INTRODUCTION
The theory of multiple intelligences has allowed us to understand and explain the ways of thinking and solving problems, with different modes of reasoning that people have. One of the main questions that arise is how do multiple intelligences affect financial decision-making? This question is especially relevant where the social systems with which people interact have increased in complexity. High levels of poverty imply less latitude to make financial mistakes on the part of the individual and even cause the loss of purchasing power for consumers.
The theory of multiple intelligences (Gardner, 2008; Ouchen, 2019) has allowed us to delve into the ways in which people solve problems in different contexts (Barrero et al. 2021). Eight have been identified thus far (Shearer, 2020): logical-mathematical, linguistic-verbal, bodily-kinesthetic, visualspatial, musical, intra and interpersonal, and naturalistic. These intelligences allow us to see forms of personal relationships and social exchange. Multiple intelligences have been defined as the adaptive way people solve problems, showing substantial proficiencies in specific areas and that can be placed among neuropsychological developments (Gardner, 2008).
Intrapersonal intelligence refers “(...) to how important it is to have a viable model of oneself and to be able to draw on it when making decisions about one’s personal life” (Gardner, 2008, p.9). From the above it can be inferred that within the decisions of personal life, those regarding purchasing that are made within the financial and economic environment are immersed.
Analogously and according to Gardner (2008) “Interpersonal intelligence” refers to the infant’s capacity to distinguish between the people in his immediate environment and to identify his various emotional states. When it shows the most advanced form, the possibility to develop interpersonal knowing shows to the adult the intentions and desires of people, even if they have been concealed, and, potentially, to act on this knowledge, such as persuading a group of dissimilar people to behave in accordance with a desired standard.
Interpersonal intelligence is the individual’s interpretation of his environment, which can influence the way in which consumption decisions are made and the satisfaction of personal desires. Through this same logic Davis, Christodoulou, Seider and Gardner (2008, p.488) maintained that logical-mathematical intelligence “is an ability to develop equations and proofs, make calculations, and solve abstract problems”. That is linked to the need to establish the relationship between money and the numerical.
Another crucial component in financial decision-making is financial education, defined in OECD (2005) as the method by which monetary buyers / financial specialists make strides their understanding of money related items and ideas and, through data, instruction and/or objective counsel, create the abilities and certainty to become more mindful of monetary dangers and openings, to form educated choices, to know where to go for offer assistance, and to require other compelling activities to progress their budgetary well-being.
The intrapersonal intelligence, explains the interaction of a person with himself and his emotions, leading to mediation in the decision-making process about what is done or not at the expense level (Polo-Vargas, Zambrano-Curcio, Muñoz-Alvis and Velilla-Guardela, 2016).
A notable example from the incorporation of latent constructs in the analysis of financial behavior is the way in which agents make their inter-temporal financial decisions (Kahneman, 2012). Mental accounting is a model that proposes that consumers have decision-making processes in a similar way to business. Consumers and households use an underlying latent structure and cognitive processes to assign financial events to specific accounts and classify them by considering their origin as income or their destination as expenses (Vidal de la Rosa, 2008).
Overall, the research program in behavioral economics, which builds on the incorporation of latent unobservable phenomena in the decision-making process, has seen repeated success and value in explaining novel explanations of consumer behavior, as well as standing empirical tests superior to classical consumer theory, such as the case of prospect theory (Ruggieri, Alí, Berge et al., 2020). This origin of the spirit of incorporation of latent and not substantially rational constructs in analyzing behavior was better summarized by Akerlof and Shiller (2009) as follows:
[The individual’s decisions] “You can only make them through your animal spirits, which are the result of a spontaneous drive towards action. They are not, as established by rational economic theory, the result of the weighted average of the quantitative benefits multiplied by their probabilities. In the initial use of the term spiritus animalis is its ancient form of medieval Latin; the word animal meant “of the mind” or “that animates” and referred to the primordial mental energy and life force. However, in the modern economy, animal spirits have taken on a different meaning and is currently an economic term that refers to the fluctuating and unconscious component of the economy. It represents our peculiar relationship with ambiguity or lack of certainty. Sometimes animal spirits paralyze us, while at other times they revitalize us and fill us with energy, causing us to overcome our fears and insecurities (Akelrof and Shiller, 2009, p.21).
