Introduction
Knowledge-Based Typology Systems (KBTSs) have been recognized as an essential resource with competitive advantages and value creation potential (King & Zeithalm, 2003). KBTSs are a necessary ingredient for the dynamic development of competition and in general, a determining factor for companies with global ambitions (Massa & Testa, 2009). Emphasis on the descriptions of KBTSs without mentioning the producers’ knowledge state compels a narrowed vision, which could inhibit the growth and capacity of the system. Companies need to integrate business functions into a unique system to address the corporate environment and the fast development of current markets (Vandaie, 2008). These types of solutions refer to the efficient planning of corporate resources and the use of information technology to allow an internal exchange of data and information and the necessary communication with suppliers and clients (Lee et al., 2003).
Notable researchers (Belanche et al., 2020; Daxini et al., 2019; Marinus et al., 2021; Munz et al., 2020; Puente Rodríguez et al., 2019; Xue et al., 2020) have approached the relationship between co-learning processes and multi-stakeholder participatory studies to address farmer’s intentions from a typological perspective. Martínez et al. (2020), Moreno and Grajales (2017), Ospina & Grajales-Lombana (2018), and Ospina et al. (2011) and have pointed out that knowledge management around productive systems is relevant to overcome technological development constraints and should be considered in co-innovation processes. Scientific studies conclude that adaptative decision-making and knowledge transfer among stakeholders are essential to data analytics architectures dedicated to precision livestock farming and policy design for agriculture (Concu et al., 2020; Derner et al., 2021; Nolack et al., 2020).
Current studies on KBTSs are also limited in their scope. For instance, a recent study by Daxini et al. (2019) recognized that typologies examine farmers’ intentions in production system management plans. However, this study only emphasized typologies by working with the nutrition component. These related studies provide valuable insights and highlight the need to address the linkages between all components of the STBC to classify farmers according to the level of knowledge and variables that account for technological changes.
A conceptual model that contains the characterization criteria for KBTSs is proposed. This knowledge is used as a fundamental source for determining the technological development level of systems, the quality improvement of producers, rural stability, the food supply for a human population in constant growth, research necessities, and the challenges posed by climate change. The global frameworks implemented should be complemented with a new criterion: the farmers’ knowledge management state, as it sets the path for analyzing relevant processes in the systems and the decision-making process.
The goals of the current article and its case study are the following: (a) to analyze new Knowledge-Based Systems trends and applications for management support in animal production, (b) to characterize sheep and goat production systems based on the knowledge management process.
Materials and methods
The methodological approach integrated: a) the development of a conceptual model that has tacit and explicit knowledge as a central connection, b) a literature analysis of the knowledge- based system for management support in animal production and the farmers’ knowledge domain, and c) the categories of knowledge obtained by farmers according to the production objective and their technological level in the study area.
Conceptual model
The contribution of farmers’ skills and abilities and their interaction with explicit knowledge has been highlighted (Concu et al., 2020) not only in the adoption of innovations in agriculture (Hou & Hou, 2019) but also in the willingness of research and extension actors to transfer knowledge reflected in the technological development level achieved. Our research highlights the importance of recognizing the complexity of knowledge transmission and the multiplicity of explicit and tacit knowledge that inform and affect the technological development process. In this paper, the assessment of explicit and tacit knowledge of the technological development level achieved in sheep farming is based on the following hypotheses:
H1: Tacit knowledge of and information on materials and energy flow to sheep and goat systems and impact technological development and innovation.
H2: The relevance of explicit knowledge to farmers’ daily management is also expected to influence the technological development level.
The model is described in Figure 1. It shows the primary inputs, outputs, and interactions that shape technological development level in sheep and goat production systems.
