1. Introduction
The design of a distribution network of a supplying chain represents a strategic decision that mainly affects stock, transportation, service levels, prices, among other policies [1,2]. In the agro-food sector, the complexity of designing these networks increases mainly because of the high uncertainty in the demand, the supply and the particular conditions like perishability and harvest performance conditions.
Decisions under uncertainty are considered one of the main problems for agriculture [3]. A strategy to reduce risks associated with this sector is the so called contract farming (CF), generally entered by only one buyer and multiple farmers [4]. The main objective of CF is to assure the quantity and price of the supply, the buyers assuming the planning for the supply and distribution [5].
In the United States more than 60% of the main farmers have used this contract policy [6]. In Mexico, CF is one of the main government programs whose objective is to reduce commercial risks and assure supplying from the farmer to the intermediaries [5] who are legally obliged to receive certain volumes of production previously negotiated.
In this context, the objective of this study was to design a distribution network with the optimal cost using a mathematical mixed-integer linear programming model (MILP) to optimize a determined scenario within CF and subsequently apply a discrete event simulation model under certain parameters of uncertainty (stochastic). The hypothesis in this case was that through a hybrid model it is possible to reduce uncertainty and identify the key factors to design the distribution network minimizing the associated cost.
The suggested model for a supplying chain of barley in Mexico is similar to the works of [7] and [8] including specific aspects of this chain like harvest uncertainties, variations in the hubs’ and malt producers capacities, as well as the penalties/incentives for the quality of the delivered grain.
Considering the growing complexity of dealing with problems in agriculture, this approach is based on a hybrid model with an alternative to support decisions regarding the design of a distribution network under certain scenarios, including simulation in those processes subject to stochastic behaviors.
For this study, it is suggested a design of a distribution network of malting barley based on a hybrid model considering costs of transportation, hubs opening, penalties/incentives, harvest performance and loss risk as factors of great influence on prices and consequently on the produced, consumed and commercialized amounts, considering that malt producers assume, through the CF, the supplying and distribution operations of grain.
1.1. Literature review
The design of distribution networks of agricultural products implies the analysis of different conditions related to the own nature of the products. In this sense, the operations research (OR) has contributed through the application of mathematical techniques that allow optimizing resources in agriculture [9].
Through the OR hybrid approaches have been suggested based on simulation, whose objective has been to integrate uncertainty within the optimization model [10], with the advantage of providing, with this models, a better understanding of a supplying chain analyzing an optimal solution under stochastic conditions [11,12].
Hybrid models are also an alternative to reduce complexity in a problem with a lot of variables, generally not linear variables [3,12].
Authors like [13] suggest a model to design a grain transportation network considering the reduction of post-harvest losses, applying a series of sensitivity analyses under different scenarios in a distribution network in the United States.
[14] suggest a MILP to evaluate the feasibility of opening new grain processing plants with the purpose of increasing the production capacities in Turkey, this model looks for determining the location of new plants while minimizing fixed costs of installation and costs of transportation.
[7] identify several strategies to enhance the distribution cost through the implementation of a hybrid model of optimization and simulation in a supplying chain of wheat in Canada. In Holland, [1] assumed uncertainty in supplying, transportation and processing operations evaluating a hybrid model of optimization and simulation to design a network in flower sector.
Other approaches include suggestions like the one of [12] which combine optimization and stochastic environments with the objective of reducing the costs of production, transportation and inventory in a supplying chain integrated by different suppliers, producers and distributors who are connected through different means of transportation. [8] present a model based on optimization and simulation techniques to estimate an optimum global solution to combinatorial problems under uncertainty conditions.
According to [15] harvest risk constitutes one of the main factors in the production environment of agriculture, in this sense, the effects on harvest yield and production risks are suggested by [16] within a model of simulation and optimization that considers different scenarios to plan production strategies.
[17] evaluate different potential locations to install malt processing enterprises and later propose a MILP model to distribute beer and barley.
In sum, the operational aspects in production and distribution costs, and the uncertainty regarding harvest and quality are considered to suggest models that support decision making to design a network. However, there were no research works that take into account, from a CF point of view, the interaction between the buyers’ capacities and production uncertainty. Therefore, our approach includes an optimal solution considering stochastic variables in harvest yields, loss risk and penalties/incentives under different scenarios in CF.
2. Methods
According to [ 18], in 2016, 141 million tons of barley grain were produced in 46.9 million hectares, and they were mainly used as forage for human consumption and to produce malt for brewing, this cultivation representing a fundamental supply for brewing industry.
In 2017, the value of malt-beer exportations in Mexico was of 3.8 billion dollars with an annual average growth of 7.53 %, which has allowed to place this country in the first place at an international level on top of beer producers like Holland, Belgium and Germany [ 18,19].