We hold then that incorporating other latent constructs from psychology, such as types of intelligence, can contribute to improve analyses of financial decision-making and, also, to how these are related to the material conditions of the economic decision-maker. To our knowledge, no author has incorporated and analyzed the role of different types of intelligence on consumer or financial decision-making, because most models of behavior assume only differences in rationality or strategic thinking, as with K-level models in behavioral economics (Dhami, 2016).
One of the most widespread decisions is the acquisition of credit cards and their use. In this regard, the demand for credit is related to variables such as labor status (since it increases for formal employees) the region, confidence in the financial sector, gender and age (Arrow, 1959).
Although the relationship between various socioeconomic factors and card acquisition has been studied in the literature, however, to the authors’ knowledge, there is still no approximation to the influence that multiple intelligences could have on this decision of financial consumption.
Thus, our objective with this study is to provide a twofold contribution, one to the behavioral economic literature by exploring the role of an under-researched set of latent constructs in financial behavior, and the other an addition to the consumer behavior literature by exploring a new set of possible causes and moderators for observed financial product behavior.
To do this, we will provide the theoretical framework from both economics and psychology, which leads us to understand their possible relationship. Likewise, various instruments will be used to corroborate the central hypothesis of the document. Finally, the requisite statistical study is carried out to conclude from the evidence found whether this type of relationship exists. Considering the above, we asked income managers about the decisions they would make in the face of investment problems and, at the same time, applied the multiple intelligences questionnaire to them.
This paper is divided into three parts. In the first, reference is made to relevant research into multiple intelligences and financial thinking. Second, this will be shown by models and their results with a corresponding discussion. Finally, the conclusions are presented.
Multiple intelligences and financial thinking
One of these fundamental exchange relationships is one that refers to financial transactions, because it is one of the bases of social relations and status in a world based on current market economies (Schmidt, R. & Hryckiewicz, A. 2006). All the above relates to ways in which what is called financial intelligence is understood and used, and which are part of the so-called personal intelligences (inter and intra).
Therefore, these phenomena are linked with financial and purchasing decisions, because people and social groups have needs, interests, and desires that they try to satisfy. In the midst of this, money must be prioritized according to the way in which a person processes the information received (García-Sánchez, W., Rodríguez-Bailón, Palacio-Sañudo, Polo & Renteria- Pérez, 2018; Leonard, Zhang & Howell, 2019). Then, the type of intelligence determines the relationship of what is prioritized and what remains of uncertainty from the decision taken or the risk involved.
It can be established that to manage financial resources, a set of competencies is necessary. In the same sense, economic intelligence can be understood as a continuous and permanent planning process, as well as the acquisition of relevant information, analyzing it to transform it into knowledge and intelligence, which supports strategic decision-making to achieve financial objectives (Hoffmann & Post, 2014; Rodríguez, 2015). This is ultimately reflected at the level of states, companies, families, and individuals. Accordingly, it is important to note that financial wellbeing or the financial situation are the reflection of the level of financial education of each person, which affects progress and social indicators, economic growth, and indicators of the development of a country’s financial system (Fernández, 2008; Vallejo et al, 2016).
Therefore, informal loans are being privileged in sectors where people do not have the means, in an agile way, to access capital, as illustrated in a study of demand for financial inclusion by the Bank of Opportunities, as shown in Table 1.
Table 1 Distribution of financial services according to income level
Income level | Formal financial service | Non-financial formal service | Informal | No access |
---|---|---|---|---|
Less than $121,98 USD | 46,8% | 12,9% | 11,3% | 29% |
Between $121,98 and $243,96 USD | 47,4% | 13,3% | 16,2% | 23,1% |
Between $243,96 and $406,59 USD | 71,4% | 7,1% | 6,7% | 14,8% |
Between $406,59 and $847,08 USD | 77,9% | 6,6% | 4,1% | 11,4% |
More than $847,08 USD | 94,9% | 0,8% | 1,2% | 3,1% |
Don't know / No Response | 71,8% | 6,9% | 5,1% | 16,2% |
Source: Authors based on Banking of Opportunities Financial Superintendence (2017). The average dollar amount for 2017 was $2.951,32, according to data from the Banco de la República.
