Introduction
The techniques of ecological niche modeling (ENM), are frequently used to study the main environmental conditions which influence the presence of a species in specific geographical regions (Anderson, 2013). Combining an ecological and biogeographical view (Shcheglovitova & Anderson, 2013), geographical data of the occurrence of the species related to different kinds of environmental variables are used to understand the distribution of the species, the potential range of occurrence, and to establish better conservation strategies (Drew et al., 2010). ENM techniques are useful for handling the problem of conservation of poorly sampled taxonomic groups with incomplete information about their distribution (Drew et al., 2010). This is the case of the genus Heterophrynus, a Neotropical group of Amblypygi, which is highly diversified in Colombia, but with a notable lack of information; in recent years, many species have been described with few individuals and distribution records (Chirivi-Joya et al., 2020).
Particularly, the species Heterophrynus boterorum (Giupponi & Kury, 2013) has been recorded from different regions of Colombia, showing a high morphological variation between populations, which could be related to environmental barriers and conditions (Vásquez Palacios et al., 2019). In this research, we use the paradigm of the ecological niche modeling of adequate conditions for the species (Anderson, 2012; Peterson et al., 2011; Shcheglovitova & Anderson, 2013). Using ENMs, we assessed the association between sites of occurrence of H. boterorum and abiotic variables (temperature, humidity, precipitation, and elevation) to understand the abiotic niche requirements of the species, inferring its potential distribution, and searching for indications of isolation between populations.
Colombia has several endemic species like H. boterorum, many hotspots and wildlife refuges have been described in this country (Camacho et al., 1992). A big part of Colombian biodiversity is found in the Andean region (Morrone, 2001; 2017) as in the case of the order Amblypygi (Chirivi-Joya et al., 2020; Joya & de Armas, 2012). However, conservation policies developed for the Andean region seldom include Arachnids (Morrone, 2001; 2017). Colombia is the country with the highest diversity of the genus Heterophrynus, with at least six endemic species, which is a high number considering that the genus is only recorded for South America and has fewer than 20 described species. However, the distribution of Heterophrynus is poorly known due to the difficulties in collecting (Chirivi-Joya et al., 2020). We used the species H. boterorum as a model to understand how much wider the distribution of an endemic Heterophrynus species could be, and how much of this potential distribution is part of an already established protected area. At the moment, only a paper about the potential distribution of species of Phrynidae has been published, however, it refers to a species of Phrynus from the Caribbean Islands (Chapin et al., 2018). So this is also the first paper about niche ENM in Heterophrynus.
Materials and methods
Occurrence data and study region. The species H. boterorum inhabits mainly the central region of the Cordillera Central of Colombia, on both slopes; in the departments of Quindío and Tolima, from 591 to 1935 m a.s.l. We modeled the environmental requirements of H. boterorum according to its currently known distribution in the east and west of the Andes Centrales, and West of the Andes Orientales, using the records of Vazquez-Palacios et al. (2019). The distribution range falls between 3.6508° to 4.8078° N and 74.7552° to -75.7937° W.
Potential distribution modeling. We made the potential distribution model using MaximumEntropy Modeling software (Maxent) (Phillips et al., 2006). We applied the criteria of fitting the model by modifying the parameters of Shcheglovitova & Anderson (2013). We filtered the occurrence records to reduce the autocorrelation effects, considering the common bias when using a data sampling from zoological collections; the autocorrelation can induce an environmental bias that affects the model complexity. We compare four different models:Linear plus quadratic (L_Q), Auto Features (AF), Quadratic (Q), and Linear (L); using three different regularization values (0.25, 1, 2) (Phillips et al., 2004; 2006) to evaluate the best performance using the metric of the Area Under Curve (AUC) (Swets, 2014), according to the recommendations for datasets of less than 10 records (Anderson & Gonzalez, 2011).
