1. Introduction
Basic and complex activities of the modern society are characterized by a high dependence on energy resources. According to the International Energy Agency (IEA) [1], energy consumption increased 125.5% from 1973 to 2016; 81% of energy consumed in 2016 was from fossil sources, such as natural gas (22,1%), oil (31,9%) and coal (27,1%); and transportation consumed 64,5% of the fuel oil commercialized that year [1]. Transportation is the largest oil consumer and the second largest source of carbon dioxide emissions in the world, so energy and environmental issues resulting from transportation are becoming increasingly serious [2]. The massive use of vehicles and their dependence on non-renewable energy resources have increased the concentration of CO2 and other contaminant emissions in the atmosphere in recent years, affecting environment and public health. Besides, the emission of carbon dioxide is actively playing its role in global warming and the increase of greenhouse gas emissions, composed of almost 72% carbon dioxide, 18% methane, 9% nitrous oxide and 1% of other gases [3]. The IEA estimated that in 2014, the transportation sector emitted 24% of the CO2 released worldwide [4].
Today, transportation is considered one of the main contributors to environmental decay, because road vehicles are used by a large percentage of the population worldwide. Light Duty Vehicles (LDV), those whose gross weight value is less than 3500 kg according to the European classification, are the main fossil fuel consumers and emitters of contaminants and greenhouse gases worldwide [5]. Due to the global challenge of climate change, air pollution and sustainability issues are of increasing interest in road transport, and in a more general context, in transportation research; considerable efforts have been devoted to innovations which maximize fuel economy and minimize greenhouse gas emissions through optimal fuel consumption estimation [6]. For that reason, different alternatives and programs have been created and implemented by governmental and non-governmental organisms, in order to diminish the fossil fuel consumption of transportation as well as the environmental decaying linked to its use. For instance, the Global Fuel Economy Initiative - GFEI [7], established alternatives to promote fuel economy on LDV, such as: energy efficiency programs, technological updates, and changing modes of transportation. Some other programs include the Corporate Average Fuel Economy (CAFE) of the United States and the LDVs labelling programs of Brazil, Chile and the European Union [8].
Vehicle emission standards are the primary technical policy tools available for mitigating emissions from vehicles. The emission test procedures for light duty vehicles are based on a transient cycle representing driving patterns of a particular country. Light duty vehicle cycles FTP-75 and NEDC are used in US emissions and European test procedures, respectively [9]. In a broad sense, driving cycles are essentially driving patterns that are synthesized to represent average real-world driving. Such synthesized driving cycles are structured as speed-time profiles that constitute snippets of different modes of operations joined up sequentially [10]. The fuel consumption of the vehicles may be indirectly derived from the measurement of carbon dioxide (CO2), hydrocarbons (HC) and carbon monoxide (CO) emissions levels during certification tests, in which vehicles are tested under cycle simulations and chassis dynamometers, while emissions are accumulated and ultimately analysed with a carbon mass balance [11].
As vehicular emissions are a complex phenomenon, regulatory agencies around the world are constantly updating their standard procedures to provide more accurate results for exhaust emissions assessment. The existing emission factors have been developed in the laboratory using dynamometer tests [12], and their regulation presents a greater impact on the peak value of vehicle emissions in the short term [13,14]. However, according to Zhang S et al., [15] discrepancies between real-world fuel and type-approval fuel consumption (also reported as the road-to-lab gap) for light-duty passenger vehicles (LDPVs) have been identified in Europe and China, which is an issue of great concern regarding transportation energy efficiency. These discrepancies have underscored the necessity for more accurate measurement techniques. Therefore, it is extremely important to develop effective eco-planning systems and energy efficiency strategies, such as intelligent transportation systems (ITSs), eco-driving assist systems, and eco-routing assist systems, to help control and reduce fuel consumption. To better evaluate these eco-driving and eco-routing systems, a model that can precisely predict fuel consumption second-by-second is necessary [2].
