Introduction
The effects of air quality on human health have been studied extensively by the medical community. There are epidemiological studies that determine the health effects in the short-, mediumand long term for different pollutants and population groups (Toro et al. 2015; Kampa and Castanas 2008). Some efforts have been made to estimate the effect of pollution on social variables such as subjective utility (Levinson, 2012), school attendance (Currie et al. 2009; Ramson and Pope 1992), student performance (Mohai et al. 2011; Marcotte 2015) and overall well-being (Román-Collado and Jiménez de Reyna, 2019).
In this paper, we estimate the effects of air quality on student performance using a repeated pooled cross-section data structure rather than the typical cross-sectional data used in previous studies. We focus on fourth graders, because they tend to experience physical symptoms much more strongly than adults (Schwartz, 2004). Studies on attendance and air quality find that, even when they are below government standards, high levels of carbon monoxide (CO) have a significant negative impact on school attendance. However, there is limited information about the impact of air pollutants on student academic achievement, and this is subject to the usual external validity concerns (Lavy, Ebenstein and Roth, 2014).
Pollution is a serious environmental problem in Santiago, Chile, because the city is located in an enclosed valley with limited wind and rain. In addition, these types of valleys are subject to the effects of a thermal inversion layer, a well-known phenomenon that causes an ‘atmospheric lid’ to form above the valley floor, preventing the escape of pollutants. Coupled with high levels of industrial development and vehicle emissions, the inversion layer contributes to levels of pollution that often exceed the values suggested by the World Health Organization (WHO, 2016), the standards set by the US Environmental Protection Agency (EPA) (EPA, 2019), and the Chilean guidelines.
The medical literature emphasizes that pollutants may have negative health effects above a certain threshold (Bernstein et al. 2004). These thresholds depend upon many factors including age, gender, and nutrition. However, based on aggregate information, regulatory agencies have established some legal maximum thresholds for different periods of time. These regulations vary by country and over time.
Particles less than 10 micrometers in diameter (PM10) can potentially increase respiratory health risks and cardiovascular problems in the long run, including chronic diseases such as asthma or lung cancer. Fine particles (PM2.5) are so small that they can get into the lungs, causing serious health problems in the long run and temporary symptoms such as irritation of the eyes, nose, and throat, coughing, chest tightness, shortness of breath, heart palpitations and fatigue. Nitrogen dioxide (NO2) can irritate the lungs and lower resistance to respiratory infections. Carbon monoxide (CO) is known to reduce oxygen in the bloodstream, while exposure to high levels of CO is associated with visual impairment, reduced work capacity, lowered cognitive abilities, and difficulty in performing complex tasks. CO in extremely high concentrations can cause death by asphyxiation. Exposure to ozone (O3) for 6 to 7 hours, even at relatively low concentrations, may induce symptoms such as chest pain, coughing, nausea, and pulmonary congestion. In high concentrations methane (CH4) weakens the central nervous system. Finally, there is sufficient evidence that short-term exposure to sulfur dioxide (SO2) for periods ranging between 5 minutes and 24 hours may induce bronchoconstriction and increased asthma symptoms, particularly among children and the elderly.
