Introduction
Forest inventory methods are subject to constant changes that allow reducing costs and destructive effects while achieving accurate estimations. Conventional methods for biometric estimations are usually derived from destructive sampling, with a subsequent negative impact on the ecosystem. In addition, they use empirical models that require manual measurements, which are costly and inaccurate (Peña et al., 2018).
Unmanned aerial vehicle (UAV) technology has great potential as a suitable tool for the scientific community (Brede et al., 2019). Its use avoids destructive sampling and excessive time consumption, including high spatial and spectral resolutions. Previous studies in Mexico and other places around the world have used this technology in plantations, where there is a regular arrangement of individual trees (Peña et al., 2018; Gallardo-Salazar et al., 2021). However, there is limited or no research whatsoever in Mexican natural forest stands because of their structural complexity. UAVs make it possible to obtain digital images with increased spectral and spatial resolutions (Tu et al., 2019), and UAV digital color images have been increasingly applied in vegetation analysis due to their advantages (high resolution, high frequency, and easy operation) (Skorobogatov et al., 2019). Thus, information from UAV digital color images can be related with some field information for individual trees and stand variables.
It is well known that forest biomass includes the largest carbon sinks in terrestrial ecosystems (Ciais et al., 2013; Sinha et al., 2015). Even so, traditional estimates of forest inventories based on allometric relationships remain a challenge in forest modeling (Chave et al., 2014). For instance, tree height is a typical parameter to quantify the commercial and ecological value of a stand, since it allows calculating the volume, biomass, and carbon contents, besides being an indicator of site quality (Krause et al., 2019). However, to the best of our knowledge, in Mexican forests, this variable is still estimated with field techniques based on trigonometric principles, which is time consuming and biased. Particularly, the estimation accuracy usually depends on the technician's experience, with the error that implies over- or underestimating reality in irregular mixed-species and high-density forests.
In northern Mexico, Ejido El Brillante, municipality of Pueblo Nuevo, state of Durango, has a great diversity of species and provides several forest ecosystems services that contribute to forest conservation and the social economy of its inhabitants. Thanks to their constant search for appropriate actions and policies, this Ejido has a sustainable management certification (Figueroa et al., 2013). Besides, it has been open to accept and promote modern technologies to refine the knowledge of its forest stands and improve their productivity.
The aim of this study was to assess parameters such as canopy height (CH), diameter at breast height (DBH), basal diameter (BD), above-ground biomass (AGB), volume (V), and carbon contents (C) using estimations via allometric equations, all of them based on the automated valuation of tree height (THUV) measured from UAV images. It was hypothesized that THUV is a statistically reliable predictor of such attributes.
Materials and Methods
Study area and data acquisition
The study area is located inside Ejido El Brillante, in the municipality of Pueblo Nuevo, Durango, Mexico. It is considered to be a site of great ecological, economic, and environmental importance. Its vegetation includes a mixture of pine and oak species with similar ecological preferences. The dominant elements of the vegetation are various species of pine (González et al., 2012), like Pinus cooperi C.E. Blanco, coexisting with P. teocote Schied. Ex Schltdl. and Cham., P. durangensis Martínez, P. leiophylla Schiede ex Schltdl. and Cham., and P. strobiformis Engelm. Figure 1 illustrates the geographical location of the study area.
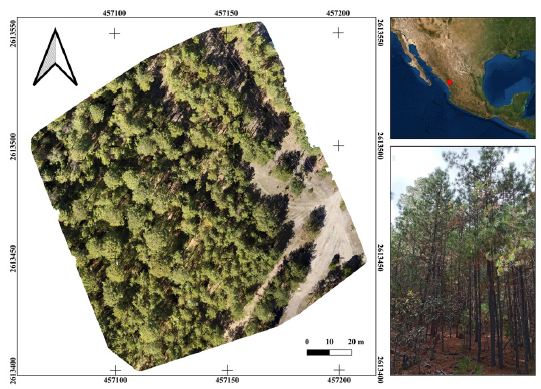
Source: Authors
Figure 1 Location of the study area corresponding to Ejido El Brillante in northern Mexico
In a previously selected forest area, data were collected at ground level over a delimited 100 x 100 m plot. The following variables were recorded for each tree (DBH > 7 cm): diameter at breast height (DBH, cm) and diameter at the base of the tree (DB, cm), measured with a diametric tape; height at the base of the live crown (HLC, m) and total height (TH, m), measured rigorously, mainly telescoped and in some cases involving tree climbing; and the lower tree crown width (CDM, m) and higher tree crown width (CDG, m), taken with measuring tape to calculate the average tree crown area (CA, m2). The distribution of the measured variables and their relationship with UAV images is represented as a flow chart in Figure 2.