The Central Plateau from Mexico that includes the states of Hidalgo, Puebla, México and Tlaxcala, is the main region that produces barley with nearly 70 % of the national production under rain-fed cultivation, this region also hosts three of the four malting agro-industries in the country, being the state of Hidalgo the biggest producer of this grain, specifically in the plateau region of Hidalgo there are nearly 6,438 farmers dedicated to cultivate this grain.
In this case study 243 farmers were selected, representing the biggest production stratum with surfaces for cultivation larger than 20 hectares, making together nearly 8,638 available hectares. Nine possible hubs were also selected and distributed throughout the cultivation zone, and two malt producers that participate as leaders in this supplying chain.
The CF in the supplying chain of barley in the plateau region of Hidalgo was used as case study to implement a hybrid approach of optimization and simulation with the purpose of showing better scenarios in terms of economy to plan the distribution of the harvested grain under certain conditions of uncertainty.
The flow of the product in the supplying chain selected for this study begins with the farmer (supplier) who, according to the CF, sends the harvested grain through a hub or directly delivers it to a malt producer (Fig. 1).
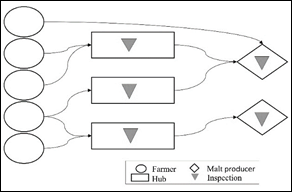
Source: The authors.
Figure 1 Schematic representation of the supply chain of barley in the plateau region of Hidalgo.
The farmers generally cultivates during rain-fed time (spring-summer) with variable historical yields between 0.7 and 4.5 tons/hectare [20], besides having a loss risk (weather effects) that has a negative impact on production. Subsequently, in the second link of this chain, the grain is received by a hub or malt producer evaluating quality under certain parameters like humidity and physical appearance, assigning incentives/penalties as a result, this means, a farmer can receive an additional percentage over the buying price per ton or, on the contrary, apply a discount for quality deficiencies. The grain is temporarily stored in the hubs to later send it according to the demand of malt producers.
In general, malt producers accept through CF to plan the supplying, working with their own hubs and associated transportation to deliver and process the grain. This influencing power over the supplying chain also determines the way of configuring the design of the distribution network.
2.1. Optimization model
The suggested mixed-integer linear programming model (MILP) was formulated considering the reduction of distribution cost of the harvest based on the malt producers’ demand. The results of this model show in a deterministic way the best allocation between farmers -hubs, farmers-malt producers and hubs -malt producers indicating the tons of grains that should be sent in each one of these nodes.
Indices and sets:
i ∈ I: set of farmers
j ∈ J: set of hubs
k∈ K: set of malt producers
n∈ N: set of candidate solution
Decision variable:
Xij: tonnes to transport from farmer i to the hub j.
X ik: tonnes to transport from the farmer i to the malt producer k.
X jk: tonnes to transport from the hub j to the malt producer k.
Y j : hub j is open or not.
Zn: binary variable for incorporating the cost of uncertainty for candidate solution n.
Parameters:
cij :freight cost (in dollars) from the farmer i to the hub j.
cik: freight cost (in dollars) from the farmer i to the malt producer k.
cjk: freight cost (in dollars) from the hub j to the malt producer k.
sumi: available offer (tonnes of harvest) from the farmer i.
capj: storage capacity (demand in tonnes) of the hub j.
demk:demand (in tonnes) of the malt producer k.
capmk: capacity to receive directly from the malt producer k.
fj : opening cost of the hub j.
Sn: uncertainty found by simulation for candidate solution n.
Tnj: binary parameter (1, if binary variable Yj in candidate solution n is 1; 0, otherwise).
Qmin: mínimum total expected cost with uncertainty obtained so far from simulations.
M: large number.
Minimize
Subject to
The objective function (1) is to minimize the transportation costs from the farmers to the hubs, from the hubs to the malt producers, from the farmers to the malt producers, the opening costs of hubs and the impact on uncertainty obtained in the simulation of the candidate solution n. A candidate solution is defined as an optimal deterministic scenario previously obtained under certain parameters. Equations (2) and (4) are restrictions indicating compliance in the demand and supply, restriction (3) represents a balance between ins and outs of the hub to avoid accumulating stock at the end of the planning period, this means, the amount of tons of barley sent from the farmer to the hub must be equal to the amount taken from the hub to the malt producer. Restriction (5) shows that the amount sent from the different farmers to the hubs must not be higher than the available capacity of storage; restriction (6) based on the CF points out that the amount sent from the farmers to the malt producers must be lower than the capacity of receiving, meaning that the malt producers only allow receiving directly (without going through a hub) certain amount of grain tons. Restriction (7) indicates that the amount sent to malt producer through hubs must be lower or equal to their available capacity. Restrictions (8) and (9) are similar to the ones suggested by [8] to include uncertainty of the candidate solution. Restriction (10) is included in the model to establish a ceiling limit obtained in the simulation of the previous candidate solution; finally restrictions (11) - (15) define the non-negative and binary variables.
