CONFIRMING THE groupings and associations between variables that make up an assessment measure is one of the typical indicators of validity. This verification is referred to as validity based on the internal structure for a scale (AERA, APA, & NCME, 2014). Typically, exploratory and confirmatory models, models with first and higher-order factors, as well as bifactor models, are used to verify the internal structure with better fit indices for a scale. In some cases, even showing better fit than IRT models (Paiva, Cavalcanti, & Lima, 2020). Our focus in this study was to investigate the internal structure of a self-report scale for pathological traits assessment, the Dimensional Clinical Personality Inventory 2 (IDCP-2; Carvalho & Primi, in press). The internal structure of IDCP-2 was only partially verified (e.g., Carvalho, 2018; Carvalho & Arruda, 2016; Carvalho & Sette, 2017), as previous studies did not use all factors of the scale in the same analysis. Therefore, to this date there is no information available on the internal structure of IDCP-2 covering all its factors. We investigated the internal structure based on confirmatory and exploratory models, including bifactor models.
Theoretical Background
The internal structure of an assessment tool indicates the level of association between the variables that compose the test (e.g., items), how these variables are grouped, and to what extent a latent construct explains them. The observed associations between items indicate the common variance, i.e., how much they measure similar constructs (AERA et al., 2014; Rios & Wells, 2014; Thompson, 2007).
Indicators of the internal structure are commonly investigated in psychological tests to explore or confirm the structure formed by variables composing the scale, and whether this structure is consistent with the expected structure (AERA et al., 2014; Thompson, 2007). Besides the level of association between the variables, another indicator of the proper functioning of the internal structure is the interpretability of the group of variables observed through data analysis. For instance, the pathological trait narcissism is multidimensional, composed of grandiose tendency (i.e., grandiose narcissism) and vulnerable tendency (i.e., vulnerable narcissism) (Stoeber, Sherry, & Nealis, 2015). From this perspective, it is expected that studies investigating the structure of scales assessing narcissism should find factorial solutions composed of two factors reflecting the two dimensions of the construct.
The analytical strategy typically used to verify the internal structure is the factor analysis. There are two major classes of factor analysis, exploratory (EFA) and confirmatory (CFA; Yong & Pearce, 2013). In EFA, the items are grouped without restrictions, and cross-loadings are allowed. CFA models are more restrictive, cross-loadings are not allowed, and factors are previously defined based on empirical studies and theory (Brown, 2014; Thompson, 2007). Both EFA and CFA enable to verify different factor structures (e.g., unidimensional, bifactor; Rios & Wells, 2014).
In the context of internal structure verification, the bifactor factor structure has been tested as a suitable model for dimensional constructs, as pathological traits composing personality disorders (PD). This structure has also been tested in self-report scales (Gouveia et al., 2018). This structure represents an approach of data modeling that reconciles multidimensionality and the existence of a general factor. In bifactor models, each item is free to simultaneously load on a general (and orthogonal) factor and one or more specific factors (see Figure 1 for a graphical representation). The high comorbidity among PD (e.g., Clark, 2007; Widiger & Trull, 2007) can indicate a common latent construct, supporting the use of a bifactor structure to investigate the internal structure of scales to measure pathological traits. The bifactor can also be interpreted as a general factor composed by the social desirability variance of items or a predominant response style present in the sample (Reise, 2012; Reise, Morizot, & Hays, 2007; Sharp et al., 2015). Contrasting with a hierarchical factor model, the bifactor provides the direct loading estimates of each item on the general factor. This information is useful because it affords the identification of the most discriminative items, which can guide item selection to compose an abbreviated version of the scale. Furthermore, advantages of the bifactor structure include whether subscales should be maintained or excluded after controlling for a general factor, investigating construct dimensionality (Reise et al., 2007), and assessing the predictability of the overall score and speciic scores according to criterion variables (Canivez, 2016).