We analyze the short-term impacts of air pollution on student academic achievement using observational data. As discussed above, pollutants produce shortand long-term negative effects on human health. Children tend to be particularly susceptible, and their experiences of temporary symptoms may disrupt usual activities (Schwartz, 2004). In this sense, pollution might impact the concentration of students while they are taking the test, significantly diminishing their ability to understand questions and search for solutions. Thus, this channel should be reflected in the coefficient estimates of pollution. Notice that we use observational data. This implies that the data is not obtained from lab conditions, so we cannot control any variable at our will. Hence, in this type of analysis there are several endogeneity concerns that should be taken into consideration. Simple cross-sectional comparisons of pollution and student achievement suffer from omitted variable bias (OVB). Air pollution is not randomly assigned, and several confounding factors (eg, urbanization, crime levels, school quality) that are correlated with air pollution and student achievement could bias the results. Furthermore, parents can choose where to live based on their preferences for clean air and school quality (Chay and Greenstone, 2005). We use two identification strategies to address these issues. Our first model uses school and year fixed effects. That is, we compare students within the same school over time, while controlling for constant unobservable school and year factors, as well as student, family, and school-observable characteristics. In our second strategy, we use this same model, but instrument the level of pollution by relative humidity levels. Relative humidity is an instrumental variable with some disadvantages because it is weakly correlated with some pollutants. Rain would have been a much stronger instrument because it strongly changes the pollution levels in an exogenous way. However, there was no rainfall during the spring period analyzed, requiring relative humidity to be used instead. In general, we find that a one standard deviation increase in pollution levels decreases student performance in math and science. Finally, the effect on language exam scores is not statistically significant, despite having the expected negative sign.
The paper is organized as follows. The next section describes the relevant background literature and some specific characteristics of the city of Santiago. Section 3 presents the data, while Section 4 explains the econometric methodology. Section 5 describes and discusses the principal results of the paper. Section 6 concludes.
2. Background
Three different literature strands are relevant to this study. The first consists of literature describing the negative effects of different air pollutants on human health, as well as their transmission mechanisms. There is sufficient evidence of the association between SO2, NO2 and CO exposures and respiratory and cardiovascular health problems. Similarly, short-term and long-term effects of particulate matter and ozone levels on human health have been found in different studies (Kampa and Castanas 2008; Bernstein et al. 2004; Brunekreef and Holgate 2002).
The second relevant line of research consists of studies of the relationship between pollution and school attendance. There is evidence of a monotonically decreasing pattern between CO levels and school attendance (Currie et al. 2009). Furthermore, an increase of 100μg/m3 in the 28-day moving average of PM10 translates to an increase in the school absence rate equal to approximately two percentage points (Ramson and Pope, 1992). These models control for climate variables such as temperature, snowfall, and variables indicating day of week, month of school year, and days preceding and following holidays and extended weekends.
The third related strand analyzes the relationship between differences in pollution across geographic areas and differences in average family income across these differences. Poorer students are exposed to higher levels of pollution than their higher-income counterparts (Levinson 2012; Chay and Greenstone 2005; Pastor, Morello-Frosch and Sadd 2006). These findings raise an important endogeneity concern for our work, namely that differences in pollution levels across geographic areas might actually pick up differences in socioeconomic status in different student populations.
All these studies emphasize that pollution may have thresholds that mean that only once they are exceeded are its effects noticed. In our case, we are studying the effects of pollution on the score achieved by a given student on the day the test was taken. These standardized tests are taken by students corresponds in the Spring. This time of the year is one of low air pollution in Santiago and air quality might be considered “good” according to Chilean regulations. This might make it harder to detect statistically significant effects. Indeed, we fail to obtain statistically significant results for some pollutants. However, in the case of CO and NOX, we do find a negative statistically significant coefficient estimate of the impact on achievement. This suggests that the threshold for both pollutants, which may harm student achievement and probably also their health, could be lower than the one imposed by the environmental agency. This result is in line with those reported in other articles (Currie et al. 2009; Levinson, 2012).
Table (1) below presents the pollution standards for the United States and Chile. We see that although some standards are the same, this is not true for all pollutants. Furthermore, these regulations are revised constantly, and modified whenever new evidence suggests the need.
In the city of Santiago, the concentration of pollutants is determined by topographical and meteorological characteristics that produce a well-established pattern of air pollution. These geophysical conditions are more relevant than the location of pollution sources when it comes to defining the areas where the pollution will be concentrated. This means that air pollutants are redistributed by wind currents, and concentrated in specific geographical areas of the city (Schmitz 2005; Gramsch et al. 2006). Higher pollution levels coincide with poorer neighborhoods because of the patterns of the airstreams and the consistent social segregation that characterizes the city (Fernández and Wu 2016; Sabatini et al. 2009). Hence, they suffer the negative impacts of the pollution more than richer towns. This could imply some endogeneity issues because of sorting. In the methods, we discuss ways to address this question.
