, we employed the EfficientNet pretrained learning model. Our experiments demonstrated improved results with this model, showing its suitability for retinopathy image analysis.
Transfer Learning: Exploring more sophisticated transfer learning techniques and architectures for retinopathy image analysis could lead to even more accurate results.
Data Augmentation: Investigating advanced data augmentation strategies to diversify the training dataset and increase model robustness.
In conclusion, the work introduces an approach for diabetic and hypertensive retinopathy image analysis. By combining the strengths of different neural network architectures and the importance in the creation of custom morphological masks for the diabetic retinopathy dataset. Future research endeavors can further advance these contributions and expand the impact of this work in the medical community.