Table 1 indicates that people who do not know their finances access loans from formal banks. Also from the table 1, it is striking that for 2017, people with less than minimum income accessed the formal financial sector at a 48,4 % rate, and at 12,9 % the non-formal financial sector. This implies that a combination of ignorance of their own finances, combined with having belowminimum income, becomes a condition detrimental to the improvement of people’s quality of life (Pinger, 2017).
These data are complemented by the indicator of schooling, relevant to the present study, as shown in Table 2.
Table 2 Financial access by levels of schooling
EDUCATION LEVEL | FORMAL FINANCIAL SERVICE | NON-FINANCIAL FORMAL SERVICE | INFORMAL | NO ACCESS |
---|---|---|---|---|
Primary or secondary Incomplete | 50,6 % | 11,6 % | 8,1 % | 29,7 % |
Completed secondary | 66,1 % | 9,6 % | 10,7 % | 13,6 % |
Technological University | 77,9 % | 0,06 | 6,7 % | 9,4 % |
Source: Authors, based on Banking of Opportunities Financial Superintendence (2017)
From Table 2, financial decision-making had a preponderance in this study, which in turn is usually related to intelligence. This aspect raises the issue of uncertainty toward the results of said resolutions, so we argue that people do not use strictly rational criteria when managing their finances and that affections play a predominant role in decision-making of this nature (Aspara, Chakravarti & Hoffmann, 2015; Kahneman, 2003; Weiss, 2016). Therefore, the randomness of an event and the emotions that accompany it regarding risk perception itself lead an individual to refuse losses, as can be inferred by Table 2.
Based on the literary review made for this study, we propose the following hypotheses:
There is a statistically significant relationship between some types of intelligence and financial literacy.
There is a statistically significant relationship between some types of intelligence and risk preferences.
Personal intelligences influence the acquisition of credit cards, and this relationship is mediated by time preferences and financial literacy.
METHOD
The present study is framed in the quantitative named of explanatory nature, among which the correlational ones were taken (Hernández-Sampieri, Fernández-Collado and Baptista-Lucio, 2014). Here, we sought to establish covariance between one or more variables. This was done to examine the potential causal nodes that could be proposed and to establish non-spurious relationship options.
It is hypothesized that multiple intelligences, particularly personal and logical-mathematical, influence the way in which the problem of managing one’s own finances is faced and, by implication, decision-making when long-term planning (García-Sánchez, Willis, Rodríguez-Bailón, García-Castro, Palacio-Sañudo, Polo-Vargas and Renteria-Pérez, 2018). In the above sense, the uncertainty, randomness of events, social exchanges, and perceptions that people have are interpreted from each of these intelligences. Additionally, they condition the behaviors and relationships established from there. In our study, we subdivided the general above hypothesis into four statistical hypotheses:
H1: People with a higher level of intrapersonal intelligence are less likely to present risky financial behaviors such as over-indebtedness, purchases of basic necessities on credit, and debts.
H2: People with a higher level of interpersonal intelligence are less likely to present risky financial behaviors such as over-indebtedness, purchases of essential goods on credit, and debts.
H3: People with a higher level of logical mathematical intelligence are less likely to present risky financial behaviors such as over-indebtedness, purchases of essential goods on credit, and debts.
H4: The interactions described by hypotheses H1, H2, and H3 are modulated by attitudes about the patience of the participants.
H5: The interactions described by hypotheses H1, H2 and H3 are modulated by the participants’ attitude toward risk.
H6: The interactions described by hypotheses H1, H2, and H3 are modulated by the participants’ financial literacy.
Instruments
The questionnaires were applied between February and May 2019. They were applied online, requesting support in various public places such as educational centers and businesses. People had their response time measured in answering the financial questionnaires. The scales were of the Likert type, with 5 to 10 response options.
The multiple intelligences questionnaire (Shearer, 1995) measured indices of the various intelligences: logical-mathematical, linguistic-verbal, bodily-kinesthetic, visual-spatial, musical, intra and interpersonal, and naturalistic. The scale had 119 items, among which were “Are you skilled with your hands in activities such as shuffling cards (or decks), doing magic tricks, or being a juggler”? “Do you get very angry when you fail or are frustrated”? Responses ranged from 1 Strongly Disagree to 5 Strongly Agree. The average Cronbach’s alpha of the intelligence scales of our study was 0,84 (minimum 0,75 and maximum 0,89). In the questionnaire there was also the option to answer “I don’t know”.