Fig. (1) illustrates that different pollutants do indeed tend to exhibit higher concentration levels in poorer towns than richer ones. The atmospheric science literature for the city suggests that this holds true for most areas.
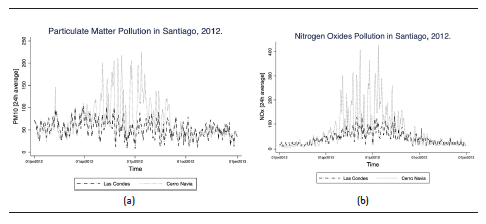
Fig. 1. Different pollutant levels for two counties: Las Condes (high income) and Cerro Navia (low income).
Finally, the National Bureau of Economic Research published a paper whose conclusions are similar to ours concerning the causal relationship between air pollution and student academic performance (Lavy, Ebenstein and Roth 2014). The general methodology of this study is similar to ours, although the structure of their data is different. The authors find that air pollution has a negative effect on standardized test scores. We contribute to this literature by disaggregating the effects by subject, namely, math, science and language. In addition, unlike the majority of studies, we provide a new case study for a city in a developing country.
3. Data
To analyze the relationship between student academic performance and air pollution, we collect data on standardized test scores regularly taken by fourth graders in the Chilean Educational System. These students are the youngest cohort to take standardized tests and are hence expected to be the most affected by air pollution. We also collect data on air quality and weather from all available monitoring stations in the city of Santiago. In addition to academic performance data, the dataset contains demographic information on every student. Using this information, we build a socioeconomic status (SES) index by means of factor analysis.
3.1 Student Achievement and Attendance Data
According to the Department of Education, in 2009 the Chilean educational system served approximately 3.5 million students in more than 11,000 schools. Ninety five percent of children aged 5 to 14 attend school, as did seventy five percent of 15-19 year-olds (OECD, 2012). One important characteristic of the Chilean educational system is that since the early 1980s, the administration of public schools has been shared between the public and private sectors. Local governments or municipalities administer public schools, which today represent forty-six percent of the schools in the country. Municipal schools are financed by the central student-based subsidy and cannot charge tuition. The private sector administers both publicly subsidized private schools (fortynine percent of schools) and non-subsidized private schools (five percent). Publicly subsidized private schools receive the same student-based subsidy, but are allowed to charge tuition up to a certain amount. When subsidized schools charge tuition, their public subsidy is reduced but not by a commensurate amount. In this study, we include both municipal and publicly subsidized schools.
We use standardized student-level achievement scores from the National Learning Outcome Assessment (SIMCE in Spanish) for all fourth graders during the years 2002, and 2005 through 2012. This national assessment is equivalent to the National Assessment of Educational Progress (NAEP) in the United States. We focus our analysis on all fourth graders enrolled in schools in Santiago. The SIMCE database also includes student demographic information, as well as family background characteristics. Given that the variables used to measure family characteristics are not always comparable across years, we estimated a composite score that measures the socioeconomic level of every student’s family. We estimated this composite score by conducting a factor analysis using the father’s and mother’s educational level, household income, and the number of books at home. Student participation is mandatory in all subjects tested, which include Mathematics and Language, as well as a test that combines natural and social sciences that we call Sciences. Student achievement results are comparable across time and are reported on a scale of mean of approximately 250 points and a standard deviation of approximately 50 test points. For this study, we standardize test scores every year to a mean zero and a standard deviation of one, so that the interpretation of the estimates is that commonly used in the literature.
