Evidence-based medicine and public health currently serve as the predominant paradigms in the provision of health services 1. Primary, secondary, and tertiary prevention interventions for specific diseases are largely based on clinical guidelines, while public health interventions to address health problems at a population level are largely based on proven successful programs 2. Both clinical guidelines and public health programs rely mostly on quantitative research that provides evidence of the efficacy, effectiveness, and efficiency of interventions, and indicate the strength of associations between risk factors and health outcomes. Contrary to the principles of evidence-based medicine, the quality of the evidence presented by health research is usually assessed exclusively according to the type of study design 3. This widely used approach assumes that the statistical methods related to a specific study design are unbiased and free of random error and misspecification.
Regression modeling is a statistical method commonly used in health research, and especially for observational studies. The selection of the type of regression model, the selection and inclusion of model variables, and the assessment of model diagnostics are some of the key actions that should be specified and properly conducted in order to obtain valid statistical results 4. The absence or misuse of an appropriate regression modeling technique could lead to erroneous results and conclusions, and while regression modeling plays a central role in quantitative health research, little attention has been given to the appropriateness of the statistical methods used by epidemiological studies to reach results and conclusions 5. In Colombia, the national bibliographic index Publindex has ranked Biomédica as a top journal in the health sciences field 6, and, therefore, a large amount of original health research performed in the country and the region has been published in this journal.
The objectives of this paper were to 1) determine the frequency with which regression modeling was reported in original biomedical and public health articles published in Biomédica between 2000 and 2017 (including online ahead of print); 2) describe the reporting of the parameters and procedures used for the statistical models, and 3) describe the quality of the information reported by the studies to explain and evaluate the statistical analyses. We also aimed at providing an overview of the statistical quality of regression modeling reported in the health literature published in Biomédica and based on those reports, identify the strengths and limitations of the statistical methods.
Materials and methods
We reviewed all original articles published in Biomédica between 2000 and 2017 (including online ahead of print publications in the 2016 and 2017 issues), which are available at the official Biomédica’s website (www.revistabiomedica.org). We then selected the articles that reported the use of at least one statistical regression model, according to the following selection criteria: 1) original articles that used one or more regression analyses; 2) regression analyses that were conducted in order to address the study’s main objective, and 3) regression analyses that were presented in the methods and results sections. When an original study included two or more regression analyses, we selected the regression model that was related to the study’s main objective. When a study had two or more main objectives, we selected one model per objective. Therefore, we included more than one regression model per original article when they corresponded to different main objectives and specifications.
We conducted a literature review to identify papers that assessed the quality of statistical reporting in biomedical journals and found four publications that presented good practice guidelines for the presentation of statistical methods 5,7-9. The first publication appeared in 1992 when the International Committee of Medical Journal Editors (ICMJE) issued a set of publication requirements for biomedical journals. Then, in order to better address the needs of readers the ICMJE proposed the first standards for the presentation of statistical information, aimed at supporting and informing authors and editors about the statistical principles behind the studies published in biomedical journals 7. The second article was published in 2013 and its purpose was to develop a checklist to determine the frequency of the use of regression models in economics, and the parameters and the amount of information reported. That checklist grouped items according to four consecutive stages of the statistical process 8. Later, the proposal titled “Strategies for the Development of Statistical Regression Models” described important points to consider when using a regression model, such as data manipulation, modeling strategies, final model evaluation, and presentation 9. Lastly, Lang and Altman recently published a general guideline for reporting methods and statistical analyses in biomedical journals, which included 12 items specific to regression analyses 5.
Some of the important items in one or two of the checklists mentioned were missing from the others, and none of the lists was comprehensive enough to assess the quality of the presentations of the statistical regression models. Therefore, we consolidated all of the items in the four publications relevant for the evaluation of regression modeling into one checklist containing 20 statistical procedures or parameters. These were evaluated based on the four different stages proposed by Kearns, et al.8: I) pre-statistical modeling considerations; II) specification of the final model; III) presentation of the final model; and IV) validation of the final model.
In general, the guidelines on good practices for statistical reporting 5,7,8, and the checklist based on these guidelines used for this critical assessment, can be summarized by the following standards: 1) agreement of the statistical method with the measurement scale and data structure of the study variables; 2) coherence between the statistical method and the study objective; 3) verification of the statistical model’s assumptions and understanding of the theoretical basis of the statistical tests used; 4) assessment of the diagnostics and goodness of fit of the final model according to the regression method used; 5) evaluation of the final model and comparing it to alternative models, and 6) discussion of the strengths and limitations of the final model.
The checklist used for this work was reviewed by two experts in statistical analyses, and then adapted based on their recommendations. Appendix 1 presents the full checklist and Appendix 2 compares the four checklists with the items included in the one used for this review. It is important to mention that we did not evaluate or validate the regression models themselves but only the reporting, according to each stage of the process.
