Smartphones have extended connecting with others, including friends and relatives, in an easy manner. These devices have been used as a tool for enhancing and maintaining social connections (Chan, 2015). However, the use of smartphones may have negative consequences including adverse effects on mental and physical health (Lee et al., 2014), reduced face-to-face interactions and worthless time spent with friends (Rotondi et al., 2017), and promotes a set of detrimental effects in terms of disruptive behaviours in social interactions and interpersonal relationships (Dwyer et al., 2018). Phubbing is a blend of the words “phone” and “snubbing” and it is understood as “the act of snubbing someone in a social setting by looking at your smartphone instead of paying attention” (Chotpitayasunondh & Douglas, 2018; Karadağ et al., 2015). This may harm social interactions and relationships, including romantic relationships (Roberts & David, 2016), parent-child interactions in family contexts (Xiao & Zheng, 2022), and boss-employee interactions in workplace situations (Roberts & David, 2020). Phubbing has been associated with aspects of the social and personal sphere of individuals including lower perceived quality of social interactions and relationships (Vanden Abeele & Postma-Nilsenova, 2018), negatively affecting impression formation processes (Vanden Abeele et al., 2016), increased romantic relationships dissatisfaction (Roberts & David, 2016), and poorer mental health (Ergün et al., 2023). Researchers investigating this phenomenon have also studied the relationship between phubbing and smartphone addiction and other behavioural addictions (Guazzini et al., 2019). For instance, phubbing has been associated with experiencing an inability to regulate mobile phone use appropriately as a result of a compulsive search for satisfying events (Chotpitayasunondh & Douglas, 2016). This may aggravate the use of smartphones leading to a sense of exclusion from the social environment. A phubber may experience an inability to control the use of their mobile phones (Chotpitayasunondh & Douglas, 2016), therefore phubbing aggravates a phubber’s smartphone use increasing the sense of exclusion from the social environment. Additionally, phubbing has been related to internet addiction (Ergün et al., 2023) (i.e., a maladaptive pattern of internet use) and to fear of missing out (FoMO) (Al-Saggaf, 2021; Ansari et al., 2024). FoMO is described as “a pervasive apprehension that others might be having rewarding experiences from which one is absent” (Przybylski et al., 2013) and this feeling is characterised by the desire to stay continually connected with what others are doing (Gugushvili et al., 2020). Both, internet addiction and FoMO, have been associated with smartphone addiction (Ben-Yehuda et al., 2016), although it is still under debate whether smartphone addiction is a form of internet addiction as it acts as a facilitator that makes it possible to connect anytime, anywhere (Barrault et al., 2019) or if they differ in terms of addiction-risk factors (Jin Jeong et al., 2020). Nevertheless, these phenomena seem to contribute to phubbing behaviours (Chotpitayasunondh & Douglas, 2016).
As phubbing is still in its early infancy, few instruments have been developed to evaluate phubbing behaviours. Measures intended to assess phubbing behaviours include the Perceived Social Norms of Phubbing Scale (Chotpitayasunondh & Douglas, 2016), the Technology Device Interference Scale and the Technology Interference in Life Examples Scale (McDaniel & Coyne, 2016). However, the psychometric properties of these instruments have not been deeply analysed beyond internal consistency and factor loadings and do not assess proper indicators of phubbing behaviours (Chotpitayasunondh & Douglas, 2016).