3.2 Pollution and Weather Data
We scrape the web for unique information on air quality from all eleven pollution-metering stations in Santiago, which have been monitoring air conditions for the past decades. These data are obtained from the National Information System on Air Quality (SINCA in Spanish). We focus our analysis on different pollutants that are measured by these stations, namely, PM10, PM2.5, CO, O3, CH4, (NOX), and SO2. Notice that NOX is the sum of nitric oxide (NO) and nitrogen dioxide (NO2).
These eleven stations are well-spaced across different counties of the city, namely Independencia, La Florida, Las Condes, Parque O’Higgins, Pudahuel, Cerrillos, El Bosque, Cerro Navia, Puente Alto, Talagante, and Quilicura. All the stations measure daily averages of the pollutants and start and stop collecting data on different dates. The only exceptions are Independencia and La Florida which do not measure CH4 at all.
To estimate the average pollution levels for each school in Santiago, we gathered their geographical coordinates alongside those of all the monitoring stations located in the city. Geographical information for schools is provided by the Department of Education, while for the monitoring stations the data is taken from the SINCA website. We then estimate the distance between each school and each monitoring station. This figure is computed using the standard formula to measure Earth distances.
where Φ is latitude, λ is longitude, 1 and 2 represent locations, Δ is the difference operator and R is Earth’s radius (mean radius = 6,371 km).
The next step is to compute the relevant pollution level for each school using two different methodologies: 1) a weighted average using the inverse of the distance between the school and each station with non-missing information; and 2) the average pollution level from the nearest station with non-missing information. We estimate the average pollution levels using the pollution level during the day of the test, as well as 2-day, 3-day, 7-day, 14-day, and 28-day averages previous to the day of the test. From 2002 to 2009, tests were conducted at the end of the first week of November, or the beginning of the second week. From 2010 onwards, tests took place during the third week of October. We use the exact dates for the estimation of the pollution averages in this study. We also collect daily information on temperature, relative humidity, and precipitation.
Table (2) presents descriptive statistics for pollutants across all years using the daily average metric.
Table 2. Descriptive Statistics for Daily Average Pollution
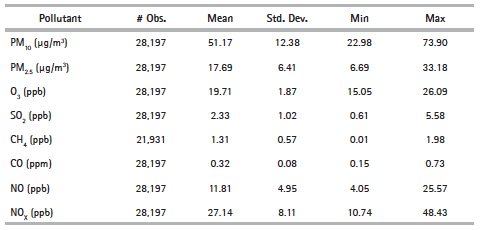
Source: Authors’ computation based on Data from the National Air Quality Agency of Chile for the years of analysis.
The data show that pollutant levels for lowand high-income counties differ significantly. For instance, in Cerro Navia, a low-income county, NOX levels exceeded the standard 22 times in 2012, while for Las Condes, a high-income county this did not happen once. Similar patterns are observed for other pollutants with the sole exception of O3, which is more concentrated in the higher parts of the city where Las Condes and most of the affluent towns are situated. This is explained by the fact that more sunlight is required to produce O3 and these conditions are met in these more elevated localities.
Given the physical characteristics of the city, most of the towns in Santiago experience pollution levels that fall between these two extremes. In addition, the regional government has implemented policies that have noticeably reduced pollution levels over time. This provides sufficient variation in the data, a permitting us to expect some statistically significant results. Had these conditions not been present, the analysis would not have been possible.
4. Empirical Methods
Given that air pollution is not randomly assigned, and that parents may choose where to live based on air quality and other factors correlated with this and with student achievement (e.g., high urbanization, school quality, density, crime), simple cross-sectional comparisons of air pollution and student achievement cannot identify the causal effect that pollution may have on short-term student performance.
Moreover, Santiago is a highly economically segregated city. For historical reasons, lowand moderate-income families live on the west side of the city, while upper-middle income and rich families congregate in the east. The east side has an elevation averaging 900 meters above sea level, compared to around 550 meters on the west side. This is not a trivial observation since most pollutants have a heavier molecular weight than air, and hence tend to follow gravity. In addition, airstreams take pollutants to the lower section of the valley (Gramsch et al. 2006). As a result, lowand moderate-income areas have a higher concentration of air pollutants than upper-middle and rich areas.