The second questionnaire was the module including measurements of risk preferences and times (Becker, Armin & Dohmen, 2016). Risk preferences were measured using an evaluation scale, for example “How willing or unwilling are you to take risks”? While time preferences were measured using both evaluation scales “How willing are you to give up something that is beneficial for you today in order to benefit more from it in the future”? and hypothetical choices between two separate alternatives in time of the style “Would you prefer to receive 100,000 pesos today or 125,000 pesos in 12 months?” 1 Not at all willing to take risk, and 10 Totally willing to risk.
After this, the participants answered questions intended to measure their level of financial literacy (Klapper, Lusardi and Van Oudheusden, 2015). This measurement included components related to understanding of the concepts of: Risk diversification (e.g., “Suppose you have some money. Is it safer to put your money into one business or investment or into multiple businesses or investments”?), inflation, “numeracy”, or interest and compound interest.
The survey ends with a personal questionnaire that included socioeconomic information and questions related to the possession and use of credit cards, savings, and purchases.
Participants
For this study, 600 people were invited, of which 498 (83 %) of valid surveys were applied virtually to people who managed income. Of the participants, 85,14 % were between 21 and 45 years old, and most lived in homes classified in socioeconomic strata 3 and 42. A detailed description is below.
Table 3 Socioeconomic strata
STRATUM* | PARTICIPATION IN SURVEY (%) |
---|---|
1 | 6,63 |
2 | 9,64 |
3 | 23,69 |
4 | 23,69 |
5 | 18,67 |
6 | 17,67 |
No stratum | 0 |
Total | 100 |
Survey participants had the occupations listed in Table 4.
Table 4 Occupational data
Occupation | Freq. | Percent |
---|---|---|
Unemployed | 40 | 8,03 |
Employed | 124 | 24, 0 |
Student worker | Four. Five | 9,04 |
Student | 239 | 47,99 |
Independent | 38 | 7,63 |
Retired | 12 | 2,41 |
Total | 498 | 100,00 |
As seen from Table 4, most of the participants (47,99 %) were students, a sector that is beginning to have greater independence in the management of resources. This is in accord with theories of developmental psychology that propose this stage as central when generating independence. On this surveyed group, 50,6 % had credit cards, of which 49,18 % reported that they had them for more than two years, 22,35 % for 1-2 years, and 15,04 % for more than six months but less than one year. Given the characteristics of the survey, 78,31 % had no dependents.
RESULTS
Multiple intelligences
We did a confirmatory factor analysis for each of the multiple intelligences. This allowed us to generate relative scores for each of the participants compared to the total sample. Then, we categorized the prevalence of a certain intelligence, using a standard deviation score above the mean as the inclusion criterion.
Our ranking indicates that 67 % of participants stood out in at least one intelligence. The percentage of participants with a prevalence in each of the intelligences varied between 15 % and 17 %. There were 17,47 %, 17,27 % and 15,86 % of the participants manifesting a high level of the logical-mathematical, interpersonal and intrapersonal intelligences, respectively.
Financial literacy
The results of the financial literacy module indicate poor management of financial concepts. For example, the last three questions of the questionnaire were about compound interest and had correct answers for the items in the order 62,65 %, 51 % and 41,97 %.
Questions about Credit Cards
In the survey, the first data that draw attention concern the report made by spending on basic goods (market, rent, and others) at 63,24 %, which is greater than 30 % and savings at 50,6 %. The most frequent amounts saved were 20 % and 10 %, with frequencies 6,83 % and 6,22 %, respectively. Now, 50 % declared that they did not have a credit card, with 49,40 % having the cards over a period between three and six months. It is striking that 50,8 % declared that they bought on credit goods other than those of the family basket, and 36 % reported having unpaid credits.
Model
We decided to use a Structural Equation Modeling (SEM) approach to the data, because the available dataset includes both observed and indicator variables, and because we were interested in direct and indirect relationships between variables (Byrne, 2001). We describe the approach in the following section.
Measurement model
We performed three separate confirmatory factor analyses (CFA) for each of the intelligences of interest (logical-mathematical 16 indicators, interpersonal 17 indicators, and intrapersonal 9 indicators). Our estimation method for each CFA was principal factors, using a polychoric correlation matrix between indicator variables. After a varimax rotation, we retained factors of eigenvalues > 1 and predicted factorial scores for each of the intelligences. For all intelligences, only one factor was retained.
