GENERAL INTRODUCTION
Avocado (Persea americana Mill.) cv. Hass is one of the most profitable fruits traded in international markets (FAO, 2021; International Trade Centre, 2021). This fruit is produced in countries with tropical and subtropical climates (Schaffer et al., 2013), many of them with emergent economies, such as Colombia. This country is the tenth largest global exporter of Hass avocados (FAO, 2021), and its production has increased exponentially in recent years (Ramírez-Gil et al., 2018a). Unfortunately, such production has been conducted without technology, which has extended the agronomic knowledge gap (Ramírez-Gil et al., 2018a; Builes and Duque, 2020). Hass avocado in Colombia can be considered a rainfed crop because most farmers irrigate empirically once a week only when trees manifest water deficit signs or do not irrigate, but recently studies demonstrated that under tropical condition irrigation can be necessary in a few months a year (Erazo-Mesa et al., 2021). Therefore, Colombian Hass avocado farmers who adopt incipient or state of the art irrigation techniques can save water and mitigate the negative consequences of water scarcity and climate change on their agriculture production system in the future.
Water scarcity and climate change will modify the water use in agriculture in the forthcoming years (FAO, 2020), including some avocado-producing areas, where changes in temperature and precipitation are projected with impacts on water balances (Ramírez-Gil et al., 2019). In addition, climatic variability under tropical conditions presents many adverse effects on crops as avocado (Ramírez-Gil et al., 2020; Erazo-Mesa et al., 2021). Climate variability is associated with seasonal and non-seasonal phenomena such as the Intertropical Convergence Zone (ITCZ) (Byrne et al., 2018; Mamalakis and Foufoula-Georgiou, 2018), and ENSO (El Niño Southern Oscillation) phenomena, respectively (McPhaden et al., 2006; McCabe and Wolock, 2013). Both phenomena have a high impact on the availability of water for crops.
Aiming to identify the volume of water and the moment to irrigate the crop (Ali, 2010), irrigation scheduling (IS) is the first step to fill the water management gap in Hass avocado in Colombia. Although other methods have been used to schedule irrigation (Yohannes et al., 2019), the most common IS methods include: soil water balance (SWB), the use of soil or plant indicators, and simulation models (Garrido-Rubio et al., 2019 Gu et al., 2020). These demand a vast amount of historical or near real-time data and specialized knowledge to perform the irrigation scheduling (Fernández et al., 2020). The factors that contribute to the reduction of the IS accuracy include the high variability of the soil, terrain, and microclimates; the changing trees' physiology and reseeding requirements; the differential gene expression; the rootstock variability in the nursery stage; and heterogeneous agronomic tasks within plots (Ali, 2010).
Currently, the concept of digital agriculture (DA), part of the revolution 4.0 concept, is being explored emphasizing the use of different technologies in agriculture (Ramírez-Gil et al., 2021) such as information and communication systems, remote and proximal sensing, modeling, programming, robotics, cloud computing, the internet of things (IoT), big data analysis, among others (Kamilaris et al., 2017; El-Gayar and Ofori, 2020). The main objective of this new approach in agriculture is the management of information for a correct, fast, and accurate decision making. From the DA approach, technologies that suit the Hass avocado IS (Ćulibrk et al., 2014) can be classified into three categories: proximal sensing (PS), remote sensing (RS), and mobile or web applications. Studies have reported the benefits of using IS in avocado orchards (Holzapfel et al., 2017; Moreno-Ortega et al., 2019; Silber et al., 2019), highlighting a reduction in crop water consumption and yield, and fruit quality improvement.
In the following chapters, an exhaustive review will be made when aspects associated with the definition of DA, the use of remote and proximity sensors, new analysis technologies, and smart devices and web pages were used for the determination, programming, and management of irrigation on agricultural systems with an emphasis on the Hass avocado crop.
DIGITAL AGRICULTURE APPROACH: GENERAL CONSIDERATIONS
In recent years, the need for new technologies in agricultural systems has been characterized by a continuous search for sustainable solutions to face global challenges, highlighting the technological tools that improve the analysis of information and the understanding of biological phenomena. New technologies have allowed a better understanding of plant interactions with biotic and abiotic actors, generating criteria for the optimization of processes, conservation of biodiversity, efficient use of resources, mass yields, and management of phytosanitary problems (Fig. 1).
This new trend in agriculture has been named digital agriculture (DA), smart agriculture, or agriculture 4.0, all these related to the same concept (Rose and Chilvers, 2018; Sharma et al., 2020). The main objective of this new era in agriculture is the management of information for correct decision-making and the search to massify the yields with a lower economic and environmental cost. This revolution implies the use of technologies such as information and communication systems, remote and proximity sensors, bio-modeling, programming, robotics, the cloud, IoT, big data analysis, artificial intelligence, machine learning, blockchain, mobile applications, and electronic devices (Karmas et al., 2016; Kamilaris et al., 2017; Kamilaris and Prenafeta-Boldú, 2018; Ramírez-Gil et al., 2018b; Smith, 2018; El-Gayar and Ofori, 2020; Sharma et al., 2020).
The DA approach presents multiple challenges to achieve a high impact on a diversity of producers, farm sizes, production systems, cultures, and social aspects. These challenges are associated with: (i) the need for reliable, fast, and accurate information as possible; (ii) design of flexible tools; low-cost solutions; (iii) easy-to-implement and friendly-interface alternatives to users, (iv) open-source technologies; and (v) responsible innovation (Ramírez-Gil et al., 2018b; Rose and Chilvers, 2018; Rijswijk et al., 2019).
The practical application of the DA approach in different parts of the value chain of agricultural systems has multiple cooperative advantages (Smith, 2018; Sharma et al., 2020). In this work, we suggest that the design and practical applications of technological developments associated with DA have the following aspects: (i) objective and problem to be solved; (ii) correct use of the principles and theoretical conceptualization of algorithms, processes, and tools used; (iii) resources necessary for its implementation under field conditions; (iv) products generated, correct interpretation, and their limitations; and (v) economic viability.
