Introduction
The increasing pace of development and fusion of new technologies (robotics, digital-IA, biotechnology, and other forms of automation) has raised growing concern among economists and policymakers about the potential effects of technological disruption in the labor market. Analyses of the effects of technological progress on labor demand have indicated long-term increases in aggregate employment and wages. However, the rapid diffusion of these new technologies has the potential to cause short-term employment declines, especially in low-skill and low-wage occupations, despite increases in overall production output (Acemoglu and Autor, 2011).
The debate no longer centers on whether technological disruption will affect the labor market in the short-term. It is already happening, it is real. To better comprehend these processes, we can categorize them into two channels through which technological diffusion influences the labor market. The first is the direct channel, involving a direct substitution between labor and capital, which, in turn, complements the remaining labor force, resulting a net negative effect. The second is the indirect channel, where increased efficiency in production leads to lower prices and, consequently, higher demand for labor (Li, An and Wang, 2023).
Empirical evidence indicates that more than 50% of jobs are susceptible to these effects. In developing countries, approximately 60% of occupations have a high risk of automation (Santos, Monroy-Taborda and Moreno, 2015). In Mexico, this estimate surpasses 63% of occupations (Minian and Martinez, 2018), while Southeast Asia reports 56% of jobs at high risk of automation (Chang and Huynh, 2016). The percentages are somewhat lower in advanced countries, with 47% for the United States (Frey and Osborne, 2017), 36% for Spain (Dómenech et al., 2018), and 31% for the United Kingdom, Japan and Germany (Hawk-sworth, Audino and Clarry, 2017). Thus, there is clear evidence of differential effects between advanced and developing countries.
Due to the structural challenges faced by emerging economies such as the abundance of low-skilled labor but scarcity of capital, and the fact that low-skilled labor often involves routine jobs that can be easily automated, the labor market in emerging economies faces greater short term difficulties in adapting to and absorbing technological changes. Consequently, countries like Colombia may experience dual changes: the relocation of foreign companies, known as reshoring, and the automation of numerous processes within the local economic sectors. These factors motivate our analysis, where we find substantial effects through direct channels in our labor market.
In this study we contribute to the empirical literature concerning the potential substitutive effects of technology on employment. We identify the proportion of workers in jobs with a high probability of automation and explore the characteristics that can mitigate the disruptive effects of technology in countries like Colombia, where structural issues such as unemployment, informality, low wages, low-skilled labor, and others persist. Consequently, these findings hold importance for employment policies.
Our research reveals that 37% of workers in Colombia hold jobs with a high probability of automation, occupations that technology could potentially replace in the short term. Strong evidence supports the idea that factors such as higher education levels, formal employments status, participation in training courses or job training, among others, can influence job vulnerability. These results align with the Task Model (Autor, Levy and Murnane, 2003) and the Revised Task Model (Frey and Osborne, 2017).
Specifically, the purpose of this paper is to analyze the proportion of workers in Colombia with jobs at high, medium and low risk of automation. Additionally, we investigate personal, occupational, and company characteristics that may reduce such risk. To achieve this, we utilize data from the Gran Encuesta Integrada de Hogares (GEIH) conducted by the Departamento Administrativo Nacional de Estadística of Colombia (DANE) in 2019 and the probabilities of automation estimated by Frey and Osborne (2017).
We develop the rest of the article in five sections: Section II introduces the theoretical foundations that underpin this empirical analysis, allowing us to explore the potential impacts of adopting new technologies on employment levels; Section III presents the data and outlines the empirical strategy; Section IV presents the findings and Section V offers a discussion of the results and presents our conclusions.
Literature review
Empirical evidence
The speed of technological change has compelled economists and policymakers to consider the impact of technology on employment. While this is a well-documented subject, there exists significant heterogeneity in the phenomena of job substitution and automation. Much of the empirical research has concentrated on the effects of technology in the labor markets in advanced countries such as the United States, the United Kingdom, Japan, Germany, Spain, Austria, among others (Frey and Osborne, 2017; Hawksworth, Audino and Clarry, 2017; Dómenech et al., 2018; Aghion, et al., 2020; Acemoglu and Restrepo, 2020; Illéssy, Huszár, and Makó, 2021; Boustan, Choi and Clingingsmith, 2022; Gravina and Pappalardo, 2022; Eckardt, 2022; Lorenz, Stéphany and Kluge, 2023).
