To study silent speech by means of electroencephalographic (EEG) signals, voluntary signals are used, which are produced autonomously by the person, a method that is a signal analysis alternative and is currently explored within the Brain-computer Interface (BCI) research area. EEG signals are easy to record. They have a high temporal resolution and are obtained non-invasively. Studies show that silent speech tasks, such as thinking or imagining vowels, syllables, or words mainly involve the Supplementary Motor Area (SMA) (DaSalla et al., 2009; Iqbal et al., 2015; Nguyen et al., 2018; Qureshi et al., 2018) and the Language Areas (Broca and Wernicke areas) located in the brain’s left hemisphere (Ikeda et al., 2014; Morooka et al., 2018; Sarmiento et al., 2021; Villamizar et al., 2021).
In this line of study, researchers seek to design and develop communication systems using BCIs, to favour the quality of life of persons with some type of language disability (Callan et al., 2000; D’Zmura et al., 2009; DaSalla et al., 2009; Fujimaki et al., 1994; Lee et al., 2021; Morooka et al., 2018; Qureshi et al., 2018). Thus, the use of EEG to study silent speech has gained momentum in recent years.
Research shows promising results in understanding and explaining vowel, syllable, and word decoding, among others (Cooney et al., 2018; González-Castañeda et al., 2017; Qureshi et al., 2018; Sarmiento et al., 2014; Yoshimura et al., 2016). Some studies take into account the potentials evoked in imagined silent speech tasks, which consist of internally simulating a motor movement without moving the body in any way (Fujimaki et al., 1994). In the case of speech imagery, the person has to imagine moving parts of the speech apparatus such as: tongue, lips, or lower jaw (Graimann et al., 2010). This task requires high concentration and training time from the person, therefore, fatigue in this type of tasks is frequent. In this line of work, evoked potentials are used for the processing of EEG signals, which include: Event Related Potential (ERP), Evoked Potential (P300), Movement Related Cortical Potential (MRCP), and Steady State Evoked Potentials (SSEP) (Rashid et al., 2020).
According to Martín et al. (2014), silent speech, imagined speech, covert speech, and inner speech are used in the same way, when a person thinks of a vowel, syllable, or word without the intentional movement of speech apparatus such as the lips or tongue. In this article we preferably use silent speech (Martin et al., 2014). In silent speech, EEG is used to decode the English vowels /a/ and /u/ (DaSalla et al., 2009; Iqbal et al., 2015), /a/, /i/, and /u/ (Nguyen et al., 2018) and /a/, /e/, /i/, /o/, /u/ (Ghosh et al., 2019) ; Korean vowels /a/, /e/, /i/, /o/, and /u/ (Min et al., 2016), syllables /ba/ and /ku/ (D’Zmura et al., 2009); words /go/, /back/, / left/, /right/, and /stop/ (Qureshi et al., 2018).
In contrast to this, other studies take into account the processing of voluntary signals for the study of silent speech, which is generated in the language area (Broca & Wernicke) (Ikeda et al., 2014; Morooka et al., 2018; Villamizar et al., 2021). Imagined speech is considered as the internal pronunciation of phonemes, words, or sentences, regardless of the movement of the phonatory apparatus and without any audible output (Cooney et al., 2020). Thinking of silent speech with electroencephalographic signals is characterised by the fact that persons do not require specific training processes and tasks are almost always performed using the native language. Therefore, it does not require high levels of training and attention which fatigue a person. (Fujimaki et al., 1994; Graimann et al., 2010). In this line of research, EEG is used to decode Spanish vowels /a/, /e/, /i/, /o/, /u/ (Sarmiento et al., 2014); Japanese vowels /a/, /i/ (Yoshimura et al., 2016). and /a/, /i/, /u/ (Ikeda et al., 2014). In the present research, this brain signal was selected because the person can generate this type of signal at will, unlike evoked potentials such as P300 or SSVEP, which depend on external stimuli.
Most studies developed based on EEG and BCIs, to identify silent speech processing, conventionally use different groups of brain rhythms in the frequency domain such as: delta, theta, alpha, beta, and gamma with linear and stationary characteristics, which require algorithms to identify based on brain signals (DaSalla et al., 2009; Matsumoto & Hori, 2014; Riaz et al., 2015; Sarmiento et al., 2014). However, in this line of research, recent studies focus on the use of methods which consider non-linear and non-stationary signals; inherent to electroencephalographic signals.