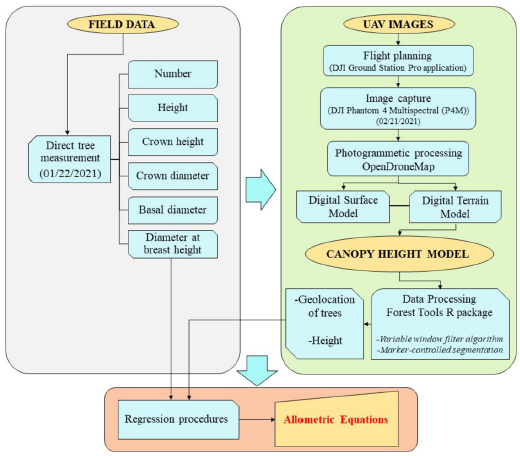
Source: Authors
Figure 2 Flow chart of stages of field measurements and unmanned aerial vehicle (UAV) image pre-processing to generate allometric equations
Using DBH as a predictor variable, the above-ground tree volume and biomass were estimated using allometric equations, which had been previously done by Návar (2009) in the forests of northwestern Mexico. Biomass estimates were transformed into annual carbon accumulation (C), whose content was calculated while assuming a 50% concentration (Rodríguez et al., 2016).
A flight was conducted to obtain and compile aerial photographs of the study area, with overlaps between the images and lines of 80 and 75%, respectively. This flight took place on February 21, 2021, which was a sunny day, with favorable wind conditions (<25 kph) and temperatures of 14-25 °C. A DJI Phantom 4 Multispectral (P4M) quadcopter was flown over the study area. The P4M camera has a total of six imaging sensors: five multispectral sensors (i.e., blue, green, red, red-edge, and near-infrared bands) and 1 RGB sensor, all with a 2 MP global shutter. The focal length of the P4M camera is 5,74 mm, the image size is 1600 x 1300 pixels, and the sensor size is 4,87 x 3,96 mm. The flight plan for automatic image collection was programmed with the DJI Ground Station Pro application (www.dji.com/mx/ground-station-pro).
A quadruple grid was established (Figure 3), covering the study area corresponding to 1 ha. The flight height was 70 m above ground level, with an angle of 90° for the first two grids and 70° for the last two grids.
Since the P4M operates under the principle of direct onboard georeferencing, it was not necessary to geo-reference the images; this quadcopter has a Global Positioning System (GPS) that geotags the coordinates of each image taken. Once the images were captured, photogrammetric and computer vision procedures were applied using the free and open-source OpenDroneMap software (ODM, www.opendronemap.org).
Data processing
For data processing, this study employed a computer with an AMD Ryzen 3900x processor, with 24 cores at 3.8 GHz, an integrated NVidia Quadro p620 quadcore 2 GHz video card, and 32 GB of RAM, running a Linux operating system based on Ubuntu, i.e., Pop!_OS version 22.04 lts.
A photogrammetric procedure was applied to the captured RGB images using ODM. This software uses the modern Structure from Motion (SfM) and Multi-View Stereo (MVS) algorithms, which generates a 3D point cloud of 1 000-20 000 points m-2 (Puliti et al., 2020), producing a digital surface model (DSM) and a digital elevation model (DTM), which are in turn used to orthorectify each image and construct an orthomosaic. Finally, the total tree height (canopy height model) was calculated from the elevation difference between DSM and DTM, detecting the top of the trees. This process was performed using the RStudio statistical software (R Core Team, 2020) and applied to the elevation models calculated by two methods (RGB and multispectral images). The P4M operates under the principle of direct onboard georeferencing, so the acquired images were directly georeferenced through GPS capabilities during the course of the flight. It was not necessary to use the realtime-kinematic (RTK) system, since the P4M manufacturer reported that the georeferencing system can reach vertical and horizontal location accuracies of ±0,1 and ±0,3 m, respectively (https://www.dji.com/p4-multispectral/specs).
The ForestTools package of the R statistical software (R Core Team, 2020) allowed calculating the height (H, m) and other parameters useful for modeling individual attributes. The vector and raster files created in R studio were processed in the free and open-source QGIS 3.16.2-Hannover software.
