Training in clinical psychology usually involves intensive theoretical and practical work, guided by a clinical supervisor, and it is broadly acknowledged as a stressful experience (e.g., Cartwright & Gardner, 2016; Kaeding et al., 2017; Volpe et al., 2014). Specifically, clinical psychology trainees often face stressors such as dealing with patients' suffering, lack of time, financial issues, self-doubts, poor supervision, academic and research workload, perceived competition between colleagues, or attending to patients with severe suicidal ideation (e.g., Cushway, 1992; El-Ghoroury, Galper, Sawaqdeh, & Bufka, 2012; Galvin & Smith, 2015; Hill, Sullivan, Knox, & Schlosser, 2007). Accordingly, some studies have found that clinical psychology trainees usually experience higher levels of emotional symptoms than the rest of the staff (e.g., Cushway & Tyler, 1994; Vredenburgh, Carlozzi, & Stein, 1999). Indeed, recent systematic reviews have found high levels of burnout among psychotherapists, with trainees showing the highest rates (McCormack, Maclntyre, O'Shea, Herring, & Campbell, 2018; Simionato & Simpson, 2018). Importantly, this is not only relevant at a personal level because research has shown that personal distress can affect training and practice among clinical psychologists (e.g., Guy, Poelstra, & Stark, 1989).
Although the results found in the literature are consistent in showing the emotional difficulties faced by clinical psychology trainees, most of the studies have used qualitative and cross-sectional survey methods (e.g., Cushway, 1992; Cushway & Tyler, 1994; Galvin & Smith, 2015; Kaeding et al., 2017; Kuyken, Peters, Power & Lavender, 1998; Vredenburgh et al., 1999). Longitudinal studies are important with clinical psychology trainees and, generally, with clinical psychologists (McCormack et al., 2018), because cross-sectional studies only show a static picture of the situation. The high level of emotional symptoms found in cross-sectional studies among clinical psychology trainees might be due to several reasons. For instance, it could be that undergraduate or graduate psychologists experiencing higher levels of emotional symptoms select clinical psychology as a self-help strategy. In this case, the higher levels of emotional symptoms shown by trainees compared with senior clinical psychologists would not seem especially problematic, as it would imply that, during their careers, clinical psychologists would be generally successful in self-helping themselves. Conversely, it could be that novice clinical psychology trainees show increases of emotional symptoms when facing the new stressors of their role. In this case, the higher levels of emotional symptoms would be of greater concern than in the previous example because they could be an important barrier to clinical psychology training (McCormack et al., 2018).
Some studies have been conducted in clinical psychology trainees exploring the effect of different psychological interventions to help them to cope in a more effective way with stressors (e.g., Dereix-Calonge, Ruiz, Sierra, Peña-Vargas, & Ramírez, 2019; Pakenham, 2015; Rudaz, Twohig, Ong, & Levin, 2017; Stafford-Brown & Pakenham, 2012). However, these studies were not designed to analyze the evolution of emotional difficulties in trainees; therefore, it is difficult to extract conclusions from them. To our knowledge, only the study by Kuyken, Peters, Power, Lavender, and Rabe-Hesketh (2000) longitudinally analysed the experience of clinical psychology trainees for a long time. A sample of 167 trainees in the United Kingdom was followed for one year of their three years of clinical training. The results showed that, between the first and second year, trainees experienced significant increases in work adjustment problems, depression, and interpersonal conflicts. Also, a sub-group of trainees reported relevant difficulties on at least one dimension of adaptation, which were enduring over time. The most frequent adaptation problems in this sub-group were internalizing symptoms and work adjustment. These problems were found at higher rates than in a large standardized sample of employed adults.
Overall, the longitudinal study by Kuyken et al. (2000) shows greater support for the hypothesis that novice clinical psychology trainees experience increases in emotional symptoms when facing the new stressors of their role. This suggests that paying more attention to the experience of novice clinical psychology trainees throughout time is merited. However, the study by Kuyken et al. did not compare the evolution of novice clinical psychology trainees with that of a control cohort of psychology students or graduates. This would be a better comparison of the evolution of emotional difficulties experienced by novice clinical psychology trainees. Accordingly, the aim of the current study is to analyse the evolution of emotional symptoms in novice clinical psychology trainees during the first months of training compared to a control cohort.