Explained variance for the predictor factor in each of the intelligences ranged from 92 % to 100 % (both intrapersonal and logical-mathematical intelligences factorial structures were Heywood cases because of abnormal commonalities).
Structural Model
Aside from the previously discussed latent variables, our proposed structural model contains the following observed variables, grouped into three categories:
Economic Preferences
Evaluated patience: Likert Scale from 1 to 10, measuring willingness to forego current rewards to receive greater rewards in the future.
Risk preferences: Likert Scale from 1 to 10, measuring willingness to take risks.
Behavioral Measures
Bad shopping: Dichotomous variable, measures if person has recently made purchases of first-need products with credit cards or other credit.
% spent in CC: Percent of income dedicated to pay Credit Card debts.
Has debts: Dichotomous variable, measures if person currently has active debts.
Financial Literacy
Financial literacy count: Number of correct answers to the six financial literacy questions included in the questionnaire; range 0 to 6.
We also included age, socioeconomic level and income bracket3 as robustness checks. However, estimation results were not significantly modified and model fit was substantially worse (see annex).
We also decided to estimate a generalized SEM model (gSEM) to address the non-normality and discreteness of response data, but it did not change the main results (see annex for three tables). We next present a graphical representation of the model.
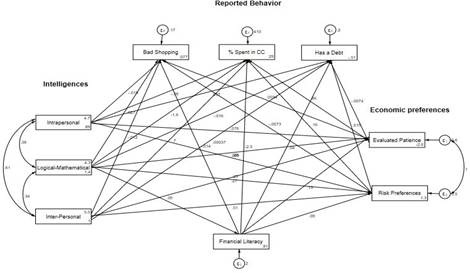
Figure 1.Structural relationship diagram. Coefficients are based on the initial linear SEM. Covariance structure between exogenous variables and between errors was included in the model with robustness checks, but not in the gSEM, owing to exogeneity constraints imposed by the model.
Table 5 shows the estimation results from the SEM diagram presented in Figure 1. It shows six simultaneous estimation equations and implied covariance structure. The estimation results for the previous structural model are presented next
Table 5 Distribution of financial services according to income level
(1) | (2) | (3) | (4) | (5) | (6) | (7) | ||||
VARIABLES | Evaluated Patience | Risk Preferences | %Spent in cc | Has Debst | Bad Shopping | Financial Literacy | Covariances | |||
a. Evaluated Patience | 0.0095** | -0,0039 | 0,0056 | |||||||
(0,0039) | (0,0091) | -0,0082 | ||||||||
b. Risk Preferences | 0,0017 | -0,0021 | -0,018 | |||||||
0,0050 | (0,011) | -0,01 | ||||||||
c. Financial Literacy | 0,15** | 0,090 | -0,024*** | 0,042*** | -0,012 | |||||
(0,074) | (0,059) | (0,0064) | (0,015) | -0,013 | ||||||
d. Factor Scores for Interpersonal Intelligence | 0.076 | 0,28** | -0,0037 | 0,084*** | -0,002 | |||||
(0,15) | (0,12) | (0,013) | (0,030) | -0,027 | ||||||
e. Factor Scores for Logical-Mathematical Intelligence | 0,063 | 0,25*** | -0,018** | -0,039** | 0,013 | 0,39*** | ||||
(0,099) | (0,079) | (0,0086) | (0,020) | -0,018 | (0,054) | |||||
f. Factor Scores for Interpersonal Intelligence | 0,27** | 0,51*** | 0,0082 | 0,018 | -0,0011 | |||||
(0,14) | (0,11) | (0,012) | (0,028) | -0,025 | ||||||
Constant | -2,56*** | 1,31** | 0,33*** | -0,073 | 0,35** | 1,09*** | ||||
(0,66) | (0,52) | (0,058) | (0,13) | -0,12 | (0,24) | |||||
cov(e,a,e,b) | 1,04*** | |||||||||
(0,21) | ||||||||||
cov(d,e) | 0,38*** | |||||||||
(0,053) | ||||||||||
cov(d,f) | 0,61*** | |||||||||
(0,051) | ||||||||||
cov(e,f) | 0,34*** | |||||||||
(0,056) | ||||||||||
(0,056) | ||||||||||
Observations | 498 | 498 | 498 | 498 | 498 | 498 | 498 | |||
Stadard errors in parentheses ***p<0,01 **p<0,05, *p<0,1 |
Regarding the hypothesis that people with a higher level of intrapersonal intelligence have a lower probability of presenting risky financial behaviors such as over-indebtedness, purchases of essential goods on credit, and debts, we found that intrapersonal intelligence is associated with an increased likelihood of having debts, i.e., an increase of 0,084 standard deviations.