From 30 randomly selected trees, the estimates of the tree-level attributes obtained with the UAV were compared to those rigorously measured in the field. First, all the variables were correlated to identify linear relationships in order to explore statistic correspondences between attributes. Through linear regression procedures, allometric equations were generated (Equation 1); the fulfilment of regression assumptions was verified (Gujarati et al., 2012).
The summary description of the variables used in the regression is shown in Table 1, while the correlation matrix for the variables is illustrated in Figure 4.
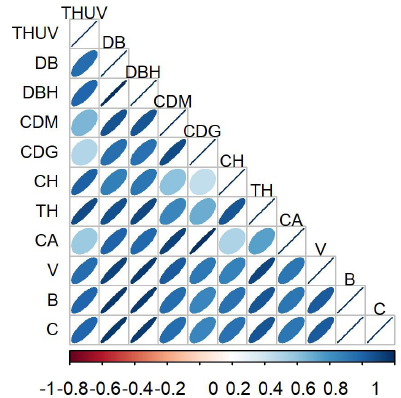
Note: The highest correlation was observed for THUV-TH relationship (p = 0.8979) and poorest for THUV-CDG (p = 0.2930). THUV = tree height measured from UAV; DB = diameter at base height (cm); DBH = diameter at breast height (cm); CDM = lower tree crown width (m); CDG = higher tree crown width (m); CH = tree crown height (m); TH = total tree height (m); CA = tree crown area (m2); V = calculated tree volume (m3); B = calculated biomass (kg); C = calculated carbon content (kg).
Source: Authors
Figure 4 Correlation matrix of studied variables
In order to test the goodness of fit, the determination coefficient (R2), the root mean square error (RMSE), and Akaikes's information criterion (AIC) were considered as fitting statistics for model classification. Although there are multiple regression approaches (Gujarati et al., 2012), a linear regression model (Equation 1) was used to relate the total tree height measured from UAV (THUV) with other individual tree variables.
where yi is the dependent variable (THUV) vector; xi represents the matrix of values of independent variables for the studied relationships (DB, DBH, CDM, CDG, CH, TH, CA, V, B, C); βi (i = 0,1) is the vector of the intercept and slope parameters; and εi represents the error vector [εi ≈idd N(μ,g)].
Table 1 Descriptive statistic for used variables in the correlation and regression analysis
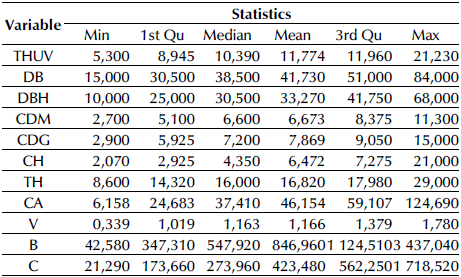
Note: THUV = tree height measured from UAV images; DB = diameter at base height (cm); DBH = diameter at breast height (cm); CDM = lower tree crown width (m); CDG = higher tree crown width (m); CH = tree crown height (m); TH = total tree height (m); CA = tree crown area (m2); V = calculated tree volume (m3); B = calculated biomass (kg); C = calculated carbon content (kg); Qu = quantile.
Source: Authors
The ten allometric relationships were fitted by lm in RStudio (R Core Team, 2020), and the RMSE, R2, and AIC fitting statistics were calculated with the residuals of each model.
Results
A total of 479 RGB images were collected and processed to generate the digital elevation model (DEM) and the digital surface model (DSM), whose difference resulted in the canopy model via the ODM algorithm (Figure 5).
Since the height obtained by the UAV (THUV) explains the height measured in the field (R2 = 95%, RMSE = 0,36 m), this was the explanatory variable that produced the results of Table 2 for the top six relationships (CH-THUV, B-THUV, C-THUV, DBH-THUV, DB-THUV, and V-THUV). The highest accuracy was achieved by the CH-THUV relationship, and the poorest one by the V-THUV relationship. The tree crown height can also be estimated as a function of the tree height measured from UAV images. This allometric relation had R2 = 0,6567 and values of 3,166 m and 137,6294 for the RMSE and AIC statistics, respectively. Regarding the top six allometric relations, only for the DBH-THUV and DB-THUV models, the intercept parameters are not significant (equal to zero at a 5% significance level).
According to the fitting statistics, CH is an attribute remarkably well modelled by the THUV, with a 65% of the total variability explained by the model. Furthermore, the THUV acceptably predicted B, C, and V with R2 > 0,61, and DBH and DB with R2 > 0,57. The statistical significance values (Pr(>| t |)) strengthen the assertion (Table 2), indicating a non-random relationship. The linear relationship between the numerical predictors was also verified, where the residuals were randomly distributed around zero, with constant variability along the x-axis. The rest of the variables had no satisfactory models (Figure 6).