Conversely, studies examining countries in emerging economies investigate potential disparities in technological adoption, wage inequalities and shifts in employment structure, and the risk of automation in occupations in countries such as Mexico, Brazil, India, Chile, Indonesia, Latin American economies, among others (Santos, Monroy-Taborda and Moreno, 2015; Chang and Huynh, 2016; Minian and Martinez, 2018; Lima, et al., 2021; Hammer and Karmakar, 2021; Brambilla et al., 2021; Ing and Zhang, 2022; Katz, Callorda and Jung; 2023).
The results and conclusions vary depending on the context and analytical methodology. For instance, in the United States, it was found that 47% of all occupations are at a high risk of automation (Frey and Osborne, 2017), which could have adverse implications for overall economic growth and wages due to the pronounced role of capital relative to labor (Aghion, et al., 2020; Acemoglu and Restrepo, 2020). However, studies by Boustan, Choi and Clingingsmith, (2022) and Li, An and Wang, (2023) indicate increases in productivity and total employment, particularly for unionized workers and individuals with higher levels of education. Similar results have been observed in Hungary, Austria, Spain, the United Kingdom, Japan and Germany, where the proportion of occupations at high risk of automation ranges from 44% and 31%,with these occupations primarly involving routine tasks carried out by less skilled workers (Hawksworth, Audino and Clarry, 2017; Dómenech et al., 2018; Illéssy, Huszár, and Makó, 2021; Lorenz, Stephany and Kluge, 2023).
The evidence for emerging countries suggests the potential for greater employments inequalities, significantly impacting the labor market structure and wages (Brambilla et al., 2021; Katz, Callorda, and Jung, 2023). Santos, Monroy-Taborda and Moreno (2015) found that 60% of occupations in 30 countries developing economies face a high risk of automation. This same percentage was estimated for Brazil, indicating that the probability of automation increases for workers with lower educational levels and salaries, as well as for younger employees (Lima, et al., 2021). For Mexico, Argentina, Chile and Peru, it is estimated that 57% and 67% of all occupations have a high risk of automation (Minian and Martinez, 2018; Brambilla et al., 2021). In contrast, empirical evidence for India suggests that the impact of automation on employment and the labor market will be limited (Hammer and Karmakar, 2021).
Regarding Colombia, Santos et al. (2015) and Brambilla et al. (2021) have analyzed the automation phenomenon, with their results indicating that between 56% and 64% of occupations have a high risk of being replaced by technology. This contrasts with our findings, as we discovered that only 37.5% of employees work in occupations with a high risk of automation. The difference can be attributed to the data and the methodological approach used, our results align with the theoretical models described below.
Theoretical foundation
The way in which capital is replaced by workers, and the associated risks of job automation, aligns with the framework proposed by Autor et al. (2003), which introduces a task-based model to elucidate the routine tasks substitution by computerized capital. For analytical non-routine tasks, there is potential complementarity, following an aggregate production function:
In this equation, Q represent output, resulting from the input of two types of labor: routine labor Lr and non-routine labor LN, C is representing computerized capital. The parameter (3 shows factor participation in production. The model hinges on the steady decline in the price of computerized capital as its the causal force behind this model. The functional form assumes that capital and routine labor are perfect substitutes, whereas capital and non-routine labor are complementary, implying there is substitution elasticity equal to 1 between routine and non-routine labor. The new technology can substitute for labor in routine tasks more than in non-routine tasks. In cognitive non-routine tasks, there is a high likelihood of complementarity, while in manual non-routine tasks, the model concludes these new technologies do not affect them.