Two independent reviewers with statistical and bio-medical experience performed a critical assessment of the 20 items included in the checklist. The reviewers were blinded to the authorship of the original articles. In addition, a third reviewer was assigned to assess three articles that were written by the other reviewers.
Most of the checklist items were dichotomized as “yes/no” responses and accompanying observations and explanations about the selections were included. A third reviewer resolved disagreements. We used the Cohen’s kappa statistic to assess agreement among the independent reviewers’ responses and we calculated a pooled (inter-rater) kappa for multiple items since the checklist contained several items. We present a descriptive analysis of the results using frequencies and grouped by six-year periods. We conducted the statistical analyses using Stata 12™ (Stata Corporation, College Station, TX, USA).
Results
We reviewed 755 original articles published in Biomédica during the study period. Regression analyses were used in 163 articles (21.6%) and after exclusions, 108 original studies and 113 regression models were included in the review (figure 1). Appendix 3 presents a full list of the articles included. The review process had an inter-rater agreement of 0.94.
Most of the studies were related to public health sciences (65.7%) and the remaining ones to biomedical sciences. In terms of study design, cross-sectional studies were the most frequent (38.9%), followed by cohort studies (23.2%). Table 1 presents the characteristics of the studies.
Table 1 Characteristics of the included original studies that used regression models by six-year periods, Biomédica, 2000-2017
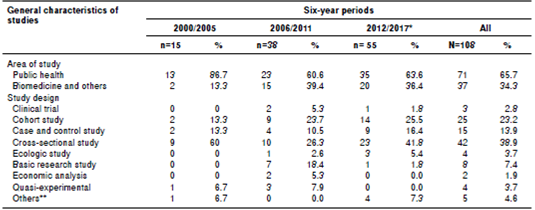
*Including online ahead-of-print publications for 2016 and 2017 issues
** Analysis of secondary and/or administrative sources without a specified design (more than two sources or information systems)
With regard to stage I, “pre-statistical modeling considerations”, 37.2% of the models reported using a combination of conceptual frameworks and statistical criteria to select the variables to be included in the regression models. With regard to the models that used statistical procedures or a combination of conceptual frameworks and statistical criteria to select the variables (n=49), the stepwise strategy was the most frequently (50%) reported. The logistic regression model was the most frequent type of model (56.6%), followed by the linear regression model (25.7%). A small proportion of studies (8.9%) mentioned “missing data” in their reporting of data quality. Table 2 presents the reporting of the statistical information related to the regression models according to checklist stages, items, and six-year periods.
Table 2 Information about regression models as reported by the original studies by six-year periods, Biomédica, 2000-2017
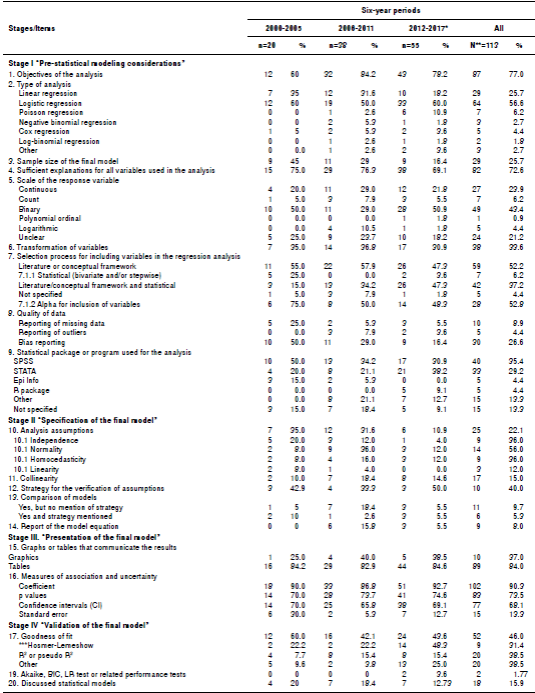
* Including online ahead-of-print publications for 2016 and 2017 issues; ** For this table, the unit of analysis is the regression model; *** This item only applies to studies that used logistic regression: periods 2000-2005 (n=7), 2006-2011 (n=8), 2012-2017(n=14), and n=29 for the total of periods.
With respect to stage II, “specification of the final model”, 22.1% of the models reported the verification of the assumptions of the model with normality and linearity being the most and least frequent assumptions verified (65.0% and 12.0%, respectively). Model comparisons (9.7%) and model equations (8.0%) were the least frequent items reported by the studies.
With regard to stage III, “presentation of the final model”, 90.3% of the studies reported regression coefficients and the uncertainty measure reported with the highest frequency was the p value (73.5%), which increased over the six-year periods.
For stage IV, “validation of the final model”, 46% of the studies reported the goodness of fit of the model and 1.7% reported on tests for model performance. Regression models were discussed only in 15.9% of the articles.
