INTRODUCTION
Precision agriculture is a holistic, data-driven systems approach aimed at reducing input costs, improving yield and quality, and increasing sustainability in agricultural production using a series of emerging technologies, particularly information technologies (Zhang et al., 2002; Saiz-Rubio and Rovira-Más, 2020; Lee et al., 2021). Its development has been made possible by multiple innovations, including global positioning systems (GPS), geographic information systems (GIS), miniaturized computer components, automatic controls, field and remote sensors, mobile computing, advanced information processing, and telecommunications (Zhang et al., 2002). Precision agriculture includes agriculture 4.0, smart farms, digital agriculture, industry 4.0 in agriculture, the fourth agricultural revolution, Internet of Things (IoT), and agriculture 5.0 (da Silveira et al., 2021; Roy and De, 2022). Sustainable agriculture in the future will rely on the incorporation of robotic solutions and artificial intelligence techniques aimed at supporting unmanned operations and autonomous decision support systems (Saiz-Rubio and Rovira-Más, 2020). The integration of these technologies into agricultural production has been associated with increased productivity, profitability, and farm income by enabling more efficient resource utilization (Cisternas et al., 2020).
In avocado crops, precision agriculture technologies are increasingly being applied to optimize production and mitigate risks. These applications include remote imaging techniques for diagnosing and monitoring pathogens in crop fields, which offer reliable and affordable plant disease management solutions (Pérez-Bueno et al., 2019). Additionally, image processing and convolutional neural networks (CNNs) are being used to recognize diseases, potentially replacing traditional manual inspection in extensive plantations (Mejiá-Cabrera et al., 2020). Moreover, soil moisture measurement tools, such as frequency domain reflectometry (FDR), are being employed to ensure efficient water management (Moreno-Ortega et al., 2019). Lastly, early warning systems based on IoT sensors, data analytics, and mobile apps are being developed to provide farmers with timely alerts regarding potential pest outbreaks, diseases, or adverse weather conditions that may impact their avocado crops (Ramirez-Guerrero et al., 2023).
The growing global demand for avocados has positioned this tropical fruit as a profitable and expanding agribusiness (FAO, 2022; Ramirez-Guerrero et al., 2023). Avocado production is mainly concentrated in the Latin American region, with Mexico leading production at 2.44 million tons per year, followed by Colombia with 0.98 million tons and Peru with 0.77 million tons (FAOSTAT, 2022). The growth of the avocado market has been attributed, in part, to the multiple uses of avocado in the food, pharmaceutical, and cosmetic industries as well as to the growth of imports by industrialized countries (Duarte et al., 2016).
To fully capitalize on the potential growth of the avocado agribusiness, adopting technologies that enhance productivity is essential (Arias et al., 2021). Emerging technologies are poised to play a crucial role in optimizing farm performance and meeting market demands. By leveraging these advancements, farmers can make data-driven decisions that improve crop management, resource allocation, and overall efficiency. The integration of precision agriculture tools, such as remote sensing, data analytics, and automated systems, enables growers to monitor crop health, optimize inputs, and respond to changing conditions in real-time (Saiz-Rubio and Rovira-Más, 2020). By embracing these technologies, the avocado industry can unlock its full potential, ensuring sustainable growth and competitiveness in the global market.
Previous literature reviews have analyzed the application of emerging technologies in the technical management of avocado crops (Erazo-Mesa et al., 2022; Ramirez-Guerrero et al., 2023). However, there is a lack of emphasis on the overall trends in technological development within the avocado production sector. While some studies have identified general trends in research production and industrial development in the avocado sector (Cáceres-Zambrano et al., 2022a), there is a need for a more in-depth and updated analysis of these trends, specifically in the context of precision agriculture and related terms in avocado production. This study aimed to address this gap by employing bibliometric and patentometric techniques to provide a comprehensive overview of the current state and future directions of research and technological advancements in avocado production.