Method
Participants
The sample consisted of 575 Psychology undergraduates (mean age = 22.62, SD = 3.70, age range = 19 to 55; 83.7% were women) from a Colombian university. Approximately half of the participants were studying the 9th semester (52.9%) and the other half were studying the 8th semester (47.1%). Unlike other countries (e.g., USA), Colombian laws permit undergraduates in Psychology to receive training in clinical psychology and to attend to clients with the guidance of a supervisor. Participants in the 9th semester were at the beginning of their clinical practice, whereas those in the 8th semester were in a regular semester and were studying some courses in clinical psychology. Almost all participants were single (93.9%). Forty-four percent of participants had received some kind of psychological or psychiatric treatment in the past, but only 5.6% were receiving treatment when the study was conducted (only 1.8% were taking psychotropic medication). A raffle of five books on clinical psychology was carried out at the end of the study to compensate the participants who finished the study.
Instruments
Depression, Anxiety, and Stress Scales - 21 (DASS-21; Lovibond & Lovibond, 1995). The DASS-21 is a 21-item, 4-point Likert-type scale (3 = applied to me very much, or most of the time; 0 = did not apply to me at all) consisting of sentences describing negative emotional states. It contains three subscales (Depression, Anxiety, and Stress) and has shown good internal consistency and convergent and discriminant validity. Scores in each subscale range from 0 to 21 points. The DASS-21 has shown good psychometric properties in Colombia (Ruiz, García-Martín, Suárez-Falcón, & Odriozola-González, 2017). The alphas of the complete DASS-21 were 0.91 and 0.93 for Time 1 (T1) and Time 2 (T2), respectively. With respect to the DASS-21 subscales, the alphas were 0.87 and 0.90 for Depression, 0.75 and 0.81 for Anxiety, and 0.82 and 0.84 for Stress for T1 and T2, respectively.
General Health Questionnaire - 12 (Goldberg & Williams, 1988). The GHQ-12 is a 12-item, 4-point Likert-type scale that is frequently used as screening for psychological disorders. Respondents are asked to indicate the degree to which they have recently experienced a range of common symptoms of distress, with higher scores reflecting greater levels of psychological distress. The GHQ-12 has shown excellent psychometric properties in Colombia (Ruiz, García-Beltrán, & Suárez-Falcón, 2017). The alpha of the GHQ-12 in this study was 0.86 and 0.88 for T1 and T2, respectively.
Procedure
The procedure of this study was approved by the institutional Ethics Committee. Potential participants were invited to participate in the study in a regular class at the beginning of the academic semester. Students were told that participation was voluntary and that the aim of the study was to analyze which psychological variables were associated with the psychological adjustment of clinical psychology trainees. Students who agreed to participate in the study signed an informed consent. Subsequently, they were given a questionnaire package including a sociodemographic form and the DASS-21 and GHQ-12. This assessment served as T1. The second assessment (T2) was conducted after 2 months, in the middle of the semester, in a week free of exams in order to avoid evaluating on academically stressful days. In this case, participants were contacted through email and were invited to respond to the DASS-21 and GHQ-12 through the website www.typeform.com. The link with the survey was active for only one week. This assessment was conducted in the middle of the semester, just after the first evaluation week.
Data analysis
Prior to conducting the data analyses, all variables were explored for accuracy of data entry and missing values. There were 22 missing values at T1, which represented only 0.12% of data points. No missing data were found at T2 because the instruments were applied through the web application. Missing data points in the items of the scales were estimated using the participant's mean score for the specific scale.
Bayesian data analyses were conducted in this study with the free software JASP 0.9.0.1 (https://jasp-stats.org/). JASP provides a graphical interface of the R package BayesFactor, which permits the computation of Bayes factors in standard designs (e.g., t-tests, ANOVA, regression, etc.). Bayes factor (BF) quantifies the relative evidence in the data, expressed as relative odds, for the null or the alternative hypotheses. The BF can also be seen as the extent to which a rational person should adjust his or her beliefs in favour of the most supported hypothesis according to the data, where a BF > 1 means that the data support the alternative hypothesis and a BF < 1 that the data support the null hypothesis. Bayes factors can be interpreted according to the guidelines provided by Jeffreys (1961): 1 = No evidence for the alternative hypothesis; 1-3 = Anecdotal evidence for the alternative hypothesis; 3-10 = Substantial evidence for the alternative hypothesis; 10-30 = Strong evidence for the alternative hypothesis; 30-100 = Very strong evidence for the alternative hypothesis; and >100 = Extreme evidence for the alternative hypothesis (note that BFs < 1 are interpreted in the same way, but favour the null hypothesis).