In terms of the frequency of reporting by six-year periods, a decreasing pattern was found in the reporting of the following checklist items: Sufficient explanations for all variables used in the analysis; alpha parameter for variable inclusion, missing data; bias assessment for data quality; verification of the model’s assumptions, and goodness of fit measures. The discussion on the statistical models presented in the studies also decreased over time. In contrast, there was an increase in reporting on the combined use of conceptual frameworks and statistical criteria for the inclusion of variables in models of association.
Discussion
We conducted a critical assessment review of original studies that used statistical regression models and were published in Biomédica between2000 and 2017. The results show significant weak-nesses in the reporting of the statistical information or parameters needed by reviewers and readers in order to correctly assess and interpret statistical models. While the number of studies that use regression models has increased since 2000, the descriptive analysis which we performed by six-year periods showed that rather than having improved, the reporting of statistical information has worsened over time for a large number of key items in the checklist.
According to Publindex, Biomédica is currently the journal that has the highest impact on biomedicine, public health, and epidemiology in Colombia 6. And while Colombian research groups have published an increasing number of studies in international journals, Biomédica continues to be the preferred journal for publishing the results of research projects with national and regional impact, and its visibility has increased over recent decades 10.Therefore, Biomédica provides an appropriate framework to analyze the quality of statistical reporting in Colombia.
Previous reports have found weaknesses in the training in statistical competencies offered by postgraduate epidemiological and public health programs in Colombia 11. To some extent, the weakness in statistical skills at the postgraduate level might be related to the misuse and simplification of statistical methods, including generalized linear regression methods 12. This review used the available guidelines on good practice for statistical reporting to describe reports of regression methods that have appeared in Biomédica over the last 17 years since these are the most common statistical methods used in observational studies. The results of this review show that most of the included studies did not meet the standards of the guidelines and the checklist used herein to assess the quality of the reporting of statistical regression modeling methods.
Good practice guidelines for statistical reporting have been established mainly by expert consensus in order to provide guidance on how to improve the quality of reporting on statistical methods 5. However, these guidelines should not be assumed as a simplification of statistical methods or used as “recipes” to conduct statistical analyses. Strictly following the guidelines does not guarantee high quality regression analyses. Assessments of the quality of statistical analyses should be comprehensive, based on statistical rationale, and meet the statistical standards discussed above.
Most of the original articles included in this review are observational studies which used regression models to control for confounders 13. The results of the analysis of “pre-statistical model considerations”, or stage I, showed an increase over time in the use of combined methods to select regression model variables for studies of association. Nevertheless, statistical criteria based exclusively on hypothesis testing methods continue to be used. That type of statistical criteria, as well as stepwise methods, have been strongly criticized in recent decades 4,14 and modern epidemiology widely recognizes that model variables should be selected according to a more comprehensive analytical process, based on theoretical and literature reviews and using multidimensional criteria 15.
In statistics, a “misspecification error” refers to the use of incorrect procedures to build a regression model 16. The misspecification of a regression model could have an impact on the estimators obtained, thereby causing bias. The importance of this type of error has been well acknowledged 17,18 and may bias the study results. It includes omitting influential or including non-influential explanatory variables, using incorrect functional forms, violating the assumptions of the model, and using incorrect approaches to choose the final model 19. In this regard, it is important to note that most of the articles reviewed herein were weak in their reporting of the verification of model assumptions, which are key criteria for the statistical validity of the models.
Critical assessment reviews are significantly limited in their ability to distinguish between the absence of analysis versus a lack of reporting since quality is judged exclusively based on published documents. However, even when statistical methods are used, not reporting on them in itself weakens an original article in terms of demonstrating the validity of its methods and results. In the case of Biomédica, there are no constraints on the complete reporting of statistical methods since there is no word limit for original articles.
One important limitation of the present review is the use of the new checklist. Although two experts in statistical analyses reviewed it, it has not been widely reviewed by the scientific statistics community and, therefore, it cannot be considered a validated instrument. In addition, since the check-list assigned equal weight to all items, it does not take into account that some of the elements used to judge the quality of reporting may be more important than others. On the other hand, its strength is that it is a comprehensive list based on previous guidelines and checklists that have been specifically used to assess the quality of reporting on statistical regression modeling analyses.
In conclusion, this critical assessment review of original articles published over the last 17 years in a top biomedical journal in Colombia shows that there are important limitations in the quality of reporting on statistical regression models. An improvement over time in the use of multidimensional statistical procedures for the selection of model variables was evident. However, there was a lack of reporting on important statistical information related to the four stages of analysis, thereby creating uncertainty about the validity of the statistical models, which in turn calls into question study results and conclusions.
Finally, we invite researchers, reviewers, and editors of biomedical journals in Colombia to use the results of this critical assessment review to develop, promote, and control an appropriate culture for statistical analysis and reporting. Attaining this goal through the use of good practice guidelines for statistical reporting will lead to a more rational and efficient use of statistical methods, higher confidence and transparency in the peer-review process, and overall, higher quality biomedical, public health, and epidemiological studies in Colombia.