Introduction
On November 17, 2019, coronavirus infection (SARS-CoV-2) was recognised for the first time in Wuhan, China. After one month, 27 cases were reported on December 15 and 60 on December 20, and since then this disease known as COVID-19 has spread worldwide [1,2]. In this sense, the main variants of coronavirus in humans are the Middle East Respiratory Syndrome Coronavirus (MERS-CoV) and the Severe Acute Respiratory Syndrome Coronavirus (SARS-CoV-1) which, through severe infections, affect the respiratory system and may finally lead to a pandemic that causes a significant quantity of deaths [3-5]. In March 2020, the World Health Organisation (WHO) declared COVID-19 to be a pandemic and declared a public health emergency [5]. The main source of transmission of COVID 19 has so far been patients with pneumonia [6]. In addition, to the best of our knowledge, coronavirus infection does not yet have a specific treatment [3,7]. Notwithstanding, some treatments have included anti-inflammatories, bronchodilators, anticoagulants, antiparasitic, antimalarial, and antiviral drugs [1,8-12]. Nonetheless, the WHO stopped using the two antimalarial medications, chloroquine, and hydroxychloroquine, as a COVID-19 treatment due to their secondary pharmacological and toxicological effects [1,8,10-12]. Indeed, there are many, direct and indirect, ways in which these drugs could inhibit SARS-CoV-2, but one of them is cogitating that the drug is able to dock with a specific active site in the major protease (Mpro) of SARS-CoV-2, which has been identified as essential in the life cycle of this virus, including its replication [13].
It is commonly acknowledged that the process of discovering and developing novel drugs is time-consuming, dangerous, and expensive [14]. A quick and efficient way to find drugs that may be successful against SARS-CoV-2 is through virtual screening (VS), a computational modeling technique [15]. One of the most popular approaches in drug development is the use of big and chemically diverse compound libraries for computational and biological screening, which has encouraged the use of VS as a quick and affordable method for assessing a variety of compound collections [16]. Applications of machine learning in drug discovery and development are well highlighted [17] and analysis of the computational methods used for COVID-19 drug finding is critiqued [18]. Drug interactions with their receptors can be studied using molecular docking and molecular dynamics (MD) simulations [19]. Molecular docking enables accurate exploration of interactions between molecules and also prediction of how these molecules will fit together as well as analyses of conformational changes of particular molecules over time. MD simulations can be utilised independently [20] since, for drug repurposing, different computational studies have been carried out that target both the structural and non-structural proteins of SARS-CoV-2 [4,21-23]. An overview of molecular docking's principles and features are highlighted [24] and the main three types of software for molecular docking are listed and described [25].
Therefore, there is an urgent need to search for active and safe antiviral drugs with broad spectrum activity that can inhibit the major protease (Mpro) of SARS-CoV-2. Among the drugs proposed as COVID-19 antiviral agents, it is possible to consider the molecules present in medicinal plants as a potentially effective source of treatment [21] when considering that nature constitutes a potential reservoir of molecules with various purposes. They are being researched for potential application as a therapy alternative for a number of disorders [26]. Considering this in these works, the proposal is to study the interaction between natural compounds against the SARS-CoV-2 protease Mpro [27].
The primary goal of this research was to evaluate the effectiveness of various phytochemicals against COVID-19 using bioinformatics tools. In total, 129 natural compounds were docked into the SARS-CoV-2 Mpro active site (Code PDB: 6LU7) to find potential inhibitors of this virus. In tandem, docking scores were obtained to separate possible active molecules from inactive ones. A prediction of Lipinski's rule of five and ADMET parameters was then performed, and finally, a molecular dynamics study was conducted on the two best molecules derived from the docking studies to determine their interaction in the presence of water molecules and ions over a period of time, to evaluate their residence time in the active site.
Materials and methods
Phytochemicals
A total of 129 phytocompounds from 34 Algerian aromatic and medicinal plants were selected. The plants were chosen on the basis of their traditional medicinal use and the amount and type of active principles present in them. The first plants selected were those used to treat respiratory infections and illnesses.