In figure 1, we present the main objective, some tools, and the potential products of the DA approach. The implementation of DA tools has contributed to a better land use; more sustainable agronomic practices; less environmental impact; greater conservation of species; indirect detection of pests; harvest forecasts and planning; irrigation management, fertilization and sanitary problems stand out; and climate prediction (Chevalier et al., 2012; Kamilaris et al., 2017; Kamilaris and Prenafeta-Boldú, 2018; Ramírez-Gil et al., 2018b; Rijswijk et al., 2019; Sharma et al., 2020).
Our results suggest that the practical application of AD tools in avocado production systems is not widespread worldwide. This situation indicates the great potential that this type of technology can generate throughout the value chain. It also indicates the great challenge that it means for the sector to be able to incorporate the new developments associated with AD and its application as a basis for evidence-based decision-making.
THE USE OF PROXIMAL SENSING
Soil-based Sensors
A broad set of soil-based sensor technologies to schedule avocado irrigation have been reported (Crowley and Escalera, 2013). These imply monitoring the soil water content (SWC) with time-domain reflectometry (TDR) probes, capacitance sensors, or reflectometry probes, or the soil matric potential (SMP) with tensiometers or granular matrix sensors (Scanlon et al., 2002). However, most irrigation studies in the Hass and other avocado cultivars have used SMP devices to schedule irrigation (Tab. 1). SMP is the amount of energy exerted by the soil particles to retain water (Miyazaki, 2005), which does not depend on the texture and other soil-related factors. Therefore, SMP measurements take advantage of SWC readings because SMP permits standardizing thresholds to initiate and stop the irrigation (Dabach et al., 2016). Some reporters considered the used of tensiometers as essential in avocado orchards to avoid over and subirrigation (Goodall, 1986; du Plessis, 1991).
Early experiences in the use of tensiometers to irrigate avocados were reported in several studies. Incipient knowledge about SMP thresholds to irrigate the crop led to select −1000 KPa (a value close to permanent wilting point) as one of the irrigation-triggering treatments. Due to tensiometer readings not falling below −80 KPa, the authors used resistance blocks to reach this value and consequently activate the irrigation. After this treatment was applied, the tree trunk diameter growth significantly reduced (Richards et al., 1962). Other authors reported an operational basis to schedule the avocado irrigation using tensiometers in drip irrigation systems in the United States (Gustafson et al., 1979). In addition, one of the first in establishing rationale thresholds to irrigate avocados was reported using water potential dynamic of the soil-plant-atmosphere continuum (Bower, 1979). He stated the total stomatal closure occurred at approximately −55 kPa and recommended maintaining the SMP between −25 KPa and −60 KPa and starting the irrigation when the soil reaches −50 KPa.
In a long-term irrigation experiment carried out between 1968-1974 and 1974-1980 (Tab. 1), assessed the effect of 7, 14, 21, and 28-d irrigation intervals (thresholds to start the irrigation ranged from −20 to < −80 KPa) on tree parameters of Ettinger, and Fuerte avocado cultivars. The application of the 21-d irrigation interval (corresponding to the irrigation trigger of −40 KPa) saved 24.9% of water (compared with the 7-d irrigation interval) while evidencing no significant reduction in trunk diameter, tree canopy volume, and yield. However, this produced a cumulative water deficit in deep soil layers throughout the irrigation season (Kalmar and Lahav, 1977; Lahav and Kalmar, 1983).
Table 1. Studies reporting soil potential matric (SMP) thresholds to trigger irrigation.
Author(s) | Region | Avocado cultivar | Crop age (years) | Sensor depth (m) | Soil texture | Irrigation system | SMP treatments (KPa) | SMP to start irrigation (KPa)2 |
---|---|---|---|---|---|---|---|---|
Richards et al. (1962) | Riverside, United States | Hass | NR1 | 0.30 | Coarse | Sprinkler | -50, 100, and 1000 | -50 (The best treatment) |
Bower (1979) | Natal, South Africa | Fuerte | 9 | 0.30 and 0.50 | NR | Draglines | NR | -50 |
Lahav and Kalmar (1983) | Acre, Israel | Hass, Ettinger, and Fuerte | 11 | 0.30 | 60-63% Clay | Sprinkler | -25 and -40 | -40 |
Bower (1985) | South Africa | Fuerte | 5 | 0.30 | Clay | Microjet | -80, -55, and -35 | -55 (the best treatment) |
Goodall (1986) | United States | NR | NR | 0.30 and 0.60 | Sprinkler, micro-sprinklers, and drip | NR | 40-50 for sprinkler, 30-40 for micro-sprinklers, and 20-30 for drip | |
du Plessis (1991) | NR | NR | NR | 0.30 | Sandy and Clayey | NR | NR | -30 (sandy) and -50 (clayey soils) |
Whiley (1994) | Queensland, Australia | Hass | NR | 0.30 and 0.75 | Clay Loam | Mini-sprinklers | NR | -40 (0.30 m depth), -50 (0.75 m depth) |
Vuthapanich et al. (1995) | Queensland, Australia | Hass | 7 | 0.30 | NR | Micro-sprinklers | -20, -40, and -70 | -20 (the best treatment) |
Hoffman and du Plessis (1999) | Nelspruit, South Africa | Fuerte and Hass | NR | 0.30 and 0.60 | Clay | Micro irrigation | -30 and -60 | -30, -60, and -30 (by season) |
Román-Paoli et al. (2009) | Isabella, Puerto Rico | Simmonds | 8 | 0.30 and 0.45 | Coarse | Micro-sprinklers | 10-15 and 40-45 | 40-45 |
Doupis et al. (2017) | Greece | Fuerte and Hass | 2 | 0.20 | Sandy Loam | Manual | NR | -30 (used as the well-watered treatment) |
Silber et al. (2019) | Western Galilee, Israel | Hass | 5 | 0.40 | 60% Clay | Drip | NR | -20 (used) |
Tzatzani et al. (2020) | Greece | Fuerte and Hass | 2 | 0.20 | Sandy Loam | NR | NR | -30 (used as the well-watered treatment) |