Although the investigation of bifactor structures has grown in the last ten years (e.g., Preti et al., 2015; Tackett, Daoud, De Bolle, & Burt, 2013), for most of the assessment tools of pathological traits, this model remains unverified. An example of this is the Dimensional Clinical Personality Inventory, currently in its second version (IDCP-2; Carvalho & Primi, in press; Carvalho, 2019). It is a self-report scale composed of 206 items to measure pathological traits from a dimensional perspective. Validity based on internal structure and relationship to external criteria has been observed in previous studies (e.g., Carvalho & Costa, 2018; Carvalho, Sette, & Miguel, 2018; Carvalho, Zuanazzi, & Miguel, 2019). Previous literature provides only fragments of the internal structure of IDCP-2 (e.g., Carvalho, 2018; Carvalho & Arruda, 2016; Carvalho & Sette, 2017), indicating a structure of 47 factors grouped in 12 dimensions.
A more recent study (Pianowski, Carvalho, & Miguel, 2019) has verified the suitableness of IDCP-2 factors according to the Hierarchical Taxonomy of Psychopathology (HITOP; Kotov et al., 2017), which represents a current dimensional proposal for mental disorder taxonomy, including PD. The HITOP constructs psychopathological disorders and their components based on the observed covariation of symptoms and traits. In this evidence-based model, symptoms are grouped in disorders and co-occurring disorders into spectra. Each spectrum is larger constellations of disorders (e.g., the internalizing spectrum is composed of disorders from fear, distress, eating pathology, and sexual problems subfactors). Almost all spectra are composed of PD, internalizing, thought disorder, disinhibited externalizing, antagonistic externalizing, and detachment, expecting the somatoform spectrum. Although the obsessive-compulsive PD is not present in the HITOP model, the literature supports a sixth spectrum representing this disorder (Forbes et al., 2017), called the compulsivity factor. In this study, we were guided by both previous studies indicating the structure of IDCP-2 and the five HITOP spectra with the addition of the compulsivity factor presenting the obsessive-compulsive PD. We could not find studies investigating the IDCP-2 internal structure using all its factors, as well as studies verifying bifactor structures for this measure.
Our aim in this study was to verify the internal structure of IDCP-2, including its 47 factors. As confirmatory models, we tested the original 12 factors structure (Carvalho & Primi, 2015; Carvalho & Primi, in press), and an alternative structure based on the HITOP five spectra and a compulsivity factor. Besides, we conducted an exploratory investigation also testing the original and HITOP structures, but without restrictions on the item loadings. The veriications included testing for bifactor solutions. We expected that the confirmatory models (original and HITOP structures), should present good fit indices, especially the bifactor models, considering the multifactorial perspective of PD (Clark, 2007; Reise, 2012; Widiger & Trull, 2007) and previous evidence of the suitableness of this structure to personality (Sharp et al., 2015; Preti et al., 2015). Exploratory models were tested for cases where the confirmatory models did not present a good fit.
Methods
Participants
Participants included 2,000 subjects from the community, aged between 18 and 70 years (M=28.5; SD=9.6), 42.2% females, and 14.7% did not inform the sex. Most of the participants were college students (37%). Regarding their history of psychiatric/psychological treatment, 19.7% of the subjects reported undergoing or having already experienced psychiatric treatment, and 38.7% reported undergoing or having undergone psychotherapeutic counseling.
Measures
Dimensional Clinical Personality Inventory 2 (mcp-2). The IDCP-2 is a self-report scale to measure pathological traits developed in Brazil. It's an updated version of the IDCP (Carvalho & Primi, 2015), based on several PD models (e.g., Millon, 2011; APA, 2000, 2013; Krueger, Derringer, Markon, Watson, & Skodol, 2012; Shedler & Westen, 2004; Clark, 1990). The IDCP-2 is composed of 206 items distributed in 12 dimensions, with a 4-point Likert-type response scale (1 = "has nothing to do with me" and 4 = "has a lot to do with me"). Previous studies indicated the validity of IDCP-2 factors (e.g., Carvalho, Pianowski & Miguel, 2015; Carvalho, Pianowski, Silveira, Bacciotti & Vieira, 2016; Carvalho, Sette & Ferrari, 2016).
Procedure
We conduct this study after the approval of the Brazilian Research Ethics Committee (CAAE: 21992113.1000.5514), and the participants signed a consent form. Online data collection was carried out using the Google Forms platform, inviting volunteers through e-mails and social networking sites (e.g., WhatsApp, Facebook). The protocol comprised a form to attest the voluntary participation, followed by a demographic data questionnaire and the instrument.