MATERIALS AND METHODS
This review aimed to identify trends in scientific and technological developments related to precision agriculture in avocado production. The study followed the Proknow-C (Knowledge Process Development-Constructivist) tool, focusing on (i) the selection of information sources, (ii) bibliometric analysis, and (iii) systematic analysis (Lacerda et al., 2012; Santos et al., 2015). The methodology was complemented by the PRISMA protocol’s statements and guidelines, which specify the eligibility criteria, information sources, search strategy, selection process, data collection process, data analysis, and reporting bias assessment (Page et al., 2021). Three general criteria were employed for both scientific documents and patent searches: (i) documents addressing precision agriculture and related terms, (ii) scientific documents and patents focusing on primary production (from planting to harvesting), and (iii) documents written in English. Figure 1 depicts the process followed from data collection to analysis.
Selection of information sources
Scopus was employed to search for scientific articles, while Lens was utilized for patents, mainly due to the multidisciplinary nature of these bases and their broad coverage. The keywords for searching in Scopus and Lens were defined according to the objective of this research. Following the Proknow-C methodology, the adherence test was conducted to establish the relevance of the keywords, as shown in table 1. This test considered that adherent keywords or those with a higher number of results significantly represent the study area (Ruthes and Silva, 2015). Scopus, ScienceDirect and Google scholar databases were used for this test, since it is only necessary to show the number of documents that mention these words.
Table 1. Keyword adherence test* in databases.
Keywords | Scopus | Science Direct | Google Scholar |
---|---|---|---|
Precision agriculture | 12,094 | 7,992 | 174,000 |
Agriculture 4.0 | 408 | 289 | 6,690 |
Smart farming | 2,297 | 1,430 | 30,400 |
Digital agriculture | 768 | 726 | 15,900 |
Agriculture 5.0 | 32 | 37 | 1,150 |
Digital farming | 169 | 162 | 3,890 |
Avocado | 6,307 | 16,599 | 325,000 |
Persea americana | 3,295 | 2,812 | 83,400 |
Total | 25,370 | 30,047 | 640,430 |
* Only the results of the selected keywords are shown.
The search in Scopus used the following search equation, covering the period from January 2012 to February 2024: (ALL (“precision agriculture” OR “agriculture 4.0” OR “smart farm*” OR “digital agriculture” OR “agriculture 5.0” OR “digital farm*”) AND TITLE-ABS-KEY (avocado OR “Persea americana”)). The search was conducted on February 16, 2024. Only articles, conference papers, reviews, and books were considered, resulting in a total of 84 documents, with one data paper being eliminated.
The patent search in Lens used three search equations to conduct a general scan of precision agriculture and a specific analysis of its application in avocado (Tab. 2). All searches were conducted in the fields of title, abstract, and claims, selecting only the document type corresponding to patent applications covering the period from January 2012 to February 2024.
Table 2. Search equations for patents in Lens.
Search equation | Documents |
---|---|
(Title: (“precision agriculture”) OR (Abstract: (“precision agriculture”) OR Claims: (“precision agriculture”))) | 390 |
Title: (“digital agriculture”) OR (Abstract: (“digital agriculture”) OR Claims: (“digital agriculture”)) | 69 |
Title: (“precision agriculture”) OR (Abstract: (“precision agriculture”) OR (Claims: (“precision agriculture”) AND (Title: (avocado) OR (Abstract: (avocado) OR Claims: (avocado))) | 54 |
Total | 513 |
Bibliometric analysis
Bibliometric analysis involves applying quantitative and statistical methods to bibliographic data to study the structure and evolution of scientific fields or research domains (Aria and Cuccurullo, 2017; van Eck and Waltman, 2014). The Rayyan web application (Ouzzani et al., 2016) was used to determine the relevance of scientific documents, while the Lens.org website was employed for patent relevance review, based on the general criteria and the objective of the study.
The Bibliometrix library of the R Project statistical software (Aria and Cuccurullo, 2017) and VOSviewer software (van Eck and Waltman, 2014) were utilized for bibliometric analysis. The Biblioshiny interface of R generated indicators for (i) annual document production, (ii) main information sources, and (iii) scientific production by country. VOSviewer was used to cluster keywords and visualize their correlations or co-occurrences for both scientific production and patents, considering keywords appearing in at least five publications, with a total of 24 selected keywords. Patentometric analysis focused on indicators related to (i) annual patent applications, (ii) patent applications by country, (iii) holding companies, (iv) main patent classification codes, and (v) subtopics in the area through keyword network analysis.
These tools allowed mapping the structure of research and patent production about precision agriculture in avocado, identifying influential works and authors and detecting research fronts and emerging trends.