1 Suggested, used, or determined after the SMP treatments application.
2 NR, not reported.
SMP devices must be buried permanently in the soil according to the highest avocado tree's root density depth (Lahav and Kalmar, 1983), which is usually no deeper than 0.60 m (du Plessis, 1991). The most appropriate SMP devices installation location corresponds to the permanently moist soil area, where the active or feeder roots are found (Fig. 2) (Goodall, 1986). In addition, it is recommended installing several in-depth (Fig. 2) and spatially distributed tensiometers in the field to counteract the high soil spatial variability (Crowley and Escalera, 2013), which is one of the critical limitations of soil-based sensors (Van Pelt and Wierenga, 2001). Once installed, tensiometer and granular matrix sensor measurements must be read manually and stored in data loggers or on the cloud, respectively. Although manufacturers' technical sheets state that current tensiometers and granular matrix sensors can read SMP in the ranges of 0, −100 KPa, and from 0, −200 KPa, respectively, in practice, these ranges can oscillate in either direction.
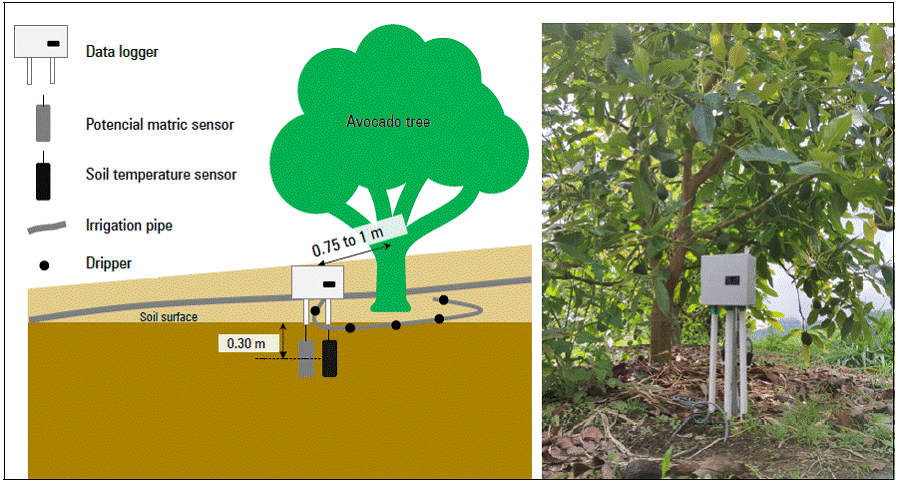
Figure 2. Representation of a soil potential matric sensor location (left) and 15 and 30-cm-depth soil potential matric sensors installed in a Hass avocado orchard (right).
Irrigation scheduling using these devices consists of identifying the appropriate moment to start and stop the irrigation event and establishing the lowest and the highest SMP limits, respectively. An irrigation event is triggered when the SMP falls below the lowest limit, and this event ends when the water replenishing within the root zone rises the SMP back to its highest limit. After a comprehensive review of those SMP thresholds as presented in table 1, it can be affirmed that although the most used lowest SMP limit is −50 KPa, an accurate selection of this limit depends on the irrigation system and the soil texture. According to Goodall's recommendation (Goodall, 1986) (Tab. 1), high-frequent systems require the lowest threshold close to field capacity (FC), while low-frequent systems require an SMP threshold far from FC. Due to water in sandy soils moving faster than in those clayey soils, the lowest SMP threshold in sandy soils must be close to FC, as this must be far from FC in clayey soils (see du Plessis' recommendation in Tab. 1). Although this was not detailed in most of the studies of table 1, it can be inferred that the highest SMP limit used to stop the irrigation was −10 KPa (FC). When two SMP devices are installed in the field, it is recommended to use the shallowest readings as the lowest SMP threshold, and the deepest readings as the highest SMP threshold (Goodall, 1986).
Plant-based sensors
Plants are living systems that take a small amount of water transported by energy gradients through the continuum soil-plant-atmosphere (Kramer, 1983). An imbalance among the soil water availability, water used by plant-water-related processes, and the evaporative demand causes plants to endure water deficit stress (Taiz and Zeiger, 2002). It is noted that plants reveal water stress through the water and energy status, electrical potential, flux of related fluids, pressure, or size variation of the trunk, stems, leaves, tissues, and other vegetative organs (Fernández, 2017). The consequences of water stress in some plants can be temporary (i.e., reversible) due to their stress adaptation mechanisms or permanent, modifying their life cycle (Silber et al., 2013). In this sense, the plant-based irrigation approach takes advantage of detectable water-deficit-stress plant signals to establish reasonable limits to trigger the irrigation (Jones, 2004).
The most common plant-based variables tested as potential triggering-irrigation parameters in Hass avocado crop are the maximum daily trunk-diameter variation (MTDV), trunk diameter growth rate (TGR), trunk diameter shrinkage (TDS), stem and leaf water potential (SWP and LWP), leaf stomatal conductance (gs), fruit diameter variation, leaf voltage differences, and photosynthesis rate (Turner et al., 2001; Winer and Zachs, 2007; Gil et al., 2011; Silber et al., 2013, 2019). A detailed list of water stress indicators in tree orchards is provided (Fernández, 2017). Furthermore, some authors had been classified in non-automated and automated the methods to measure plant-based variables (Fernández, 2017). Porometers, infrared gas analyzers, portable photosynthesis systems, and Scholander chambers are part of the first group. In the second group sap flow sensors, magnetic leaf patch-clamp pressure probes and TDR probes are included.