Data Analysis
Confirmatory factor analyzes (CFA) were conducted to verify the fit of the data to the model representing the original 12-factors structure of IDCP-2 and the HITOP six-factors structure. Bifactor models were tested as well. Factorial loadings above .30 were considered as substantial. Four models were tested: (model C1) 12 factors; (model C2) bifactor and 12 factors; (model C3) six factors; and (model C4) bifactor with six factors. Chi-square and degrees of freedom ratios (x2 / DF <2), Confirmatory Fit Index (CFI;> .90), Tucker-Lewis Index (TLI), and Root Mean Square Error of Approximation (RMSEA; <.05) were used as parameters to verify model fit (Hu & Bentler, 1999). As the indexes indicated just poor it to the models, exploratory factor analyzes (EFA) were performed, less restrictive models with items free to be grouped without prior determination, allowing cross-loadings. The following models were tested: (model E1) six factors; (model E2) bifactor with six factors; (model E3) 12 factors; and (model E4) bifactor with 12 factors. We used Cronbach's Alpha and Omega to analyze the internal consistency of factors. We performed the statistical analysis with the Mplus version 7.11 software.
Results
We conducted CFA and EFA. Table 1 presents fit indexes of four tested models, exploratory and conirmatory.
Table 1 Fit Indexes for Confirmatory and Exploratory Models.
Model | Structure | x2 /df | CFI | TLI | RMSEA |
---|---|---|---|---|---|
Model C1 | 12 factors | 32361.871/1024 | .472 | 443 | .124 |
Model C2 | Bifactor and 12 specific factors | 12210.306/ 921 | .786 | .748 | .078 |
Model C3 | 6 factors | 19997.192/986 | .680 | .660 | .097 |
Model C4 | Bifactor and 6 specific factors | 17330.926/ 986 | .725 | .698 | .090 |
Model E1 | Bifactor and 6 specific factors | 6399.979/ 773 | .905 | .867 | .060 |
Model E2 | 6 factors | 6399.979/ 773 | .905 | .85 | .060 |
Model E3 | Bifactor and 12 specific factors | 2598.288/ 548 | .965 | .932 | .043 |
Model E4 | 12 factors | 3030.778/ 583 | .959 | .924 | .046 |
Note: In bold fit index showing good adjustment; C: confirmatory model; E: exploratory model.
Although any of the conirmatory models presented good it indexes, the bifactor model with 12 speciic factors was the one to show the best it. According to that, we tested exploratory models and the best it model was the bifactor with 12 specific factors. The loadings and reliability of this model are presented in Table 2.
Table 2 Factorial Loadings of the Exploratory Bifactor Model With 12 Specific Factors
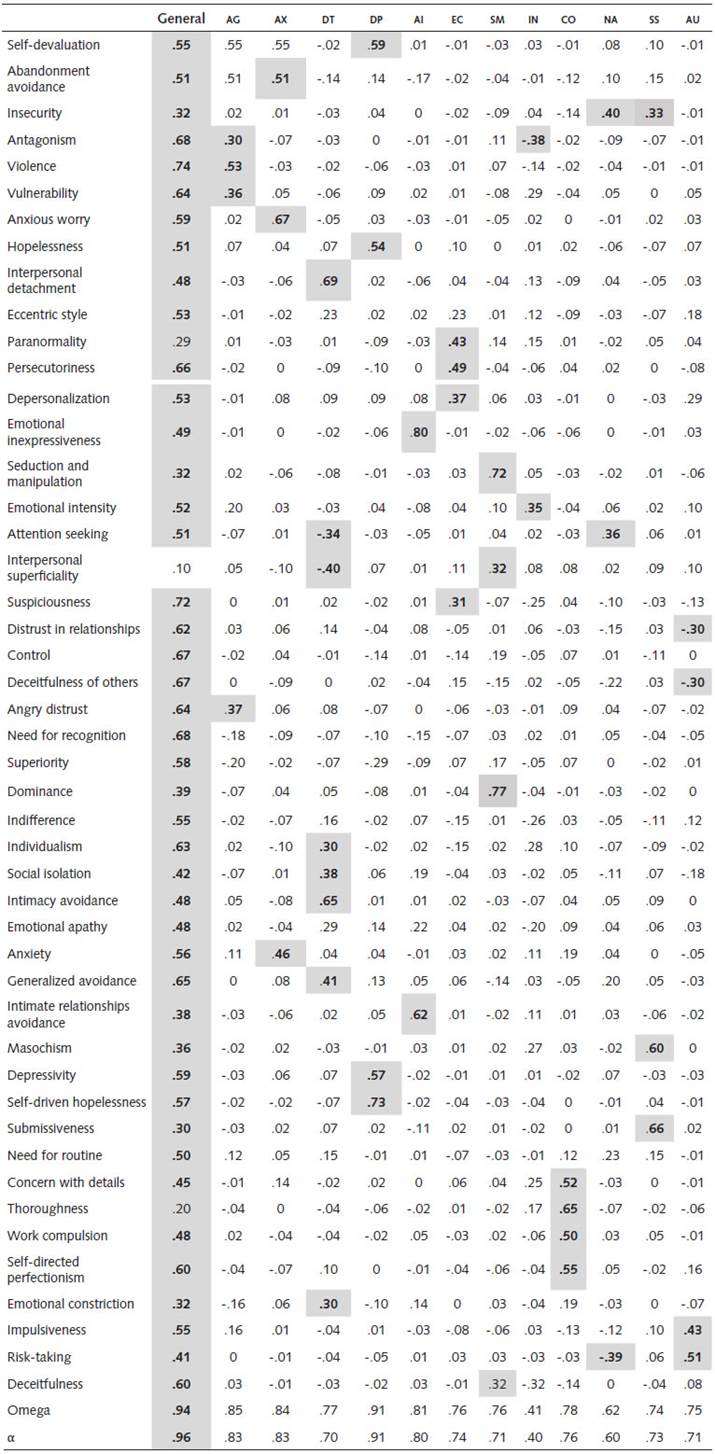