Karadağ et al. (2015) created the 10-item Phubbing Scale aiming to assess phubbing through two different factors (phone obsession and communication disturbances). Although the original Phubbing Scale by Karadağ et al. (2015) lacks a thorough psychometric study including different measures of validity and test-retest reliability, a small number of language scale adaptations (Blanca & Bendayan, 2018; García-Castro et al., 2022) and a recent cross-country study (20 countries) better analysed psychometric properties and measured invariance (Błachnio et al., 2021) of the Karadağ et al. (2015) scale. However, the Phubbing Scale still contains several items that seem to represent general phone addiction rather than phubbing behaviours (e.g., “My mobile phone use increases day by day”). In this sense, additional instruments that screen the possible phubbing behaviours are required. In this regard, the Generic Scale of Phubbing (GSP), developed by Chotpitayasunondh and Douglas (2018), exemplifies a multidimensional and generalisable scale composed of 15 items with four factors: nomophobia, the fear or worry at the idea of being without a mobile phone or unable to use it; interpersonal conflict, a form of conflict between oneself and others; self-isolation, understood as escaping from social activities and avoiding contact with other people; and problem acknowledgement, relating to the idea that the individual is facing a phubbing problem. These factors were not previously revealed in other instruments, however latter studies have related them to phubbing behaviours (Tomczyk & Lizde, 2022).
To our knowledge, there have been limited adaptations and validations of the GSP (Generalised Problematic Internet Use Scale) in different language and cultural contexts. Notably, adaptations in Turkish and Lebanese contexts have confirmed the four-factor structure of the original GSP (Bitar et al., 2022; Yam & Kumcağız, 2020). Additionally, a shorter version of the scale, consisting of 12 items while retaining the original four factors, has been adapted to the Chinese context (Li, 2023).
Method
The goal of the present study is to adapt the GSP to the Spanish language in a European Spanish context and to analyse its psychometric properties, including the factor structure following the international guidelines for adapting tools across different cultures (Hambleton & Zenisky, 2011; Muñiz et al., 2013), among a sample of the general Spanish population. The database, questionnaire, and R code used for this study can be found at https://osf.io/7tvd9/?view_only=263d2c0f47e8424fa63a0a28ada30e93
Procedure
A sample of smartphone users from the general Spanish population were recruited using a non-probabilistic sampling method. Despite attempting to recruit participants from the general population, there is an over-representation of undergraduate students in our sample. An online questionnaire was advertised on notes posted on community and university bulletin boards, social networking sites, civic associations, and by word of mouth. Data-collection was performed using an online tool and the inclusion criteria were as follows: (1) 18 years of age or over, (2) Spanish nationality, (3) being a native Spanish speaker, and (4) intentionally using a smartphone or the internet at least once a day. Participants signed an electronic informed consent after being provided explanations regarding the nature of the study and then responded to a set of sociodemographic questions and a battery of instruments whose completion took approximately 15 minutes. The study protocol was approved by the Bioethics Committee of the University of Extremadura (code 52/2022).
Participants
We collected 359 responses to our online survey between April and May 2022. Thirteen participants were excluded because they were not 18 years old or older (8) or their native language was not Spanish (5). The final sample was composed of 346 (Mage = 21.25, SD = 7.10; 66.18% women) participants. Most participants reported using their phone (for habitual activities such us calls, games, messages, social networking sites, etc.) for two to three hours (20.23%), three to four hours (22.83%), for four to five hours (17.05%) or for five to six hours (12.43%).
Measures
Sociodemographic Information. We collected information regarding participants’ age, gender, marital status, education, and employment status (see Table S1 in Supplementary Materials).
Phubbing. We measured phubbing using the Generic Scale of Phubbing (Chotpitayasunondh & Douglas, 2018). This scale includes 15 items that assess phubbing behaviour in four sub-dimensions: nomophobia (NP), interpersonal Conflict (IC), self-isolation (SI), and problem acknowledgement (PA). This scale has a 7-point Likert scale ranging from 1 (never) to 7 (always). The original scale’s study reported adequate internal consistency of the scale’s subdimensions: Cronbach’s alphas ranged from .82 to 92. The scores for each subscale are aggregated.
In this study, the original version of the GSP (Chotpitayasunondh & Douglas, 2018) was translated and back-translated into Spanish following Hambleton and Zenisky’s (2011) recommendation for the translation and adaptation of psychological instruments established by the International Test Commission (Muñiz et al., 2013). We composed a research team of different psychology experts from different fields (psychological assessment, psychometry, and social psychology).