Software programmes
In this study, ChemSpider and Drugbank were used as the databases to find all molecular structures [28-29]. The RCSB Protein Data Bank's crystal structure for the receptor was used (PDB code 6LU7) [30]. All the preparation was done using Autodock tools 1.5.6 [31]. Autodock vina was used to carry out the docking study [32,33]. The pkCSM [34] and Swissadmet [35] online servers were carried out to calculate the Lipinski's rule of five and ADMET properties [36]. Simulation of molecular dynamics MD was carried out using Gromacs 2019 package [37] and CHARMM 2017 force field. The results were analysed applying the Discovery studio 2016 package [38].
Molecular docking
Molecular docking, which is performed prior to conducting any experiments, can show that any biochemical process is feasible [39]. Generally, there is four-step procedure to perform the molecular docking, namely, target selection, Ligand selection, preparations, and docking. In this study, molecular docking was performed to predict poses in which the different phytocompounds may be in the Mpro active site. This allows analysing possible intermolecular interactions between the target and the ligand, where various orientations and conformations of the protein interacting with its ligand are generated [20].
The extended PDB format (PDBQT) was employed for ligand and enzyme preparations. Further receptor preparation includes the elimination of cofactors, water molecules, and other ligands; and the addition of polar hydrogens. To obtain the binding site, the crystallographic structure of SARS-CoV-2 Mpro (PDB code 6LU7) was imported into the programme's "work space" in Discovery Studio 2016 [40]. Based on the co-crystallised inhibitor, the active site's centre was then identified and its coordinated extracted (x= -10.782, y= 15.787, and z= 71.277) [22]. For the x, y, and z points, the grid size was set to 20x20x20, and a grid spacing of 1 Á was established to cover the full binding pocket.
Lipinski's rule of five and ADMET prediction
The rule of five (ROF) is a general guideline for assessing drug similarity or determining whether a chemical compound with a particular pharmacological or biological activity possesses characteristics that would make it likely to be an orally active drug in people [41]. Molecular weight (≤ 500), logP (< 5), the number of rotatable bonds (< 10), the number of hydrogen bonds (HB) acceptor (≤ 10), and the number of HB donors (< 5) are among the parameters computed (values of a given property which will not be exceeded) for the Lipinski's rule of five; so, for more than one violation of the ROF, molecules will be viewed as being unimportant for future development, according the authors themselves.
In the study, ADMET was used to predict the absorption, distribution, metabolism, excretion, and toxicity parameters of the examined compounds.
Molecular dynamic simulations
Macromolecular structure-to-function relationships can now be effectively understood using a mature method called molecular dynamics simulations. Current simulation times are close to biological times and the wealth of data collected on the dynamic properties of macromolecules allows structural bioinformatics to change its standard paradigm from studying single structures to analysing conformational ensembles [42].
For this study, simulations of molecular dynamics (MD) were carried out only on the two best molecules derived from the docking studies. The main objective of MD simulations is to evaluate the stability of the ligand-enzyme complex found in the docking study. The results obtained from the docking calculation were used as input for the MD simulation. The ligand-receptor complex was prepared by adding water molecules to solvate the system and sodium and chlorine atoms to neutralise it. Prior to the simulation, the system was relaxed for 100 ps at 300 K and 1 bar. Then, simulation was run for 20 ns.
Results and discussion
Since the emergence of the Covid -19 pandemic, many phytochemicals were evaluated to find candidates which might be able to combat the primary proteins of SARS-CoV-2 [43-52]. Several of them have already been shown to display effective activity against SARS-CoV-1 Mpro [44]; others have been evaluated from Algerian herbal medicines [43], from Indian traditional medicine [45,48-51], from traditional Vietnamese therapy [52], or from Thailand's National List of Essential Medicines [46] as well as compounds selected from the literature with a wide range of biological activities and which were chosen based on their highest activity according to Dr. Duke's phytochemical and ethnobotanical databases [47].