The model proposed by Autor et al. (2003) leaves out the possibility of automating manual and cognitive non-routine tasks. In contrast, Frey and Osborne (2017) emphasize that the generation of complex data sets complements ongoing technological advancements, allowing increased computational capacity and automation, even in the case of non-routine tasks. The authors attempted to measure the technological impact on employment, assuming an automation approach to occupations, which is encapsuled in the following model:
Ls represents labor susceptible to automation and LNS represents labor not amenable to automation, which is further divided into the three challenges that the development of automation technologies of any task will have to overcome; Lpm represents perception and manipulation tasks, Lc represents creative intelligence tasks, and Ls represents social intelligence tasks. The authors suggest that technology opens the possibility of automating a wide range of non-routine tasks as long as there is sufficient information and the ability to recognize patterns.
Based on this model and machine learning techniques, Frey and Osborne (2017) rank and assign a probability and risk of automation to 702 occupations. The probabilities reflect the technical feasibility and chances of overcoming bottlenecks to generate the technology to automate one or a set of occupations 20 years from now. The automation risk categorizes occupations based on the predetermined probability threshold (0.3 and 0.7). In this context, the risk categorizes automation probability into high risk (likely to be automated in the short term), medium risk (likely to be automated in the medium term), and low risk (likely to be automated in the long term).
These models highlight a particular feature of the dynamics of the current technological disruption. The conclusion they draw is that the technological change is skill-biased, meaning it makes individuals with high level skills more productive compared to those with medium and low-level skills. High level skills are non-routine, and less susceptible to automation. Conversely, within the medium-level skills, there are repetitive technical tasks, while low level skills do not require great deal of intellectual work but involve unstructured, manual tasks. Thus, new technologies complement workers with high level skills and make them more productive, but replace those workers with medium level skills, who must either acquire skills or perform low skills with lower payment (Autor and Dorn, 2013).
This approach focuses on the future reconfiguration and occupational restructuring to automate any labor. For this to happen, we must consider other types of factors beyond the technical and knowledge possibilities available, such as traditional market mechanisms and investment opportunities, government policies, which are protectionist to a greater extent, as well as economic, political, legal, and social factors.
Data and empirical strategy
Data
To analyze the proportion of workers with jobs having a high automation probability, we used the national GEIH representative survey to analyze the labor market in Colombia, which includes socioeconomic, labor, and company variables. The survey has a cross-sectional data structure and has classified occupation-specific questions to assign the automation probability. We focus on the employed population, as these are the workers at risk of having their occupation automated before technological disruption.
For assigning the probability, we matched the Standard Occupational Classification (SOC) 2010 used by Frey and Osborne at six digits with the Colombian International Standard Classification of Occupations (ISCO) 1968 at five digits (large groups at one digit, subgroups at two digits and primary groups at five digits). However, the occupations assignments used by dane for geih is at two digits (subgroups), the occupations assignments used by dane for geih are at two digits (subgroups), and as the correspondence between the two classification systems is not exact, we estimated the probability from a simple average for those occupations that do not have a unitary correspondence, following the method by Minian and Martinez (2018). An example is shown in Table A1 of the appendix. We assigned the 83 types of occupations in the geih the automation probability of Frey and Osborne (2017). Table 1 shows the descriptive statistics of the variables.
Table 1. Descriptive statistics
Note: This table shows the mean of each variable over the total observations.
Source: Own elaboration, GEIH.
We worked with 183,228 observations, with an average age of 39 years old, of whom 47% are women. Regarding the labor market results, more than 50% of workers are in the private sector, 37% are self-employed, 51% are informal workers, 26% have a fixed-term contract, while only 3% belong to a union association. The predominant economic activities are wholesale and retail trade, with 23% of participants, followed by manufacturing industries and real estate activities with 14% and 10%, respectively; other economic activities represent the remaining 53%. Thirty-seven percent of the workers have a high school education, and 35% have a university or higher education; the remaining 28% have secondary or primary education or no education at all.
Empirical strategy
We used the Generalized Linear Model (GLM) developed by Papke and Wooldridge (1996) to estimate the probability of job automation from the projections provided by Frey and Osborne (2017) and to analyze the influencing factors affecting this probability.