To study these signals, the following methods are used: empirical mode decomposition (EMD) (Hansen et al., 2019), multivariate empirical mode decomposition (MEMD), and recently, the method referred to as adaptive-projection intrinsically transformed MEMD (APIT-MEMD) (Hemakom et al., 2016; Villamizar et al., 2021; Yuan et al., 2018). Despite the studies conducted, there is still no consensus among the academic community regarding which brain rhythms are the most appropriate for identifying and processing silent speech or which signal processing methods are the most effective (Fujimaki et al., 1994; Morooka et al., 2018; Qureshi et al., 2018).
A clear understanding and explanation of the fundamental processes of silent speech processing has not yet been achieved. It is likely necessary to consider some of the differential characteristics of the subjects when they process information, which may be associated with cognitive style, and in this regard, studies show that there are individual differences in cognitive information processing (Evans et al., 2013; López-Vargas et al., 2020; Solórzano-Restrepo & López-Vargas, 2019).
Specifically, it is noteworthy, that probably, the most studied cognitive style is the one referred to as Field Dependence/Independence (FDI), proposed and developed by Witkin and his colleagues (Witkin, H. A. et al., 1977). FDI establishes a difference between persons with a tendency towards an analytical-type processing, regardless of contextual factors (field independent persons (FI), and those with a tendency to a global-type processing, highly influenced by the context (field dependent persons (FD).
FI persons follow an analytical information trend, fact which allows them to break down the information into its different components and restructure it according to their needs. In addition, they have strategies to organise, classify, and store information, and they resort to different clues in order to retrieve it later. For their part, FD persons are more sensitive to external signals and tend to receive the information as it is presented to them. In other words, they prefer externally structured information and address its global aspects (López et al., 2012; Valencia-Vallejo et al., 2019).
Within this area of research, electroencephalography is promising. For example, using EEG, it was found that FI persons exhibit less coherence between hemispheres, which indicates a greater hemispheric specialisation (Oltman et al., 1979; Zoccolotti, 1982). Also, when developing visual tasks and exercises related to auditory and somatosensory aspects, it was determined that FI persons show a greater neuronal activity for executive and inhibitory response processing. These studies support the idea that FI persons possess better inhibitory control (Imanaka et al., 2017; Jia et al., 2014).
Along these lines, few studies inquire into the possible relationships that may exist between the processing of voluntary signals in imagined speech and a persons’ stylistic characteristics when performing silent speech tasks using vowels. Understanding and explaining a persons’ individual differences, within the study of silent speech, may aid in the design of BCIs that favour the quality of life of people with some type of language disability. Consistent with these approaches, this study hereby poses the following research question:
Are there any significant differences in silent speech production using the vowels /a/-/u/ between persons with different cognitive styles in the Field Dependence/Independence (FDI) dimension?
This research proposes considering a subject’s cognitive style in the FDI dimension and using intrinsic mode functions (IMF) produced by APIT-MEMD to choose the signals related to silent speech using vowels. Also, the combination of the APIT- MEMD method with power spectral density (PSD) is used to analyse the data captured from EEG signals. The APIT-MEMD method allows for multivariate separation, over time, of brain signals that have non-linear and non-stationary characteristics.
In addition, the aim is to identify the best location for the electrode that enables generating higher energy levels in the PSDs of 14 electrodes placed on a neuroheadset and arranged in a matrix especially designed for the language region in silent speech tasks using vowels.
In this order of ideas, the objective of the research is based on determining whether the cognitive style in the FDI dimension of people, when performing silent vowel speech tasks, generates significant differences in the energy levels of the PSDs and also, to establish precisely the areas of the language region where these energy levels are maximal in order to take them into account when designing BCIs.
Method
Participants
This study involved 51 undergraduate and postgraduate students (21 women and 30 men), one group enrolled in a university in the city of Bogotá-Colombia and another group enrolled in a university in the city of Popayán-Colombia. Ages ranged from 18 to 41 years (M = 24.76, SD = 7.66). Participants did not exhibit any type of medical or neurological problem. All persons involved gave their written informed consent and the experiment was approved by the Ethics Committee of the Universidad Nacional de Colombia.