Maxent can produce over-fitted predictions when imprecise occurrence records are used (Peterson et al., 2011). When over-fitting occurs, the obtained model is more complex than the real relationship between the evaluated variables and the niche of the species (Peterson et al., 2011). For this reason, we included only one record from each locality, thus avoiding including two very close records with similar environmental characteristics, which can increase the chance of overfitting (Veloz, 2009). We used only the records with a linear distance of more than 1 km from their closest neighbor. Considering the low dispersion recorded for Amblypygi (Weygoldt, 2000), the distance between records must be coherent to the dispersion capacity of the species (Shcheglovitova & Anderson, 2013). We calculated the distance between each pair of localities and identified all clusters of localities containing pairwise distances less than or equal to 1 km; for each such cluster, we determined all possible deletions that would yield a smaller cluster with pairwise distances of at least 1 km; we selected the largest number of optimal records using a random selection system (Jackknife). From the 38 records of H. boterorum in Colombia (Vásquez Palacios et al., 2019), we removed 27 duplicated records, conserving nine records after filtering. After a taxonomic check, some of the records from Vasquez-Palacios et al. (2019) were excluded because they may be a new species.
The dataset was divided into two groups: 75 % of the data were used for the prediction of the model and 25 % for its validation (Fielding & Bell, 1997; Guisan & Zimmermann, 2000; Roa et al., 2015).. We used a background grid of 10 000 points for Colombia. We used the default values according to Shcheglovitova & Anderson (2013). To generate the map of predicted distribution, we assigned a threshold, which is defined as the maximum sum of the more sensitive specificity (Elmore et al., 2003; McBride & Ebert, 2000; Saseendran et al., 2002). The probabilities above the threshold took a value of one (red painted area Figure 2) and those with a lesser value took a value of zero green painted areas Figure 2).
Table. 1 Climatic and topographic variables included in the distribution model (from Worldclim and USGC).
Climatic variables (Worldclim 1.4 et al.) (Hijmans et al., 2005) |
Bio_1. Average annual temperature (°C) |
Bio_2. Temperature oscillation during the day (°C) |
Bio_3. Isothermality (°C) |
Bio_4. Temperature stationarity (ºC) |
Bio_5. Average maximum temperature during the hottest season (°C) |
Bio_6. Average minimum temperature during the coolest season (°C) |
Bio_7. Annual temperature oscillation (°C) |
Bio_8. Average temperature during the rainiest trimester (°C) |
Bio_9. Average temperature during the driest trimester (°C) |
Bio_10. Average temperature during the hottest trimester (°C) |
Bio_11. Average temperature during the coolest trimester (°C) |
Bio_12. Annual precipitation (mm) |
Bio_13. Precipitation during the rainiest season (mm) |
Bio_14. Precipitation during the driest season (mm) |
Bio_15. Precipitation stationarity (mm) |
Bio_16. Precipitation during the rainiest trimester (mm) |
Bio_17. Precipitation during the driest trimester (mm) |
Bio_18. Precipitation during the hottest trimester (mm) |
Bio_19. Precipitation during the coolest trimester (mm) |
Topographic variables |
alt. Elevation digital model (USGS, 2001) |
National park. Parques.shp |
Regional Natural Parks. Parque_Natural_Regional.shp |
Districts of soil conservation. Distrito_de_Conservacion_de_Suelos.shp |
Regional Protective Forest Reserve. Reserva_Forestal_Protectora_Regional.shp |
National Protective Forest Reserve. Reserva_Forestal_Protectora_Nacional.shp |
Environmental variables. We selected layers of environmental variables from the database of the WorldClim (Booth et al., 2014), 19 climatic variables with a resolution of 30 s (Hijmans et al., 2005; Naimi & Araújo, 2016), and one elevation variable, also with a resolution of 30 s (Elevation digital model: USGS, 2001/ https://lta.cr.usgs.gov/HYDRO1K; Table 1). These variables represent the climatic seasonality and environmental limiting conditions considered as factors that influence biodiversity by conditioning water and energy availability in the ecosystems (Hawkins et al., 2003). Finally, we performed a jackknife test in MaxEnt, to estimate the relative contribution of each variable to the generated models (Elith et al., 2010, 2011; Phillips & Dudík, 2008) (Figures 1, 2 et al.).