Papke and Wooldridge (1996) specified a set of functional forms for E(x) to obtain parameter estimates using Bernoulli quasi-likelihood methods, eliminating the need for ad hoc transformations to handle data at the extreme values of zero and one in the automation probability. This assumes the availability of a sequence of independent observations: [(x i ,y): i = 1,2,…,N], where 0 < y i < 1 and N represents the sample size.
The asymptotic analysis is conducted as N→∞; the assumption we operate under is that for all i: E (x i ) = G (x i β), where G(.) is a known function satisfying 0 <G (z)< 1 for all z ϵ R. This ensures the predicted values of y lie within the interval (0,1).
We estimated the equation:
In this equation, the dependent variable yi represents the automation probability of the occupation for worker i, and the independent variables Xi, Ti,... Si, correspond to sex, educational level, attendance at job training courses, age, occupational status, contract, seniority, economic activity, informal work and membership in a trade union association. All these variables are distributed into categories based on the literature review carried out.
Results
Probability and risk of automation
Utilizing the GLM method, we were able to predict the automation probability according to the Colombian labor market. As shown in Table 2, 37% of the total workforce occupies roles with a high risk of automation, equating to 68,455 jobs. These results differ with the automation probability figures for Colombian occupations directly assigned probabilities provided by Frey and Osborne (2017), which indicated that 55% of employees in Colombia faced a high risk of substitution by new technologies. Occupations categorized with a medium risk of automation exhibited the highest participation rate, encompassing 55% of the total workforce, or 101,549 workers. Following this, roles with low risk constituted 7% of the workforce, involving 13,224 workers.
Table 2 Occupational automation risk
Assigned probability Frey and Osborne (2017) | Predicted probability GLM | |||
---|---|---|---|---|
(1) | (2) | (3) | (4) | |
Automation Risk | Employees | % | Employees | % |
Low | 41.635 | 22,72 | 13.224 | 7,22 |
Medium | 41.454 | 22,62 | 101.549 | 55,42 |
High | 100.139 | 54,65 | 68.455 | 37,36 |
Total | 183.228 | 100 | 183.228 | 100 |
Note: This table illustrates the distribution of workers based on high, low, and medium probabilities of automation. Column (1) presents the total number of workers categorized by automation risk according to the work of the Frey and Osborne (2017) along with the corresponding percentage in column (2). Column (3) shows the total workers categorized by automation risk estimated using the proposed method, along with the corresponding percentage in column (4).
Source: Own elaboration, GEIH, Frey and Osborne (2017).
In Figure 1, a comparison of this probability density is presented. As observed, the probabilities assigned by Frey and Osborne (2017), without considering the specific characteristics of Colombian employees, tend to concentrate in the high automation risk level. However, a different scenario emerges when estimating this probability, as the highest concentration is in the medium risk of automation. This shift in the probability density implies that, considering the labor market characteristics, the majority of employees are not at high risk in the short term but rather in the medium term. A significant portion of employees operates in the commercial sector, which might resulting a slower adoption technology compared to the manufacturing sector, thus delaying the impacts of technological disruption.
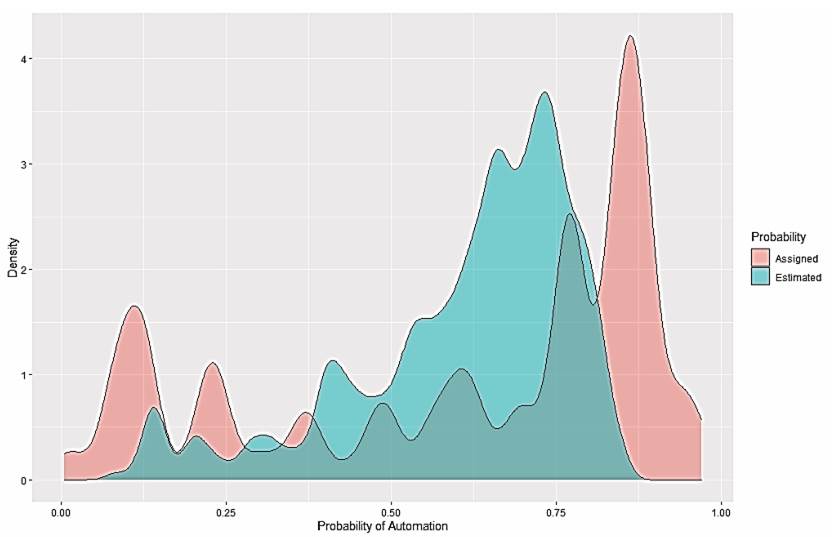
Source: Own elaboration, GEIH, Frey and Osborne (2017).