The team prepared the material, including the items and instructions, that two experts translated, both experts were bilingual university professors, one an expert in English grammar and the other in personality and assessment in psychology. Then, the material was back-translated by bilingual psychologists living in Spain whose native language was English and who were not engaged in the first step of the translation. Finally, the research team examined every item and instruction for a better adequacy, understandability, and clarity. Authorisation from the original GSP authors was obtained to adapt the scale to a Spanish context.
Internet Addiction. We measured this variable using the 12-item version (IAT-12) adapted to the Spanish population (Pino et al., 2022) with a six-point Likert scale (0 = never to 5 = always). This scale is composed by two factors: Control and time management problems and Salience and neglect of social life. The first factor refers to a deficient self-regulation in the context of internet use, while the second addresses the situation when the activity takes precedence over crucial life responsibilities and in negligence of important life activities. The IAT-12 Spanish version reported good internal consistency for both the whole questionnaire and the subscales, Cronbach’s alphas coefficient ranging from .80 to .87 (Pino et al., 2022). In this study we found good internal consistency indicators for the overall scale (a = .90, who = .92), Control and time management problems (a = .87, w = .87), and Salience and neglect of social life (a = .85; w = .85).
Smartphone Addiction. This variable was measured using the Spanish short version of the Smartphone Addiction Scale (SAS-SV; López-Fernández, 2017) and evaluates a possible maladaptive mobile phone use (loss of control, disruption of family or schooling, disregard for consequences, withdrawal, preoccupation and tolerance) This measure is composed of ten items with a 6-point Likert response format from 1 (strongly disagree) to 6 (strongly agree). As in the Spanish adaptation study (a = .88), the scale cohered well (a = .88; w = .88).
Fear of Missing Out. We measured this construct through the Spanish Fear of Missing Out Scale (FoMO) (Gil et al., 2015). This scale is composed of ten items with a Likert response format from 1 (nothing) to 5 (a lot). Both the original (a = .87 to .90) and the Spanish version (a = .85) showed good internal consistency. Cronbach’s alpha in this study was .85 and the omega was .86.
Analytical strategy and results
We decided to carry out a confirmatory factor analysis. This is mainly because we have a strong hypothesis regarding the number of factors in the scale and the item-construct relation in the scale, albeit in other contexts, has been previously established (Brown, 2015). We expected to replicate the factorial structure found in previous studies using the scale (Bitar et al., 2022; Chotpitayasunondh & Douglas, 2018; Ergün et al., 2020; Li, 2022; Yam & Kumcağız, 2020).
All the analyses were performed in R programme (version 4.1.3) (R Core Team, 2022). First, we obtained descriptive statistics for each item and examined both the adequacy of the sample and the data for factor analysis. Some skewness and kurtosis values were higher than 2 and 7, respectively (see Table 1). Anderson-Darling test results showed that the univariate normality assumption was violated across all the items (p < .001). As expected, both Mardia (Skewness = 3375.4, p < .001; Kurtosis = 49.26, p < .001) and Henze-Zirkler’s (HZ = 1.74, p < .001) tests indicated that we are dealing with a non-normally multivariate data. The lack of univariate and multivariate normality can lead to inference problems, especially when using Maximum Likelihood (ML) as an estimator method. In our analyses we used a robust variant of ML, the Maximum Likelihood with the Satorra-Bentler correction (Satorra & Bentler, 1994) and robust standard errors (MLM). Robust versions of ML are robust to non-normality (Brown, 2015) and MLM has shown better results when calculating fit indices such as RMSEA (Gao et al., 2020).