Molecular docking
Among the five therapeutic protein targets of SARS-CoV-2: 3CLpro (main protease), PLpro (papain-like protease), SGp-RBD (spike glycoprotein-receptor binding domain), RdRp (RNA dependent RNA polymerase), and ACE2 (angiotensin-converting enzyme 2) the poses and potential types of interactions between the 129 compounds and SARS-CoV-2 Mpro were carried out using molecular docking. The results are reported on Table 1. Among the 129 molecules docked, warfarin (compound No. 107) was previously studied by us [43].
Table 1 Best conformation affinity in the SARS-CoV-2 Mpro binding pocket.
N° | Name of molecule | Score (kcal/mol) | N° | Name of Molecule | Score (kcal/mol) | N° | Name of molecule | Score (kcal/ mol) |
---|---|---|---|---|---|---|---|---|
1 | p-pinene | -4.6 | 44 | Epi-cadinol | -5.9 | 87 | 7-epi-a-Eudesmol | -5.6 |
2 | Sabinene | -4.4 | 45 | n-Tetradecanol | -4.3 | 88 | Spathulenol | -5.8 |
3 | Limonene | -4.5 | 46 | Hexadecanoic acid | -4.5 | 89 | Rosifoliol | -6.0 |
4 | p-Cymene | -4.6 | 47 | Ethyl linoleate | -4.6 | 90 | Ledene | -5.8 |
5 | p-Myrcene | -4.8 | 48 | Phytol | -4.8 | 91 | n-Hexadecanoic acid | -4.4 |
6 | (Z,E)-farnesol | -5.3 | 49 | Neophytadiene | -4.7 | 92 | Geranyl isovalerate | -5.3 |
7 | Cedrol | -5.0 | 50 | Terpenyl acetate | -5.2 | 93 | Ledol | -5.3 |
8 | Verbenone | -5.1 | 51 | Tetradecanoic acid | -4.4 | 94 | Perillaldehyde | -4.7 |
9 | Trans-Calamenene | -5.9 | 52 | 6,10,14-trimethyl-2-pentadecanone | -4.8 | 95 | a-Terpinyl acetate | -5.2 |
10 | Trans, dihydrocarvone | -4.7 | 53 | p-caryophyllene | -5.8 | 96 | p-Selinene | -5.7 |
11 | Geranial | -4.5 | 54 | a-humulene | -5.7 | 97 | Germacrene | -5.6 |
12 | 1,8-Cineol | -4.3 | 55 | Aromadendrene | -5.3 | 98 | p-Cadinene | -5.8 |
13 | Acetophenone | -4.5 | 56 | y-Eudesmol | -5.7 | 99 | Isoniazid | -4.8 |
14 | a-Muurolene | -5.4 | 57 | Myristicin | -5.3 | 100 | Methacrylonitrile | -3.2 |
15 | p-Eudesmol | -5.7 | 58 | Elemicin | -4.9 | 101 | 2-Propenenitrile-2-methyl | -3.2 |
16 | Cis-peperitol acetate | -5.0 | 59 | Linalool | -4.8 | 102 | Diethyltoluamide | -5.0 |
17 | y-terpinene | -4.6 | 60 | Benzyl salicylate | -6.6 | 103 | Benzoic acid | -4.4 |
18 | Germacrene D | -5.5 | 61 | Ethyl hexadecanoate | -4.4 | 104 | Benzene | -3.4 |
19 | (E)-p-ocimene | -4.5 | 62 | Decanoic acid | -4.3 | 105 | Prasterone | -6.6 |
20 | (Z)-p-ocimene | -4.1 | 63 | Ethyl dodecanoate | -4.6 | 106 | Bicyclo[4.1.0]hept-2-ene, 3,7,7-trimethyl | -4.1 |
21 | Carvacrol | -4.9 | 64 | Ethyl hexadec-9-enoate | -4.9 | 107 | Warfarin | -7.1 |
22 | Davanone | -5.8 | 65 | Dodecanoic acid | -4.6 | 108 | Iodoquinol | -5.5 |
23 | Davana ether | -6.1 | 66 | Ethyl tetradecanoate | -4.3 | 109 | Phenol,4-(2-aminopropyl)- | -4.6 |
24 | Camphore | -4.5 | 67 | a-Eudesmol | -5.6 | 110 | Phenol,2-methoxy-4-(1-propenyl)-, (E)- | -5.1 |