2.2. Simulation model
Based on the frame of reference suggested by authors like [18] the simulation has as objective to evaluate under uncertainty conditions the feasibility of the solution suggested by the MILP model, stochastically integrating possible variations in the levels of supply, storage capacity and demand.
The harvest yield, loss risk, penalties/incentives, as well as hubs’ capacities and malt producers’ demands were determined as stochastic entries of the simulation model, while locations of hubs and malt producers, transportation costs, opening costs of hubs and production capacities of farmers are considered as deterministic entries.
For this simulation, data from historical harvest periods since 2003 to 2015 were collected by using information from 20] which were analyzed through distribution adjustments with the software ExpertFit selecting the top ranked distributions. Harvest yields for each farmer were adjusted to Beta, Weibull and Pearson type VI distributions depending on the historical data (2004-2016) of each one of them, in the case of loss risks, Beta, Weibull and Johnson distributions were obtained as best adjustments.
To determine penalties/incentives that originate in the grain delivery, data were adjusted to a uniform distribution based on the quality levels suggested in the standard NMX-FF-043-SCFI-2003 4]. Demands in malt producers through adjustments of distribution were generated randomly between 1,000 and 13,000 tons of grain based on a uniform distribution. The capacities of hubs according to the adjustments of obtained historical data are considered normally distributed with variations in the standard deviation of 15%, 25% and 35% compared to the average, in the case of production capacities they are considered within a range from 20 to 80 available hectares for cultivation per farmer.
The costs of transportation were calculated based on the distance between the farmer, the hubs and the malt producer. The opening cost of the hubs was determined between a range of 3,000 and 4,500 dollars.
In this simulation it is also assumed that:
Barley production (expressed in tons) obtained with a certain yield is considered an entity which is totally consumed by malt producers, being them the only clients.
Hubs work as inspection points and also as temporary warehouses at malt producers.
The amount of product carried form a hub to a malt producer does not fall into any penalty nor incentive in the delivery, since the grain quality has been previously controlled and it is kept that way from the hub.
Malt producers previously define the percentages that each one of them will consume from the total production obtained in the harvest.
Transportation has the enough capacity to move the grain.
Fig. 2 shows the layout used for this simulation, in which 243 farmers generate certain levels of tons of barley (entities) based on the suggested distributions and then, according to the MILP model, it is sent to one of the 9 available hubs which will send these entities to the malt producers.
The way out of this model are the total transportation and opening costs between the different nodes, as well as the distribution cost for all the suggested supplying chain considering uncertainty in harvest yields, capacities, penalties/incentives and loss risk.
2.3. Hybrid model
The hybrid approach in this proposal uses the deterministic solution of a MILP model to later, through simulation, estimate the uncertainty impact (S n ) in the objective function, the difference between the cost in the MILP model and the simulation is incorporated again to formulate the optimization with this parameter. This process is performed in an iterative way until finding the network design that minimizes the total cost of distribution and the impact in the uncertainty under the particular conditions of this supplying chain.
The steps for the solution of this approach are shown in Fig. 3, this procedure starts by obtaining a possible design for the distribution network with the MILP model that indicates the hubs (Y J) that must be opened and the flows in the amount to be sent ( x ij , x ik, x jk ), then, the network is evaluated through a simulation to calculate the impact of uncertainty(S n ) also obtaining a value Q min updating if necessary, the values Z n and T nj that are incorporated to evaluate a possible solution in particular. The obtained information from the previous steps serves as feedback on the optimization model and the network design, the procedure continue to evaluate other candidate solutions until finding the one with the best design under conditions of uncertainty.
This model was solved through coding in Matlab R2015a, Microsoft Excel 2016 and the simulator ProModel v4. First, the MILP model is executed in Matlab using the intlinprog algorithm which applies three methods (Branch-and-Bound, Cutting Plane and Heuristics) to find a solution and later, through a Microsoft Excel interface, the obtained results are included as entries to the simulation model in ProModel.
3. Results and discussion
Based on the CF nine possible scenarios were designed (table 1) formed by variations of 15%, 25% and 35% with respect to the average capacity of the hubs and three possible levels for the assignation capacity of the grain among the malt producers (10%-40%, 41%-70% and 71%-90%).