To remove the influence of key confounding unobservable factors, we exploit the panel nature of the data by estimating the econometric model given in equation (2). We estimate all models for three different time periods: i) 2002 and 2005 through 2012; ii) 2006 to 2012; and iii) 2008 to 2012. We do this to take into consideration the fact that not all pollutants started being collected at the same time. We present the results using (i), that is, including all years.
where As is the standardized test scores for student i, in school j at time t for subject s (math, language and sciences). The variable Pol captures the pollution level of the different pollutants (i.e., .). . is a vector of student demographic characteristics including family socioeconomic status (SES), and . includes school level characteristics. This model includes school and time fixed effects (hj and lt , respectively). In simple words, it compares the achievement level of fourth graders at a given school across time, while controlling for the observable time-variant socioeconomic characteristics of the students and schools, as well as unobservable fixed characteristics. The results of this estimation are presented as Model B. We also estimate this equation without school fixed effects (Model A). See Rojas-Vallejos and Lastuka (2020) and references therein for a further discussion on the application of fixed effects in a panel context.
We then estimate a Two-Stage-Least-Square (2SLS) model where the instrument for the level of pollution is relative humidity, presenting these results as Model C. The ideal instrument is the level of precipitation, since rain clears the skies and lowers the levels of pollution exogenously. However, at the time of the test (i.e., mid-October and November) the level of precipitation in Santiago is zero for our dataset. These results must be interpreted with caution since the instrument is not very strong by looking the first stage output. Despite this, they do shed some light on the relationship being explored.
Our models provide consistent estimates of the effects of air pollution on student achievement as long as no other factors systematically affect both pollution and student achievement at the school-year level. One example that would violate this assumption would be a fire that affects air quality and student achievement at the same time. During this time period, we are not aware of any fires or large environmental disasters affecting Santiago. Another potential violation would be that certain areas of the city are undergoing high levels of development that have increased pollution levels and changed the demographic characteristics of the school population, factors that could affect final student performance levels. This is a potential threat to our models. We do our best to control for it by including the socioeconomic status of the students attending each school. Results for our estimates, including attendance data, are not included in this study since our focus is on shortrather than longrun effects. Nevertheless, we obtain some insight into the longer-term effects of pollution on student performance by regressing the cumulative averages of pollution of different time periods on test scores.
5. Results and Discussion
In this section, we present our estimates of the short-term and cumulative effects of air pollution. Short-term effects are estimated by considering the pollution levels on the date the test was taken, while cumulative effects are estimated using the average pollution level for a number of days before the test and its date of application.
Panel (1) in Table (3) presents variants of equation (2) for three different pollutants (NOX, NO, and CO) and math test results of fourth graders in Santiago for the years 2002, and 2005-2012. Each row represents a different estimating equation. Model A is constituted by OLS estimates that exclude fixed effects. Model B is the preferred model and shows the estimates for equation (2). Model C estimates a 2SLS equation model of (2). For each model we present the results using the weighted pollution level of all monitoring stations (“All” column), as well as the pollution level of the nearest station (“Nearest” column).
For the case of NOX, our OLS results suggest that pollution levels have no impact on the math achievement levels of fourth graders in Santiago, raising the possibility of unobserved confounding variables. We find a negative and statistically significant association between NOX and math test results in Model B. In terms of magnitude, the estimates imply that with a one standard deviation (sd) increase in pollution levels (i.e., 8.11 units in the case of NOX) math test results decrease by about 0.06 sd and 0.008 sd including all stations and the nearest, respectively. These results are considered medium to low size effects on student performance. The results of Model C also show a negative impact of NOX on math test results but are not statistically significantly different than zero. The results for NO are quite similar to those observed for NOX.