25 | Eucalyptol | -4.2 | 68 | p-bourbonene | -5.3 | 111 | Geranyl acetate | -5.2 |
26 | Chamazulene | -6.0 | 69 | a- terpinolene | -4.6 | 112 | Methyl eugenol | -4.8 |
27 | p-thujone | -4.5 | 70 | 5-cadinene | -5.8 | 113 | p-Linalool | -4.9 |
28 | Catalponol | -6.6 | 71 | 1-Octen-3-ol | -3.8 | 114 | 4-Terpineol | -4.3 |
29 | Piperitone | -4.7 | 72 | a-copaene | -5.1 | 115 | Elemol | -5.1 |
30 | Borneol | -4.3 | 73 | p-elemene | -4.9 | 116 | p-sesquiphellandrene | -5.9 |
31 | 1-epi-cubenol | -5.8 | 74 | 1,8-cineole | -4.3 | 117 | Bicyclogermacrene | -5.0 |
32 | Valencene | -5.3 | 75 | Globulol | -5.6 | 118 | Curcumene | -5.6 |
33 | Humulene oxide II | -5.3 | 76 | a-phellandrene | -4.7 | 119 | Pulegone | -5.2 |
34 | Caryophyllene oxide | -5.5 | 77 | p-Bisabolene | -5.6 | 120 | Iso-pulegyl acetate | -4.9 |
35 | Terpinen-4-ol | -4.3 | 78 | 4,8,12,16-tetramethylheptadecan-4-olid | -5.5 | 121 | Carvone | -4.8 |
36 | Bornyl acetate | -5.2 | 79 | y-Cadinene | -5.7 | 122 | Thymol methyl ether | -4.8 |
37 | a-Terpineol | -4.8 | 80 | Germacren D-4-ol | -5.7 | 123 | Linalyl acetate | -4.8 |
38 | Thymol | -4.7 | 81 | p-Citronellol | -4.4 | 124 | Allo-ocimene | -4.4 |
39 | Cymene | -4.6 | 82 | Trans-pinocarveol | -4.5 | 125 | Neryl acetate | -5.1 |
40 | Camphene | -4.0 | 83 | Cis-verbenol | -4.4 | 126 | a-amorphene | -5.3 |
41 | Eugenol | -4.9 | 84 | Manoyl oxide | -5.9 | 127 | E-caryophyllene | -5.2 |
42 | Isomethyl-a-ionol | -5.2 | 85 | a-Cadinol | -5.9 | 128 | Carvotanacetone | -4.7 |
43 | Dihydrocarveol acetate | -5.2 | 86 | Pinocarvone | -4.9 | 129 | a-Pinene | -4.8 |
Warfarin has the highest docking score out of all the compounds (-7.1 kcal/mol). Here it is interesting to mention that warfarin is an anticoagulant and is used to prevent thrombosis and embolism. Its anticoagulant effects are based on blocking the vitamin K-dependent (VKD) stage in the full synthesis of several blood clotting components necessary for healthy blood coagulation [53]. In consequence, interactions between warfarin and Chinese herbal medicine were reviewed and described [54]. Additionally, some features of warfarin's anti-tumor effect are thought to be a result of its antithrombotic activity. It's also crucial to note that warfarin's inflammatory and immunomodulatory actions point to a far wider range of possible consequences [55]. Additionally, warfarin toxicity and metabolism are highlighted [56].
Other than warfarin, six additional molecules were found to have interesting docking scores which include catalponol, benzyl salicylate, Prasterone, davana ether, chamazulene, and rosifoliol (Table 2). Therefore, these seven molecules underwent further study for being the best possible candidates to inhibit SARS-CoV-2 Mpro.