Energy efficiency programs and strategies based on eco planning systems require improving and constantly updating their measurement procedures, in order to analyze and establish controls over the processes, identify energy losses, and optimize fuel consumption. These procedures depend on accurate measurement systems to assess the performance of processes, to identify the main causes of inefficiencies, opportunities for improvement, as well as the right implementation of energy efficiency strategies. These strategies have to be measured, evaluated, and rearranged in order to maintain constant improvement actions. Consequently, metrology has been identified as a useful tool for the implementation and evaluation of energy efficiency strategies, as the decision-making actions for future scenarios will depend on the measurements that may have been conducted, which illustrate the current state of the systems involved.
Measurement begins with an appropriated specification of the measurand, the method, and the measuring procedure. Besides, measuring should be replicable and accurate in order to guarantee the independence of the results from human error associated with the measurement instruments. This means independence may be reached by quantifying the uncertainty of measurement, which is defined as a parameter associated with the result of measuring, that characterizes the distribution of values that could reasonably be attributed to the measurand [16].
Particularly, Colombia does not have an energy efficiency program that regulates and measures fuel consumption and greenhouse gas emissions for LDV. Though Colombia is not a highly dependent fossil fuel consumer because 70% of its energy is obtained from hydro sources [17], the national transportation sector consumes 44% of the fuel oil commercialized within the country [18].
Consequently, road transportation becomes an important target for researching alternatives to reduce fossil fuel consumption and greenhouse gas emissions in Colombia. Atabani et. al. [8] concluded that fuel consumption and greenhouse gas emissions standardization are an important alternative to improve fuel consumption on LDV. Thus, the development of this methodology for measuring fuel consumption for LDV in Colombia emerged as the first step to initialize the implementation of standards and regulations that Colombia currently has not established, in order to minimize fuel consumption and greenhouse gas emissions by energy efficiency maximization. Even though there exist more advanced and accurate methodologies, technologies, and procedures for measuring fuel consumption, including real-time and dynamic measuring techniques, this methodology was elaborated as one of the first developments regarding energy efficiency strategies based on existing worldwide implemented methodologies, focused on measuring fuel consumption by metrology principles and capacities for Colombia.
2. Methodology
Fig. 1 shows the procedure conducted to develop a methodology for measuring fuel consumption for LDV in Colombia. This procedure was set in four stages: Review of the local transport legislation,
Review of the fuel consumption measuring techniques available and the metrological capabilities of the country,
Review, select, and attach the methodology for its implementation in Colombia,
Conduct the uncertainty analysis of the measurement process,
Validation of the methodology.
2.1. Policies for transportation in Colombia
Currently, policies that dictate road transportation in Colombia, as well as other countries in South America, are only focused on ensuring the security and well-functioning of vehicles. Local policies only demand periodic checks on mechanical components and contaminant and greenhouse gas emissions tests under protocols based on static regimes, based on a specified engine velocity. However, these tests do not represent the real operative vehicle conditions on road, due to variable conditions that are not considered such as speed, power, traffic density, traffic signals, and the heterogeneous traffic which is highly complex and nonlinear in nature. So, a higher and significant contribution amount of atmospheric pollution may not be considered.
According to Jaikumar R, et al. [19] operating conditions of vehicles strongly influence exhaust emissions and increase fuel consumption. On the other hand, reduction of energy consumption by the implementation of energy efficiency strategies, being the main objective of energy management programs [20], may diminish overall fuel consumption and greenhouse gas emissions for road transportation.
The unsteady state operation and the optimal control of variables associated with the energy efficiency may be satisfied by fuel consumption measurements for LDV tested under dynamical conditions. Currently, there are three main methods applied worldwide to conduct measuring of fuel consumption on chassis dynamometers: gravimetric, volumetric, and carbon balance, the last one being the most studied and used [21].
The selection of one of these techniques depends on the metrological capacities of the country, the capacity for calibration of the equipment, and the availability of reference patterns for measurement within the country.