Experimental Protocol
The experiment was carried out in the Laboratory of Cognition and Intelligent Systems of a public university in the city of Bogota-Colombia, under controlled lighting conditions of 80 lm/m2 and minimum ambient sound (ASTM STC 63). First, the subjects were given an embedded figures test (EFT) to determine their cognitive style in the FDI dimension. Subsequently, each of them was asked to sit in a comfortable chair and was fitted with an EEG neuroheadset, and the 14 electrodes were placed on the scalp located over the Broca, Wernicke, and motor areas in the left hemisphere. Two reference electrodes were also placed on the frontal region. An abrasive gel was used to clean the scalp before placing each electrode.
The placement of the electrodes was done according to the neurological models on language by: Geschwind (1965) and Poeppel and Hickok (2004). To reference the neuroheadset on each subject’s head, the T3 and C3 positions were used according to the 10-20 system (Figure 1). Finally, a light source was placed at a distance of one meter from the person to signal the time to begin the task of thinking of a specific vowel with silent speech, and also, to end the activity. During the experimental phase, persons were asked to keep their eyes closed to reduce artifacts, such as blinking and eye movement, while developing the task.
Signal Acquisition
Each person was told that as long as the light source was turned on, they were to think about the corresponding vowel continuously and with silent speech. They were also told that when the light source was turned off, they had to stop thinking about said vowel and go into a state of bodily relaxation. During the experiment, the light source was kept activated for four seconds and then, it was turned off for three seconds. The procedure was repeated 25 times for each of the two vowels. Between each silent speech task, the subjects rested for 5 minutes before proceeding to the next vowel change. The vowels were arranged in the following order: first /a/; and second /u/. Although some authors suggest that data collection in EEG signals should be taken randomly (Li et al., 2018), other researchers suggest performing the data collection of vowels or syllables with EEG signals in an orderly and deterministic way (Cooney et al., 2020; Pressel Coretto et al., 2017). The sampling intervals in silent speech were (385-896, 1281-1792, ...) (Figure 2).
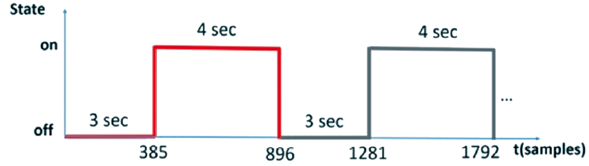
Figure 2 Time intervals for the experimental process. During the intervals (385-896, 1281-1792, ...), the subject thought silently about the corresponding vowel. During the intervals (1-384, 897-1280, ...), the person was in a state of relaxation.
The signals were recorded with a 14-channel EMOTIV EPOC+ amplifier, with a sampling frequency of 128 Hz, a 14-bit resolution with 1 LSB with 0.51 m V in monopolar configuration. The 14 electrodes of the EMOTIV EPOC+ device were arranged on the neuroheadset (E1, ..., E14) considering the name of each electrode of the EMOTIV EPOC+ device. For this experiment, the electrodes are numbered from E1 to E14 and the relationship with the name of the Emotiv electrodes is as follows: E1 (AF3), E2 (F7), E3 (F3), E4 (FC5), E5 (T7), E6 (P7), E7 (O1), E8 (O2), E9 (P8), E10 (T8), E11 (FC6), E12 (F4), E13 (F8), E14 (AF4). The two reference electrodes were placed on the subject’s forehead (Sarmiento et al., 2021; Villamizar et al., 2021) (Figure 1).
To export the data in MATLAB’s Simulink, Xcessity EpocSimulinkImporter acquisition software was used. Brain signal processing was performed with MATLAB R2016 software (The MathWorks, Inc., Natick, MA). Subsequently, data analysis was carried out using the Statistical Package for the Social Sciences (SPSS) Version 25 software.
To filter the EEG signals, the APIT-MEMD method has been selected, which is particularised by allowing the separation of multivariate, non-linear and non-stationary signals, into components called IMFs (Hemakom et al., 2016). The central concept is based on assuming that the data or signals are composed of simple intrinsic modes of oscillation that are characterised by having the same number of extremes and the same number of zero-crossings. These intrinsic modes of oscillation are called intrinsic modes of function (IMF). The APIT-MEMD algorithm has got the following steps: First, it calculates the covariance matrix and the eigenvalues of the multivariate signal; Second, it selects the first principal component related to the highest eigenvalues; Third, it develops a uniform Hammerseley sequence over an n-sphere; Later, it calculates the projection of the vectors in order to calculate new mean enveloping curves; Finally, with an iterative process, the designated MFIs are found (Hemakom et al., 2016).
