1. Introduction
Due to biogeographic conditions, Colombia has a great amount of biodiversity, but it is unfortunately among the top ten countries that have shown important loss of forest habitat. It has an annual destruction rate of 0.5% [1]. It is calculated that by the year 2050 almost 80% of species extinction will be the result of deforestation [2]. This is an ongoing cycle in Latin America that is brought about by the development of road infrastructure projects [3], which, without suitable environmental planning, contribute to the fragmentation of forests and biodiversity loss.
The main failures of environmental impact studies such as the descriptive nature of the assessments, the lack of measurable indicators and the absence of quantitative predictions [4] do not allow the potential impacts of new roads to be rigorously established, which could compromise the conservation of valuable resources in the long-term. These assessments should be complemented with spatial analysis models that help predict potential changes in the landscape caused by the construction of a road.
Statistical models have been widely used to model deforestation in the tropics, [5, 6] mainly in the Amazon [7,8]. Various pieces of research that included these models had identified the places most susceptible to deforestation and the main determinants. There has been no deep analysis or estimation of the condition of forests after the construction of new road infrastructure projects; this would help to improve the local management and conservation of forests.
Statistical models are attractive for decision makers due to their methodological stability and long application history. However, for these types of models, a priori assumptions should be made about the relationship between variables, which could not exist. The main advantage in the use of ANNs to make predictions is that this type of assumption is not required as they have the ability to capture nonlinear relationships. They can, therefore, be a more appropriate instrument when emphasis is placed on self prediction and not on the underlying relationships between independent and dependent variables [9].
ANNs have been widely applied in medicine and molecular biology. At the beginning of the 1990s the first studies were reported in the areas of ecology and environmental sciences [10]. Khoi and Murayama [11] used ANNs to predict the areas most vulnerable to deforestation, Larsen [12] used ANNs to evaluate the response of forest ecosystems in the presence of high amounts of carbon dioxide and ozone, and Pijanowski et al. [13] constructed an ANN model to predict changes in coverage and land use on a national scale.
The objective of this research is to construct neural network models, evaluate and choose the best model based on its performance (highest AUC value) to predict deforestation from 1980-2000, and evaluate the change in the probability of deforestation for a scenario that simulates the construction of new roads.
2. Methodology
2.1. Study area
The study area includes the Bajo Cauca and Northeast sub-regions of the department of Antioquia (Fig. 1) which contain six and ten municipalities and have a total area of 8.585 km2 and 8.645km2 respectively. These sub-regions are mainly devoted to mining and cattle ranching and have only marginal agricultural production [14]. These regions still have forest areas with a great diversity of natural resources; however, their geographical locations has led to them being involved in the planning of new road infrastructure projects aimed to improve the department´s connectivity with the northern part of the country. Therefore, it is an interesting zone to develop spatial deforestation models as a way of reducing the impacts caused by the construction of new roads.
Two roads were considered for this research: the “ El Bagre - San Jacinto del Cauca” road, 72 Km in length; and the “Variante Porce” road that goes from the municipality of Yolombo up to the municipality of Zaragoza, approximately 150 km in length [15]. This road was originally designed in the study region to reduce the traveling time from Medellín to the coast; however, the feasibility studies were rejected by the environmental authorities in the 90s due to environmental impact on ecosystems. Their current stance is to rethink the assessments since this “Variante” would arrive in Zaragoza using 60 km less road than what was proposed in section 2 (T2) of the “Autopistas de la Prosperidad” (Fig. 1).
2.2. Data and variables
Land coverage information for the years 1980 and 2000, using a scale of 1:25.000, was employed in the deforestation modeling. Land coverage information for 1980 was obtained after digitalizing more than 500 thematic plates [16] in analog format provided by the Ministry of Agriculture of Antioquia, and the land coverage for 2000 was the result of an inter-administrative agreement between the Ministry of Agriculture, the Administrative Department of National Planning and the “Instituto Geográfico Agustín Codazzi”. The information processing and homologation of coverage was carried out in a previous study using the Corine Land Cover methodology [16], and these were used as the basis to identify the changes from forest to no forest.
Six variables were built in raster format with a 30m pixel size. Orrego [16] indicates that it is best suited to represent deforestation and those variables that explain its occurrence.
Variables
Euclidian distance to rivers.
Euclidian distance to roads.
Distance to forest border.
Distance that represents the least cost to municipalities.
Slope.
Agricultural aptitude.
Although deforestation can be explained by a large number of variables, the underlying causes of deforestation in the study zone (poverty, poor forest management, insecurity in land tenure, etc.), which constitute the fundamental elements of the human-environmental relationship [17] are more difficult to identify than the proximate causes due to the availability of data. Therefore, biophysical and accessibility variables were used that provide a better spatial representation [18].