To assess the model fit to the data, we calculated the chi-square (X2) test, comparative fit index (CFI), the Tucker-Lewis index (TLI), the root means square error of approximation (RMSEA) with a 90% confidence interval, and the standardised root mean square residual (SRMR). Different authors have proposed different guidelines for interpreting these statistics. Hu and Bentler (1999) proposed a cut-off value of .95 for CFI and TLI, .08 for SRMR and .06 for RMSEA. Other studies have suggested that RMSEA values lower than 0.8 indicate acceptable fit (Browne & Cudeck, 1992) or that CFI and TLI values higher than .90 indicate good fit (Bentler, 1990). However, strict adherence to these guidelines may lead to erroneous conclusions (Marsh et al., 2004).
We fitted different models using the lavaan package (Rosseel, 2012). First, we fitted the four-factor model with a second order factor proposed in the original version (see Table 2). We modified the model to obtain a better fit to the data. We observed the modification indices and the standardised expected parameter change. Then we built other models (Models 2 to 4 on Table 2) including a covariance path at a time. We decided to keep Model 4, presented in Figure 1. In this model, all factor loadings were higher than .60.
We calculated the Average Variance Extracted (AVE), composite reliability of the items, and the shared variance of the constructs. In particular, we used the coefficient omega higher order (who) for the second order factor and the omega (w) coefficient for the subscales (Flora, 2020). As shown on Table 3, AVE’s values, a measure of convergent validity, were greater than .5 for all factors meaning that each construct explains, on average, more than a half of the variance of its indicators and it is considered acceptable (Hair et al., 2022). Indicators of construct reliability ranged from .77 to .81 for the subscales and the reliability was also acceptable for the high order factor (who = .77). The four-factor structure for the GSP is also supported in terms of internal consistency (see Table 3). Values found were acceptable and similar to those reported by the original authors of the GSP for the four dimensions. The lowest value was for the dimension of Problem Acknowledge (a = .78), and the highest for the dimension of Nomophobia (a = .84), both fairly similar to those obtained by the original GSP authors (2018) (a = .82 and a = .84 respectively). Regarding discriminant validity, no factor intercorrelation exceeded .80, ranging from .28 to .58 (see Table 4). Factor intercorrelations above .80 could mean lack of discriminant validity (Brown, 2015). We used the Fornell-Larcker criterion (Fornell & Larcker, 1981) to assess discriminant validity. In every case, the square root of the AVE scores were larger than the highest correlation of the construct with the other one, indicating discriminant validity.
Table 4 Correlation among variables
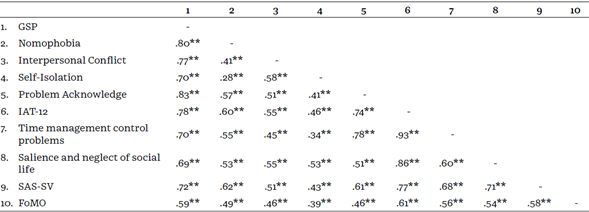
Note: IAT-12 = 12-item Internet Addiction Test; SAS-SV = Smartphone Addiction Scale; FoMO = Fear of Missing Out Scale. ** p < .001
Validity evidence based on the relationships with other variables was tested. We found a pattern of correlations with other variables similar to that of the original study (see Table 4). All specific dimensions and the general factor of phubbing were related to smartphone addiction with a medium to large magnitude of the coefficient effects. We also found medium to large correlation coefficients among the IAT-12, as well as its dimensions, and the GSP and its factors. Finally, we identified the pattern of correlations between the overall GSP score and its dimensions and FoMO’s scores.
