Introduction
Throughout history, humanity has faced multiple potentially deadly viral and parasitic diseases, including yellow fever, dengue, zika, chikungunya, and malaria, which are closely related because they are transmitted by a mosquito (Diptera: Culicidae). These diseases are highly prevalent in tropical areas and the risk of contracting them depends, to a large extent, on rainfall, temperature and the living conditions of each place.2
Mosquitoes are considered one of the most versatile living organisms in the world as they can breed in any pool of water, such as puddles or tanks; however, their reproduction is influenced by certain biotic and abiotic factors.3,4These insects need the blood of humans and/ or other vertebrates to make their eggs fertile and viable, so they represent a threat to humanity since they are vectors of pathogenic microorganisms of several vector-borne diseases that can become burdens for many countries from the health, economic and social point of view.5,6
Natural disasters threaten the safety of people, hurricanes being the most destructive phenomenon in the tropics because of all that their passage entails.7-10 Since the frequency of these events is on the rise, knowing their behavior is essential; for this reason, in Cuba, seasonal forecasts are constantly made based on the oceanic and atmospheric conditions that preceded the past hurricane seasons (active or inactive), as they provide useful information about trends that could be observed in similar future seasons.11
Although the occurrence of hurricanes cannot be predicted accurately, their impact can be determined in advance. Moreover, research in this area has made significant progressin recent years, so much so that in 2012, for the first time, it was possible to forecast an event of this type a year in advance.12 To this end, high-quality forecasts are made using the regressive objective regression (ROR) methodology, which, due to its simplicity and accuracy, can be useful to know the future of climate variables or daily data years in advance.13,14 Forecast times can extend to 11 years of the solar cycle or other longer cycles known in nature.
By using the ROR methodology, the population dynamics of insects such as mosquitoes, and their interactions with certain environmental variables, can also be modeled. This is helpful to establish timely control and prophylactic measures in epidemiological surveillance programs.12,13,15
In this sense, the objective of this research was to assess the impact of atmospheric pressure on mosquito population density in the province of Villa Clara, Cuba, through mathematical models based on the ROR methodology.
Materials and methods
Area and period of study
The study was carried out between 2007 and 2017 in Villa Clara, a province located in the central region of Cuba, bordering on the north with the Atlantic Ocean and on the east, west and south with the provinces of Sancti Spíritus, Matanzas and Cienfuegos, respectively. Politically and administratively speaking, Villa Clara has 13 municipalities, and its capital is Santa Clara (Figure 1).
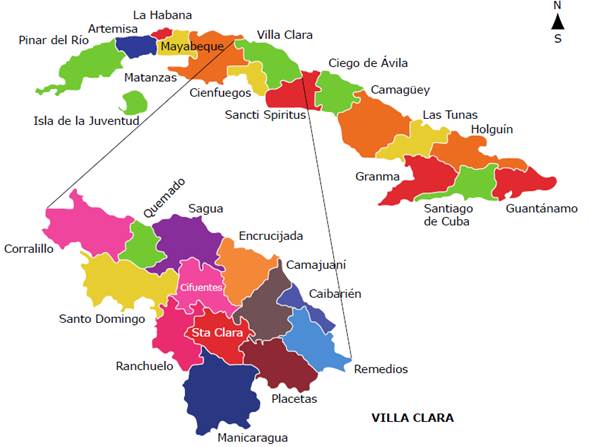
Source: Own elaboration.
Figure 1 Political map of Cuba and the province of Villa Clara. Source: Own elaboration.
Currently, Villa Clara has five weather stations located in Sagua la Grande (latitude 22°48'17" N and longitude 80°05'32'' W), La Piedra (latitude 22°06'48'' N and longitude 79°58'48'' W), Santo Domingo (latitude 22°35'08'' N and longitude 80°13'35'' W), Caibarién (latitude 22°29'48'' N and longitude 78°28'14'' W) and El Yabú (latitude 22°27'43'' N and longitude 79°59'30'' W), the latter located in the city of Santa Clara.