For this research, brain signals were processed with the APIT-MEMD method, where p = 14 electrodes (E1, … E14) located over the left hemisphere. The results of this algorithm are multivariate IMFs related to p electrodes, v vowels with silent speech, and m multivariate levels.
In other words, power spectral density (PSD) is a statistical measure that quantifies the power of a signal based on a finite group of data. One outstanding application of PSD is the detection of signals immersed in noise (Sarmiento et al., 2014). Subsequently, the power spectral density (PSD) of each brain signal was determined, for which the periodogram (X p s, v (f)) was used, which determines the energy levels of each IMF using equation 1 (Li & Wong, 2013; Proakis & Manolakis, 2007).
Based on the above equation, S is the person, v is the vowel, p is the electrode, A is the width of the window, IMF is the multivariate function resulting from the APIT-MEMD, m is an IMF level, and n are the samples to be analysed. In this case, the number of IMFs analysed was 10 (Figure 3).
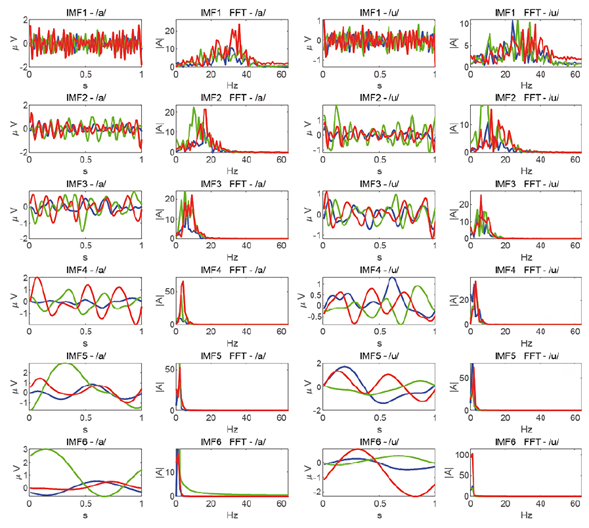
Figure 3 Shows the first 6 IMFs and their respective frequencies for the E3 electrode, with a sample of 1 second, for a FD subject (blue color), an INT subject (green color) and a FI subject (red color), in speech imagery tasks. The first column corresponds to the IMFs of the silent speech /a/. The second column corresponds to the Fourier transform of the previous IMFs. The third column corresponds to the IMFs of the silent speech /u/, and the fourth column corresponds to the IMFs Fourier transform of the silent speech /u/. In the Fourier transform for IMF 4 of /a/ and /u/, delta and theta waves are presented among 2 to 8 Hz.
Subsequently, through a mixed repeated measures analysis, each IMF was analysed to determine the brain rhythms most related to silent speech and it was found that the multivariate IMF with the greatest significant differences corresponded to level 4. Finally, the PSD data were averaged among the participants to obtain the overall PSD average and thus proceed with the mixed repeated measures analysis (Figure 3).
Cognitive Style Test
The test used to determine cognitive style in the FDI dimension was Witkin’s Embedded Figures Test (GEFT). This perception test requires the person to locate a simple, previously seen, figure within another figure with a complex design. The test contains 18 complex figures. The task is to find the simple figures in a given amount time. The test lasts approximately 20 minutes. A version of the online instrument has been given to Colombian students (Hederich-Martínez et al., 2016). The test showed a Cronbach’s alpha of 0.847.
Results
The samples’ EFT average was 10.59; the standard deviation (SD = 3.656). Out of a maximum score of 18; the minimum value was 1 and the maximum value was 18 points. The subjects were grouped into FD, intermediate (INT), and FI, defining terciles for the total test score. Thus, three ranges of scores were identified, namely: (a) 17 FD persons (first tercile), (b) 21 INT persons (second tercile), and (c) 13 FI persons (third tercile).
A mixed repeated measures ANOVA analysis was used. The two intra-subject variables are: (1) Thinking of vowels with two values; /a/ - /u/ and (2) PSD recorded by EEG from 14 electrodes (E1, E2, …E14). The inter-subject variable was the cognitive style with three values; field dependent, intermediate, and independent. Table 1 shows a summary of the descriptive statistics of the PSDs recorded for each vowel from the 14 electrodes, considering cognitive style.