RESULTS AND DISCUSSION
Scientific landscape
The scientific production of precision agriculture in avocado production showed an upward trend (Fig. 2), indicating its scientific importance. However, there was evidence of a low number of published documents (83) compared to other fruits, suggesting that it is still an emerging research field, especially considering the significant increase in publications in 2023. Cisternas et al. (2020) conducted a systematic review on the implementation of precision agriculture technologies in different crops (23 in total), including maize, sugarcane, wheat, cotton, grapes, and soybeans, but reported no documents on avocado. Similarly, Zude-Sasse et al. (2016) analyzed precision agriculture applications in fruit trees, vegetables, and other horticultural crops but did not report any research on emerging technologies in avocado. Lee et al. (2021) conducted a review of the attributes stimulating the adoption of precision agriculture techniques in different agricultural systems, and of the 33 documents analyzed, only one was focused on avocado. One reason for the low reporting of precision technologies in avocado is that unlike other fruits with a relatively long production history, avocado production has experienced rapid growth only in the last two decades, with production increasing from 1.69 to 4.20 million tons between 2011 and 2021 (FAOSTAT, 2022).
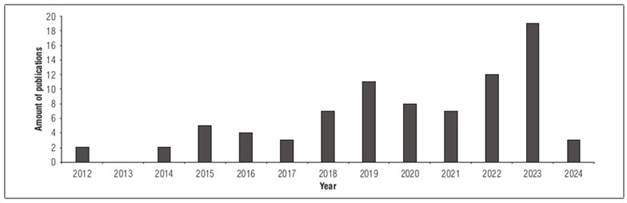
Figure 2. Scientific production about precision agriculture on avocado production from 2012 to 2024.
The three main journals addressing precision agriculture in avocado production were Remote Sensing, Computers, and Electronics in Agriculture, and Agriculture (Switzerland). According to Scimago (2023), Remote Sensing was classified as Q1 and is part of the MDPI publishing group. The journal covers scientific information related to remote sensing science in various application sectors, including earth and planetary sciences. Computers and Electronics in Agriculture was also classified as Q1 and is published by Elsevier. It covers topics related to the use of computers or electronics in agricultural sciences as well as elements of precision agriculture. Agriculture (Switzerland), also part of the MDPI publishing group, was classified as Q2 and publishes on a variety of interdisciplinary topics, including agricultural technology, such as machinery, remote sensing, and GIS (Tab. 3).
Table 3. Main journals publishing on precision agriculture in avocado production from 2012 to 2024.
Journal | Documents |
---|---|
Remote Sensing | 12 |
Computers And Electronics in Agriculture | 11 |
Agriculture (Switzerland) | 4 |
Agronomy | 2 |
Biosystems Engineering | 2 |
Crop Protection | 2 |
Food And Bioprocess Technology | 2 |
Horticulturae | 2 |
Irrigation Science | 2 |
Plant Disease | 2 |
Sensors | 2 |
Sustainability (Switzerland) | 2 |
Others | 38 |
Total | 83 |
In terms of researchers, seven authors were highlighted based on their number of publications (Fig. 3). The most active author was Andrew Robson, associated with the Precision Agriculture Research Group, School of Science and Technology, University of New England, Australia. Robson published with Kasper Johansen, Stuart Phinn, and Yu-Hsuan Tu. This collaboration can be seen in the work of Tu et al. (2019), which used multispectral images acquired from an unmanned aerial system (UAS) to measure the structural attributes of avocado trees. Robson et al. (2017) utilized WorldView satellite images to estimate preharvest yield in avocado. Another study in which Robson participated was Salgadoe et al. (2018), which used image analysis (photos taken with Flir One camera and WorldView-3 satellite images) to quantify the severity of Phytophthora root rot.
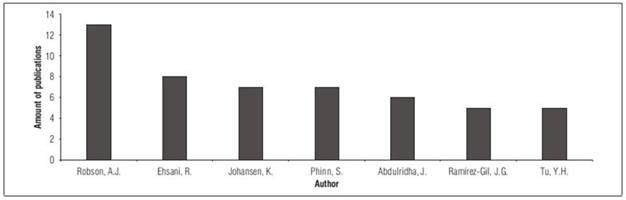
Figure 3. Researchers with the highest number of publications about precision agriculture on avocado production from 2012 to 2024.