Environmental variables. We obtained layers from the Sistema de Información Ambiental de Colombia (SIAC/http://www.siac.gov.co/) with information on conservation areas in the country. According to the SIAC (2020), Colombia considers there to be five main categories of conservation areas: National Natural Parks, Natural Regional Parks, National Protector Forest Reserves, Regional Protector Forest Reserves, and Soil Conservation Districts. We used the “Intersect” tool from ArcGis to observe the overlap between the potential distribution of H. boterorum and the conservation areas in Colombia, according to these categories. For this analysis, we include the regions with an occurrence probability greater than 0.5 (Figure 3 and Table 2).
Results
The potential distribution model obtained a high fitting level (Average Test AUC=0.97, prevalence probability= 0.04). All the evaluated regularization values showed high performance in the four models (Anderson & Gonzalez, 2011; Shcheglovitova & Anderson, 2013). The lowest regularization values (0.25) showed the highest AUC performance. According to the AUC criteria, the best models were the Linear (0.96), and the model of Auto Features (0.96). Maxent chose model L, by considering it as the most parsimonious.
The environmental variables that best explain the distribution of H. boterorum were Precipitation during the driest season (Bio_14), Average temperature during the driest trimester (BIO_9), Average annual temperature (BIO_1), Annual precipitation (BIO_12), Annual temperature oscillation (BIO_7), Average temperature during the coolest trimester (BIO_11), Precipitation during the rainiest season (BIO_13), and Precipitation stationarity (BIO_15). The influence of other variables is provided in Figure 1.
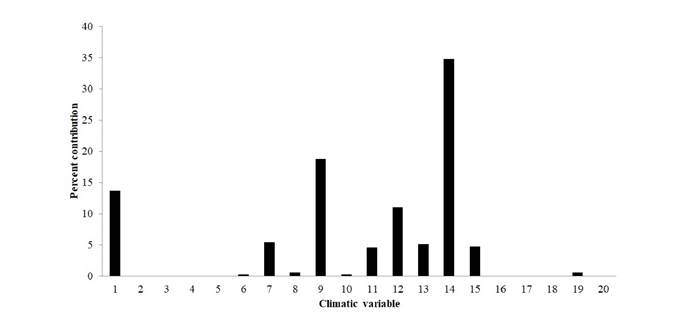
Figure 1 Contribution percentage to the potential distribution model of Heterophrynus boterorum. Average annual temperature (°C) (BIO_1), Temperature oscillation during the day (°C) (BIO_2), Isothermality (Bio_3), Temperature stationarity (ºC) (BIO_4), Average maximum temperature during the hottest season (°C) (BIO_5), Average minimum temperature during the coolest season (°C) (BIO_6), Annual temperature oscillation (°C) (BIO_7), Average temperature during the rainiest season (°C) (BIO_8), Average temperature during the driest trimester (°C) (BIO_9), Average temperature during the hottest trimester (°C) (BIO_10), Average temperature during the coolest trimester (°C) (BIO_11), Annual precipitation (mm) (BIO_12), Precipitation during the rainiest season (mm) (BIO_13), Precipitation during the driest trimester (mm) (BIO_14), Precipitation stationarity (mm) (BIO_15), Precipitation during the rainiest trimester (mm) (BIO_16), Precipitation during the driest trimester (mm) (BIO_17), Precipitation during the hottest trimester (mm) (BIO_18), Precipitation during the coolest trimester (mm) (BIO_19), Elevation digital model (alt_20).