Table 1 Silent Speech PSD results for vowels /a/ - /u/ from each electrode: Mean scores and standard deviations in parentheses
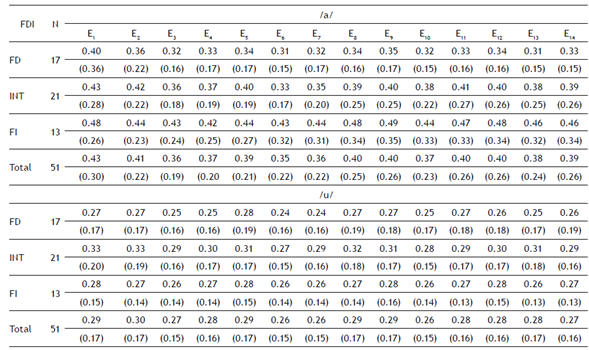
Mauchly’s test indicated that the sphericity assumption was not met. The data show that the main effect of PSDs on electrodes is: (X2(90) = 847.91, p < 0.05). Therefore, the degrees of freedom were corrected with Greenhouse-Geisser (ε = .17). The multivariate tests indicate that there are significant differences in silent speech using vowels /a/ - /u/ (Pillai’s Trace = 0.190, F (1,48) = 11.27, p = 0.002, η2 = 0.190) and electrode PSDs (Pillai’s Trace = 0.553, F (13,36) = 3.42, p = 0.002, η2 = 0.553). However, there are no significant double interactions between the intra-subject variables and between the intra-subject and inter-subject variables, suggesting that the PSDs recorded from the 14 electrodes depend on the silent speech of the vowels (Figure 4). In figure 4, estimated marginal means is presented for vowels /a/ and /u/. This figure shows that there are significant differences for the 14 EEG electrodes. Figure 5 and 6 shows a topographic map with the 14 electrodes, for a subject and a sample of silent speech vowels /a/ and /u/. Colors between 0 (blue) and 0.4 (yellow) indicate the value of PSD. (Figure 5 and 6).
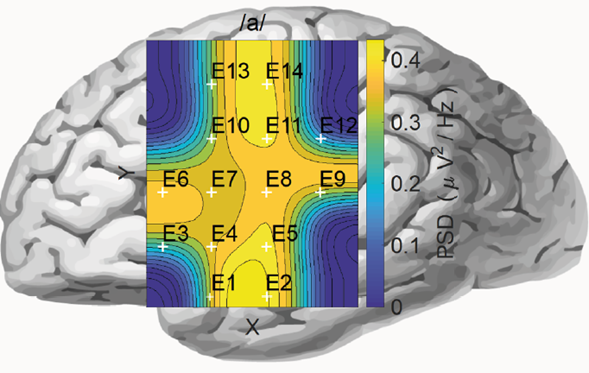
Figure 5 Topographic map of PSD overall average, done with cubic splines, for all persons during silent speech tasks for the vowel /a/. In the figure, the yellow color’s intensity represents the highest PSD levels (μV2/Hz) and the blue color’s intensity represents the lowest PSD values. The location of the electrodes is denoted by E1, E2, ..., E14
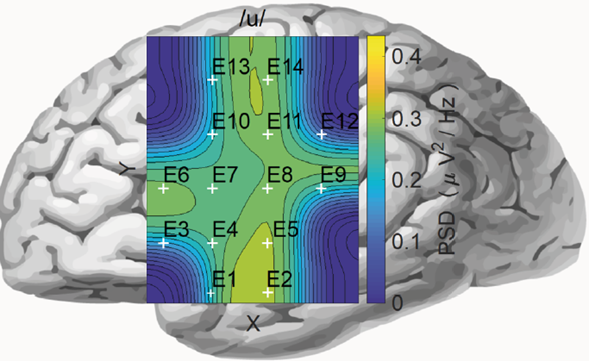
Figure 6 Topographic map of the PSD overall average, made with cubic splines for all persons during silent speech tasks for the vowel /u/. In the figure, the yellow color’s intensity represents the highest PSD levels (μV2/Hz) and the blue color’s intensity represents the lowest PSD values. The location of the electrodes is denoted by E1, E2, …, E14
With regard to intra-subject contrast tests for the vowel variable /a/ - /u/, the data show that the average PSD values for vowel /a/ (M = 0.39) differ from the average PSD va-lues for vowel /u/ (M = 0.277). This contrast is significant (F (1,48) = 11.27, p = 0.002, η 2 = 0.190). Also, the Electrode variable shows significant differences (F (2.16,103.43) = 4.74, p = 0.009, η 2 = 0.090). For the Electrode variable, there are significant differences in the following contrasts: The first contrast (Level 2 vs. Level 3) is the average of the PSDs generated in electrode E2 (M = 0.35) with the PSD of electrode E3 (M = 0.32). This contrast is significant (F (1,48) = 13.92, p = 0.001, η 2 = 0.225). The second contrast (Level 4 vs. Level 5) shows an average PSD from electrode E4 (M = 0.32) with the PSD of electrode E5 (M = 0.34). This contrast was also significant (F (1,48) = 8.22, p =0.006, η2 = 0.146). The third contrast (Level 5 vs. Level 6) indicates an average PSD from electrode E5 (M = 0.34) with the PSD of electrode E6 (M = 0.31).