Now, about whether people with a higher level of interpersonal intelligence are less likely to present risky financial behaviors such as over-indebtedness, purchases of essential goods on credit, and debts, the model showed that indirect effects on behavior are weaker. Mainly, the effect of evaluated patience for credit card payments implies a small indirect effect of interpersonal intelligence on the share paid in credit cards (indirect effect = 0,002). Following this, the impact of risk on “bad” shopping behavior implies an indirect effect of all three intelligences on this behavior; indirect effects were −0,009, −0,005, and −0,004 for inter, intra and logical-mathematical intelligences, respectively.
In reference to whether people with a higher level of logical-mathematical intelligence are less likely to present risky financial behaviors such as over-indebtedness, purchases of essential goods on credit, and debts, it was shown that a higher score for such intelligence is associated with a lower likelihood of having debts (𝛃 = −0,018) and a smaller portion of income dedicated to paying credit card debts (𝛃 = −0,039).
The structure of the model allows also for the calculation of indirect effects on behavior. The model suggests that for logical-mathematical intelligence, there is an indirect effect on the percentage that participants dedicated to pay credit cards, which further the increase decreases later in the magnitude of this effect by nearly 30 % (total effect = −0,027), as well as having a suppression effect via financial literacy on the possibility of having debts, which reduces the total effect to −0,022.
Important results about the interactions described by the hypotheses H1, H2 and H3 were modulated by attitudes about the patience of the participants, leading to the finding that for the other two intelligences, indirect effects on behavior were weaker. Mainly, the effect of evaluated patience on credit card payments implies a small indirect effect of interpersonal intelligence on the share paid in credit cards (indirect effect = 0,002). Following this, the impact of risk on bad shopping behavior implies an indirect effect of all three intelligences on this behavior; indirect effects were −0,009, −0,005, and −0,004 for inter, intra and logical-mathematical intelligences, respectively.
In addition to the first interactions, when the interactions were analyzed by the hypotheses H1, H2 and H3, they were modulated by attitudes towards risk of the participants. We found that an increased score in all three intelligences was associated with a greater willingness to take risks; an SD increase in the logical-mathematical, intrapersonal and interpersonal intelligences was associated with increases of 0,25, 0,28 and 0,51 standard deviations on the risk scale. Patient-reported behavior was mostly positively associated with financial literacy and interpersonal intelligence (𝛃 = 0,15 and 0,27, respectively). Financial literacy was also predicted by interpersonal intelligence.
Finally, the interactions described by hypotheses H1, H2, and H3 were modulated by the participants’ financial literacy. The effect of these moderator variables on observed behavior is more widespread when compared with the direct effect of the intelligences. Financial literacy was associated both with a smaller share of income dedicated to paying credit card debts (𝛃 = −0,024) and a greater likelihood of having debts. Risk behavior predicted a reduced likelihood of making bad purchases, although only weakly (𝛃 = −0,018). Participants with a higher score on the patience scale also showed a tendency to dedicate more of their income to pay credit card debts (𝛃 = 0,095).
DISCUSSION
We begin by highlighting that a novelty of the work is that, having reviewed the WoS, SCOPUS, EBSCO, PROQUEST and Google Scholar databases, no research was found that related our variables. In this order of ideas, we argue that the value of personal intelligences when working on financial education has been overlooked.
From our study, people with greater intrapersonal intelligence tend to have a greater probability of contracting debts. However, they dedicate less of their income to the payment of these obligations. This is in accord with theories that suggest that with greater introspection, determinations are made with a higher level of acceptance (Kahneman, 2012; Polo-Vargas, Zambrano-Curcio, Muñoz-Alvis and Velilla-Guardela, 2016). This reinforces the hypothesis that an efficient financial education must start from self-knowledge.