For example, in a possible scenario there is a variation of 15% in the available capacity of hubs and a malt producer can plan on receiving between 10% and 40% of the total harvest, while the other producer must receive, according to the contract, the rest of the production.
The location of farmers, hubs and malt producers selected for this design are shown in Fig. 4.
For each scenario, 20 problems were randomly generated with the suggested variations with the objective of comparing the different possible configurations under uncertainty.
3.1. Scenarios
Each one of the random problems formulated within the different scenarios were solved through a MILP model with the purpose of finding the best combinations to design the network, subsequently, the deterministic solutions were simulated under conditions of uncertainty considering ten replicas suggested by [18] to enhance the precision of the simulation results. Afterwards, the hybrid model determined the best solution under conditions of uncertainty minimizing the difference between the deterministic model and the stochastic model.
For example, in the case of the scenario two where the variation of a malt producer capacity is between 41% and 70% and there is a variation of 25% in the capacity of hubs, the results indicate four possible arrangements to design the network considering the opening of four to five hubs, these arrangements were analyzed in an iterative way under conditions of uncertainty resulting as optimal solution a design considering just four hubs with a total cost of 259,759 USD. Fig. 5 shows the procedure, rejecting in this case those solutions above Q min in each iteration.
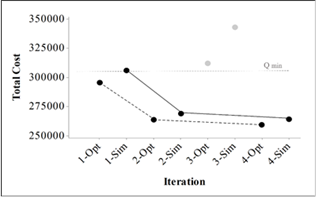
Source: The authors.
Figure 5 Obtained costs from the optimization and simulation models in each iteration for scenario two.
The suggested solution in this scenario indicates a network design like the one showed in Fig. 6.
Fig. 7 shows the obtained results for the set of scenarios, initially for a level one (assignment of grain 10% - 40%) with a variation in the capacity of hubs of 15% the best cost was 272,723 USD considering the opening of five hubs, with variations of 25% and 35% were obtained for this level with a minimal cost of 259,759 and 268,261 USD respectively suggesting the opening of four hubs in both cases.
In the level two (assignment of grain 41% - 70%) the cost was 260,821; 265,436 and 262,238 USD for variations of 15%, 25% and 35% respectively considering the opening of four hubs in all cases. In the last level (assignment of grain 71 % - 90%) the obtained costs were 235,245; 232,463 and 242,571 USD for the variations of 15%, 25% and 35% respectively considering in the first case the opening of five hubs and four hubs in the other cases. In Fig. 7 it can be observed the improvement of costs as the capacities of malt producers vary, in this case, the higher the assignment, the better the distribution cost.
Finally, the previous results were applied in an Analysis of Variance (ANOVA) with the purpose of determining the effects on the suggested variations (table 2).
It was found that there is a dependent relationship between the variation of capacities of grain assignment of malt producers and the distribution cost in this supplying chain, meaning that the different levels in the capacities have an influence on the cost of the network design.
The level of significance associated to malt producers in this study was of 0.001 which demonstrates that the capacities of grain assignment planned by malt producers through CF explain a significant part of the observed variation in the distribution cost for this chain. The level of significance of the hubs’ capacity was of 0.801 and for the interaction hub capacity ( malt producer capacity was of 0.852 indicating that these factors are not significant.
The value R-squared obtained in the ANOVA was of 0.817 proving that, in general, factors of capacity of hubs and of malt producers explain the 81.7% of variance of the cost-dependent variable.
4. Conclusions
The hybrid model applied to a supplying chain of barley in Mexico allows obtaining a solution under an approach that combines the optimization and simulation of discrete events in order to include particular aspects like variability of harvest yields, levels of loss risk, as well as certain penalties/incentives that are applied based on the quality delivered throughout different links (farmers - hubs - malt producers). The simulation evaluates the obtained results in an optimization model recognizing the influence of stochastic factors on the operations of harvest and distribution.
Through nine possible scenarios for the planning of CF, this approach determines the best combinations for a grain distribution that minimizes the difference between a deterministic and a stochastic model.
The obtained results for the case study show that the planning of malt producers’ capacities are determining factors to enhance the cost associated with the grain distribution and, therefore, they must be analyzed within the CF.
Although harvest yields and loss risk are indicators that must be evaluated in agricultural planning, for future research on modelling, other uncertainty elements can be included that have an impact on the quality of the product like the type of seed used for cultivation, the weather conditions, the type of soil, vegetation index, harvesting machinery and the available infrastructure for storage. The model suggested herein evaluates in economic terms the convenience of the distribution network design from the buyer’s perspective, so it is also suggested to analyze the benefits from the farmer’s perspective.