Other identified authors included Reza Ehsani and Jaafar Abdulridha. Abdulridha was affiliated with the Agricultural and Biological Engineering Department, Southwest Florida Research and Education Center, University of Florida, United States, and Ehsani was affiliated with the University of California, United States. Some of the work of this group included the use of a portable spectral data collection system to identify avocado trees affected by laurel wilt and nutritional deficiencies (De Castro et al., 2015; Abdulridha et al., 2018). Joaquín Ramírez-Gil from the Universidad Nacional de Colombia, Colombia, published studies related to the adoption of technologies in avocado production systems (Cáceres-Zambrano et al., 2022b).
The most cited article in precision agriculture for avocado production is “Comparison of convolutional neural networks in fruit detection and counting: A comprehensive evaluation” by Vasconez et al. (2020), with 106 citations; however, this work does not belong to any of the most active researchers. Among the most active researchers, the top-cited works include: (i) “A remote sensing technique for detecting laurel wilt disease in avocado in presence of other biotic and abiotic stresses”, co-authored by Reza Ehsani and Jaafar Abdulridha, with 80 citations (Abdulridha et al., 2019); (ii) “Assessing radiometric correction approaches for multi-spectral UAS imagery for horticultural applications”, authored by Yu-Hsuan Tu, Kasper Johansen, Stuart Phinn, and Andrew Ronson, with 68 citations (Tu et al., 2018); and (iii) “Design of electronic devices for monitoring climatic variables and development of an early warning system for the avocado wilt complex disease”, co-authored by Joaquín Ramírez-Gil, with 17 citations (Ramírez-Gil et al., 2018).
The scientific production of precision agriculture in avocado production has been distributed across several countries (Fig. 4). The country with the highest activity was Australia, followed by the United States, Colombia, Chile, Spain, and Mexico. Studies were mainly conducted in industrialized countries (Australia, the United States, and Spain) and some middle-income countries (Colombia, Chile, and Mexico). This could be related to the fact that farmers in high-income countries have a greater capacity to implement precision technologies. For example, Rogovska et al. (2019) argued that one of the reasons for farmers’ low adoption of big data tools is the high cost of such technology. This situation underscores the need to promote the adoption of precision technologies in the main producing countries, most of which are in Latin America, to improve efficiency in avocado production (Erazo-Mesa et al., 2022; Ramirez-Guerrero et al., 2023). This research interest may also be driven by the fact that avocado production is a significant economic sector in terms of production and exportation, in countries including Mexico, Peru, Chile, and Colombia (Schwartz et al., 2018; Builes and Duque, 2020; Díaz, 2021).
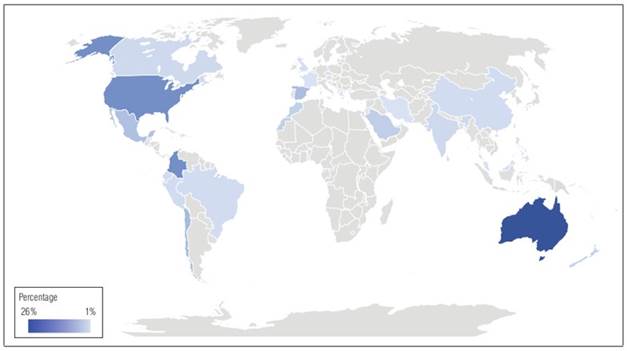
Figure 4. Map of scientific production about precision agriculture on avocado production from 2012 to 2024.
The analysis of keywords and their correlations revealed three clusters, as shown in figure 5. The first group, represented in red, included 11 keywords: avocado, decision trees, detection method, fruit, fruits, image analysis, infrared devices, plants (botany), remote sensing, and the United States. This group was called “remote sensors”, which refers to a series of systems or instruments for obtaining rapid and viable information about an object, area, or phenomenon from a distance (Veneros et al., 2020). These findings are consistent with those of Cisternas et al. (2020), who reported that the main precision agriculture technologies were remote sensors, followed by GPS, wireless network sensors, unmanned aerial vehicles (UAVs), and sensors in general.