Note: Loadings > .30 are in bold and grey; AC = Aggressiveness; ax = Anxiety; DT = Detachment; DP = Depression; AI = Avoidance of Intimacy; EG = Eccentricity; sm = Seduction and Manipulation; IN = Interpersonal Need; GO = Conscientiousness; NA = Need for Attention; ss = Self-sacrifice; AU = Audacity.
Excepting Thoroughness, measures presented loadings in the general factor. We found 12 factors, namely, Aggressiveness, Anxiety, Detachment, Depression, Avoidance of Intimacy, Eccentricity, Seduction and Manipulation, Interpersonal Need, Conscientiousness, Need for Attention, Self-Sacriice, and Audacity. We sought to avoid cross-loading. However, three measures loaded on two factors: Attention Seeking, Insecurity, and Interpersonal Superficiality. The decision was to maintain these measures in two factors due to their interpretability. Internal consistency was assessed by alpha ranging from .40 to .91 for specific factors, and alpha equal to .96 for the general factor. Omega coefficient ranged from .41 to .91 for specific factors and was equal to .94 for the general factor.
Discussion
Our aim in this study was to investigate the internal structure of IDCP-2, using the 47 factors composing the scale. We tested for diferent structures based on the instrument's previous literature (e.g., Carvalho, 2018; Carvalho & Arruda, 2016; Carvalho & Primi, 2015; Carvalho & Primi, in press; Carvalho & Sette, 2017), as well as the HITOP model (Kotov et al., 2017). Therefore, we used CFA and EFA models. Among the tested models, the one presenting the best it to data and the best interpretability of the factors was the bifactor exploratory model with 12 specific factors.
CFA models composed by 12 factors (IDCP-2 original structure; Carvalho & Primi, 2015; Carvalho & Primi, in press) and by six factors (HITOP model with an extra compulsivity factor; Kotov et al., 2017; Forbes et al., 2017), did not present satisfactory it indices, indicating that the predeined factor structures were not adjusting to the data. These results can be explained by the features of CFA restricting cross-loadings and requiring prior setting of all parameters, making this kind of analysis less flexible (Thompson, 2007; Brown, 2014) in comparison to exploratory models. We could observe the existence of cross-loadings in some factors (e.g., Self-Devaluation and Depressivity) that may have occurred due to the similarity of the composing pathological traits. Previous ind-ings using IDCP-2 factors under the perspective of the HITOP observed cross-loadings (Pianowski, Carvalho, & Miguel, 2019).