This contrast was significant (F (1,48) = 20.15, p <0.001, η 2 = 0.296). A fourth contrast (Level 9 vs. Level 10) indicates an average PSD from electrode E9 (M = 0.35) with the PSD of electrode E10 (M = 0.32). This contrast was also significant (F (1,48) = 36.29, p < 0.001, η 2 = 0.431). The fifth contrast (Level 10 vs. Level 11) indicates an average PSD from electrode E10 (M = 0.32) with the PSD of electrode E11 (M = 0.34). This contrast shows significant differences (F (1,48) = 11.58, p = 0.001, η 2 = 0.194). Finally, a sixth contrast (Level 12 vs. Level 13) indicates an average PSD from electrode E12 (M = 0.35) with the PSD of electrode E13 (M = 0.33). This contrast indicates significant differences (F (1,48) = 9.49, p = 0.003, η2 = 0.165).
Multiple comparisons according to Bonferroni indicate statistically significant differences (p < .05) between the PSDs of the following electrodes: (a) The E2 (M = 0.35) electrode is significantly larger than E3 (M = 0.32), E4 (M = 0.32), and E6 (M = 0.31), (b) E6 (M = 0.31) electrode, is significantly lower than E5 (M = 0.34), E8 (M = 0.35), E9 (M = 0.35), and E12(M = 0.35). (c) E7 (M = 0 .32) electrode, is significantly lower than E8 (M = 0.35), E9 (M = 0.35), and E12(M = 0.35), and finally, (d) E10 (M = 0.32) electrode, is significantly lower than E8 (M = 0.35), E9 (M = 0.35), and E12(M = 0.35).
Regarding the test of inter-subject effects, the data show that there are no significant differences in silent speech using vowels /a/ - /u/, in the PSDs generated from the different electrodes due to the subjects’ cognitive styles (F (2,48) = 0.75, p = 0.477, η2 = 0.030) (Figure 7).
Discussion
The results show that PSDs in silent speech of the vowels /a/ and /u/ are not associated with a person’s cognitive style in the FDI dimension. The study’s analyses indicate that there are no significant differences between field independent, intermediate and dependent persons. Silent speech tasks using vowels /a/ - /u/, with eyes closed, likely differ from completing visual tasks and exercises related to somatosensory aspects, where FI persons were found to show increased neuronal activity for executive and inhibitory response processing (Imanaka et al., 2017; Jia et al., 2014).
The characteristics of a silent speech task with eyes closed, so as to reduce artifacts such as blinking and eye movement, ostensibly allowed field dependent persons to exhibit a greater attentional control. This preventing irrelevant or distracting aspects from arising within their perceptual field, which could alter their attention on thinking of silent speech using vowels /a/ - /u/, while completing the task. In this regard, field dependent, intermediate, and independent persons would have the same capacity for attention. Regarding field intermediate persons, it is noteworthy, that their relative functional mobility allows them to get a little closer to FI persons. However, it is not possible to assert that the former are under the same condition as the latter; but it is possible to assert that the former are closer to the level reached by the latter, as reported in other studies (Evans et al., 2013).
In this area of research, there are previous studies that addressed the classification of EEG signals through imagined speech, where a persons’ task was to imagine vocalising and pronouncing the vowels (DaSalla et al., 2009; Iqbal et al., 2015; Min et al., 2016; Nguyen et al., 2018). In these investigations, the signals were studied in the time domain, where advanced decoding algorithms are designed and validated to classify them. The present study is based on thinking of silent speech using vowels /a/ - /u/, where the object of study is the neuronal activity that takes place in the language region of the left hemisphere.