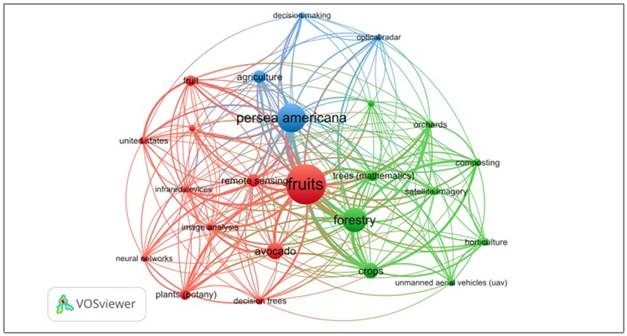
Figure 5 Analysis of keywords and their correlations about precision agriculture on avocado production from 2012 to 2024.
The second group, represented in green, encompassed nine terms: composting, crops, forestry, horticulture, image processing, orchards, satellite imagery, trees (mathematics), and UAVs. This group was called “sensor image analysis”. One related study in this group included that of EL Amraoui et al. (2022), who provided images taken from UAVs for tree segmentation, counting, and classification based on tree canopy area, disease detection, and the application of artificial intelligence for image classification, among other uses.
The third group, represented in blue, consisted of four terms: agriculture, decision making, optical radar, and Persea americana. Optical radar might be related to LiDAR (Light Detection and Ranging), since this technology is essentially an optically sensing method that uses laser light pulses to measure distances. This group was called “LiDAR”. Rivera et al. (2023) stated that LiDAR is a versatile remote sensing technology that utilizes pulsed laser light to measure variable distances, enabling its application in various precision agriculture tasks. By employing different types of LiDAR sensors, such as Airborne Laser Scanners (ALSs), Mobile Laser Scanners (MLSs), and Terrestrial Laser Scanners (TLSs), farmers and researchers can monitor crop health, evaluate soil properties, optimize fertilizer application, and estimate yields with high accuracy. For instance, TLSs have been used to digitize avocado tree structures, paving the way for automated pruning recommendations based on factors like sunlight penetration. Torres-Madronero et al. (2023) contributed to this research area by performing a spectral characterization of avocado crops. They utilized an optical sensor called GreenSeeker to conduct their study.
To identify the latest trends in precision agriculture for avocado, a comprehensive review of the most recently published documents was conducted, focusing on the period from January 2023 to February 2024. The same search criteria and equation as described in the methodology section were employed. As a result, six documents were found that met the selection criteria and contributed to the understanding of current developments in this field. Two subthemes were identified in these publications regarding the use of precision agriculture: (i) data and tools for fruit recognition and classification, and (ii) data collection and analysis for crop management.
The first document proposed a multilevel method for fruit classification, including avocado, and automated identification of diseases in these products using image processing (Mehmood et al., 2023). Similarly, Tran et al. (2023) presented a method for fruit classification (apple, avocado, dragon fruit, guava, and mango), emphasizing speed and cost effectiveness. This method was based on a low-cost device called a Vector Network Analyzer (VNA), achieving high accuracy in the classification process (over 98.7%). Abdulsalam et al. (2023) introduced a multimodal fruit dataset called Fruity for the recognition and visual representation of these products as part of collection and selection processes. Data were collected using different sensors and recorded with platforms such as a robotic manipulator and UAV, potentially to be used in an autonomous fruit-picking and harvesting tool.
Ramirez-Guerrero et al. (2023) performed a literature review of emerging technologies for identifying agroclimatic and phytosanitary events in avocado crops. They found that the most used technologies were based on machine learning algorithms, such as convolutional neural networks as well as image capture using sensors, such as cameras, for data collection aimed at pest control, humidity, and temperature monitoring. Following the same orientation, Moreno-Bernal et al. (2023) proposed an IoT-based platform to collect and process soil nutrient and meteorological information in avocado crops in Mexico. The sixth document by Yábar-Gamarra et al. (2023) developed a radiofrequency robot for data collection and analysis of soil conditions in avocado crops, primarily targeting small- and medium-sized producers and featuring an easy-to-use interface and image visualization for decision-making.