Some of these compounds were found to possess biological activities, such as chamazulene, which is considered a natural anti-inflammatory [57] associated to its antioxidant properties, and contributes to the in vivo antiphlogistic activity by inhibiting the leukotriene synthesis, and it has antitumor activity as well [58].
According to Lobo [59], Prasterone has been approved by the US Food and Drug Administration (FDA) for symptoms of dyspareunia in postmenopausal women; and Heo [60] proposes intravaginal Prasterone as a treatment for vulvovaginal atrophy in postmenopausal women. Huang et al. [61] confirmed the pharmacological functions of catalponol which improved the biosynthesis of dopamine through the activation of tyrosine hydroxylase and the protection of PC12 cells cytotoxicity induced by L-DOPA.
To verify the reliability of the procedure and the software used in this work to produce reliable results, the co-crystallised ligand (N3) was re-docked into the active site and a docking score of -6.9 kcal/mol was found. Successively, the N3 structure was compared to the docked one (Figure 1a) and 16 interactions were found (Figure 1b [62]). The interactions between warfarin and the Mpro were established previously [43]. For the other interactions between compounds and SARS-CoV-2 main protease, they are shown in Figures 2 (a-f).
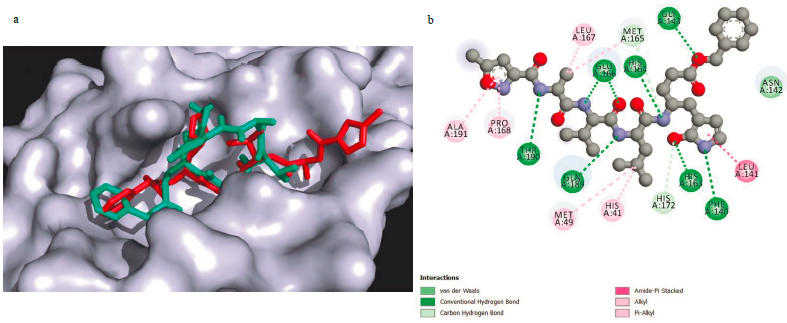
Figure 1 (a) N3 co-crystallised ligand (red) and N3 docked ligand (cyan); (b) Interactions between inhibitor N3 and SARS-CoV-2 Mpro[53].
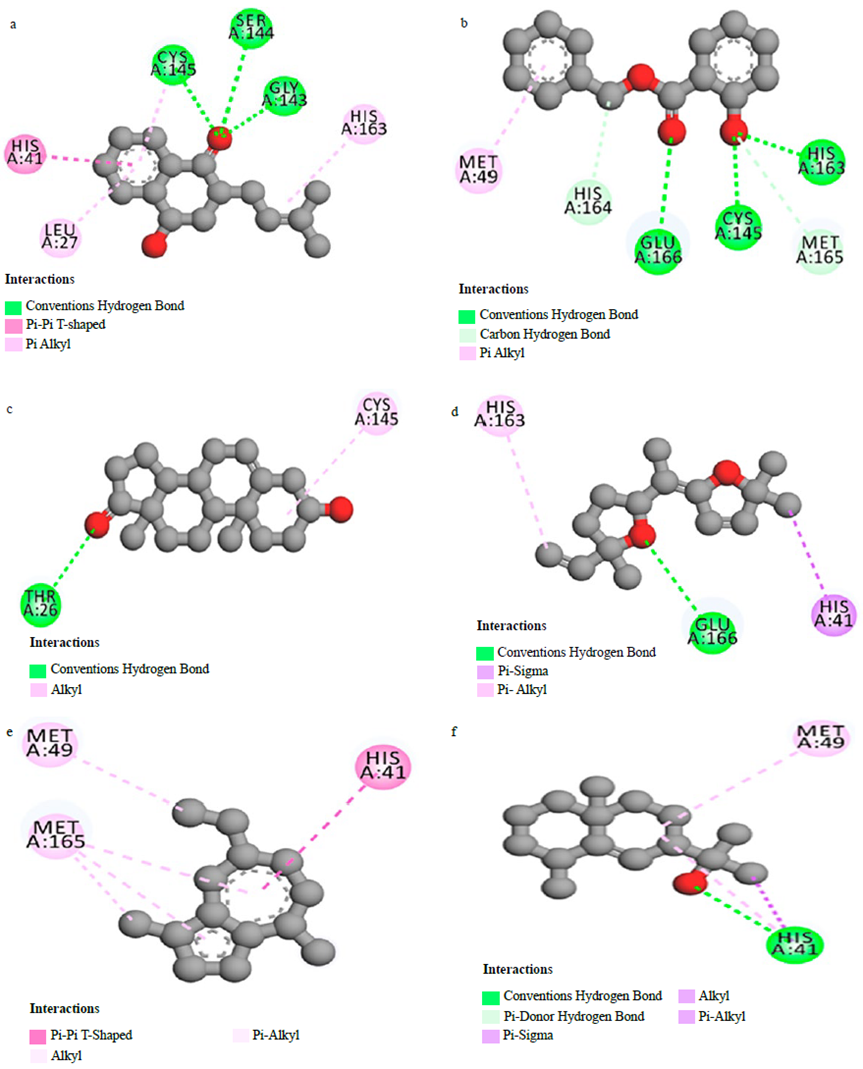
Figure 2 Interactions between Catalponol (a), Benzyl salicylate (b), Prasterone (c), Davana (d) Chamazulene (e), Rosifoliol (f) and SARS-CoV-2 main protease
The results show that both structures overlap in most of the molecule, demonstrating that the procedure employed is able to reproduce the experimental results. The difference between the two structures is noted at one end of the molecule, where the docking result shows that the chain is oriented upward due to the flexibility of the peptide losing the interaction with the receptor, while the co-crystallised structure is interacting with the whole pocket. Analysing the N3-Mpro complex, 16 interactions were found, which include a Van der Waals interaction with Asn142, HB interactions with Glu 166, His 164, Gly 143, Thr 190, Gln 189, His 163, and Phe 140 residues, amide-n Stacked interactions with Leu 141 residue, Carbon HB with Met 165 and His 172 residues, and Alkyl and/or n-Alkyl interactions with Leu 167, Ala 191, Met 167, Pro 168, Met 49 and His 41 residues [62].