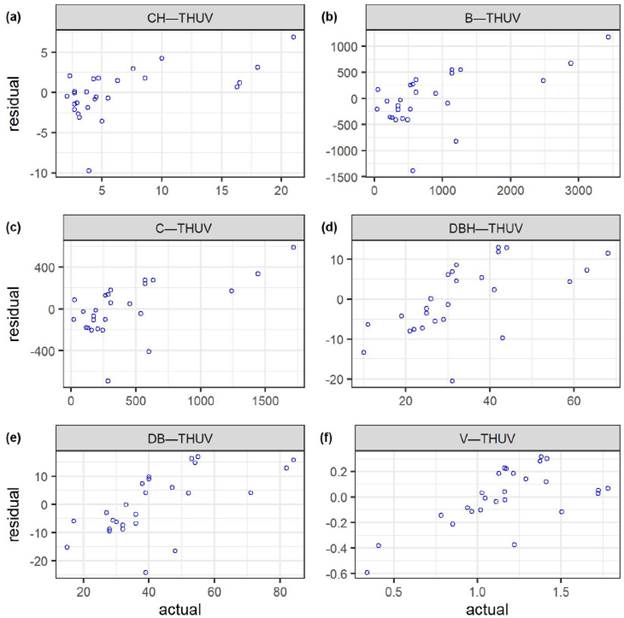
Note: THUV = tree height measured from UAV; CH = tree crown height (m); B = biomass (kg); C = calculated carbon content (kg); DBH = diameter at breast height (cm); DB = diameter at base height (cm); V = tree volume (m3): (a) CH-THUV relationship; (b) B-THUV relationship; (c) C-THUV relationship; (d) DBH-THUV relationship; (e) DB-THUV relationship; (f) V-THUV relationship.
Source: Authors
Figure 6 Residuals of the top six relationships
Discussion
The findings of our study indicate that tree height is one of the parameters that can be best estimated with UAVs. This result agrees with Krause et al. (2019) and Karpina et al. (2016). As it is well known, the maximum error allowed for a typical forest management inventory is 10% of the standing volume, considering the entire forest property, which is valid for practical purposes (SEMARNAT, 2006).
Total height estimation becomes relevant because it is rarely subject to precision evaluations, knowing that, in dense forests stands, it is difficult to visually detect this variable from the ground. The occlusion of branches, neighboring trees, and the ruggedness of the relief complicate the inspection of the top of the trees and, consequently, its precise estimation, so it usually depends on the good judgment and experience of the forest technician. Although the aim of this study was not to evaluate the quality of the estimations made by technicians, it is evident that indirect measurements lead to a bias that should be reduced. This error is exacerbated when the data have been obtained by several different technicians, as well as when the forest stands are very dense. Thus, an important limitation is that automated tree height detection was disrupted by the presence of overlapping crowns. Consequently, in the near future, we suggest including more sophisticated sensors to prevent this. Drones can have an important role in forest management, but progress is incremental.
Quantitative analysis during data processing made it clear that the accuracy of the estimations is highly dependent on the density of points to generate the cloud, as previously documented in Krause (2019). Therefore, we recommend increasing the number of flight lines and testing different camera settings according to technical possibilities. It is known that the application of Structure from Motion (SfM) algorithms works by matching equal pairs of points located on overlapping images, and the correct generation of output results depends on the quantity of points in the cloud. For instance, Peña et al. (2018) argue that a good quality of the UAV images allows for the easy detection of such points, which could be improved by optimizing the values of image contrast, saturation, and brightness. The correct measurement of height has direct implications in the calculation of volume, biomass, and carbon content, among others, which has a direct impact on decision-making for forest management. From a practical point of view, this strategy allows enhancing the use of individual tree-level attribute measurements, saving time and improving certainty.
For the estimation of CH, DB, DBH, V, B, and C based on height (THUV), it was found that the models explain more than 57% of the variability in the data. It is advisable to perform a stratification of crown size classes because it is well known that dimensions and arrangement of branches play a major role in the estimation of volume (Gallardo-Salazar and Pompa-García, 2020).