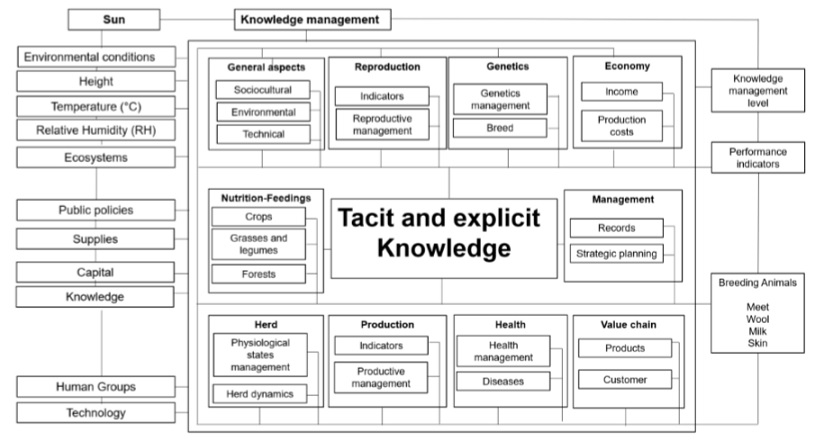
Source: Elaborated by the authors
Figure 1 Materials and farmer indicators in ovine and caprine systems in Colombia.
Figure 1 shows tacit and explicit knowledge corresponding to the central idea or meaningful connection between several elements and parts of an animal production system. The KBTS involves the connections and interdependencies between the components of sheep and goat production systems (Figure 1). Tacit and explicit knowledge influence these relationships and the level of intervention required to strengthen producers' capacities in a knowledge specific area (Daxini et al., 2019).
The KBTS involves the connections and interdependencies of each component of sheep and goat production systems (Figure 1) related to tacit and explicit knowledge and the intervention level to strengthen producers’ capacities in each area (nutrition, reproduction), not only emphasized typologies by features in a unique component (Daxini et al., 2019). Knowledge is cataloged as the primary input in the system. Its interactions through the system determine the technological development level and innovation process, which is relevant to overcome technological development constraints and implement co-innovation processes (Martínez et al., 2020; Moreno & Grajales, 2017; Ospina & Grajales-Lombana, 2018; Ospina et al., 2011).
Furthermore, the information level handled by producers, their training processes, and the knowledge of professionals who have had an impact throughout research and extension projects may be defined by assessing system inputs. The firts level formed by interactions can set producers’ knowledge level around the areas feeding the subsystems. At the third level, we analyzed how, from the producer’s applied knowledge in each thematic area, the existing systems are configured with the expression level of the producers’ knowledge (intensification). In addition, we analyzed how those skills are reflected in the indicators of production and reproductive performance, population, and knowledge management. From the location of the expression levels of knowledge, needs are clarified in terms of strengthening exchange processes, dissemination, expression, and appropriation of knowledge.
The second scenario includes the characterization and analysis of the two fields of knowledge where producers develop their production targets. These include the organization of the productive system (analysis by subsystems/characterization of explicit knowledge) and performance indicators (characterization of tacit knowledge and impact indicators). The methodological approach to the characterization process using knowledge management is shown in Figure 2.
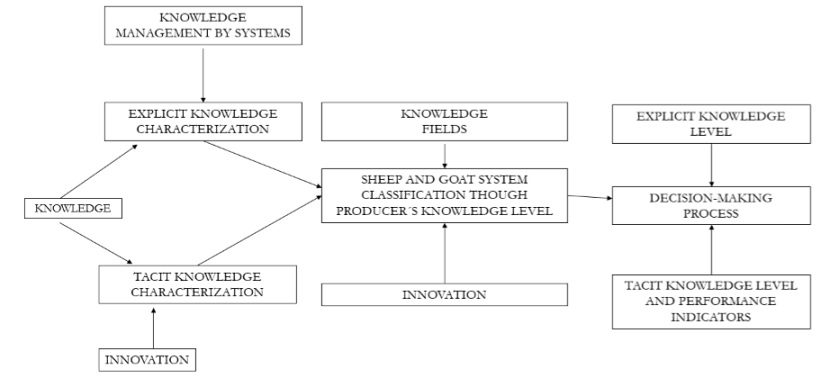
Source: Elaborated by the authors
Figure 2 Methodological approach to the classification process of producer’s knowledge management.
The methodological basis takes as its starting point the characterization of producers’s tacit and explicit knowledge, their influence on the configuration, the degree of system intensification, and how the state of knowledge affects the decision-making process.
Data collection and analysis
Literature search
Searches were carried out between 2011 and 2021 using Science Direct and Scopus as bibliographic databases. Studies selected for data extraction met all the following criteria for inclusion:
a) The study was an original research work reporting a knowledge-based system for management support to animal production.
b) Applications included learning outcomes, assessment changes in farmer choices and practices, and farmers’ knowledge domain.