2.2. Selection of the measurement technique
In Colombia, there are some organizations that makes the calibration of the measurement instruments. Those that measure mass and volume are the most “advanced” or used in Colombia. That is why we say “capacities” (the aptitude of the organizations in the country related to the calibration of those instruments).
Advanced measurement instruments in Colombia have the capacities to measure mass and volume at the required testing resolution. On the other hand, there is no availability of the equipment needed to conduct measurements by carbon balance techniques. Because reference gases required for conducting calibration procedures are not produced within the country, they would have to be imported, increasing the cost of the implementation. For these reasons, fuel consumption measuring procedures by carbon balance techniques are not feasible.
On the other hand, the dependence of volume measurements to the variability of the ambient conditions is one of the main problems associated with the implementation of volumetric techniques. The error margin due to the variability of ambient conditions might have wider effects over the uncertainty of measurement. In addition, this technique requires changes in the fuel level measurement system [21], rendering it unfeasible. Therefore, among the fuel consumption measurement methods that were identified and evaluated, the gravimetric method was chosen to be implemented as the methodology for measuring fuel consumption. Measurements conducted by this method do not have high dependency on the variability of ambient conditions in the testing zone, and essential modifications on the systems of the vehicle are not required.
The implementation of this technique requires an external fuel tank, which is connected to the feeding fuel line of the vehicle by a fast coupling, as showed in Fig. 2. This tank is placed on the scale, which measures the mass of fuel before and after the initialization of the driving cycle simulation, so the mass consumed during the test is calculated by the difference registered.
2.3. Setting of the test procedure
Today, there are two procedures for dynamic testing in a laboratory to determine fuel consumption for LDV, the American and the European. The main difference between them is that they are not based on the same driving cycle simulation. The selection of one of these procedures for its implementation in Colombia was done according to the tendencies in the current automotive market of the country. Table 1 and Fig. 3 show the origin of LDV commercialized within the country in 2015.
According to Table 1 and Fig. 3, approximately half of the vehicles assembled in Colombia are manufactured in North America, and the main proportion of imported vehicles comes from countries like Mexico and Korea, whose official testing protocol for greenhouse gas emissions and fuel consumption is based on the Code of Federal Regulation (CFR) 40 which has established the FTP-75 Driving Cycle Simulation as its official protocol for testing on chassis dynamometer. Vehicles commercialized within Colombia have been tested under the protocol mentioned. For these reasons, The Code of Federal Regulation (CFR) 40 was adopted as the method for estimating fuel consumption for LDV in Colombia, considering gravimetric measuring techniques and not carbon balance techniques because of the identified metrological limitations of the country. Additionally, it was established that the implementation of this methodology should be conducted with the Cold Start Up, Stable and Hot Start Up stages of the FTP-75 Driving Cycle Simulation as shown in Fig. 4.
2.4. Setting of the Test Procedure
Fuel consumption can be determined by Eq. (1), which is a common reported value for establishing and evaluating the effectivity of energy efficiency strategies applied to Light Duty Vehicles:
During the process of adjusting the methodology for measuring fuel consumption in the laboratory, variables that had a direct effect over the measurand were identified because when measured independently, the measurement has an error, and uncertainty, that accumulates and impacts the final result. This uncertainty depends on the resolution and calibration of the instruments as well as how accurate the measuring process was executed. Therefore, the basic equation, Eq. (1) was modified in order to include the influence of all these variables over C.
Because the chosen methodology is based on gravimetric methods, the direct measuring of volume was not involved in the procedure. Therefore, the volume of fuel consumed was calculated by measuring the density of the fuel ρ f and the mass consumed m before and after the FTP-75 Driving Cycle Simulation. Distance was calculated by the product of the net number of revolutions of the rolls N during the cycle and their diameter D. Therefore, fuel consumption C in km/l could be determined by Eq. (2).