Catching and identifying mosquitoes
Trees and shrubs located on the margins of river ecosystems, as well as discarded artificial water-holding containers (cans, bottles, tires, etc.), were inspected to capture mosquitoes in preimaginal stages. Samples were collected in rivers, streams, ditches, gullies, lagoons, marshes and swamps, with special emphasis on banks because they are usually quiet and shallow environments where mosquitoes breed.16 Similarly, in order to compare the results obtained with the information available in the area and establish possible coincidences, the records and maps of the Unidad Provincial de Vigilancia y Lucha Antivectorial (Provincial Unit for Antivectorial Fight and Surveillance - UPVLA) of Villa Clara were consulted, as it archives the entomological records of the region.
Larvae and pupae were captured using ladles17 and transferred to the laboratory in 250mL plastic bottles duly labeled; general larval density was considered for this process. On the other hand, adult mosquitoes were captured using light traps and were transported in 50 mL glass jars, which contained absorbent cotton balls impregnated with ether and/or chloroform.
Preimaginal and adult specimens captured were identified in the Medical Entomology Laboratory of the UPVLA according to dichotomous and pictorial access keys18-20 and following the taxonomic classification of the Systematic Catalog of Culicidae of the Walter Reed Bio-systematics Unit.21 As part of the research, the annual number (2007-2017) of breeding sites per municipality in the province was considered and the impact of atmospheric pressure on mosquito population in the Santo Domingo station was analyzed since the results can be extrapolated to the rest of the municipalities in the province due to their geographical proximity.
Statistical analysis
The ROR methodology, which consists of several steps, was used to develop the mathematical model to predict breeding sites.22,23 First, sawtooth (S) and inverted sawtooth (IS) dichotomous variables were created for the number of cases (NoC) in the database used for ROR modeling. The NoC coefficient in the model represented the trend of the series and the variable to model was the number of mosquito breeding sites. Thus, the variability of the number of breeding sites was established by S and IS coefficients plus the NoC coefficient, explaining a large percentage of the variance (close to 100%). The S value was 1 for odd NoC, the IS value was 0 for even NoC, and vice versa.
Later, a regression analysis was performed using the statistical package SPSS version 19.0, specifically with the ENTER method, which allowed obtaining the predicted variable and the ERROR.
Then, the autocorrelogram of the variable ERROR was obtained taking into account the maximum values of the partial autocorrelation function (PACF). New variables were then calculated based on the significant lag of the PACF and were included in a new multivariate linear regression analysis, where they were regressed in a successive approximation process until a white noise was obtained in the regression errors. In the case of the atmospheric pressure, one-year lags were used, as done by other authors for climatic indexes.10,22,23 It should be noted that, in the Provincial Meteorological Center of Villa Clara, the atmospheric pressure values are recorded every three hours.
For mathematical modeling, information on the number of breeding sites reported annually in each of the municipalities of Villa Clara between 2007 and 2017 was taken into account; these data were analyzed through descriptive statistics: the minimum and maximum values were established and the average was estimated.
Results
In the present research work, 43 species of mosquitoes of 13 genera were identified in Villa Clara. Several of these species had marked ubiquity (Table 1).
Table 1 Distribution of the identified mosquito species in the province of Villa Clara, Cuba. 2007-2017.