2.3. Neural network and validation training
The ANN training took place with the software R [19, 20] using the AMORE package, which, in turn, uses the back-propagation algorithm [21].
The proposed neural network has a unidirectional multilayer perceptron structure, with an input layer, a hidden layer and an output layer. Each explanatory variable is associated with a neuron in the input layer, and one neuron was assigned to the output that corresponded to the dependent variable. For this last layer, a logistic activation function was used because it is a categorical variable that takes values of zero and one (1= deforested, 0= not deforested)
According to the features of the package used, the weights start with random values within the range.
Where eo is the number of connections between the input layer and the hidden layer, and os is the number of connections between the hidden layer and the output layer.
The training was carried out with a balanced sample of 250,000 points (3% of the data). That is to say, from this sample 50%, of the data corresponds to random deforested points and the other 50% to random non-deforested points. The selection of this sample was made in order to improve the network performance and to be able to make better predictions since the learning network tends to be biased towards the class that has the majority in the training group [22,23] for a non-balanced sample.
The input data were standardized so that the values were in the range (0,1) since this speeds up the neural network training [24]. In addition, the standardization enables better initializations in the weights, which reduces the possibility of finding a local minimum. The data was transformed by using the maximum and minimum values in the original data set. By standardizing each variable, the ANNs assign equal importance to the data, so the problem of variables with different relevance due to their value range is avoided. The transformation was performed based on the following equation.
Using a standardized sample and changing the value of the training parameters, network models were trained. As Moreira and Fiesler [25] recommended, the values that were considered for the training rate were 0.01 and 0.05; both used a momentum of 0.5 and a rate of 0.1 with a momentum of 0.9. Similarly, the number of neurons in the hidden layer were adjusted and Hecht-Nielsen´s suggestion [26] was taken as the basis. This author states that the use of 2n + 1 hidden neurons (with n being the number of input neurons) can guarantee perfect adjustment of any ongoing function.
24 networks were constructed and trained. Due to the instability in training and poor error reduction after 5000 iterations, the cycle was interrupted for all networks, without significant accuracy losses. The logistic activation function was used for both the hidden layer and for the output layer, and the online learning method was used based on its ability to work with large training sets [27]. In addition, the network convergence is faster compared to the learning method in batch mode [28,29].
The quality of a model can be assessed in terms of its discriminatory capacity, that is to say the ability to predict the presence or absence of a feature [30]: in this case the presence or absence of deforestation. Therefore, the ROC curve (Receiver Operating Characteristic in English) and its associated area (AUC) were used. The ROC curve is independent of a threshold and provides a unifying criterion when evaluating the process of a model [31]. A detailed description of the ROC curve´s application to evaluate the prediction of land use and land cover change can be found in [32].
To perform the validation, a random sample that was independent from the training set, and had a size of 250,000 points, was selected. Using the ROCR package [33], the AUC values were estimated. This allowed some trends to be identified in the network's predictive capacity with respect to different sets of parameters (momentum, rate and amount of hidden neurons) as well as the best suited ANNs to predict deforestation.
The network that had best predictive capacity was chosen from 24-trained networks: that is to say, the one with the highest AUC value. The deforestation surface for the baseline scenario and the simulated scenario were estimated with this network under the assumption that they both had similar deforestation patterns. For the simulated scenario estimation, the new roads were added and the variables distance to roads, distance to forest border and distance representing the least cost to the municipalities were updated. The original values for the other variables were maintained.
The pixels affected by the new roads were identified by overlapping the raster files of the deforestation probability surface in the baseline scenario with the estimated surface for the simulated scenario.
3. Results
The networks that showed high AUC values were those trained with a lower training rate and greater amount of neurons in the hidden layer; this can be observed in Fig. 2.
The ANN that showed best predictive capacity obtained an AUC value of 0.8212 (Table 1). Based on this, a deforestation probability surface was generated for the area covered by forests in 1980 as well as the deforestation probability surface for the simulated scenario.
The comparison of the scenarios suggests that 1,152,565 pixels, approximately 103,729 ha of forest (pixel area of 900 m2), would increase its probability of deforestation (Fig. 3); 75,622ha showed a low increase (0-0.11), 20.186ha an average increase (0.11-0.37), and 7.922ha a high increase (0.37-0.9). However, some pixels, approximately 7%, showed a reduction in the probability of deforestation for the simulated scenario, which goes against what was expected based on the literature, which indicates that the probability of deforestation increases with the proximity to roads [34, 35]. This may be attributable to the expected error in the simulation, taking into account that the predictive capacity for the best ANN was approximately 82%.
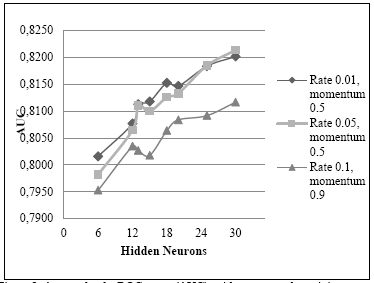
Source: The Autors
Figure 2 Area under the ROC curve (AUC), with respect to the training rates and the number of hidden neurons for a cycle of 5,000 iterations.