However, this equation was adjusted in order to include the effect of thermal dilatation of the material of the rolls, which might alter the calculation of distance, and the buoyancy effect of the air over the external fuel tank placed above the scale, which can alter the measuring of the mass consumed. These effects were included in Eq. (2), by considering the thermal dilation coefficient of steel, the density of the external fuel tank, and the density of calibration weights of the scale. In addition, the effect of the ambient temperature, barometric pressure, and humidity over the air density were considered by the state equation, Eq. (3). So, fuel consumption C could be determined by the following adjusted Eq. (3).
These variables and parameters are found in Table 2, which shows the resolution of the instruments used. Finally, including the expanded uncertainty U(C) associated with the overall measuring process, fuel consumption could be determined by Eq. (4).
The ambient air density may be calculated by Eq. (5). [24]. This equation allows to adjust the air density value more precisely as a thermodynamic property, as it includes the effect of humidity over density.
Each of the terms in the equation, were obtained based on the recommendations established by Picard et. al. [24]. On the other hand, the expanded uncertainty U(C) could be calculated by Eq. (6), where k is known as the coverage factor. In this study, the value for k was chosen as 2, giving a 95% level of reliability [16].
u(C), is the combined uncertainty which may be calculated as the square root of variance as showed in the Eq. (7), while variance was obtained by Eq. (8).
Terms ∂C/∂x i , represent the sensitivity coefficients of each variable measured. These coefficients were calculated as shown in Table 3, where c ρ is showed in Eq. (9).
Additionally, the uncertainty related to the measurement of each variable, u(y i ), was calculated by Eq. (10), and the uncertainty associated with the calibration of measurement instruments, u(y i ) cal was calculated by Eq. (11).
3. Results and discussion
The experiment was conducted by selecting the vehicle to be tested. So, the automotive market of LDV in Colombia was analyzed. and the most representative vehicle was chosen. According to the National Entrepreneurs Association of Colombia - Asociación Nacional de Empresarios de Colombia - ANDI [25], 53% of the new vehicles registered in Colombia were LDV, and 24,3% of them were Chevrolet in November of 2016. Besides, 89 % of the vehicles registered within the country had an engine smaller than 1800 cm3 [26]. For this reason, the Chevrolet Aveo, with an engine of 1600 cm3, was chosen as the representative vehicle of the Automotive Colombian Market for conducting the test of measuring fuel consumption.
Tables 4, 5, 6 and 7 show the results of the fuel consumption measuring process done by simulations of the FTP-75 driving cycle on a Chevrolet Aveo with a 1600 cm3 engine.
3.1. Setting of the test procedure
First, a normality test was conducted in order to determine the normal distribution data for each test. These are the hypothesis that were evaluated:
Null hypothesis: Data {C1, C2, …, Cn}, is a random sample of size n of normal distribution.
Alternative hypothesis: Data {C1, C2, …, Cn} is a random sample of size n of non-normal distribution. It means that it belongs to another probability distribution.
In Figs. 5, 6, 7 and 8, the probability distributions for data are shown. These results came from the normality test
Anderson - Darling conducted by MiniTab. P-values p i : p 1 =0,440, p 2 =0,512, p 3 =0,148, and p 4 =0,449 were obtained for each test. Due to p i >z, there was no evidence to reject the null hypothesis (z=0,05, a level of confidence of 95% for the normality test), so it was concluded that the data was normally distributed for each test.
Table 8 and Table 9 show the results obtained from the ANOVA done for data obtained from the measurements conducted during the fuel consumption experimental tests. Table 9 illustrates the results of the analysis of the data shown in Table 8 and proves there is not enough evidence to reject the null hypothesis, due to p>0,05 and F<F crit . Therefore, the alternative hypothesis was rejected, and the means of the tests were considered comparatively equal according to the level of confidence established, z = 0,05.
Fig. 9 shows that the line zero of the coordinate plane was contained within the intervals defined by the comparison of means of each test, according to the level of significance established. Therefore, it was concluded that a means of each test that represent the measurements of fuel consumption conducted in the laboratory, were significantly equal.