According to Rouder, Morey, Verhagen, Swagman, and Wagenmakers (2017), there are at least two pragmatic advantages of Bayesian analyses. Firstly, Bayes factors are a symmetrical measure of evidence and, thus, they can provide evidence for the null hypothesis in the same way as for the alternative hypothesis (Dienes, 2016). This contrasts favorably with frequentist statistics based on p-values, which can lead to supporting the alternative hypothesis when the sample size is very large, as in this study, even when the effect size is small and trivial (Morey & Rouder, 2011). Secondly, Bayes factors permit researchers to provide a graded measure of evidence for different models and not make dichotomous reject and fail-to-reject decisions.
Bayesian statistics include prior expectations of the parameters. These prior expectations are expressed by prior distributions that receive high density at plausible parameter values and low density at implausible parameter values (Lee, 2004). Prior distributions can be determined based on previous research, expert knowledge, scale boundaries, and statistical considerations (Wagenmakers et al., 2018).
Firstly, we explored whether there were differences between participants who responded to the survey at T2 and those who did not. For continuous variables, we computed JZS Bayesian independent t-tests (Rouder, Speckman, Sun, Morey, & Iverson, 2009). The JZS independent t-test suggests Cauchy prior distributions in which the effect size of the factor, termed δ, is located at 0, and the researcher can modify the parameter r that represents the width of the distribution (higher values of r place more density at higher effect sizes). The default value of r was used (r = 0.707). For nominal variables, we computed Bayesian multinomial tests (Gunel & Dickey, 1974) with a default prior concentration of 1. Secondly, JZS Bayesian independent t-tests with the same prior distribution as above were conducted to analyse the differences in emotional symptoms between participants in the 8th and 9th semester.
Lastly, Bayesian two-way repeated-measure ANOVAs were conducted to analyze the effect of the factors Time (T1 versus T2) and Semester (8th without clinical practice versus 9th with clinical practice) on emotional symptoms. Five models were compared in the conducted Bayesian ANOVAs: (a) the null model (factors do not affect the dependent variable), (b) the model with only Time affecting the dependent variable, (c) the model with Semester affecting the dependent variable, (d) the model with both factors affecting the dependent variable (Time + Semester), and (e) the model with both factors and their interaction affecting the dependent variable (Time + Semester + Time*Semester). All models were given the same prior probability (i.e., 0.200).
The Bayesian ANOVA framework advocated by Rouder et al. (2017) suggests Cauchy prior distributions in which the effect size of the factor, termed 6, is located at 0, and the researcher can modify the parameter r between the recommended values of 0.2 to 1.0 that represents the width of the distribution (higher values of r put more density at higher effect sizes). As differences in emotional symptoms across Time and Semester were expected to be relatively small, we selected r = 0.35 as the width for the prior distributions. However, we also conducted a Bayesian sensitivity analysis that investigated the robustness of the results with r values of 0.2 and 0.5, which posit higher density in the Cauchy distribution at, respectively, lower and higher effect sizes. Conducting sensitivity analyses is frequently suggested by Bayesian statisticians to investigate whether the results obtained are excessively dependent on the selected prior distribution (Gelman et al., 2014).
Results
Equivalence between completers and non-completers
Of the 575 participants who responded at T1, 367 responded at T2 (i.e., 63.8% of participants finished the study). Table 1 shows the descriptive data for participants who responded at T2 and participants who did not in continuous variables. All Bayes factors supported the null hypothesis of no differences between completer and non-completer participants. Regarding dichotomous variables, Bayes factors were 0.34, 3.27, 0.06, and 0.01 for gender, past psychological/psychiatric treatment, current psychological/ psychiatric treatment, and psychotropic medication, respectively. Accordingly, completers and non-completers did not differ in sociodemographic variables and emotional symptoms. The only exception was the variable past psychological/psychiatric treatment, in which a lower proportion of participants who had a history of treatment responded to the assessment at T2 (51.6% versus 62.9% of participants who did not state a history of treatment).
Equivalence of participants at T1
Table 2 shows the descriptive data for completers at T1 in emotional symptoms. All Bayes factors supported the null hypothesis of no differences between participants in the 8th and 9th semester at T1.
Evolution of emotional symptoms
Figure 1 depicts the completers' scores on emotional symptoms at T1 and T2 for the five variables considered (i.e., DASS-Total, DASS-Depression, DASS-Anxiety, DASS-Stress, and GHQ-12). Overall, the scores in emotional symptoms at T1 were equivalent across semesters. However, clinical psychology trainees (i.e., participants in the 9th semester) showed higher increases in symptoms from T1 to T2 than participants in a regular semester (i.e., participants in the 8th semester).