Discussion
This study provides support for the factorial structure of the Generic Scale of Phubbing (Chotpitayasunondh & Douglas, 2018) in a Spanish sample. Overall, our results suggest that the Spanish version of the GSP is a valid and reliable instrument to study phubbing among Spanish individuals (see supplementary material for a full version of the instrument). First, we found the same four factor structure as the pioneer authors did, that empirically tested the instrument in an English-speaking sample, as well as in other adaptations (Bitar et al., 2022; Li, 2022; Yam & Kumcağız, 2020). The Spanish version of the GSP, like the original, provides a four-factor model which is useful to capture such a multifaceted construct as phubbing in comparison with two-factor measurement models (for example Karadağ’s Phubbing Scale). A general factor captures the commonality shared by the common content of all items, and the four factors represent the unique contribution of individual domains, beyond the unique factor. In this sense, the results of this study support the unidimensional use of the scale, as well as the four-factor use.
No original item was removed due to lacking significance or low discrimination index. The theoretical structure of item-factor relationships was fully replicated in our study. In addition, the magnitude of the correlations between the general and the specific factors of the Spanish version of the GSP and related constructs were medium-high, positive, and significant. These provide sufficient certainty regarding its uses and the scoring interpretation of the Spanish version of the GSP results. These results are in line with the development of the original scale and the Turkish adaptation. In general, the correlations between the general factor of the Spanish version of the GSP and other related constructs evidenced that phubbing is related to internet addiction, smartphone addiction and FoMo (Al-Saggaf, 2021; Chotpitayasunondh & Douglas, 2016; Guazzini et al., 2019; Karadağ et al., 2015). Finally, the specific factors of the Spanish version of the GSP were also strongly correlated with the aforementioned constructs in the expected direction.
Despite the relevant findings, this study is not without limitations. On one hand, this paper presents only a confirmatory study, whereas adaptations typically include both an exploratory and a confirmatory study. We believe this approach is justified because we had a priori hypotheses regarding the instrument’s behaviour based on previous research (Chotpitayasunondh & Douglas, 2018). On the other hand, participants were not selected using a random sampling strategy, therefore the generalisability of results is restricted. Moreover, the sample representation in terms of education, gender and age is unbalanced. Although there is an overrepresentation of young people, this is relevant due the fact that young people are more at risk of engaging in phubbing behaviours as they engage more in using the internet and smartphone compared to adults (Yu & Sussman, 2020). Future adaptations of the GSP may ensure balanced samples to rule out the possible implications of these variables. Even though the sample is similar to the original scale development and our scale included at least five participants per parameter (Gorsuch, 1983), testing the original and adaptations of the GSP with larger and different kinds of populations (e.g., clinical, adolescents) would be crucial to better understand phubbing behaviours, and further cross-cultural adaptations in Spanish-speaking countries with different cultural and linguistic backgrounds would also be an asset to this instrument. Related to the above, it is important for future research with specifically designed samples to analyse the measurement invariance of the instrument. This means examining whether the instrument behaves consistently and measures the same construct across different groups (e.g., based on gender, age, ethnicity, socioeconomic status, etc.). Although the characteristics of our sample did not allow us to delve into this aspect, future research should address it.
Phubbing is an area of research that has not been developed substantially. The present findings support the suitability of the Spanish version of the GSP to evaluate this behaviour in general populations to better examine associations between phubbing and personality factors, cognitive processes, behavioural issues and/or psychosocial variables, and their potential impacts on mental health. This is important because, as mentioned in the introduction, other phubbing scales, although potentially interesting, combine several constructs in their measurement (e.g., phone addiction). This makes them conceptually less suited for capturing the specific construct of phubbing. The adaptations of this tool to different languages can facilitate the identification of cross-cultural differences which is essential for conducting reliable cross-cultural comparisons. This instrument can be used in an auto-administered manner and in large groups, and can be used by psychology researchers, epidemiologists, and social science researchers to assess phubbing prevalence, and by psychologist practitioners to assess pre- and post-intervention programmes aiming to reduce phubbing behaviours and to improve social interactions and interpersonal relationships. It also allows more insight for policymakers and mental health professionals to effectively implement programmes to detect at-risk individuals and reduce the associated consequences of phubbing behaviours not only for phubbers but also for those that may feel unintentionally snubbed1 2 3 4.