INTRODUCTION
The upwelling of water masses from the water table in lowland areas adjacent to the coast (Arana and Salinas, 2003), surface flows, and temporary mixtures of marine and inland water masses favor the development of coastal wetlands such as swamps, marshes, estuaries, reefs, seagrass meadows, and mangroves. These constitute a mosaic of seasonal, periodic, or continuous vegetation and water bodies (Berkowitz et al., 2018). Coastal wetlands offer recreational opportunities such as tourism (Aponte-Ubillús, 2015) and bird-watching (Woodward and Wui, 2001), in addition to providing ecosystem services such as climate regulation through carbon sequestration, erosion reduction, and the provision of habitats for hundreds of species (Engelhardt and Ritchie, 2001; Jiang et al., 2015; Gonzales et al., 2019; Pérez et al., 2022, Ochoa-Balbacea et al., 2022). Despite these benefits, these ecosystems are globally threatened by the influence of anthropic activities (Wang et al., 2021). The conversion of areas for agriculture and timber extraction are activities that alter wetlands, which is the case of mangroves in west-central Africa (Feka and Ajonina, 2011). Socioeconomic development (market integration, socio-political and economic reforms, and technological innovation) has an indirect impact as a conversion enhancer of wetland and estuaries for agricultural purposes, as has been reported in Europe in southeastern Spain (Zorrilla-Miras et al., 2014). Moreover, sewage inflow, poor environmental policies, and species introduction affect the conditions and composition of wetland populations, as is the case of San Francisco Bay in the Pacific (Cloern and Jassby, 2012). Likewise, heavy metal pollution and fires can impact the biodiversity inhabiting these environments and thus reduce the benefits they provide (Ram et al., 2018; Ramirez, et al., 2018).
Drivers of change (DCh) are defined as factors that entail direct and indirect changes in the functioning of ecosystems (Sarkar et al., 2020). Direct DCh reflect the physical and chemical impacts that immediately influence an ecosystem, such as agriculture and shepherding, while indirect ones influence direct DCh (Finlayson et al., 2005). This is the case of population growth (direct DCh) and socioeconomic reforms (indirect DCh), which put pressure on the quality of wetlands and modify the activities carried out in its surroundings (Rongoei et al., 2013; Zorrilla-Miras et al., 2014). DCh can also develop synergistically, favoring the magnification of negative effects (co-occurrence) such as agricultural activity along with population growth, economic development (Asselen et al., 2013), and the influence of superficial and groundwater drainage (McKenna et al., 2019). Such interactions have allowed studying direct and indirect DCh and the joint effect they trigger in wetlands (Zorrilla-Miras et al., 2014), which can be represented through a conceptual model.
A conceptual model (CM) is a graphical representation of the intrinsic relationships and structural elements of a specific problem, which allows identifying affinities between the objects studied (Chamanara and König-Ries, 2014). Conceptual models have been applied to elaborate dynamic tools that can strengthen wetland management using scientific evidence (Broszeit et al., 2019). Sarkar et al. (2020) developed a CM depicting the interactions between DCh and their potential consequences. Developing other CMs in wetlands has allowed representing the links between DCh regarding ecosystem processes and services (Wingard and Lorenz, 2014). On the other hand, Finlayson et al. (2005) pointed out the relationship between direct and indirect DCh, ecosystem services, and human development, also indicating that CMs are useful tools for understanding the rational use of resources and the importance of a joint administration by the authorities which ensures human well-being and the conditions of wetlands.
The Peruvian coast shows an interconnected network of coastal wetlands, along with different anthropic impacts that have to date been studied or commented in a variety of works (Aponte-Ubillús and Ramírez-Huaroto, 2011; Ruiz-Santillán et al., 2020; Mendoza-Carbajal, 2020; Rivera et al., 2022). However, the types and number of DCh are still unknown, and their interactions are not yet understood. Considering this potentiality, this study aimed at a) identifying and quantifying the wetland DCh in the Peruvian coast as reported between 2000 and 2020; b) determining the distribution of said DCh along the Peruvian coast; c) evaluating the relationship between DCh and the number of published articles, as well as between the number of articles and the number of works with reported DCh; d) evaluating the co-occurrence of DCh in the evaluated wetlands and the similarities between wetlands regarding their DCh; and e) analyzing the interactions between DCh and the main wetland ecological processes through a CM.
STUDY AREA
The north, central, and south coasts of the Peruvian littoral show a series of marine-coastal, continental, and artificial wetlands, which are part of marine, desertic, and urban spaces (Jimenez et al., 2010), located between the 3 and 18º south latitudes. The extension of mangroves (Charcape-Ravelo and Moutarde, 2005; Cabanillas et al.,2016), lagoons (Díaz et al., 2021), and swamps (Ramirez and Cano, 2010) is just an example of the types of coastal wetlands, which are characterized by the biological and phylogenetic richness they harbor (Gonzales and Aponte, 2022). The relevance of these ecosystems allows conducting an analysis of the scientific production for the 2000-2020 period, taking estuaries and mangroves with scientific reports as continental coastal wetlands, and using the list of coastal wetlands known in this region as a reference, which are reported in the Atlas of the Wetlands of the Pacific Coast (humedalescosteros.org). Figure 1 shows the coastal wetlands that meet these characteristics.