In the potential distribution model (Figure 2), the red areas represent regions with the highest occurrence probability. H. boterorum could live mainly along the center of the Cordillera Central and Occidental, as well as in other small regions along both Cordilleras. Also, there is an area with high probability at the west side of the center of the Cordillera Oriental. The foothills showed a lower occurrence probability (≤ 50 %). In eastern Colombia, in the department of Guainía, there is a region with a high occurrence probability, which could be related to some similarities in the environmental conditions.
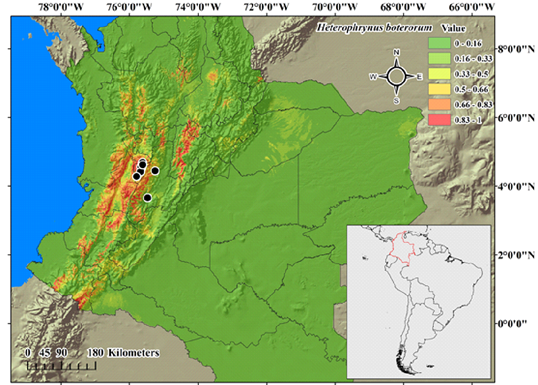
Figure 2 Potential distribution model of Heterophrynus boterorum. The color scale indicates the occurrence probability (from green = 0 to red= 1). Black dots indicate known distribution records.
Distribution model and conservation areas in Colombia. A small portion of the potential distribution of H. boterorum coincides with different areas of conservation in Colombia, mainly with National Natural Parks, and National Protective Forest reserves. However, most of the currently known records for the species are outside of the protected areas. Only some individuals from the Quindío region were collected in a protected area, the Barbas-Bremen Soil Conservation District, which is considered as a water source for the department.
H. boterorum could potentially be distributed in 137 Colombian conservation areas: 24 National Natural Parks, three of them considered as Flora and Fauna Sanctuaries, and one considered as a Flora Sanctuary, 38 Natural Regional Parks, 30 National Protector Forest Reserves, 37 Regional Protector Forest Reserves, and eight Soil Conservation Districts. Only 103 of the areas of the potential distribution have an overlap with the conservation areas greater than 50 % (Figure 3, Table 2).
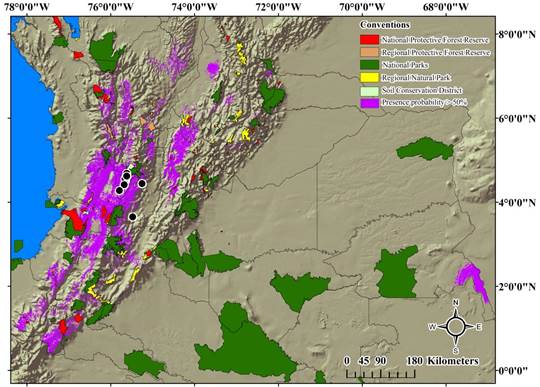
Figure 3 Potential distribution model of H. boterorum over the National Protected Areas System of Colombia. Red areas: National Protective Forest Reserves. Orange Areas: Regional Protective Forest Reserves. Green areas: National Natural Parks. Yellow areas: Regional Natural Parks. Light green areas: Districts of soil conservation. Purple areas: Potential distribution. Black dots: known distribution records.
Table 2 Conservation areas in Colombia with overlap with the potential distribution of H. boterorum. The number of areas with overlap>10 % and > 50 % is indicated, as well as the overlap portion (h= hectares).