Despite their potential benefits as described in detail (Jones, 2004), the following limitations restrict in-field usage of plant-based methods to the wise irrigation management of avocado orchards (Jones, 2004; Silber et al., 2013; Fernández, 2017):
The variability of in-field LWP measurements is equal to or greater than the lowest threshold (−2 MPa), making it challenging to recognize the right time when the plant has reached the LWP threshold.
Specific processes of avocado tree flushes could induce physiological parameters and water demand changes, resulting in a lack of precise indicators of plant-based thresholds.
The tree growth rate of some woody crops such as the avocado, depends on alternate bearing years, and this involves relative trunk diameter measurements in on-crop and off-crop years.
Most plant-based water stress indicators exhibit a recurring-diurnal behavior, which means they decrease at midday and increase at night, reaching a peak in the early mornings.
Plant-based sensors can be calibrated to identify the plant's water-stress condition and, consequently, trigger the irrigation. However, these sensors neglect how much water needs to be applied and the right moment to stop irrigation.
A few studies have attempted to establish rationale irrigation indices for the Hass avocado crop. In an experiment the authors tested triggering the irrigation when gs fell below 25% (by arbitrary criterium), obtaining a reduction of 33% in the water applied and of 30 fruits per tree, as compared to the 120% pan evaporation triggering irrigation treatment (Turner et al., 2001). In addition, Winer and Zachs (2007) proposed a method to remove the water-stress cumulative effect when the soil water was depleting from the MTDV, joining successive MTDV peaks through a reference line. The authors affirmed this could improve water irrigation management decisions for avocado orchards. On the other hand (Oyarce and Gurovich, 2010), measured in laboratory conditions a significant electrical potential falling of 7.10 ± 1.56 mV in the trunk (recorded at 25 cm above the ground) of 2-year trees when the irrigation was applied.
Silber et al.(2012) and Silber et al. (2013) delved into the response of irrigation treatments to IS plant-based parameters of Hass avocado trees, considering the effect of environmental variables on these parameters. Additionally, has been reported a poor hourly correlation between the tree's water uptake, the applied water volume, and the trunk diameter growth. As the hourly water uptake rate peak occurred around midday (12 L h−1 / tree), the trunk diameter growth decreased (the 0.1-mm peak was identified in the early morning, around 6 AM) (Silber et al., 2012). The daily course of the trunk diameter growth responds to a circadian-cycle behavior. In this same study, the authors determined that TGR was inversely correlated to the hourly vapor pressure deficit. TGR reached negative values (i.e., a shrinkage) in the early afternoon but returned to zero or positive values at night. Therefore, it can be affirmed that differential irrigation strategies significantly influence plant-based parameters of avocado trees at the end of the season. However, no apparent effects were found on the daily dynamics, which is an indispensable condition required for effective IS. Furthermore, any plant based IS strategy must be calibrated using the actual crop water requirement (Gu et al., 2020).
THE USE OF REMOTE SENSING
RS fundamentals
Remote sensing is a term that gathers a broad set of non-contact platformed-based sensors, techniques, models, communication protocols, and applications providing electromagnetic spectrum, geometrical and biophysical data from the earth's surface and atmosphere (Tempfli et al., 2009). Based on the acquisition-data distance above the earth's surface, sensors can be classified into the following categories: ground-based, (i.e., systems articulated to terrestrial vehicles and hand-held sensors); aerials, which include platforms on airplanes and unmanned aerial systems (UAVs); and space-based, as integrated by satellite constellations (Sishodia et al., 2020). From reduced spectral datasets in the 1970s (Madry, 2017), the RS concept became an integrated related products' collection boosted through the internet of things (IoT) and artificial intelligence (AI) (Jung et al., 2021). Stored in a proper location and warranting their accessibility and ease to interpreting, RS data could be regarded as a common heritage of humankind that provides factual evidence of historical and current changes of the earth (Pelton et al., 2017).
Platform-based remote sensors measure the amount, quality or surface-sensor traveling time of the energy emitted or reflected by the objects' surface on the earth or the atmosphere's particles. Active sensors emit their energy over the target's surface and measure the resulting reflected energy, and passive sensors measure the reflected energy primarily sourced by the sun (Reddy, 2018). Target's surface properties such as scattering, absorbance, and the signal's angle, direction, and polarization are measured by remote sensors (Tempfli et al., 2009). Sensors capture the energy in some wavelength ranges of interest. The most common to agricultural applications are the so-called visible (0.38-0.75 μm), infrared (0.7-1 μm), short-wavelength infrared (1.5-3 μm), thermal infrared (3-15 μm), and microwaves (1 mm-1 m). The number of pixels with which the surface is rebuilt, revisiting days, and wavelengths captured by these sensors correspond to the spatial, time, and spectral resolution (Sishodia et al., 2020). RS measurements become biophysical variables through empirical and theoretical models, which must be calibrated with field readings (Tab. 2) (Huang et al., 2018). Pondering the shortage of RS concepts presented above, an extended background is thoroughly described by different studies (Schowengerdt, 2007; Tempfli et al., 2009; Pelton et al., 2017).
Since Google Earth Engine's (GEE) arrival in 2010, the use and application development of the satellite RS have massified enormously (Tamiminia et al., 2020). GEE is a cloud-based petabyte platform that provides a refined way of acceding, visualizing, downloading, and processing publicly accessible, near-real-time, and historical satellite RS datasets (Gorelick et al., 2017). In its robust platform, GEE hosts earth's observations from Landsat, Sentinel, and MODIS projects; high-resolution imagery from Planet SkySat and The National Agriculture Imagery Program (NAIP); biophysical (DEMs, landforms, lithology, and vegetation coverages); environmental (ecoregions, deforestation, emissions, and forest); and climate-weather (surface temperature, LAI, rainfall, water vapor, and droughts indices) datasets (Google Inc., 2021). Significant disparities are presented in the coverage, processing level temporal, and spatial resolution, and quality of these datasets by which users are aimed to understand the specific features of the dataset of interest (Xie et al., 2019). Functionalities such as ready-to-use datasets, parallel processing, machine learning, image and specialized packages, and object-oriented programming (Tamiminia et al., 2020), allow users to convert RS datasets into excellent end products.