Table 1 Parameters of the five artificial neural networks that showed best predictive capacity.
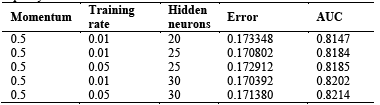
Source: The Autors
4. Discussion
As the training rate decreased, the AUC value increased, but we must bear in mind that even though the networks with low training rates show less error, compared with other rates, training is slower. Therefore, the value of the learning rate should be large enough to allow a quick learning process but small enough to ensure their effectiveness. For the current research, the training parameters that showed the best performance in terms of training times and predictive capacity was 0.05 for training rate and 0.5 for the momentum.
Another alternative for training would be the use of an adaptive network with a resilient back propagation algorithm in which the rate changes automatically during the training. This means that the problem of defining a global training rate that is appropriate for the whole learning process [36] is avoided.
The models generated in the training phase did not show wide differences in terms of accuracy; the AUC values were between 0.79 and 0.82. This is consistent with Müller and Mburu [37] who also found similar results when modeling deforestation with different neural network architectures; and Okwuashi et al. [38] who simulated changes in land use in Lagos, Nigeria for three time periods, obtaining AUC values between 0.7574 and 0.7850.
The evaluation of the potential effect of the two roads proposed for the simulated scenario suggests an increase in the deforestation probability for 103.729ha of forest. Wilson et al. [39] in their study, carried out in the temperate zone forests in southern Chile, found an increase in deforestation probability of about 27,000ha after simulating the construction of a new road. In the central region of México, Nelson and Hellerstein [34] simulated the impact of new roads on deforestation and demonstrated that access to roads significantly influenced the change in land use.
The results of the research show an increase in the probability of deforestation within 6 km of roads; the probability diminishes as the distance increases. Mertens and Lambin [40] found a negative relationship between proximity to roads and deforestation at a distance beyond 7.5 km, and Barber et al. [36], in their study undertaken in the Amazon, found that 95% of the deforestation occurred within 5.5 km of roads. The results suggest that there is an agreement between different studies on the spatial pattern of environmental impacts resulting from the construction of roads. This offers the possibility to develop appropriate models for spatial scales that are larger than what is considered in this investigation.
Other than the immediate impact of road construction within or near to forest areas, roads are permanent elements of the landscape [41]. So, even when they do not directly influence deforestation, they represent a long-term determinant to forest conversion. This is the case with the simulation of the “El Bagre - San Jacinto del Cauca” road, which does not go through forest areas but generates an increase in the deforestation probability in the eastern part of the study area. This shows that the spatial configuration of the scenery is determined, to great extent, by the proximity of forests to roads.
Freitas et al. [41] indicate that although the variable of distance to roads is a strong predictor of forest dynamics in deforestation processes, the effect can only be detected when agricultural expansion has stabilized over a certain period. However, the effect of the construction of a new road such as the “variante Porce”, which would cross areas with low agricultural aptitude could be the increase in mining and excessive wood extraction and not the planting of agricultural crops. This is disturbing if we take into account that ecological studies suggest tropical forest based on poor soils have greater levels of biodiversity than those that have better quality soils [42].
The simulation shows the areas for which there is an increased deforestation probability are associated with the roads designed. Consequently, this estimation is a conservative one since many paths and temporal trails could be built from new roads facilitating access to forests. Therefore, the area that will experience an increase in deforestation could possibly be greater, which would put at risk the environmental services that house the forest ecosystems.
5. Conclusion
The simulation with the network that presented the best predictive capacity during the training process showed an increase in the deforestation probability for 103.729ha of forest. Although the results cannot be validated with real data since the proposed roads have not yet been built, the development of this type of analysis provides planners with an empirical orientation on the magnitude and location of damage. Therefore, after the completion of road projects strict surveillance by the environmental authorities is needed and the design of policies aimed at creating incentives for forest protection, which would enable the population to take part in forest conservation activities.
Although carrying out projections for change through empirical deforestation models generates uncertainty, the study shows how the ANN has the ability to capture changes and trends in terms of deforestation probability. Therefore, greater precision can be achieved by developing models for shorter time periods that help not to predict the exact location of future deforestation, but rather the places that have a high risk of conversion.
Furthermore, the ANNs could be important tools to select alternative routes of linear structures since they allow all the environmental information for the area in which it is intended for the civil work to be located to be integrated. This would help environmental science technicians to visualize landscape transformation while the location of the linear infrastructure is defined. This represents valuable information that is useful in order to formulate and implement compensation forestry projects under the mechanism for reducing emissions for deforestation and degradation (REDD).