Technological landscape
Patent applications related to “precision agriculture” showed a growing trend between 2012 and 2022. In 2021, the highest record was observed with 93 patent applications, as shown in figure 6. China led patent applications with 197 applications, followed by the United States with 83 applications. The three main patent-holding companies were DTN LLC, ClearAg INC, and Iteris INC. DTN LLC, an American company, offers innovations related to agricultural intelligence data in its agriculture section (DTN, 2023a). ClearAg INC, a subsidiary of DTN offers ClearAg Viewer, a subscription service providing information on weather, soil conditions, crop growth, irrigation conditions, water management, irrigation, and harvest optimization modeling, among other uses (DTN, 2023b). Iteris INC, another American company, provides software, hardware, and services for intelligent mobility infrastructure management, particularly in agriculture. This company developed products to determine the best timing for planting, harvesting, fertilizing, and treating crops based on predicted soil and atmospheric conditions and crop moisture levels (White, 2015).
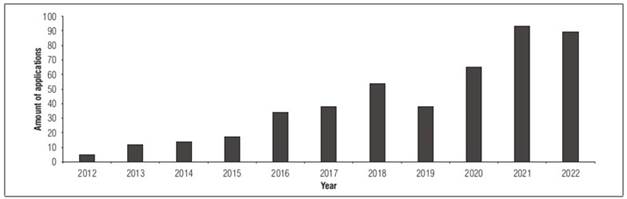
Figure 6. Patent applications related to “precision agriculture” and “digital agriculture” from 2012 to 2022.
In terms of patents related to “digital agriculture”, the Climate Corporation stands out as a leader in patent applications. The Climate Corporation, a subsidiary of Bayer, offers innovations related to data science for crop management, crop health analysis, and vegetation mapping using satellite imagery and weather forecasts (The Climate Corporation, 2023). In patents related to “precision agriculture” and “avocado”, the Stout Industrial Technology INC., based in the United States, stands out. The company holds patents related to crop-tracking systems using artificial vision and a modular smart implement for precision agriculture (USPTO, 2023).
In terms of the International Patent Classification (IPC) codes for the first and second search equations, the most representative was “G06Q50/02”, which refers to “information and communication technology” in agriculture. Additionally, “A01B79/00” is significant, involving “methods for soil working that essentially require the use of particular machines”. In the third search equation, 47 results were found during the observation period. The two most representative patent classification codes were “G06K 9/00”, referring to “methods or arrangements for reading or recognizing printed or written characters or for recognizing patterns”, and “A01B79/00”, which involves “methods for soil working that essentially require the use of particular machines”. Although there was not a clear upward trend in patent applications in avocado production, in the last decade, the number of applications was above 5 per year (Fig. 7).
Co-occurrence analysis of the keywords used in patent applications related to “precision agriculture” and “avocado” revealed two clusters (Fig. 8). The first group, identified in red, had eight items. A patent associated with this group was “US 2018/0330487 A1”, which detailed a computer platform technology to predict output conditions, such as diseases, salt damage, soil issues, water leaks, and generic abnormalities in crops (Cohen, 2018). The second group, represented in green, had three words. A patent included in this cluster was “US 2019/0059203 A1”, a system and method for determining fertilizer application rates for spatial distribution of a product (Staples et al., 2019).
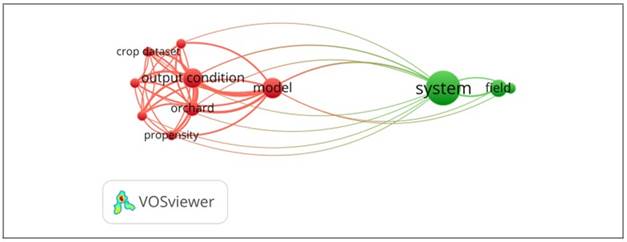
Figure 8. Analysis of keywords and their correlations in patents related to precision agriculture and avocado from 2012 to 2022.