Data were extracted from ten articles on Excel spreadsheets by a single reviewer, recording information on a knowledge-practice interface and the type of intervention carried out by the researchers.
Study area and statistical analysis
Information was collected from Colombia’s most important sheep and goat production areas at the farms monitored by the SIGETEC project from 2009 to 2011. Two hundred and three sheep farms (40% of the existing ones), and twenty-seven goat farms (20% of the existing ones), belonging to the Central, North, and Inter-Andean Valley regions of Colombia were selected (Figure 3). Our team interviewed farmers on knowledge fields such as general aspects of the system, nutrition and feeding, nutrition and feed management, population, production, genetics, health, economics, administration, and the market. Sheep and goat systems were stratified according to species, production objective, and geographical area. During the development of the SIGETEC research program, the information was recorded. A primary structured expert survey called SIGETEC (Microsoft Access software) was developed, which consisted of 122 questions and 48 variables.
The experts identified the five dimensions in the tests based on explicit knowledge characterization. The criteria included contrasting the highest and lowest frequencies recorded by the expert survey and the major trends within the scale of the technological changes affected by the expression level of sheep and goat producers’ knowledge. Frequencies were measured for the most informative variables using SAS V.9.1. The methodology is consistent with what was implemented by Hernández et al. (2011), Moreno and Grajales (2017), and Usai et al. (2006).
Results and discussion
The open Knowledge-Based Systems for management support to Animal Production literature provide compelling evidence of technological changes and value created from inbound knowledge flows. It creates value through innovation (Torres de Oliveira et al., 2021).
Knowledge-Based Systems for management support to animal production are abundant in the literature (Table 1). For instance, Ospina and Grajales-Lombana (2018) and Ospina et al. (2011) developed management models on tacit and explicit knowledge sources for sheep and goat producers. Xue et al. (2020) advanced a tool to measure the knowledge domain of stakeholders. The study provides a holistic knowledge map for past, current, and future stakeholder perspective studies in construction projects. Another typology is brought by Marinus et al. (2021), who created and tested an integrated co-learning approach for fostering sustainable intensification in smallholder agriculture. The complementary knowledge of farmers and researchers contributed to providing contextualized options for system intensification.
Vidmar (2021) defines knowledge as a resource to improve innovation processes among stakeholders. This knowledge must be provided systematically to be deployed in innovation processes (Vidmar, 2021). Combining these insights from the literature, we propose that KBTS can be sorted into roughly five dimensions: technical assistance, rotational grazing systems, type of mating, recording and control system implemented, and decision-making (Table 2). In this sense, the proposed typological model has caught on to the primary trends or dimensions within the scale of the technological changes affected by the expression level of sheep and goat producers’ knowledge. It included technical assistance, a rotational grazing system, controlled mating, a recording and control system implemented, and decision-making and innovation in processes.
Figure 4 shows the dimensions that allowed the identification of groups according to the production target. A total of eight groups based on production targets were identified in the study area, five from sheep systems and three from goat systems. Technical assistance has been more dynamic on the specialized systems and integrates the main knowledge areas such as animal health and production. The systems with a higher degree of specialization carry out rotational grazing or stabling with the availability of improved grass and leguminous grasses and legumes or protein and energy banks for cutting, allowing for constant supplementation. The higher the degree of specialization of production systems, the more control of breeding has been implemented by farmers in the study area. Strategic planning tools that facilitate decision-making processes and animal identification and registration systems are used less frequently in systems with a low specialization level.