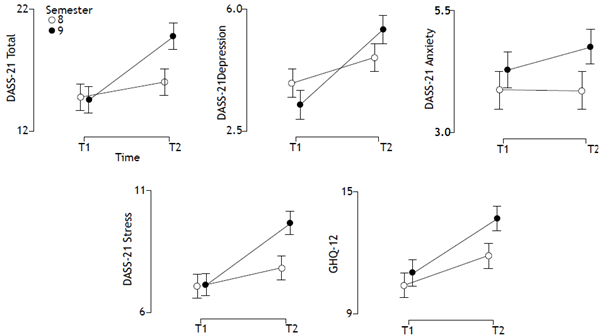
Figure 1 Evolution of symptoms in participants from 8th semester (control cohort) and 9th semester (clinical psychology trainees). Error bars represent the 95% credible intervals.
Table 3 shows the results of the Bayesian two-way repeated-measures ANOVAs conducted. As previously stated, all models were given the same prior probability (i.e., 0.200). In the table, the column "P(M|data)" shows the updated probabilities after having observed the data, the column "BFM" shows the degree to which the data have changed the prior model odds, and the column "BF" shows the Bayes factors associated with each model.
Table 3 Results of the Bayesian Repeated Measure ANOVA on the Total Score of the DASS-21
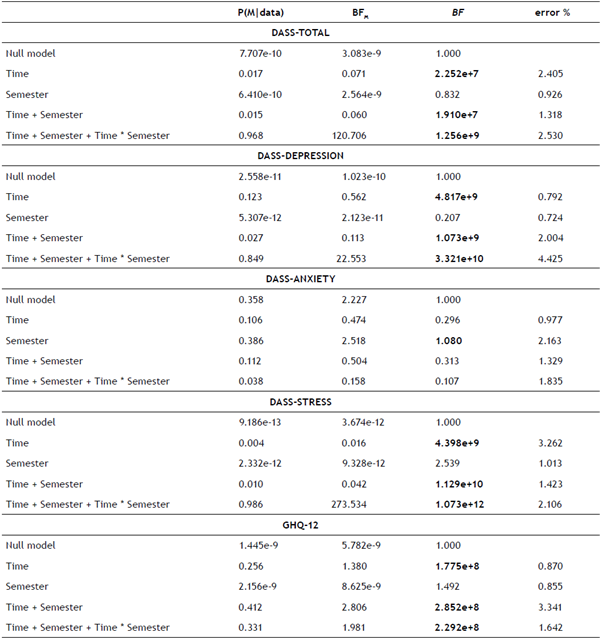
Note. BF = Bayes factors; BFM = degree to which the data have changed the prior model odds; P(M|data) = updated probabilities after having observed the data.
Regarding the DASS-Total scores, the fifth model with both Time and Semester and their interaction affecting the scores was clearly superior to the remaining models. The updated probability of this model was 0.968, and the data changed the prior model odds by 120.706. The BF indicated that there was overwhelming evidence favoring this model. When comparing with the second best model (Model 2: only Time affecting the DASS-Total scores), the BF obtained was 498.413 (this value is obtained by dividing the BF of the first best model by the second best model), which indicated that there was overwhelming evidence favoring the fifth model over the second one.
With respect to the scores on the Depression subscale, the fifth model (Time + Semester + Time*Semester) was also the best one. The updated probability of this model was 0.849, and the data changed the prior model odds by 22.553. The BF indicated that there was overwhelming evidence favoring this model. When comparing with the second best model (Model 2: only Time affecting the DASS-Total scores), the BF obtained was 6.894, which indicated that there was substantial evidence favoring the fifth model over the second one.
Regarding the scores on the Anxiety subscale, the third model (Semester) was the best one, although it was not shown to be a particularly good model. The updated probability of this model was 0.386, and the data changed the prior model odds by 2.518. The BF was only of 1.080, which indicated that there was only anecdotal evidence favoring this model over the null model.
Table 3 also shows that the fifth model (i.e., Time + Semester + Time*Semester) was the most appropriate to explain the scores on the Stress subscale. The updated probability of this model was 0.986, and the data changed the prior model odds by 273.534. The BF indicated that there was overwhelming evidence favoring this model. When comparing with the second best model (Model 4: Time + Semester), the BF obtained was 950.399, which indicated that there was overwhelming evidence favoring the fifth model over the fourth one.