This result is relevant to analyse whether the studied molecules may interact with the active site and whether those interactions may be strong enough to compete with the natural substrate inhibiting the enzyme. Based on the docking score, only warfarin shows a higher value compared to the N3 inhibitor. The docking results of the best seven molecules based on the docking score show that Warfarin interacts with five residues [43], catalponol with five residues, benzyl salicylate with six, prasterone with two, davana ether with three, chamazulene with three, and rosifoliol with two residues. It is important to notice that the N3 inhibitor forms more than twice the interaction of any of these drug candidates which may infer a weak interaction in case any interaction at all is produced. Delving deeper into the analysis of the interactions between warfarin and the Mpro, the interactions include HB with Glu 166, Ser 144 and Cys 145 residues, n-n T-shaped with His 41 residue and n-alkyl with Met 164 residue [43]. Also, the presence of HB and n-n T-shaped interactions in the complex formed by warfarin and Mpro increase the affinity of the complex and may be related with its pharmacological activity. This is in accord with the reports in the literature where the HB role is identified with the pharmacological effect of a ligand [21,62].
The values obtained for Mpro docking with warfarin, catalponol, benzyl salicylate, prasterone, davana ether, chamazulene and rosifoliol may be related to the number and type of bonds observed in the ligand-target formed, in fact the presence of the HB suggests an important potential pharmacological effect for the Mpro inhibition of SARS-CoV-2. Thus, the inhibition of this protein will stop viral replication and may become useful in the clinical management of COVID-19. These results are supported by many other studies where Glycyrrhiza glabra is used in traditional medicine to treat cough and as a laxative as mentioned by Chouitah [63]. Warfarin and catalponol, found in this study to be two of the most interesting molecules, are part of the active ingredients present in Glycyrrhiza glabra. Therefore, the extract of this plant could have very interesting effects on the inhibition of SARS-CoV-2 Mpro. According to Soleiman-Meigooni, liquorice (Glycyrrhiza glabra L.) syrup can be used as an adjuvant treatment for mild to moderate Covid-19 in addition to the recommended course of care [64]. It was found that, glyasperin A, glycyrrhizic acid, and compounds having oxane ring and chromenone ring substituted with hydroxyl 3-methylbut-2-enyl group could be considered as the best molecules and leads for the development of a new drug based on liquorice against COVID-19 [65,66].