In this line of research, some studies have focused on the development of specialised algorithms for the classification of vowels (Sarmiento et al., 2014). Another study compared functional magnetic resonance imaging (fMRI) and EEG signals to identify the locations on the brain related to vowel production in silent speech (Yoshimura et al., 2016). Also, in the work by Ikeda et al. (2014), it was found that the study of Japanese vowels /a/, /i/, /u/, is related to the premotor, lower frontal gyrus, upper temporal gyrus, and motor areas. This research supplements these results insofar as the areas corresponding to electrodes 2,5,8, 9, and 12 are related to the areas found by Ikeda and collaborators.
For several years, motor imagery or movement processes have been the object of study by different researchers. In these studies, regions of the motor cortex (pre-central gyrus) and/or the somatosensory cortex (post-central gyrus) are activated, finding that motor imagination tasks are related to mu and Gamma waves (D’Zmura et al., 2009; DaSalla et al., 2009; Iqbal et al., 2015).
Within the studies of silent speech with non-invasive methods, it has been chosen to use delta, theta, alpha, beta, gamma and high gamma brain rhythms. For vowel recognition with silent speech, the following rhythms have tended to be used: theta, alpha and beta (Chi et al., 2011); delta, theta and alpha (Sarmiento et al., 2014); alpha, beta and gamma (Riaz et al., 2015); delta, theta, alpha, beta and gamma (Matsumoto & Hori, 2014) and delta, theta, alpha, beta, gamma and high gamma (Matsumoto & Hori, 2013). In the case of syllable recognition with silent speech, the following rhythms have been used: delta, theta and alpha (D’Zmura et al., 2009); theta, alpha and beta (Wang et al., 2013) and high gamma (Jahangiri & Sepulveda, 2019). In this sense, more research is needed to determine the frequency ranges for the different elements of language in speech imagery.
In contrast, this study found that the Delta and Theta waves related to IMF 4 are the most suitable rhythms for the study of silent speech using vowels /a/ - /u/ (Figure 3). These results require further study to be able to assert that these brain rhythms are the most relevant. In this vein, the development of research on the language region is a promising line of study to understand and explain EEG signals in thinking of silent speech tasks, whether using vowels, syllables, or words.
On the other hand, the study’s data show that multivariate IMFs, resulting from applying the APIT-MEMD method (Hemakom et al., 2016), evidence greater significant differences in different persons’ IMF 4, obtaining the best results in the PSD of this IMF. However, these results are not conclusive and, therefore, other studies on silent speech using different language units, such as syllables, consonants, or words, are required to corroborate that delta and theta brain rhythms are generalisable to other language units. In this regard, brain signal processing by means of the APIT-MEMD method together with the PSD, is a potential analysis alternative for identifying the signals related to thinking of silent speech.
The method used in this research considers non-linearity, non-stationarity, and existing correlations between the voluntary signals, which are more consistent with the nature of brain signals (Hemakom et al., 2016). By using these models, better results may likely be achieved when identifying and interpreting different brain processes. However, further in-depth studies are needed to contrast these results with methods based on linear and/or stationary systems, where different filters and transformations are used to identify brain rhythms (Delta, Theta, Alpha, Beta, and Gamma) while completing thinking of silent speech tasks.
Finally, when observing the different levels of PSDs from the 14 electrodes used, it is possible to identify that electrodes E2, E5, E8, E9, and E12 located in the premotor, motor, and Wernicke areas, exhibit significant differences that allow identifying vowels /a/ - /u/. Based on these results, it is possible to assert that identifying vowels with silent speech may be studied through the use of electrodes located in the premotor, motor, and Wernicke areas. These results are consistent with the spatial positions proposed in (Geschwind, 1965; Poeppel & Hickok, 2004) models. Hence, future studies on silent speech tasks should focus the spatial location of electrodes on the areas proposed above in order to enable the design of BCIs that relate specific tasks to the thinking of a specific vowel, consonant, or word.
Conclusions
It is possible to conclude that there are no significant differences in the PSDs, in the silent speech of vowels /a/ and /u/, due to the effect of the cognitive style in the FDI dimension of the participants. This result is promising in the design of BCIs, since it is an initial approach in establishing that people’s stylistic characteristics are not determining factors in the design and implementation of technological devices.
Also, the study shows that the APIT-MEMD method, together with the PSD, is a promising alternative for identifying voluntary signal processing related to the thinking of silent speech. Finally, to study silent speech using vowels /a/ - /u/, it is possible to suggest positioning the electrodes in the premotor, motor, and Wernicke’s areas.