In the case of CO, we find that the OLS results suggest a negative association between this pollutant and student performance. Model B, our preferred model, also suggests that CO has a negative impact on student achievement. Model C shows statistically insignificant results. In terms of the magnitude of the effect in Model B, the results indicate that a one sd increase in CO (0.08 being the sd for this pollutant in the data: see Table (2)) decreases math test results by about 0.02 sd.
Table 3.b The Impact of Air Pollution on Academic Performance
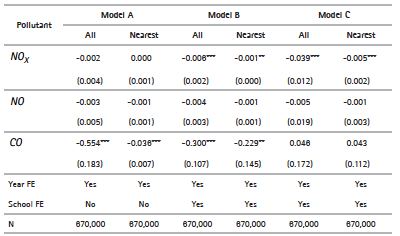
*** p < 0.01, ** p < 0.05, p < 0.10*. Clustered standard errors are in parentheses. All models control for student and school socioeconomic level.
Panel (2) in Table (3) presents the same types of results as Panel (1), but this time for science. The patterns in Models A and B are quite similar to the ones observed for math. The only difference is that the results for the 2SLS (Model C) for the case of NOX are now statistically significant and larger in magnitude. We also observe a perverse sign for CO in the case of Model C. This could be explained by the weakness of the instrument. Let us stress once again that we are using relative humidity as an instrument since there is no rainfall for the application dates in this sample. Relative humidity as an instrument is relatively weak for different pollutants.
Panel (3) in Table (3) presents the language test results. Observe that the patterns are again consistent with the ones observed for math and sciences. However, the magnitudes of the effects are somewhat smaller, with the exception of those associated with CO.
Nevertheless, there is sufficient evidence to argue that, compared with math and sciences, pollution seems to have almost no negative effect on language performance. This result might be expected if we consider that language is a more intuitive skill than math and sciences, and is also more frequently used in daily life. Furthermore, medical research shows that different parts of the brain deal with different cognitive skills. Notice that the impacts of pollution levels seem to be robust to whether we consider the average of all monitoring stations or the value of the nearest. The estimates change in magnitude, but the signs and significance levels remain similar in most cases.
It is worth pointing out that on the dates the tests were administered pollution levels were relatively low. However, some pollutants do still have negative and significant effects on students’ achievements, while it appears that the levels of PM10 are so low that we fail to detect significant effects. For these periods, PM10 is on average around 50μg/m3 per day with a standard deviation of about 10μg/m3, while the regulation states that the maximum cannot exceed 150μg/m3 in 24 hours. Thus, pollution remains considerably below the threshold that is considered to have negative health effects in Chile.
Thus, as the medical research shows, at higher pollution levels, health effects are intensified. Hence, in the months of June and July when pollution reaches its highest levels, exceeding the threshold at least 20 of the time, the impact on learning and performance may be quite significant. June and July are months that form part of the academic calendar in Chile, so students attend classes regularly during these months.
Next, we briefly discuss the impact of other relevant variables on student performance. In Panel (1) in Table (4) we show that socioeconomic status (SES) plays an important role in student performance. A one sd increase in socioeconomic status will increase student achievement by around 0.35 sd. This is quite high considering that policies exclusively targeting student performance achieve improvements of around 0.1 sd. We can also see that whether the school is private (benchmark group), public or subsidized (similar to charter schools in the United States) also affects student academic performance. The data tell us that students from public schools tend to do a lot worse than otherwise similar students from private schools, while in the case of subsidized schools this reduced academic performance is less marked but still present.
We find important negative effects of certain pollutants on students’ academic performance (0.02-0.06 sd), equivalent to around 30% of a successful educational policy in developing countries [18]. Reducing pollution levels has many benefits, and this research finds evidence that it would likely increase student performance, thereby reducing Santiago’s achievement gap. This holds for the city of Santiago given the specific geographical and environmental characteristics discussed above.