RS applications in agriculture: Irrigation traits
Even though there is a broad range of approaches to classify RS applications in agriculture (Weiss et al., 2020), the most integral, suitable-for-farmers, and challenging must be based on agricultural tasks (Sishodia et al., 2020). Taking advantage of recent IoT and AI advances in agriculture (Singh et al., 2021), an ideal task-based approach could consist of: acceding RS data stored on the cloud or downloading data from in-field RS platforms; processing data using theoretical, empirical or AI models by agronomists and RS experts (Huang et al., 2018); uploading the modeling results on the cloud to the end-user; accessing those modeling results through a smartphone app; and deciding how to improve the task of interest comparing the modeling results with field observations (Fig. 3). The usage of RS in new scouting areas, soil survey, land designing and preparing, seeding, irrigation (Tab. 2), drainage, fertilization, weed and pest management, and harvesting has been appropriately documented (Sishodia et al., 2020; Weiss et al., 2020).
Table 2. Remote sensing platforms, characteristics, and models used in irrigation traits.
Platform | Bands / B. Variables | Resolution | Trait | Model name (References)1 | |
---|---|---|---|---|---|
Spatial (m) | Temporal (d) | ||||
Landsat L5, L7, and L8 | RGB, NIR, SWIR, TIR | L5 RGB-NIR-TIR: 30 L7 and L8 RGB-NIR- SWIR: 30 TIR: 100 | L5, L7, and L8: 16 | Evapotranspiration | ALEXI, Dis-ALEXI (Knipper et al., 2019); pySEBAL, SEBS, and METRIC (Xue et al., 2020) |
Variable rate irrigation | TSEB (Barker et al., 2018; Bhatti et al., 2020); NDVI -Kc (Mendes et al., 2019) | ||||
Crop water stress | CWSI (Veysi et al., 2017) | ||||
Monitoring irrigation water use | Kc - vegetation indices (Bretreger et al., 2020) | ||||
Irrigation Efficiency | Evapotranspiration-SEBAL (Awada et al., 2019) | ||||
Crop water consumption | Evapotranspiration-SEBAL (Costa et al., 2019) | ||||
Groundwater | NDVI-Evapotranspiration (Nhamo et al., 2020) | ||||
MODIS | TIR | 1000 | 1 | Soil moisture content | DISPATCH (SMOS) (Fontanet et al., 2018) |
NR | 500, 1000 | 8 | Evapotranspiration | ASEBAL (Silva et al., 2019) | |
Albedo, LST | 5600 | 1 | Irrigated areas detection | Irrigation map (Zohaib et al., 2019) | |
SR 1-7, Albedo, LST | 250-500, 1000, 1000 | 1, 8, 1 | Irrigation efficiency | Evapotranspiration-SEBS (Ma et al., 2018) | |
Sentinel-1 | C-Band | 10 | 6 | Soil moisture content | ML (Datta et al., 2020) |
Irrigation events detection | IED (Bazzi et al., 2020) | ||||
Sentinel-2 | R, NIR | 10 | 2-3 | Evapotranspiration | LAI-WDVI (Schulz et al., 2021) |
G, R, NIR, SWIR | 10-20 | 5 | Monitoring irrigation water use | HidroMap (Piedelobo et al., 2018) | |
AMSR2 | Soil Moisture | ~25 km | 1 | Irrigation water use | SM2RAIN (Jalilvand et al., 2019) |
UAVs | TIR | NR2 | 6 | Crop water stress | CWSI (Quebrajo et al., 2018) |
Ground-based vehicles | RGB, RE, and NIR | ~0.06 | 22 | Crop water stress | Vegetation indices (Ranjan et al., 2019) |
TIR | NR | 1 | Transpiration | 3T (Asher et al., 2013) |
1 Most of models use two or more sub-models and RS platforms.
2 NR, not reported.
Delving into the irrigation studies using RS products, it can be inferred that most of them computed instantaneous evapotranspiration (ET) and the basal crop coefficient (K cb ) from optical satellite imagery to manage the irrigation. In such studies (Tab. 2), ET was estimated through surface energy balance models (ALEXI, Dis-ALEXI, SEBAL, TSEB, and METRIC) and K cb was estimated by correlating vegetation indices (NDVI) with theoretical or field K cb measurements. Furthermore, table 2 helps to identify, as a first step, the suitable RS platform for several irrigation traits. A study described a web-GIS-based decision support systems required to schedule irrigation based on ET and K cb (Calera et al., 2017). One of these is IrriSAT (Hornbuckle et al., 2016), a web platform that uses Landsat and Sentinel optical satellite imagery to estimate K cb from NDVI values.
Despite the resolution-related advances described previously, most notably in the tropics, the following factors hinder practical applications of RS-based IS in crops: the high-resolution RS datasets required to schedule irrigation at a plot scale (Calera et al., 2017) do not have a high-frequent revisiting time needed to daily track the soil water depletion (Li and Roy, 2017); the high-cloud coverage in the tropical hillslope areas evidenced for most of the year (Prudente et al., 2020), does not provide cloud-free time series pixels to compute the irrigation parameters; although most studies in table 3 did not include precipitation in water balance, it has a critical influence on the soil water balance (SWB) throughout the year (Richter, 2016); and some RS models required to compute ET such as METRIC (Olmedo and de la Fuente-Saiz, 2018), must be calibrated with hourly climate data corresponding to the area of interest.