In this sense, people with lower levels of intrapersonal intelligence could perceive the benefits of using the credit card, associating it with results in terms of pleasure and taste. There is no internalization, at least in the first instance, of the influence of interest rates on the purchase, or of the deadlines, ignoring the elements of a more informed decision (Kahneman, 2012; Polo-Vargas, Zambrano-Curcio, Muñoz-Alvis and Velilla-Guardela, 2016).
In the same sense, people with higher scores of interpersonal intelligence can better recognize the probabilities of risk. This allows us to argue that the greater such intelligence, the greater the evaluated patience and a better understanding of the risk. This suggests that people who are able to understand the environment, read the emotions of others, and identify social trends can better adjust the risk taken (Leonard, Zhang & Howell, 2019).
Delving into the previous logic, our results support that people with greater interpersonal intelligence will tend to avoid financial behavior with substantial uncertainty. Also, they tend to have more patience, which leads to a delay of reward or consumption needs. This could decrease the rates of both impulsive and compulsive buying.
One of the areas that are usually associated with financial and economic processes is mathematical logic, owing to the numerical reasoning that is carried out. This can be seen in the model, in which financial intelligence is influenced by mathematical logic (Skagerlund et al., 2018) in such a way that it permeates the decision to acquire a credit card in this process. The results allow us to assert that people with large indexes of logical-mathematical intelligence usually avoid debts.
This implies from developmental psychology that the foundations of both logical and mathematical reasoning must be improved in financial education programs, instead of performing calculations or memorizing formulas; it is about potentiating higher cognitive processes. Consequently, the processes associated with deductive logic are essential to lay the foundation for more complex analysis of credit card use.
Another contribution to consider is about the finding of a positive relationship of covariance between logical-mathematical and interpersonal intelligence seems to contradict the myth -socially accepted- that people with high levels of numerical reasoning have difficulties in socializing. Now, this connotes that reasoning about cost benefits -which is usually numerical- is related to the interaction of people, an argument that leads us to strengthen interaction with others at the same time as mathematical reasoning.
On the other hand, based on the results, a new level of analysis is supported, which points to a higher-level construct that modulates the relationship between preferences and observed behavior (in our case, intelligence) that allows causal relationships to become more complex and has implications when choosing a product. The foregoing implies that it is necessary to condition education processes to the types of intelligence, favoring those that allow open interaction with the household spending and consumption system.
Another important implication of our research is that financial education does indeed play a role in both modulation of the effect of higher-level constructs on financial behavior and of economic preferences. This implies that financial education initiatives might have greater value in behavior modification, since it might very well affect economic behavior through both direct and indirect ways.
LIMITATIONS OF OUR RESEARCH ARE AS FOLLOWS
When dealing with a significant number of students, it is possible that these types of intelligences are more developed in them. An analysis that differentiates gender could be attempted. Given the correlational nature of our study, we could not isolate a true causal effect of our higher-level constructs on observed behavior. It could be argued that reverse causality might explain a role in the observed relationship. This is a more pressing issue with financial literacy, and further examination of the causal relationship between such literacy and observed financial behavior is warranted.
Furthermore, the fact that our sample mostly took participants from better-off sections of society (university students and early career workers) leaves open the question of the magnitude of the effects for more socioeconomic vulnerable members of society. However, previous experimental evidence makes us confident that our correlations would hold for other samples (Snowberg & Yariv, 2018). Further research must be oriented toward incorporating other high-level constructs for observed behavior and their indirect effects such as personality and overall competitiveness, as well as toward expanding samples to include more non-student populations, although their measurements might be more noisy (Snowberg & Yariv, 2018).
By way of closing, research gives us the bases to manage more precise marketing processes that target specific populations with products needed by people. The data could contribute to three specific areas: first, marketing strategies that are precise by type of intelligence; second, financial education processes contextualized by types of intelligence; third, the promotion of public policy regarding habits and credit consumption processes.
CONCLUSION
An important element we found on this research is related with the possibility to understand the people motivation when demand credit cards. An innovation in this paper is to introduce the multiple intelligence’s theory in discussion. Economic analysis has many limitations because the rationality assumption and Economics Psychology can answer about real motivations and biases in economic choices. We have many limitations, in order to extend the sample, because social perceptions an cultural institutions makes this field of study a complex matter.
Building on the previous statement, it is possible argue that the decision making around economy situations and, particularly about credit cards, is based on schemes related to more personal effects and structures than the rational analysis purposed by the “Homo economicus” model.