Lipinski's Rule of five and ADMET Prediction
Using the web servers pkCSM and Swissadmet, the Lipinski's rule of five, and the ADMET parameters of the chemicals examined in this paper were computed. According to "the rule of 5", an orally active drug may have no more than one violation of the following criteria (Table 3): molecular weight less than 500; not more than 5 H-bond donors, 10 H-bond acceptors and 10 rotatable bonds; an octanol-water partition coefficient log P not greater than 5.
The results obtained for all the studied compounds in this work respect Lipinski's rule of five, including warfarin [43]. The N3 inhibitor has three Lipinski violations [30], which is to be expected, since it is a peptide-type inhibitor, characterised by a high MW, and many more than 10 rotational bonds. The parameters predicted (Table 4) were water solubility (WS) and human intestinal absorption (IA), volume of distribution (Vd), blood-brain barrier permeability (Bbp), cytochrome P450 (CYP450) metabolism, total clearance (TCl), hERG I and II inhibition (hERGi) and syntetic accessibility (SA).
All the molecules exhibit a low value for the blood-brain partition coefficient. This may indicate that, there will not be any risk of central nervous system (CNS)-related toxicity because these molecules have poor potential to pass the blood-brain barrier. The WS, IA, and metabolism pharmacokinetic parameters present drug-like values with more than 92% of intestinal absorption, WS between 6.3x10-4 and 4.0x10-6 mol/l, and a hepatic metabolism for catalponol, benzyl salicylate, and Prasterone. Also, the toxicity predicted for hERG I and hERG II inhibitors indicates that any compound exhibits hERG I, while only prasterone has hERG II inhibition. SA values show the feasibility of experimentally synthesising all the studied compounds.
Molecular dynamics simulation
Although the seven studied compounds were found to have good docking scores against Mpro, following Lipinski's rule of five and exhibiting appropriate ADMET properties; only the two best compounds (warfarin and catalponol) were taken to further MD simulations to determine the possibility of keeping the ligand-receptor complex in a solvated system for a period of time. Results will increase the feasibility of these compounds for being possible COVID-19 treatments through Mpro inhibition. In Figure 3, the results for the simulation of Warfarin against Mpro is shown.
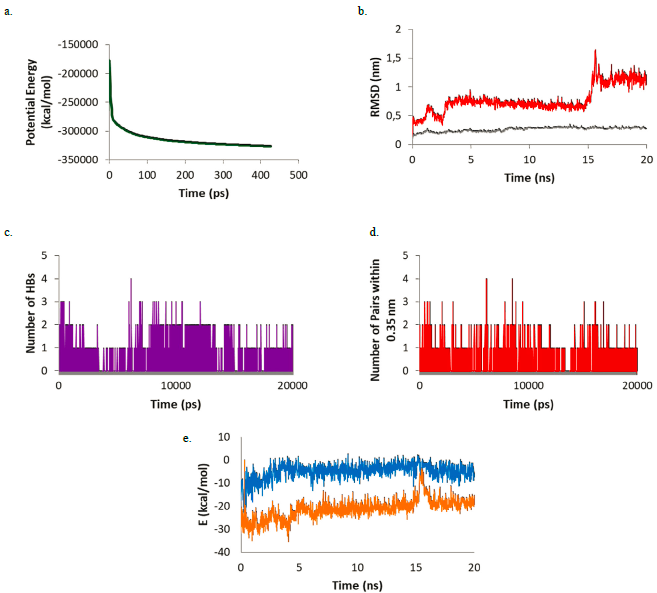
Figure 3 (a) Potential energy; (b) RMSD of Warfarin (red) and the Warfarin-Mpro complex (gray); (c) number of HBs; (d) number of pairs within 0.35 nm; (e) Coulomb (blue) and Lennard-Jones (orange) interaction energy during the simulation.