Soil moisture retrieval from SAR images
The soil moisture content (SMC) plays a role in the hydrologic processes that control the water availability for life on earth (Salas et al., 2014). Plants can fulfill their growth cycle thanks to the water regulation capacity exerted by soils. Measuring SMC to schedule irrigation allows for the direct detection of cumulative and instantaneous water changes in the soil (Gu et al., 2020), and no other variable or IS approach offers such flexibility. The lack of a wide spatial influence of proximal sensors' punctual SMC readings (Rodríguez et al., 2018) is outperformed by the global coverage offered by remote sensors (Gorelick et al., 2017). Moreover, the soil moisture can be retrieved through optical and radar satellite images (Calera et al., 2017). As the optical images acquisition process is hampered by the high cloud coverage in the tropics (Prudente et al., 2020) and highly conditioned by target-surface-reflectance properties (Dorigo et al., 2015), radar sensors can work 24 h a day while not being affected by the atmospheric scattering and while controlling the energy emitted to the target surface (Tempfli et al., 2009).
Spaceborne radar platforms can be passive or active. Passive platforms receive naturally emitted energy by objects on the earth's surface, while active platforms emit energy pulses and collect radiometric and geometrical properties of objects upon the earth's surface in wavelengths from 1 cm to 1 m in distinct bands of interest (Reddy, 2018). Such bands are labeled with capital letters P, L, S, C, X, K, Q, V, and W, from the shortest to largest wavelength (Schowengerdt, 2007). Bidirectional emitted and reflected energy pulses (according to the electromagnetic theory) are decomposed into horizontal (H) and vertical (V) vectors, producing a set of co-polarized (VV or HH) and cross-polarized (VH or HV) microwave bands (Parikh et al., 2020). Multi-polarization sensors (e.g., Radarsat-2) can retrieve several polarized bands (Sinha et al., 2018), and others do this in single bands.
By advancing the sensed surface exposition time, synthetic aperture radar (SAR) technology has enhanced the spatial resolution of images (Tempfli et al., 2009). Singhroy (2017) described current and future radar satellite platforms' characteristics and affirmed that some publicly available SAR satellite projects like Sentinel-1 by ESA, AMSR-E, SMAP, and SMOS by NASA, among others, have been used for SMC estimation (Sishodia et al., 2020). According to Peng et al. (2021), Sentinel-1 is currently the most sophisticated SAR platform to estimate SMC. In orbit since April 2014 and aimed to map the planet, this mission provides radiometric data in C-band. Its revisiting time in the tropics is 12 d with a spatial resolution of 20 m (ESA, 2021). The Ground Range Detected (GRD) Level 1 Sentinel-1 dataset is available on GEE and can be fully accessed with name collection 'COPERNICUS/S1_GRD' (Google Inc., 2021).
The energy intensity captured by radar platforms depends on sensor-related factors (e.g., polarization and wavelength), the platform pathway (e.g., incidence angle and the trajectory direction), and the large-and-short-scale surface properties (Tempfli et al., 2009). Surface properties at large scales lead to terrain distortions in the capturing process, which must be corrected to effectively interpret on-ground observations (Vollrath et al., 2020). On a short scale, the roughness and dielectric properties and the surface coverage type (bare soil or vegetation) define the noise of the signal (Reddy, 2018). To retrieve SMC, this noise is theoretically or empirically analyzed through backscattering models (BM) (Hoeben et al., 1997). Moreover, machine learning (ML) and AI approaches are faster and simpler alternatives to conventional models (Datta et al., 2020). Notably, the radar estimated SMC represents the amount of water in the first 10 cm of the soil profile, which is perhaps its main limitation (Peng et al., 2021). On bare soils, the integral equation method (IEM), advanced integral equation model (AIEM), Dubois, and Oh are the most used BM (Choker et al., 2017). The semi-empirical water cloud model is the most reported in vegetation-covered soils (Kweon and Oh, 2015). A comprehensive review of BM for retrieving SMC is found in Karthikeyan et al. (Karthikeyan et al., 2017).
Low spatial and temporal resolutions hinder practical applications of SAR images in agriculture (Peng et al., 2021). Considering irrigators who require near-real-time data availability to trigger, for instance, irrigation events and finer spatial resolution (compared to the unit management) to differentiate the water amount and timing by plot, radar images must be carefully used. However, studies in irrigation detection and water volume estimation have been reported. Brocca et al. (2018) used SMAP, SMOS, ASCAT, and AMSR2 radar mission data (~12.5 km for the finer spatial resolution and a daily revisiting frequency being the most common) to estimate the irrigation water amount through the SM2RAIN algorithm. They validated their results in nine areas of the United States, Europe, Australia, and Africa and found a good correlation between the monthly irrigation amount radar estimates and field measurements. In this regard, Jalilvand et al. (2019) retrieved SMC from AMSR2 data (daily revisiting time and ~25 km of spatial resolution) by using the SM2RAIN algorithm in the Miandoab Plain (Iran). The radar SMC estimates performed by authors followed the temporal soil water dynamics, overestimating the amount of water needed for irrigation.
On the other hand, Le Page et al. (2020) successfully detected irrigation timing in six maize plots (southwest France), analyzing direction changes of the SMC time series retrieved through Sentinel-1 observations. They recommended using SAR images with revisiting times of 2 to 4 d to manage the irrigation. Consistent with the study above, Bazzi et al. (2020) retrieved SMC from Sentinel-1 data using the irrigation detection BM (IDM) to detect near-real-time irrigation events in 46 intensively irrigated grassland plots in Crau Plain (France). The novel IDM operates at grid (10 x 10 km) and plot scales to discriminate changes in the Sentinel-1 backscattering coefficient σ 0 due to precipitation and irrigation, respectively. Irrigation events were accurately detected by this method. In addition, Lawston et al. (2017) compared the efficiency of five low-spatial-resolution SMC products (SMAP at 1 km and 9 km, SMOS at 1 km, ASCAT at 12.5 km, and Sentinel-1 at 1 km of spatial resolution) to discriminate irrigation from rainfed crops in north-eastern Spain. SMOS and SMAP were the most relevant datasets in detecting irrigated areas. Other studies combined optical and radar platform data to boost former images in irrigation traits (Bousbih et al., 2018; Fontanet et al., 2018; Datta et al., 2020; Lozac et al., 2020; Dari et al., 2021).