The EFA models were tested with the same number of factors of the CFA models (i.e., ix factors and 12 factors solutions). However, the parameters were not fixed for EFA, and the items were freed to grouping based on their correlations (Ferguson & Cox, 1993; Yong & Pearce, 2013). The bifactor with 12 speciic factors model was the best regarding it indices and theoretical interpretability of factors (Carvalho, 2019). The literature demonstrates that the bifactor model is indicated for constructs that are admittedly multidimensional (Clark, 2007; Reise, 2012; Widiger & Trull, 2007). Furthermore, studies corroborate that the bifactor model is suitable for scales that measure pathological traits (Sharp et al., 2015; Preti et al., 2015), and the high comorbidity rate among PD indicates to a general factor (Sharp et al., 2015). The results found in our study corroborate the use of bifactor models for self-report tests that assess pathological traits, specifically the IDCP-2. Expressly, our findings indicated that (a) factors that compose IDCP-2 show a common ground, probably respecting a broad pathological functioning of personality; (b) the specific 12 factors of IDCP-2 remain significant even after controlling for this general factors. This last inding is clinically relevant, as indicates the usability of IDCP-2 scores for measuring pathological traits.
The 12 observed factors (i.e., Aggressiveness, Anxiety, Detachment, Depressivity, Avoidance of intimacy, Eccentricity, Seduction and manipulation, Interpersonal need, Conscientiousness, Need for attention, Self-sacrifice, and Audacity), agree with the core domains that characterize PD (e.g., APA, 2013; Kotov et al, 2017). Besides, these factors approximate the original dimensions of IDCP and IDCP-2 (Carvalho & Primi, 2015; in press; Carvalho, 2019). Although some factors do not precisely reflect IDCP-2'S original dimensions, as Anxiety and Audacity, they do identify coherent and consistent groups of variables, and are similar to some IDCP-2 dimensions (e.g., Criticism avoidance and Inconsequence). For instance, Anxiety is composed of Avoidance of Abandonment, Anxious Concern, and Anxiety. These factors share a common trait, the anxiety related to concerns about the future. The correspondence between the observed actors and IDCP-2 original 12 factors are presented in Table 3.
Table 3 Correspondence Among IDCP-2 Observed and Original Factors.
IDCP-2 observed factors | IDCP-2 original factors |
---|---|
Aggressiveness | Aggressiveness |
Anxiety | Mood instability* |
Detachment | Isolation |
Depressivity | Mood instability* |
Avoidance of intimacy | Criticism avoidance |
Eccentricity | Eccentricity; Distrust |
Seduction and manipulation | Grandiosity |
Interpersonal need | Dependency |
Conscientiousness | Conscientiousness |
Need for attention | Attention seeking |
Self-sacrifice | Self-sacrifice |
Audacity | Inconsequence |
Note: * The original Mood instability dimension is mainly composed of depressivity and anxiousness traits. Because of that, we understand that the better correspondence is to consider this dimension as representative of Anxiety and Depressivity factors.
The reliability estimates were generally high, indicating homogeneity among items composing the same factor (Tavakol & Dennick, 2011). An exception to it was the Need for attention factor, presenting low internal consistency, probably related to the restricted number of variables composing this factor. This issue should be investigated in future studies.
Our results indicated the bifactor with 12 speciic factors as the best model. Although the original structure of IDCP-2 is not based on a bifactor model, the specific factors reflect, in general, the original factors of the scale, as observed in Table 3. Future studies should investigate the meaning of the general factor. Moreover, future studies should investigate the clinical use of the new factors presently observed, especially those showing compositions dissimilar to the original dimensions (e.g., Anxiety).
The main limitations of this study should be highlighted. The sample of this study did not have people with PD diagnoses, this may have restricted the variability of the items. Furthermore, we observed the presence of a general factor, corroborating the literature. However, we could not establish the meaning of this factor. For instance, it could represent a pathological common ground between specific factors, social desirability, or response style bias. Future studies should be conducted to investigate this issue further.