Mosquito species | Classification and year | Municipalities * | Total number of municipalities |
---|---|---|---|
Aedeomyia squamipennis | Lynch Arribálzaga, 1878 | 9, 12 | 2 |
Anopheles albimanus | Wiedemann, 1821 | 1, 2, 3, 4, 5, 6, 7, 8, 9, 10, 11, 12, 13 | 13 |
Anopheles atropos | Dyar & Knab, 1906 | 5, 6 | 2 |
Anopheles grabhamii | Theobald, 1901 | 5 , 6,11 | 3 |
Anopheles vestitipennis | Dyar & Knab, 1906 | 3, 5, 6, 7, 8, 9, 11 | 7 |
Anopheles crucians | Wiedemann, 1828 | 5 , 8,12 | 3 |
Aedes aegypti | Linnaeus, 1762 | 1, 2, 3, 4, 5, 6, 7, 8, 9, 10, 11, 12, 13 | 13 |
Aedes albopictus | Skuse, 1894 | 1, 2, 3, 4, 5, 6, 7, 8, 9, 10, 11, 12, 13 | 13 |
Aedes mediovittatus | Coquillett, 1906 | 1, 2, 3, 4, 5, 6, 7, 8, 9, 10, 11, 12, 13 | 13 |
Aedes scapularis | Rondani, 1848 | 2, 3, 4, 5, 6, 7, 8, 9, 10, 11, 12, 13 | 12 |
Aedes sollicitans | Walker, 1856 | 1, 3, 4, 5, 6, 7, 10, 11 | 8 |
Aedes taeniorhynchus | Wiedemann, 1821 | 1, 2, 3, 4, 5, 6, 7, 10, 11 | 9 |
Aedes tortilis | Theobald, 1903 | 3, 4, 5, 7, 9 | 5 |
Aedes walkeri | Theobald, 1901 | 2, 6, 11, 12 | 4 |
Coquillettidia nigricans | Coquillett, 1904 | 9, 11 | 2 |
Culex americanus | Neveu-Lemaire, 1902 | 6, 9 | 2 |
Culex atratus | Theobald, 1901 | 4, 5, 6, 8, 9, 10 | 6 |
Culex bahamensis | Dyar & Knab, 1906 | 6, 8 | 2 |
Culex cancer | Theobald, 1901 | 1, 5 , 6 | 3 |
Cx. chidesteri | Dyar, 1921 | 1, 2, 6, 8, 9, 11, 12 | 7 |
Culex corniger | Theobald, 1903 | 2, 3, 4, 5, 6, 7, 8, 9, 10, 12, 13, 13 | 12 |
Culex erraticus | Dyar & Knab, 1906 | 4, 5, 6, 7, 8, 9, 10, 12, 13 | 9 |
Culex iolambdis | Dyar, 1918 | 8, 9 | 2 |
Culex nigripalpus | Theobald, 1901 | 1, 2, 3, 4, 5, 6, 7, 8, 9, 10, 11, 12, 13 | 13 |
Culex pilosus | Dyar & Knab, 1906 | 1, 3, 4, 5, 6, 8, 13 | 7 |
Culex quinquefasciatus | Say, 1823 | 1, 2, 3, 4, 5, 6, 7, 8, 9, 10, 11, 12, 13 | 13 |
Culex sphinx | Howard, Dyar & Knab, 1915 | 6 | 1 |
Culex secutor | Theobald, 1901 | 8, 13 | 2 |
Mansonia titillans | Walker, 1848 | 3, 6, 8, 9, 10, 11, 12 | 7 |
Limatus durhamii | Theobald, 1901 | 9, 12 | 2 |
Orthopodomyia signifera | Coquillett, 1896 | 8, 12 | 2 |
Psorophora ciliata | Fabricius, 1794 | 1, 2, 3, 4, 6, 7, 8, 9, 10, 11, 12, 13 | 12 |
Psorophora confinnis | Lynch Arribálzaga, 1891 | 1, 2, 3, 4, 5, 6, 7, 8, 9, 10, 11, 12, 13 | 13 |
Psorophora howardii | Coquillett, 1901 | 6, 7, 8, 9, 10, 12, 13 | 7 |
Psorophora johnstonii | Grabham, 1905 | 6 | 1 |
Psorophora pygmaea | Theobald, 1903 | 1, 4, 5, 6, 7, 9, 10, 13 | 8 |
Psorophora santamarinai | González Broche, 2000 | 6 | 1 |
Psorophora insularia | Dyar and Knab, 1906 | 6 | 1 |
Psorophora infinis | Dyar and Knab, 1906 | 8 | 1 |
Toxorhynchites portoricensis | von Roder, 1885 | 5, 6 | 2 |
Uranotaenia sapphirina | Osten-Sacken, 1868 | 3, 4, 5, 6, 7, 8, 9, 11, 12 | 9 |
Wyeomyia vanduzeei | Dyar & Knab, 1906 | 9 | 1 |
Wyeomyia mitchellii | Theobald, 1905 | 8, 9 | 2 |
* Each municipality was identified with a number: 1 : Corralillo, 2: Quemado de Güines, 3: Sagua la Grande, 4: Encrucijada, 5: Camajuaní, 6: Caibarién, 7: Remedios, 8: Placetas, 9: Santa Clara, 10: Cifuentes, 11: Santo Domingo, 12: Ranchuelo, and 13: Manicaragua.