IRRIGATION SCHEDULING APPLICATIONS
Applications comparison
Although it is demonstrated that using precision irrigation technologies significantly improves irrigation management (Abioye et al., 2020), most irrigators, instead of scheduling irrigation using these technologies (Khabba et al., 2013; Vellidis et al., 2016), irrigating empirically based on external variables such as water and personnel and infrastructure availability, crop water deficit signs, and commercial trends. Multiple causes explain why irrigators do not implement new irrigation technologies (Abdullah and Samah, 2013; Mottaleb, 2018). Irrigation scheduling decision-support applications such as smartphone apps, web-based platforms, and desktop programs bridge the existing timing and water-related knowledge gap in irrigation methods (Migliaccio et al., 2016). Thousands of valuable irrigation-related data can currently be retrieved in a publicly available and near-real-time manner through online applications. Unfortunately, the poor internet connection in worldwide rural zones impedes their use (Chiaraviglio et al., 2017).
An extended and diverse ecosystem of applications to schedule irrigation is found on the web, as intended for various crops, farming organizations, commercial and research interests, output targets, available input data, and development levels. A small sample is characterized in table 3, taken from studies (in journal indexes) that reported their use and irrigation companies' catalogs on the web. According to the listed IS applications developers, primary motivations to build these are set out to provide farmers with an easy-to-use tool to support irrigation management decisions, saving water irrigation, and optimizing the water productivity of selected crops. IS applications are structured into the core engine, where the IS model is allocated; the database, where input, ancillary, and output data are hosted; and the graphical interface, where users interact with the application.
Table 3. Characteristics of some mobile, web, desktop, and multiplatform IS apps.
Platform | Name | Reference | Newest Version | Operating System | Free Access | IS Approach2 | Available input data? |
---|---|---|---|---|---|---|---|
App | Smartirrigation | (Migliaccio et al., 2016) | 1.1.2 | iOS, Android | ✓ | SWB | ✓ |
SoilWaterApp | (Freebairn et al., 2018) | 8.0.3 | iOS | ✓ | SWB | X | |
Irrigator Pro | (Sigua et al., 2017) | 2.0.3 | iOS, Android | ✓ | SWB, PS | ✓ | |
Chloe | (LP Laboratories, 2019) | 1.1 | Android | ✓ | PS, RS | ✓ | |
IrriMobile | (Ferreira et al., 2020) | 1.0.2 | Android | ✓ | SWB | X | |
VegApp | (Miller et al., 2018) | 4.3.2 | Android | ✓ | SWB | ✓ | |
Crop Water | (UNL, 2019) | 2.0 | iOS, Android | ✓ | PS | ✓ | |
SWAMP Farmer | (Sales et al., 2020) | 2.4.0 | Android | ✓ | SWB, PS | X | |
Web-based | CIMIS | (Kisi, 2011) | NR1 | Web | ✓ | SWB | ✓ |
IRRISAT | (Hornbuckle et al., 2016) | NR | Web | ✓ | RS | ✓ | |
AQUAMAN | (Chauhan et al., 2013) | NR | Web | X | SWB | ✓ | |
IRROcloud | (Irrometer, 2021) | NR | Web | X | PS | X | |
IRRIX | (Domínguez-Niño et al., 2020) | NR | Web | X | PS | X | |
EO4Water | (IVFL, 2021) | NR | Web | ✓ | RS | ✓ | |
Desktop program | CROPWAT | (Smith, 1992) | 8.0 | Win | ✓ | SWB | ✓ |
PROBE-w | (Chopart et al., 2009) | 1.0 | Win | ✓ | SWB | X | |
DIDAS | (Friedman et al., 2016) | 1.1.1 | Win | ✓ | SWB | X | |
AquaCrop | (Linker and Sylaios, 2016) | 6.1 | Win | ✓ | SWB | X | |
IMIS | (Ng Cheong and Teeluck, 2018) | 1.0 | Win | X | SWB | X | |
FIS-DSS | (Yang et al., 2017) | 1.0 | Win | X | SWB | X | |
BUDGET | (Raes, 2002) | 5.0 | Win | ✓ | SWB | X | |
Multi-platform | IrriMAX | (Sentek, 2019) | 10.1 | Win, Android | X | PS | X |
HidroMap | (Piedelobo et al., 2018) | NR | Win, Web | ✓ | RS | ✓ | |
IrrigaSys | (Simionesei et al., 2020) | NR | Web, Android | ✓ | SWB | ✓ | |
Lynks App | (Lynks Ingeniería, 2016) | 1.2.4 | Win, Android | ✓ | PS | X |
1 NR Not reported. 2 SWB: Soil Water Balance; PS: Proximal Sensing; RS: Remote Sensing.
Apps boast user-friendly, geo-location, graphical, intuitive, free-access, and tactile front ends, but lack robust-IS-model back-ends. Many of them are developed for both iPhone (iOS) and Android operating systems. Web-based platforms, as depicted in table 3, are designed to store and accede to available (e.g., remote sensing RS) data on the cloud (as symbolized in the available data and IS approach columns in table 3 with ✓), which can be processed, retrieved, and interpreted easily by farmers. These platforms run on web browsers where users must always log in to access their services thus avoiding inter-operative system obstacles. Desktop programs are boosted by the robustness, stability, and offline advantages of desktop computers to implement SWB complex models. However, the high and specialized knowledge required to operate them commonly exceeds farmers' abilities, and thus these programs must be managed by agronomists, who as a result occasionally assist farmers in the field. As a result, desktop programs lack enough portability to support farmers' in-field decisions.
