Dear Editor
A study published in Issue 1 of Volume 69 of this Journal evaluated burnout levels in Colombian medical residents and demonstrated the impact of this syndrome on those suffering from it.1 Burnout is a response to the emotional and interpersonal problems that arise at work, and it involves feelings of exhaustion, indifference, perception of incompetence due to a lack of resources to cope with responsibilities, dissatisfaction, and low self-esteem. 2
Burnout syndrome has become more common in both students and university professors during the COVID-19 pandemic due to a shift in the approach to academic responsibilities and the management of personal and social life. 3 Therefore, determining its causes is critical to understanding, preventing, and managing it. This assessment can be made using network analysis, a method that graphically represents a series of entities or nodes that may correspond to variables, constructs or individuals; the most common type of network analysis is social network analysis, which considers people as network units. 4
However, during the last decade, another variant of network analysis has been included in the clinical setting, represented by statistical correlations (directed or undirected) and based on graph theory, 5,6 in which the edges represent zero-order or partial causal correlations between nodes (variables), and associations structure the dynamic model. The graphical representation of this model facilitates the interpretation of the results: the thicker the connection between the variables, the greater the statistical relationship. 6,7
Network analysis is a multivariate model composed of numerous regularized nonlinear relationships after multivariate control of network elements, i.e., after eliminating the most spurious correlations using the LASSO method. 5,6 This favors the inclusion of variables of different nature and allows to evaluate multiple health aspects and their comorbidity, as well as the interaction between etiological factors and modulators. For example, Isvoranu et al.,7 through a network study, evaluated the correlation between psychiatric variables such as anxiety, depression and psychosis, and quality of life; physical and mental health status; and chronic diseases such as diabetes, high blood pressure, asthma, back problems, migraines and smoking in adult Singapore residents.
The analysis of networks allows to establish the "core" elements (high centrality indices) that have the greatest clinical implication in the assessment of the burnout syndrome and its comorbid prevalence with other diseases. This is paramount in the current context where COVID-19 has caused a negative psychological impact on society as a whole. These core elements influence the interactions of the other components, with higher values increasing the likelihood of strengthening the other correlations and lower values reducing the other connections and even causing the entire network structure to collapse. 5,6 Thus, core elements are critical in the planning and personalized development of clinical interventions in the current COVID-19 pandemic.
This approach is also useful in the evaluation of the metric properties of a clinical instrument because of its statistical similarity to factor analysis. 8 For example, Peixoto et at.9 used it to assess the dynamics of association between the variables investigated (anxiety, depression, stress, and life satisfaction) in a study in which they validated a questionnaire on suicidal ideation, impulsivity, and self-harm in Brazilian adolescents, while Ramos-Vera et at.10 used it to assess a post-traumatic growth scale in Peruvian adolescents who experienced potentially traumatic events.
Network analysis also allows to synthesize a general description of the literature based on the search results obtained by several researchers; this is known as a citation network, and it facilitates the inspection of the relationships between several articles, as well as the measurement of their relative importance. 11
Similarly, estimating two simultaneous network structures using network analysis provides a novel way of dynamically comparing associations between variables and allows exploring different clinical hypotheses, even at different times (before and after an event or clinical intervention). For example, Di Blasi et al.12 evaluated two simultaneous networks of anxiety, depression, stress, and fear of COVID-19 at two different times: during (April 7-24, 2020) and after (May 18-31, 2020) one of the mandatory lockdowns decreed in Italy. In turn, Fried et al.13 published another study that evaluated simultaneous networks before and after covariate control, including biochemical (inflammatory markers), anthropometric, and psychological measures to specify those variables with the greatest causal influence on the network. Moreover, the use of other clinical measures such as neuropsychiatric, 14 neuroanatomic, 15 and genomic measurements, 16,17 as well as the inclusion of risk and protection factors, has been reported in network analyzes. 18
Currently, the study of multiple clinical entities related to the negative impact of the COVID-19 pandemic, such as fear, anguish and stress, has been promoted, 12,19 and two articles using network analysis in this context were published in South America in 2021. 19,20 However, more dissemination and training on this approach are urgently required to promote its widespread use in health science research.
Network analysis also allows the assessment of dynamic changes through a network of directed relationships over time. This approach was used by Martin-Brufau et al. 21 to assess longitudinally (two weeks) the dynamics of the emotional states of 187 participants during the first COVID-19 quarantine in Spain.
Below, we present an example of a network analysis based on data obtained by Ramos-Vera et at. 22 in a study that involved a network analysis of depressive symptoms in 2 915 Peruvian adults diagnosed with hypertension who responded to the Patient Health Questionnaire-9 (PHQ-9). There, using a network clustering algorithm, system variables were labeled with specific colors that refer to an underlying causal structure (items with stronger relationships) and represent two factors of depressive symptoms: somatic and cognitive-affective Figure 1.
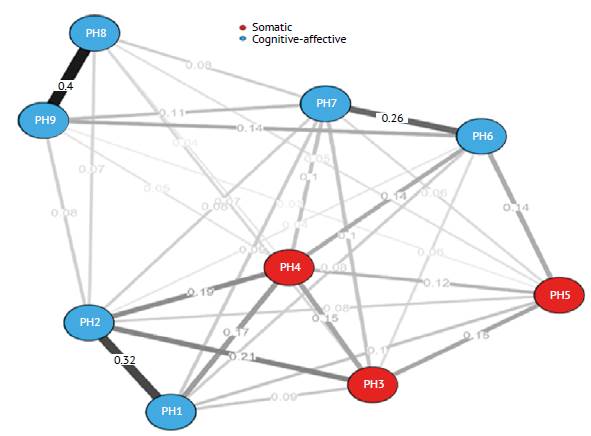
Source: Elaboration based on Ramos-Vera et al.22
Figure 1 Network analysis based on data obtained using the Patient Health Questionnaire-9 (PHQ-9) in Peruvian adults with hypertension.
In conclusion, network analysis offers a valuable methodological and practical contribution to medical research by allowing clinicians to interpret and analyze information derived from their research, which is reflected in comprehensive care; in other words, this approach allows for a concrete intervention based on the results obtained through the analysis of complex model systems.23 Furthermore, the latter models provide a better explanation of how various health conditions function when they are impacted by multiple diseases and situations, such as the COVID-19 pandemic, as well as the etiological mechanisms that interact with each other.