Source: Own elaboration.
Table 2 presents the descriptive statistics for the number of breeding sites per municipality; it is possible to see that the highest mean and standard deviation (σ) are found in the municipality of Santa Clara and that the lowest are in Encrucijada. The maximum value obtained for the time series (11 years of annual data) was 8 778 in Santa Clara, while the minimum value was 0 in Corralillo, Quemado de Güines, Encrucijada and Caibarién. The overall average number of breeding sites in the province was 7 143, with a σ of 2 165.
Table 2 Descriptive statistics of the number of annual breeding sites in the province of Villa Clara, Cuba 2007-2017.
Municipalities | N | Minimum | Maximum | Mean | σ |
---|---|---|---|---|---|
Corralillo | 11 | 0.00 | 44.00 | 12.72 | 15.49 |
Quemado de Güines | 11 | 0.00 | 117.00 | 17.00 | 34.33 |
Sagua la Grande | 11 | 4.00 | 1 199.00 | 475.27 | 468.07 |
Encrucijada | 11 | 0.00 | 14.00 | 6.36 | 5.35 |
Camajuaní | 11 | 1.00 | 108.00 | 44.36 | 36.37 |
Caibarién | 11 | 0.00 | 54.00 | 18.81 | 18.55 |
Remedios | 11 | 1.00 | 249.00 | 52.00 | 76.57 |
Placetas | 11 | 10.00 | 438.00 | 89.45 | 126.31 |
Santa Clara | 11 | 3 734.00 | 8 778.00 | 5 987.63 | 1 549.21 |
Cifuentes | 11 | 3.00 | 78.00 | 25.72 | 25.29 |
Santo Domingo | 11 | 5.00 | 204.00 | 91.09 | 80.06 |
Ranchuelo | 11 | 36.00 | 675.00 | 185.81 | 214.27 |
Manica ragua | 11 | 6.00 | 381.00 | 137.09 | 145.17 |
Provincia | 11 | 4 497.00 | 10 806.00 | 7 143.36 | 2 165.59 |
σ: standard deviation; N: number of years studied from 2007 to 2017.
Source: Own elaboration.
The ROR model was chosen because, during the investigation, it was concluded that by combining lags 1, 2 and 6 (corresponding to 1 year, 2 years and 6 years, respectively) a perfect model could be obtained for each municipality but the parameters of influence could not be measured since there were divisions by zero. The proposed model proved to be perfect according to the analyzed data since no standard error was observed and no autocorrelation was detected in the residues by means of the Durbin Watson statistic. The analysis of variance (ANOVA) for the model indicated that Fisher's F could not be determined because the error was zero, and to calculate this statistic, it is necessary to divide by the error, which creates a mathematical uncertainty.
Figure 2 shows the result of the modeling for the province; it is possible to see that the predicted value has excellent coincidence with the real value from 2013 to 2017.
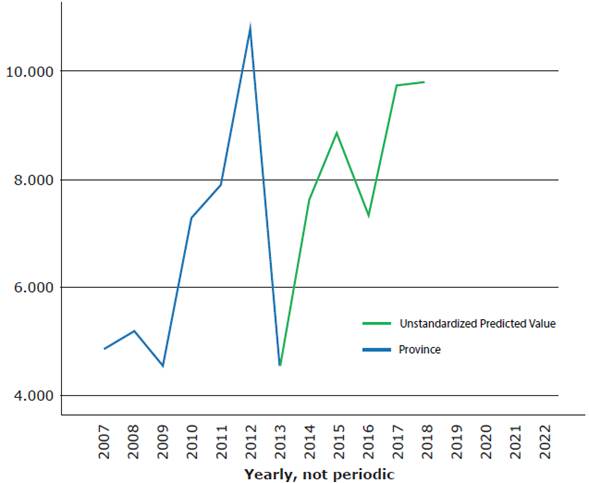
Source: Own elaboration.
Figure 2 Real and predicted value of the number of mosquito breeding sites in the province of Villa Clara, Cuba.