Table 4. Pollution, Demographics and Cumulative Effects
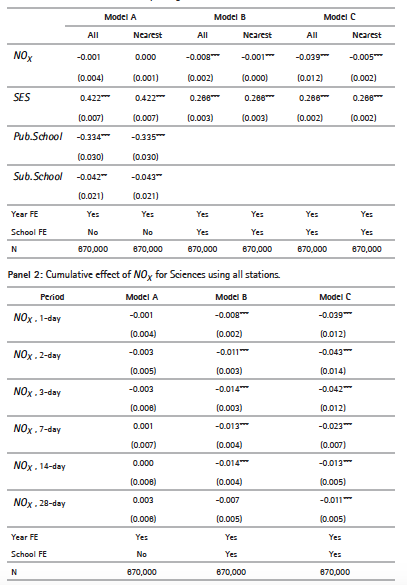
*** p < 0.01, ** p < 0.05, p < 0.10*. Clustered standard errors are in parentheses. All models control for student and school socioeconomic level.
Panel (2) in Table (4) shows a non-monotonic behavior of the impact of pollution (in this case NOX) on student achievement. However, this adverse effect seems to be persistent and does not go away. This could play a significant role in the accumulation of human capital over the long run. Suppose that achievement is negatively affected every year by 0.2 sd, then a student’s years spent at primary and secondary school, the effect could reach a value between 0.24 sd and 0.28 sd. This type of behavior is similar for other pollutants. The results for math are similar, and for language are mostly non-significant.
6. Conclusion and Policy Recommendations
The main results of this study suggest that air pollution has a negative impact on student academic performance. The magnitude of this impact seems small. However, it represents around 30% of a successful intervention targeting educational achievement in developing countries (JPAL, 2019). Furthermore, there could be important cumulative effects in cases where students are exposed to a combination of different pollutants.
Obtaining convincing estimates of the causal impact of air pollution on student performance is a difficult endeavor. There are two major obstacles. The first is the presence of confounding factors resulting from residential sorting that is correlated with both air and school quality. The second is that there is a paucity of air pollution measurements and of student test score data readily available. By merging administrative student achievement records with data that we scraped from the web, we were able to create a cross-sectional pooled dataset that allows us to control for schooland time-unobservable constant characteristics that could bias our estimates. Nonetheless, we provide sufficient evidence and robustness checks to suggest that air pollution has a negative and statistically significant impact on student achievement, a relation that is particularly robust in the cases of math and science.
Notice that not all pollutants have a statistically significant negative sign. One reason could be the low levels of pollution during November in the city of Santiago. Thus, air quality standards for pollutants such as CO and NOX, which show a negative effect, should be revised. In addition, given the specific environmental conditions of Santiago, poor neighborhoods tend to have lower air quality levels. We rarely think of air quality distribution as an inequality concern in education, but our results suggest that pollution could be an important factor in the academic achievement gap observed across students of different socioeconomic backgrounds.
All this suggests two straightforward policy recommendations. First, the air quality standards for CO and NOX should be revised. The data show that even when concentration levels of these pollutants are low, during November (Spring in Chile), they may have a negative impact on student achievement. In addition, these pollutants reach higher levels during winter and tend to be present at higher levels in poorer towns. Thus, there are some inequality concerns that should be addressed. One short-term policy for this would be to create a compensation scheme to benefit schools located in those towns. These benefits might consist of providing complementary health insurance or improving the quality of the food supply for these students. In the longer run, sources of pollution should be moved away from all schools, in a joint effort of the private sector and the government. In the mediumand long-term improving the academic performance of thousands of students will pay off in terms of increased tax revenues for government and growing sales for companies.
Finally, future research on this topic should aim to understand the long-run effects of pollution on student achievement and, consequently, on wages. The challenge to exploring this question lies in the difficulty of obtaining a panel dataset containing information on pollution, education and wages for different cohorts of people.