Figure 1. Occupational automation risk densities Note: This figure shows the density of automation probability for workers' occupations in Colombia, directly assigned with the probabilities calculated by Frey and Osborne (2017) in pink, alongside the density of automation probability for occupations estimated using the proposed methodology in green.
Table 3 Results of occupational status, educational level and economic activity
Note: This table illustrates the marginal effects of the average level for each occupational for each occupational status category in relation to the base categories (private sector employees for educational status, (base category: no educational level) and economic activity (base category: Agriculture).
Source: Own elaboration, GEIH, Frey and Osborne (2017).
Factors influencing the likelihood of automation
In Table 4, column (1) displays the results of the GLM estimation, while column (2) presents the marginal effects of the average characteristics of the observations. These effects indicate the changes in the automation probability resulting from alterations in the personal, labor, and firm characteristics of Colombian workers.
Table 4. Results of occupational status and educational level
Note: This table presents the marginal effects of the average level for each age category in relation to the base category (under 20) and seniority (base category: less than 6 months' experience).
Source: Own elaboration, GEIH, Frey and Osborne (2017).
It is observed that the likelihood of a domestic employee being replaced by a machine is 52 points lower, ceteris paribus, than that of an employee in the private sector. Similarly, employers have a 24 points lower automation probability, ceteris paribus, than this same base category. That aligns with expectations since domestic employees perform handling and care tasks that require a certain level of creativity (non-routine manual task), making them less susceptible to automation. In contrast, employers, with their responsibilities and dependents (non-routine cognitive tasks), are considered to have a low risk of automation.
Regarding workers’ personal characteristics, having a university education or higher corresponds to a 15-point lower automation probability than the base category (no educational level). Conversely, lower levels of education, such as high school, preschool, and elementary school, exhibit a significant but comparatively low impact on reducing the automation probability. These results were anticipated, given that higher educational levels enable the acquisition of skills and abilities that are difficult to replace with technology.
In terms of the sector of employment, workers in the education sector (teachers, tutors, instructors) have a 38-point lower automation probability, ceteris paribus, than those in the agricultural sector, followed by the social services and health sector (27 points lower), mining and quarrying (21 points lower), and other service activities (19 points lower). As expected, working in the service sector has a significant impact on the automation probability, as these activities require social intelligence to perform. The opposite is true for the primary sector, which has a high automation probability.
Those belonging to the formal sector (contributing to pension and health contributions) have a probability 2.2 points lower than those working in the informal sector. Another interesting result is the difference in gender; female workers have an automation probability 1.2 points higher than of male workers. These results indicates that many activities engaged in by women are manual and routine, leading to greater automation effects as seen in Table 5.
Among other notable findings, it was observed that having 10 to 14 years of experience in the same job results in an automation probability 3 points lower than the base category (less than 6 months of seniority). Additionally, attending job training courses decreases the automation probability by 2.9 points compared to those who do not attend. Moreover, being a member of a union association can decrease the risk of automation by 2.5 points compared to those who are not members. The latter is an expected result, highlighting the effect of labor organizational cohesion in Colombia on the decision makers process among political leaders, specifically in negotiating employer-employee relationships, which can reduce the automation probability of occupations.
Analysis of results
The revised task model developed in section II helps to explain our results. The tasks less susceptible to automation found above characterize by possessing one or more of the three challenges to be overcome by the technologies development to automate almost any task, especially those tasks that have high levels of perception, manipulation, creative intelligence, and social intelligence tasks such us domestic employees, employers, workers with high levels of education, workers in the service sector, including education, health, community activities, and social services, among others.