The highest variance explained was the same for all municipalities (100%), but there was an upward trend in Cifuentes since the NoC coefficient was positive, while the trend was downward in Caibarién and Ranchuelo since the NoC coefficient was negative (Table 3). NoC coefficient was recorded when it was not significant. The ANOVA was only doneforthe larval stage.
Table 3 Number of breeding sites per municipality for larval density in the province of Villa Clara, Cuba 2007-2017.
S: sawtooth; IS: inverted sawtooth; NoC: number of cases.
* Lag 1, Lag 2, and Lag 6 are the predicted lags, which in this case are 1 year, 2 years and 6 years.
Source: Own elaboration.
The forecast values obtained using the atmospheric pressure prediction in the Santo Domingo station showed a behavior similar to that calculated without it (Figure 3).
Discussion
From the 70 species of mosquitoes identified in Cuba so far,16,24 43 were found in Villa Clara in the present study.
The most common genera with the highest presence in the municipalities studied were Anopheles, Culex and Psorophora, while the most common and widely distributed species were Anopheles albimanus, Culex quinquefasciatus, Culex nigripalpus, Aedes mediovittatus, Psorophora confinnis, Aedes aegypti and Aedes albopictus (found in the 13 municipalities), followed by Culex corniger, Aedes scapularis and Psorophora ciliata (found in 12 of the 13 municipalities). The most common species were An. albimanus, Anopheles crucians, Culex atratus, Cx. quinquefasciatus, Cx. nigripalpus and Ps. Confinnis, which were found in practically all the ecosystems sampled and in relatively high abundance, a fact that coincides with what was reported by Mar-quetti-Fernández,25 specifically for Cx. quinquefasciatus in urban ecosystems. The great phenotypic plasticity of the mosquitoes was also evident since it was found that they are capable of exploiting any habitat.16,26-30
Ae. aegypti and Ae. albopictus are largely found in Villa Clara, which is noteworthy because they are high-risk entomoepidemiological species due to their involvement in infectious diseases such as dengue fever, yellow fever, West Nile fever, chikungunya and Zika virus.31-34 Currently, these two species are present practically in the entire Cuban territory and they are increasingly expanding because human activity generates an important number of breeding sites.33 This expansion shows their high phenotypical plasticity and great capacity of adaptation to different ecological niches.35-37
According to the descriptive statistics, there is great variability between municipalities regarding the number of breeding sites per municipality (Table 2), which may be explained by the physical and geographical characteristics of each place, as well as the quality of the samples, aspects that are consistent with the results of previous research.30,31
Considering that the value obtained in the present study using the Fisher's F test was high, the model designed here to establish mosquito population density is extremely good since the higher the Fisher's F, the higher the probability of having a good model in the ANOVA. 38,39
During the research, influence parameters could not be measured because they were divided by zero, so it was necessary to resort to mathematical definitions made by other authors and even for other organisms.40,41 The greatest variance explained was the same for all municipalities and it was 100%. Moreover, as mentioned above, there was a trend towards a decrease in the number of breeding sites in Caibarien and Ranchuelo, while there was an upward trend in Cifuentes. The most significant predicted lags were at 1 year, 2 years and 6 years (Table 3), which coincides with other studies where similar results were obtained and good modeling results were achieved through ROR models.42-44
The impact of the atmospheric pressure predicted in the four stations using the ROR methodology explains 100% of the variance in this new model. From all the pressure measurements per station obtained, the model selected the atmospheric pressure registered in the Santo Domingo station, which resulted in an impact coefficient of 13.84. Therefore, as atmospheric pressure increased in Santo Domingo, the number of breeding sites in the provinces increased; this coincides with previous works carried out in other municipalities of the province to measure larval density9,12,40 and confirms the impact of atmospheric pressure and, consequently, the performance of the North Atlantic anticyclone, which is the main climate modulator in the island of Cuba.
Conclusions
There is a close relationship between atmospheric pressure and mosquito population density since both total and specific larval densities increase as atmospheric pressure increases. These findings confirm the possibility of mathematically modeling the effect of atmospheric pressure on mosquito population density (Diptera: Culicidae) in Villa Clara, Cuba.