Lastly, the results were unclear to explain the scores on the GHQ-12 because Model 2 (i.e., Time), Model 4 (i.e., Time + Semester), and Model 5 (Time + Semester + Time*Semester) obtained similar BF scores. Specifically, with the prior distribution chosen (r = 0.35), the fourth model was the most appropriate.
The results of the sensitivity analysis conducted can be seen at https://osf.io/m3rwb/. Overall, the results did not change significantly with alternative prior distributions. This indicates that the results obtained are robust under the different reasonable prior distributions.
Discussion
Research has shown that clinical psychologists tend to present high levels of emotional symptoms and emotional exhaustion (e.g., McCormack et al., 2018; Radeke & Mahoney, 2000; Simionato & Simpson, 2018). Among them, clinical psychology trainees usually show higher levels of emotional symptoms than the rest of the psychology students (Vredenburgh et al., 1999). Accordingly, some studies have analyzed the effect of psychological interventions on reducing emotional symptoms and promoting wellbeing in clinical psychology trainees (e.g., Dereix-Calonge et al., 2019; Pakenham, 2015; Rudaz et al., 2017; Stafford-Brown & Pakenham, 2012).
Although the evidence is consistent in showing the emotional difficulties of clinical psychology trainees, to our best knowledge, there is no evidence of the longitudinal increase of emotional symptoms among trainees compared to a control cohort. This is a relevant limitation of the empirical evidence collected so far because the high level of emotional symptoms found in cross-sectional studies might be due to alternative reasons such as the tendency of selecting clinical psychology as a self-help strategy (McCormack et al., 2018). Accordingly, the current study conducted a 2-month, longitudinal analysis with a large sample size to compare the emotional symptoms presented by novice clinical psychology trainees with those of a control cohort.
The results obtained showed that there were no differences in emotional symptoms between the two groups at T1 (i.e., at the beginning of the semester, just before initiating the clinical practice). Bayesian repeated measures ANOVA showed that, after two months (T2), the data strongly support the hypothesis that clinical psychology trainees show higher increases in emotional symptoms than the control cohort for the DASS-Total, DASS-Depression, and DASS-Stress. Contrarily, there was no interaction effect for DASS-Anxiety, and only anecdotal one for the GHQ-12 scores.
Some limitations of the current study are worth mentioning. Firstly, the sample of this study consisted of undergraduate clinical psychology trainees. However, in many countries, training in clinical psychology is only permitted at postgraduate level, which might complicate the generalizability of the current findings. Accordingly, further studies might replicate the results presented with clinical psychology trainees at a postgraduate level. Secondly, the fact that the sample of the current study was recruited from only one university can also hinder the generalizability of the results. Thirdly, only 63.8% (N = 367) of the sample who responded to the questionnaire package at T1 also responded at T2. However, there was no evidence that completers were different from non-completers according to the Bayesian t-tests conducted. Fourthly, this longitudinal study does not allow for attributions of causality because no independent variable was manipulated. However, note that longitudinal studies such as this can be the only way to explore the research question presented in this study because manipulating the independent variable (i.e., assigning participants to the semester with clinical psychology practice versus the control semester) is not possible and would be unethical. Lastly, the current study did not explore which psychological variables (e.g., coping styles, experiential avoidance, self-efficacy, repetitive negative thinking, etc.) might moderate and/or mediate the increase of emotional symptoms in clinical psychology trainees compared to the control cohort. In this sense, the study conducted in a similar sample of trainees by Dereix-Calonge, Ruiz, Cardona-Betancourt, and Flórez (in press) found that repetitive negative thinking (RNT) focused on the clinical practice longitudinally predicted the increase of emotional symptoms. However, this study did not recruit a control cohort. Accordingly, future studies might analyze the role of RNT focused on the clinical practice as a moderator and mediator of the differential increase in emotional symptoms observed in clinical psychology trainees compared with a control cohort.
The current study is the first one showing that novice clinical psychology trainees tend to experience an increase in emotional symptoms in the first months of clinical practice as compared with a control cohort. This indicates that training programs in clinical psychology should address the emotional difficulties and barriers in learning found by trainees in a more detailed way. According to Luciano, Ruiz, Gil-Luciano, and Ruiz-Sánchez (2016), this difficulty should be addressed in the process of the clinical supervision because it is the context in which the emotional barriers of a therapist usually emerge and can be identified. In this sense, the results of the current study call for developing models of clinical supervision that integrate a therapist's barriers as an essential part of the work.