Fig. 10 represents the probability distribution of the residuals of each test. It was concluded that these were normally distributed because they followed the tendency established by the line on the graph, meaning, a low dispersion of data was found.
Fig. 11 shows that dots that represent the residuals appeared randomly distributed on the graph. Apparently, a recognizable pattern linking dots and the graph that did not contain atypical dots could not be identified. Because no recognizable pattern was detected on this graph, it could be concluded that there was homogeneity among variances as well as the independence of their residuals.
3.2. Uncertainty
Table 10 shows the uncertainty for calibration and resolution of measurement instruments used during tests.The results showed that the uncertainty for replicability was 0,08032 km/l. Thus, the combined uncertainty u(C) was estimated as 0,08039 km/l. Choosing a coverage factor k=2, the expanded uncertainty U(C) of the measuring process was equal to 0,1608 km/l. From the data, the mean was 10,8840 [km/l] and the standard deviation was 0,3213 [km/l].
Table 10 Uncertainty for calibration and resolution of the measurement instruments.
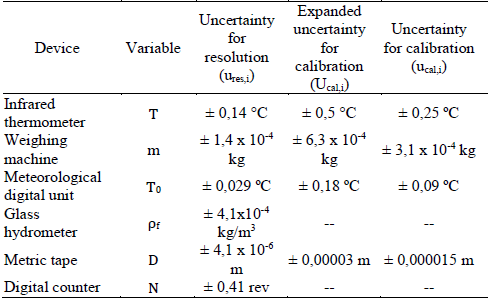
Source: The Authors.
Considering there’s a 95% reliability level for the evaluation of data, and calculating the uncertainty values for calibration, resolution and replicability, as well as the sensitivity coefficients for each test, the real fuel consumption for the tested vehicle, a Chevrolet Aveo 2010 with an engine of 1600 cm3, which was selected as the representative LDV in Colombia, was within the range:
4. Conclusions
A new methodology was elaborated based on existing and worldwide implemented methodologies, focused on measuring fuel consumption by metrological principles and capacities for Colombia as one of the first developments regarding energy efficiency strategies for this country.
A methodology for measuring fuel consumption of LDV in Colombia was developed based on the CFR 40 of the United States of America. The methodology was adjusted for its implementation using a gravimetric measuring method, in order to adapt it to the metrological capacities of Colombia.
Energy efficiency regulations on transportation in Colombia should begin by establishing the fuel consumption baseline of the national automotive fleet. For that reason, the importance to normalize and standardize the procedure of fuel consumption measurement for LDV was identified as well as the need to establish regulations benefiting the most efficient vehicles and penalizing the worst ones. In this way, it would be possible to achieve a constant reduction of fuel consumption of LDV and greenhouse gas emissions considering the automotive fleet for Colombia.
Metrology may be considered an important tool for the evaluation of the energy efficiency strategies, as it provides not only the mechanisms to determine the effectiveness and performance of these strategies, but also the way to identify the main causes of inefficiencies and losses. This would provide the country with a benchmark to rearrange its objectives in order to maintain constant improvement actions. Additionally, metrology allows the verification of reliable measurements and the validation of the regulations and policies.
The expanded uncertainty associated with the measurement of each of the variables involved in the measuring test was analyzed, and it was proven that air density had no effect on the fuel consumption measuring process. This means that the contribution of the correction factor c p , due to the buoyant effect of the air over the external fuel tank was meaningless, as its contribution was lower than 0,02%.
The expanded uncertainty obtained from the measuring process was 0,1608 km/l. Therefore, the fuel consumption of a vehicle from a representative brand of the Colombian automotive vehicle market might be reported as 10,8840 ± 0,1608 km/l.
Because means and variances of each test conducted stayed within the level of reliability when the tests were repeated and performed by different drivers, the study concluded that the methodology for the estimation of fuel consumption for light duty vehicles (LDV) in Colombia, as well as its analytic model, issubstantiated.