These findings can contribute to global efforts to understand the magnitude of carbon contributions to ecosystem dynamics (Dubaya et al. 2020). For example, Brede et al. (2019) report that global estimates are biased, requiring validation of pilot sites for calibration. Thus, stand datasets such as this one, with high resolution and high heterogeneity, are ideal for this purpose. The ability to acquire information from multispectral remote sensing potentially broadens its application, given the large amount of low-cost data collected in a short period of time. In that sense, our data collection and processing appeared to be efficient (e.g., an experienced team would require 3 to 6 days for a 1,0 ha plot) and to be consistent with similar research (Wilkes et al., 2017).
Table 2 Parameter estimates, standard error, and fitting statistics for the studied relationships
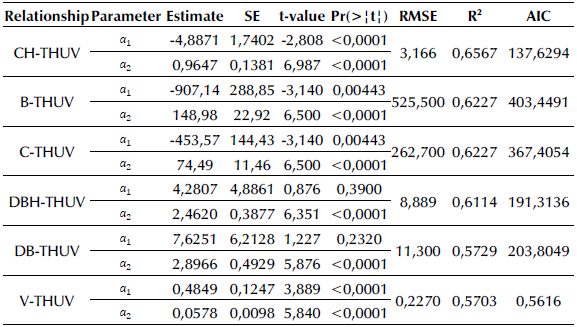
Note: THUV = tree height measured from UAV images; DB = diameter at base height (cm); DBH = diameter at breast height (cm); CH = tree crown height (m); V = tree volume (m3); B = biomass (kg); C = calculated carbon content (kg). SE = standard error of parameters; RMSE = root mean square error; R2 = coefficient of determination; AIC= Akaike information criterion.
Source: Authors
The efficient application of these technologies to large forest areas would depend on benefit-cost ratios in the framework of forest management programs. For those interested in replicating these technologies, it is advisable to seek partnerships, with the consequent benefit of generating multipurpose knowledge. For example, the resulting products offered at stand level by the sensors used in this work seem to promise attractive perspectives that should be addressed in future studies (phenology and stand dynamics, among others).
The challenge posed by constructing tree geometry and counting at the individual tree level in the whole forest seems to be a limitation of this study when compared to regular plantations (Tu et al., 2019). The cameras of our UAV have limitations, such as low efficiency in closed forest areas due to the relatively poor penetrating capacity of natural light. Consequently, the allometric equations could improve the estimations of forest attributes. However, in future research, tree metrics could be estimated by combining many types of equations and several regressions (Chave et al., 2014; Gujarati et al., 2012). As shown by the literature (Lin et al., 2018; Jones et al., 2020), accurate individual tree attributes extraction is improved by choosing tree height as predictor variable. Nevertheless, we recognize that, even though the UAV's image-based total height estimations were very capable predictors, there are still a lot of challenges for better predicting tree attributes. As a complementary strategy, it is advisable to complement the current efforts with different sensors such as terrestrial laser scanning, including a larger sample size with sophisticated remote sensing platforms and other modeling strategies (Brede et al., 2019; Gao et al., 2021). To the best of our knowledge, this is the first study in Mexico that documents the generation of allometric equations in natural forests upon the basis of UAV images.
Finally, we recognize that, although destructive methods for estimating biomass and carbon content are often reported to be reliable (Karpina et al., 2019), our results offer the advantage of being able to make faster and less expensive re-measurements and adjustments depending on stand structure and dynamics. Under a multitemporal perspective, the periodical re-measurement of forest attributes also represents a source of indicators on the response of site productivity to the hydroclimatic variations faced by forests (Pompa-García et al., 2021). Thus, our findings, in terms of the use of total height measured using UAVs (THUV), offer a promising future in forest resource management towards precision forestry.
Conclusions
This study reports that height measurements from unmanned aerial vehicles (UAVs) show proficiency in estimating tree measurements in an unevenly aged pine stand. Currently, direct tree height measurement represents a large operational effort. However, it is possible to generate efficient biometric height estimates using UAV images while avoiding destructive sampling. The long times required for individual field estimations are considerably reduced by our methodology. Its extensive implementation depends on the technical and financial possibilities of forest managers, so institutional partnerships are recommended. It was also found that increasing flight missions produce better resolutions, which translates into better possibilities for periodic and permanent monitoring, in order to generate long-term series. Our estimates of biomass, volume, carbon content, and diameters can be calibrated and validated in future studies, which could also support mission estimates on a sort of hectometric-scale.
Despite the promising results, certain limiting factors that merit further research can be highlighted. For instance, a range of models and deep statistical analysis are important, as well as highly technical requirements and trained personnel. It is therefore advisable to test more sophisticated approaches.