The main strength of multi-platform applications is their responsive web design, which easily overcomes the agronomist-farmer problem described previously. Moreover, users with administrator, agronomist, or farmer roles can monitor real-time PS devices and feed these applications with data. A flaw associated with apps, web-based, and multi-platforms is their online dependency since rural zones lack a stable internet connection. Being state-of-art and portable and offering client support, commercial applications are more beneficial than free access applications (symbolized in table 3 with ✓). Farmers who need to choose the right IS application undertake a complex task because of the enormous number of variables to consider.
Apps
First attempts to deal with irrigation traits through mobile appliances were made through PDA and PC pockets (Hornbuckle et al., 2006; Molina-Martínez and Ruiz-Canales, 2009), equipped with a GPS sensor, a tactile screen, a camera, and an operating system. The irrigation software was then externally programmed in LabView, Visual Basic, Java or C, and installed on these devices. Early efforts to remotely control the irrigation through smartphones were accomplished by wiring PS devices in the field to a central unit, processing PS readings, and triggering irrigation event once the SMP threshold was reached (Fernández et al., 2008). All these strives were made in parallel with Android and iOS projects launched between 2005-2009 (Islam and Want, 2014), and the mobile applications were trending in the agriculture field in this same period. Regarding the studies mentioned above, the most limiting factors to achieving a whole operative irrigation system via the available smartphone apps were the lack of operational wireless PS infield networks, which made it challenging to monitor broad areas; the limited battery life, memory, and storage resources; and the expensive implementation.
Between 2010 and 2019, various studies demonstrated how apps were used and evaluated in-field practices, highlighting their valuable support to farmers (Hamad et al., 2018). Authors as Dehnen-Schmutz et al. (2016) reported that although most farmers owned a smartphone, more than one-third of those interviewed did not use any agriculture app. In addition, Pongnumkul et al. (2015) described the use of apps in agriculture in 2010-2014, underlining GPS and cameras as the most-used smartphone sensors for various agricultural tasks. Meanwhile, Kaewmard and Saiyod (Kaewmard and Saiyod, 2014) designed an automatic irrigation system and tested its start-stop irrigation signal transmission's accuracy, finding an accuracy greater than 95%. Taking advantage of available real-time data from weather networks in Georgia and Florida, the United States, Migliaccio et al. (2016) developed irrigation scheduling smartphone apps for avocado, citrus, cotton (called Cotton App), peanut, strawberry, and vegetable crops, grouped by the name Smartirrigation Apps (Tab. 3). When compared to other IS methods, Vellidis and others (Vellidis et al., 2016) found that after using Cotton App to schedule irrigation, the cotton yield was significantly higher in the 2013 and 2014 seasons and higher in the 2015 season, and the water use efficiency (WUE) was higher in the 2013 and 2014 seasons.
The IS smartphone app used by the avocado growers detailed above goes by the name Smartirrigation Avocado and can be found in the Google Play and Apple App Stores (Migliaccio et al., 2016). This app requires input parameters such as the irrigation system, the crop, the soil type, and IS characteristics to output the accumulated precipitation for the seven previous days, the applied irrigation events timing, and the reached moisture depth. Each day, Smartirrigation Avocado shows the irrigation events required for the next 15 d, based on the five-previous-day crop evapotranspiration, as computed using nearby-station weather data from the Florida Automated Weather Network. Irrigation doses change throughout the crop seasons according to K c of 0.6, 0.8, 0.7, 1.0, and 0.7 to dormancy, flower bud development, flowering and fruit set, fruit growth, and after harvest. Mbabazi et al. (2017) compared the IS provided by this app with SWB field observations and found that the drainage, irrigation depth, and wetted area simulated errors were not significant. Mbabazi et al. (2017) determined that using Smartirrigation Avocado could reduce the irrigation water used for the crop between 62 to 67% moistening the first 12.7 mm of the soil depth by any given event, compared with irrigating three times per week.
Recently, other IS apps have been developed for tomato (Miller et al., 2018) and grains crops (Freebairn et al., 2018), exploiting artificial intelligence techniques (Ferreira et al., 2020), reading soil matric potential devices (UNL, 2019), and integrating IS data to IoT environments (Sales et al., 2020). After validating in field conditions, authors of the three first apps mentioned above concluded IS apps do reliably estimate the soil water dynamic and positively impact WUE. Moreover, other authors compared IS apps features and found the most common were Map View, farm divisions (plots), and irrigation planning (Sales et al., 2020). In line with the agriculture 4.0 concept, IoT and smartphones will lead the progressive transformation of irrigation technology toward irrigation 4.0 as the direct connection between farmers and irrigation systems (Nawandar and Satpute, 2019; da Silva et al., 2020; Li et al., 2020). Therefore, there is enough sustained evidence to affirmatively respond the question put forward of whether crops can be watered by our phones (Puértolas et al., 2019).
CONCLUSIONS
Traditional irrigation scheduling methods have been invariantly reported in Hass avocado irrigation dedicated studies for decades. Soil water balance and soil-based sensors such as tensiometers and granular matrix sensors are the most mature methods. Fortunately, new digital agriculture technologies are changing how such methods and their data are controlled in the field and handled, respectively, boosting the decision-making process toward increasing water use efficiency and the fruit quality parameters and reducing the amount of water used for Hass avocado crop. Although remote sensing technologies are no being widely used in the Hass avocado crop irrigation, radar images stand out above optical images because the surface soil moisture, their specific product to schedule irrigation, can be retrieved in acceptable periods, avoiding the high cloud coverage problem presented by optical images in tropics. Avocado dedicated applications represent a trustful tool for Hass avocado growers to irrigate their orchards technically. Integrating remote and proximal sensing technologies through user-friendly applications can represent a suitable option to improve Hass avocado irrigation in developing countries.