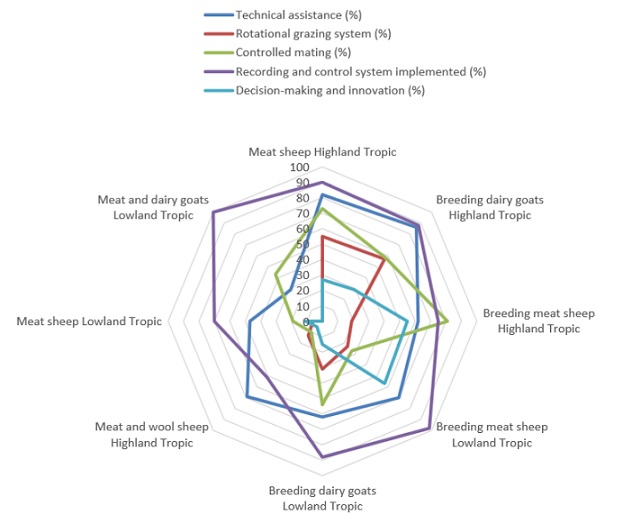
Source: Elaborated by the authors
Figure 4 Frequencies in the technological scale-up variables relevant to the study typologies.
Table 2 shows the categories of knowledge obtained by farmers according to the production objective and their technological level in the study. Three categories are identified through the study (Table 2). Class 1 was the smallest, with 18 farmers in the highland tropics with a high knowledge level and development of production targets such as breeding meat sheep and dairy goats. Class 2 corresponded to an intermediate knowledge level in highland and lowland Tropics with two production targets breeding meat sheep and dairy goats. Class 3 was the largest, with 153 highland and lowland Tropics farms with three production targets: meat and wool sheep, meat sheep, and meat and dairy goats.
Table 2 Classification of Farmers’ Knowledge Management in Sheep and Goat Production Systems according to technological level
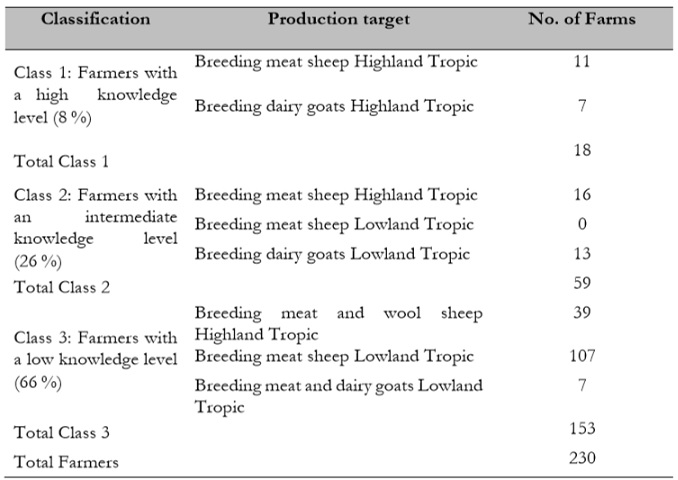
Source: Elaborated by the authors
The results from our study show that sheep and goat producers do not see knowledge as a factor of competitiveness. There is a gap in future research about analyzing training programs and their contribution to knowledge management. The emphasis of training for producers should focus on records management, implementing information systems, and analyzing performance indicators to strengthen the producers’ decision-making process.
Furthermore, the new typology (Table 2) is analytically helpful in enhancing the understanding of farmers’ knowledge systems, and it could be an operational/developmental tool for farmers, professionals, and researchers. Consequently, a detailed assessment of needs is recommended for farmers, professionals, and researchers planning or assessing any intervention.
Accordingly, the typological model suggested has been able to identify significant trends within the knowledge expression of sheep and goat producers. Based on those findings, we proposed a new typology for farmers to group by their innovations, technological level, and intervention necessities.
Conclusions
The SIGETEC methodology provides a new approach to identifying the technological development level of small ruminant systems based on the characterization of knowledge management in the components, its impact on performance indicators, and producers’ needs in terms of dissemination and implementation of knowledge in research and extension processes. The tacit knowledge and information on materials and energy flow in sheep and goat systems affect technological changes and innovation. The explicit knowledge of farmers’ day-to-day management influences the technological scale-up, mainly technical assistance, rotational grazing system, controlled mating, recording and control system implemented, and decision-making and innovation in processes. The new typology is analytically helpful in enhancing the understanding of farmers’ knowledge systems and could be an operational/developmental tool for farmers, professionals, and researchers.
Authors' contributions
Diana Cristina Moreno Vargas registration of information in the field, construction of databases, analysis of information and preparation of manuscript; Henry Alberto Grajales Lombana: preparation of project for access to economic resources, design of methodologies, recording of information in the field, supervision of activities, analysis of information and preparation of manuscript.