Types of Conservation Areas | No. of Areas with Overlapping > 10 % | Area (H) | No. of Areas with Overlapping > 50 % | Area (H) |
---|---|---|---|---|
Conservation districts | 8 | 0.05 | 1 | 0.03 |
Natural regional parks | 38 | 0.49 | 24 | 0.08 |
National natural parks | 20 | 2.15 | 17 | 0.22 |
National protector forest reserves | 30 | 0.39 | 23 | 0.14 |
Regional protector forest reserves | 37 | 0.10 | 28 | 0.02 |
Flora sanctuaries | 1 | 0.01 | 1 | 0.00 |
Flora and fauna sanctuaries | 3 | 0.01 | 2 | 0.00 |
Total | 137 | 3.19 | 103 | 0.49 |
Discussion
The MaxEnt algorithm works better the more records are used, with poor data the predictions of presence could be overestimated. However, the software usually provides good results even with few records. In this case, MaxEnt can be used as a tool to accelerate the new population finding process, considering the difficulty of localizing individuals of species as those of Amblypygi. Also, it was useful to know which variables are predictors of the presence of H. boterorum (Barba Hernandez et al., 2017; Varela et al., 2014)
The species H. boterorum is a good case study to explore the utility of the potential-distribution models in a conservation frame work. This species has a poorly known distribution, like many other Amblypygi. However, it has high importance as an endemic species, and could even be a complex species considering its morphological variation (Vásquez Palacios et al., 2019). It is useful to know other possible places to search for more populations and to evaluate its current protection status.
This is the first research with a niche modeling focus on Amblypygi; and as was expected, the environmental variables which condition their occurrence are those associated with precipitation, and thence with temperature and humidity. Most of the model’s prediction is based on precipitation and temperature during the driest season, which is coherent with the idea that the Amblypygi depends on environment stability for having correct body thermoregulation, as well as the fact that dry environments are not favorable for this group of arachnids (Weygoldt, 2000).
The potential distribution model showed that the localities near the currently known distribution have a high occurrence probability. However, there are other small regions along the Andes in which the probability is also high, which is feasible with the possible expansion of the species, considering other known distributions of the species of the genus (Chirivi-Joya et al., 2020; Harvey, 2003). The occurrence prediction for the Guainía region could be related to the model parameters. However, the endemic species in the family Phrynidae do not have distributions as wide or interrupted as in the prediction (Harvey, 2003). It is difficult, but not impossible, that H. boterorum could be present in the Guainía region. The records of Heterophrynus for eastern Colombia correspond to Heterophrynus batesii and Heterophrynus origami (Chirivi-Joya et al., 2020). Most of the Cordillera Oriental presents a low occurrence probability which could be related to the environmental differences with the Cordillera Central (Bürgl, 2017; Churchill, 1995; Gentry, 1982; Gómez et al., 2015), with the model considering mainly the best-sampled localities and nearby sites as a base for the predictions (Elith et al., 2010). According to the potential distribution, the environmental conditions would allow communication between populations. However, the high occurrence probability does not guarantee the presence of the H. boterorum, as other non-considered variables, could also be determinant factors. The obtained model can be improved in the future, adding new population records and adding other environmental parameters. Our contribution can help to make sampling efficient and by determining other environmental variables that possibly affect this species’ geographic range.
Most of the recorded populations of H. boterorum are outside protected areas in Colombia. It is necessary to consider that the potential distribution can mean that the species is present in the predicted localities, but still not recorded; but it also could mean that the species could be present there in the future (Drew et al., 2010). It is important to corroborate the presence of the species in the more likely localities, and also to consider these places as possible refuges. Currently, there is a lack of Amblypygi distribution information in Colombia, especially about H. boterorum, while many of the protected areas included in its potential distribution have never been sampled searching for Amblypygi, or, at least, there are no records in the main Colombian arachnological collections (Chirivi-Joya et al., 2020; Joya & de Armas, 2012). Furthermore, nowadays there is no connection between the protected areas, and there is no protection in the areas that connect the recorded populations of H. boterorum and the possible refuge areas where the species could live. Considering the accelerated deforestation of unprotected areas in Colombia (http://www.ideam.gov.co/web/bosques/deforestacion-colombia), the populations of H. boterorum could be in an anthropic isolation process. This contribution could be used first for searching for more populations of the species, and as a basis for creating strategies to establish connectivity between the populations and the protected areas.