We have identified factors beyond the scope of the economic model and technological predictions that can mitigate workers’ vulnerability to technological disruptions. Government policies promoting formal employment, enhancing educational levels, facilitating attendance at job training courses, implementing gender equity policies, and ensuring workers’ protection through unions are examples of actions that can safeguard workers’ well-being in the face of potential unemployment workers due to technological advancements.
Conclusions
Nowadays, trends of job destruction and transformation have accelerated, driven by rapid technological advances. Studying the impact of digitization on the labor market is not merely a contemporary concern. Given the swift pace of the current industrial revolution, there is a pressing need for new models that predict the risk of various occupations becoming automate. These models should acknowledge that routine tasks, whether manual or cognitive, can be substituted by existing technology (Autor et al., 2003), even those non-routine cognitive or manual tasks that susceptible to substitution due to the convergence of technological developments (Frey and Osborne, 2017).
The disappearance of jobs increases, affecting all fields and countries, at least partially. Empirical analyses underscore the disruptive influence of technology on employment, contingent upon each country’s labor characteristics, especially the timing of technology integration into the economic system and the existing technological gaps. Given this scenario, characterizing the problem becomes crucial, especially in countries like Colombia, where challenges combined with the pre-existing difficulties in the labor market (unemployment, informality, low-skilled labor, among others).
This study marks the first step to determine the proportion of occupations with high, medium, and low automation risk in the Colombian labor market. It delves into the socioeconomic factors and worker characteristics linked to these risk, addressing an analytical gap. This analysis contributes to understanding labor dynamics amid technological advancements in various countries globally. Furthermore, this study can serve as a foundation in programs of national impact and inform public policy decisions in the labor market.
The findings reveal that 37% of occupations in Colombia face a high risk of automation. Workers’ educational levels and experience play pivotal roles seniority are personal characteristics of workers that can contribute to lowering the probability of job automation. Labor characteristics such as attendance of courses and training, economic sector, union association and formal work can be significantly in mitigate the adverse impacts of technological progress on employment.
Public policies should discuss a greater extension, quality, and promotion of education together with developing specialized skills such as creativity, communication, and negotiation, among others. Moreover, labor policies, with a gender differential approach should be strengthened. The study highlights that the occupations with the highest risk of automation are those performed by women, which could widen the employment gap between men and women. It is important to emphasize Policy makers, job stability, and even unemployment insurance for those occupations at high risk of automation.
In this sense, it is imperative for all economic, political, and social agents to collaborate in guiding this change. A harmonious transition, devoid of significant major disruptions to societal disruptions, requires concerted efforts. These issues need to be incorporated into academic, political, economic, and social discussions, with particular attention to educational policies in this uncertainty landscape.
Discussion
To broaden the forward-looking analysis of employment automation in the country, updating existing information is crucial. This includes business surveys incorporating questions related to technological adoption. It is noteworthy that DANÉS method of classifying occupations in the GEIH until 2019 is based on ISCO in 1968, a classification that has become outdated. Many occupations have evolved or disappeared over 50 years. Additionally, the limited disaggregation level of the nomenclature hampers capturing the transformations in the labors market’s occupational landscape.
Conducting a comparative analysis of employment automation in Colombia, suggest various reference years, would be valuable. This would help discern real changes in the dynamics and composition of the probability and risk of employment automation, following the method proposed here. Such analyses could also consider the technological adoption lag index published by the World Bank.
In terms of the validity of the task model presented, recent studies suggest basing the analysis on production functions with both increasing and decreasing returns to scale, resulting in more robust models. There is a large break from considering a production function with exogenous technological change and perfect substitutability between the task content of each occupation is essential. Models proposed by Acemoglu and Restrepo (2018 and 2019) advocate working with models that include dynamic equilibria and endogenous technological change. This involves considering within-firm innovation, future technological advancements, and dynamic changes in employment composition solely due to technology factors.