Nine recent patent applications (January 2023-February 2024) related to “precision agriculture” and “avocado” were found. Notably, the agricultural technology manufacturers Stout Industrial Tech INC from California (United States) submitted three documents, and Maschio Gaspardo from Spain submitted two patents. Two of Stout Industrial Tech INC’s patents corresponded to the same invention but were filed through two different patent offices (United States and European Office); the same applies to Maschio Gaspardo’s two documents. Therefore, there were seven recent patent applications on the subject. Table 4 presents the main technological areas addressed by the most recent patent applications. These areas focus on crop-related activities in conjunction with precision agriculture techniques, such as image capture and processing. The patent of Jezewski (2023) proposed a crop surveillance and management system activated by energies from various sources and was thus considered a hybrid system. The patent of Jeong et al. (2023) showed a system and apparatus based on drone use for precision agriculture in extensive areas. Antle and Snyder (2023)’s patent introduced an intelligent atomizer for digital agriculture, enabling the application of substances for various crop treatments in specific locations. Another patent presented a lifting system for a processing device included in a precision agriculture vehicle (Marconi et al., 2023). Donadon et al. (2023) had a patent for a device that is part of a precision agricultural seeder that can select the seeds to be sown. Gokcebag et al. (2023) introduced a precision agriculture kit for automated pest monitoring, utilizing wireless IoT-enabled devices and integrated cameras for detection and counting. Grant et al. (2023)’s patent proposed a plant tracking system using computer vision that detects objects of interest in the field, captures images, and later processes them through a neural network-based controller.
Table 4. Main technological areas of the most recent patents on precision agriculture and avocado.
IPC | Description | Documents |
---|---|---|
A01B79/00 | Methods for working soil | 3 |
A01B63/00 | Lifting or adjusting devices or arrangements for agricultural machines or implements | 2 |
A01C7/04 | Single-grain seeders with or without suction devices | 2 |
A01M7/00 | Special adaptations or arrangements of liquid-spraying apparatus for purposes covered by this subclass | 2 |
G06V10/82 | Neural networks (NN) specially adapted for image or video recognition or understanding | 2 |
G06V20/10 | Terrestrial scenes (scenes under surveillance with static cameras; scenes perceived from the exterior of a vehicle; scenes perceived from the interior of a vehicle) | 2 |
In summary, the leaders in research on precision agriculture in avocado differ from those in technological development. While the most active author in scientific publications was associated with an Australian institution, the leading patenting company was from the United States. This demonstrates that dominance in scientific developments is not necessarily a guarantee of market dominance. Some of the most relevant topics in scientific production in avocado include remote sensing, image analysis (spectral and satellite), and UAVs, which aligns with findings by Cisternas et al. (2020). In terms of patents, information technologies were the most prevalent in avocado production. One of the approaches identified in recent research has not yet been represented in patent applications, which refers to tools for automating fruit detection and classification. However, the second focus of these recent investigations, related to crop monitoring and management, was clearly represented in the inventions identified in patent documents published in 2023. It is necessary to continue both scientific and technological production as well as the implementation of these innovations to improve profitability and productivity (Saiz-Rubio and Rovira-Más, 2020) and mitigate climate change (Balafoutis et al., 2017) in avocado production.
Future research should prioritize two key areas to advance precision agriculture in the avocado industry. First, it is essential to investigate strategies that encourage avocado farmers, particularly in major production countries, to adopt precision agriculture technologies, leading to data-driven decision-making and increased productivity. Second, there is a need to assess market-ready and commercially available emerging industrial developments specifically designed for avocado production. While scientific research often focuses on prototypes and in-process technologies, as well as on controlled laboratory conditions, examining existing industrial solutions can provide valuable insights into the current state of precision agriculture in the avocado sector and identify areas for further improvement and integration.
The primary limitation of this study lies in the use of a single database for searching both scientific and patent literature, potentially leading to the omission of relevant documents. To gain a more comprehensive understanding of the trends in precision agriculture within the avocado industry, future research should consider expanding the search to include multiple databases and extending the observation period.
CONCLUSION
Scientific and technological production in the field of precision agriculture in avocado is mainly concentrated in high-income countries (United States, Australia, and some European countries) and China, which lead patent applications. These countries are also important avocado importers. Less research and patent productions are reported from avocado producer’s countries (Mexico, Colombia, and Peru). This situation calls for the promotion of public and private investments to close this gap. Research has focused on the application of remote sensing and the analysis of information captured by these devices both in cultivation and harvesting. In terms of technological production, innovations are related to devices/systems for remote sensing, implements for precision agriculture, and systems for analyzing data, mainly oriented towards crop health and nutrition and soil conditions.
It is evident that precision agriculture is a growing field both in research and technological development, making it an area of interest for developed countries, as well as for countries such as Colombia, where avocado is a crop with high economic and social relevance.