For this complex, the potential energy (Figure 3a) reaches a plateau before 500 ps showing that after this time, the system is at its lowest potential energy. The RMSD plot (Figure 3b) shows the ligand-enzyme complex maintains values lower than 0.5 nm while the ligand presents values lower than 1 nm during most part of the simulation (first 15 ns). During the last 5 ns the RMSD values in the ligands increase to around 1.1 nm. Looking at the HB formation and the number of pairs within 0.35 nm (Figures 3c and 3d) it may be observed that the maximum number of HBs and pairs is four and the minimum number is zero. In most parts of the simulation, at least one HB and one pair are formed. Finally, the Coulomb and Lennard-Jones interaction energies were evaluated (Figure 3e). While the Coulomb energies stayed constant throughout the simulation with values around -5 kcal/mol, the Lennard-Jones interaction energies increased as the simulation proceeded starting at -25 kcal/mol and ending at around -17 kcal/mol. Comparing the structure at the beginning of the simulation and at the end, it was observed that warfarin moves out of the active site cavity and stayed in the most superficial part of the pocket (Figure 4). This movement will make warfarin lose most of its potency although there is still some chance based on the interaction formed to be strong enough to compete with natural substrates.
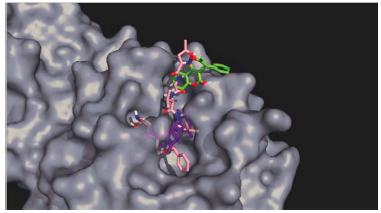
Figure 4 Docking (Purple) and MD (Green) results of the interaction between warfarin and Mpro. N3 inhibitor is shown in pink.
Analysing the simulation between catalponol and Mpro, the potential energy plateau is reached at 1181 ps. The three-dimensional structure of the complex appeared after 20 ns, the ligand could not manage to stay in the active site and leaves the system. This can be reflected in the plots found in Figure 5. The RMSD plot shows catalponol RMSD values are less than 0.5 nm in the first 5 ns of simulation. In the next 5 ns, values rise up to 2 nm. After that, in the final 10 ns, values experience a great increase reaching values of 8 nm. Looking at the HB and the number of pairs within 0.35 nm, catalponol makes interactions only during the first 10 ns of simulation. After that, all interactions are lost. In the first 10 ns, catalponol makes between 1 and 4 HBs and between 2 and 7 pairs within 0.35 nm. The same can be observed in the Coulomb and Lennard-Jones interaction energy where negative values are found up to 12 ns. After that all interaction is lost and both energies are zero.
Conclusions
Herbal medicine, natural and safe, requires consideration by scientists. Essential oils from aromatic and medicinal plants are used by people for the treatment of infectious diseases throughout the world. Bioactive compounds contained in essential oils may be studied for drug development. Because traditional drug research and development takes a lot of time and money, computational study at multiple scales becomes a crucial instrument in drug discovery. In this sense, by using in silico methods, from the 129 natural compounds of Algerian essential oils, molecular docking indicated that warfarin (in this study and according our previous finding [43]), catalponol, benzyl salicylate, prasterone, davana ether, chamazulene and rosifoliol are the molecules that showed the best docking score against Mpro. Furthermore, each of them adheres to Lipinski's five requirements and provides suitable premeditated ADMET characteristics. Among these compounds, warfarin displayed the best results and presented a better docking score than Mpro inhibitor N3. A simulation time of 20 ns shows that warfarin manages to stay in the active site although it moves from the active site's cavity to a more superficial section of the active site. Furthermore, catalponol did not stay in the active site after the simulation. Results suggest that warfarin may be an interesting candidate for performing further studies to try to find a new and effective treatment against COVID-19. However, before reaching a firm conclusion, there are still a number of measures to be taken in order to experimentally verify and validate the current result.