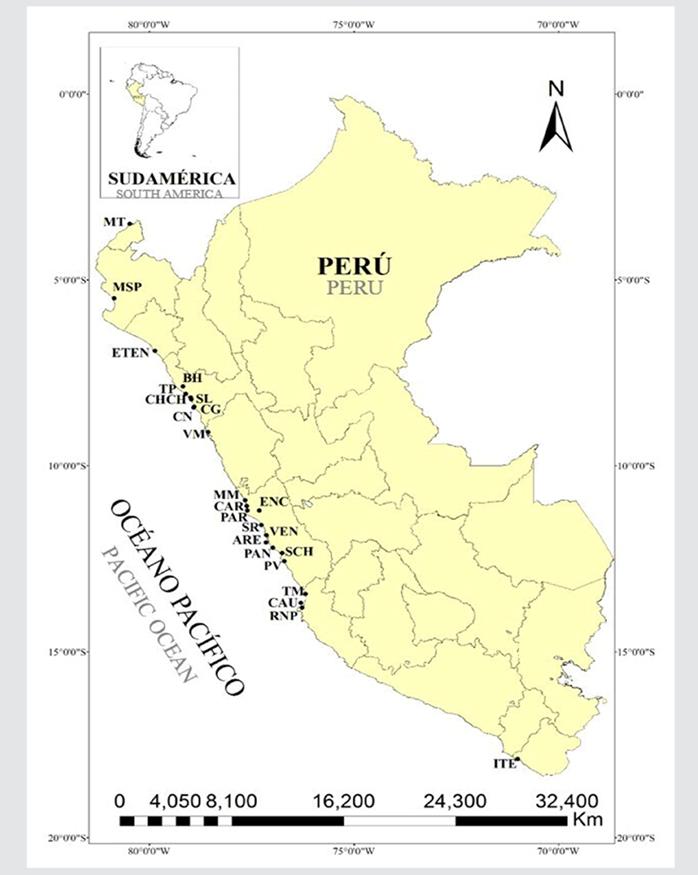
Figure 1 Wetlands of the Peruvian coast studied in this research. Mangroves of Tumbes (MT), San Pedro (MSP), Eten (ETEN) Salaverry (SL), Choc Choc (CHCH), Campo Nuevo (CN), Cerro Negro (CG), Tres Palos (TP), Balsares de Huanchaco (BH), Villa María (VM), La Encantada (ENC), Medio Mundo (MM), Carquín (CAR), Paraíso (PAR), Santa Rosa (SR), Ventanilla (VEN), Arenilla (ARE), Pantanos de Villa (PAN), Puerto Viejo (PV), Tambo Mora (TM), Caucato (CAU), Las Salinas de Chilca (SCH), Paracas National Reserve (RNP), and Wetland of Ite (ITE).
MATERIALS AND METHODS
Identifying the Drivers of Change (DCh)
The DCh were identified from a systematic review of scientific papers on Peru’s coastal wetlands published during the 2000-2020 period. The search for articles was conducted on digital platforms (i.e., ScienceDirect, Scopus, Google Académico and SciELO, using the following keywords: wetland, mangrove, lake, albufera, lagoon, estuary, and their equivalents in Spanish, in combination with the words Peru and Peruvian Coast (i.e., “manglar Perú”, “lago Perú”). All articles published in Spanish or English were considered in this study. The acknowledgment of DCh was made by reviewing the whole material of each work, as indicated in the method developed by Sarkar et al. (2020), while identifying the impacts on the systems’ ecological processes. The collected information was organized in tables (Supplementary Material 1)
Distribution of the number of DCh for the Peruvian coast
By using the collected data on the number of DCh in each wetland, a map was drawn with the distribution of these impacts on the Peruvian coast. The district containing each coastal wetland was used as the location. It should be noted that, in some cases, more than two districts were selected, as they were adjacent or shared territory with a specific wetland. The distribution map was elaborated via the Arc Gis V.10.8 software, using the natural break Jenks method (Dent et al., 2009). The information of the DCh was added as a new column to the table of attributes in the departmental and district layers. Then, the properties of symbology, quantities, color adjustment, and the scale to obtain the distribution map were modified with the number of DCh in the coastal wetlands in relation to their districts. Once the DCh were plotted, a distribution map was obtained divided into three sections: north (Tumbes Mangrove - Villa María Wetland), central (Medio Mundo - Puerto Viejo), and south (Tambo de Mora - Ite).
Correlation analysis
A paired correlation test was carried out between a) the number of DCh per wetland and the number of publications and b) the number of DCh per wetland and the number of publications with reported DCh. A Spearman correlation test was conducted since the data did not show a normal distribution (p < 0.05 in a Shapiro-Wilk test). The analyses were done in the PAST V.3.15 software (Hammer, et al., 2001), and figures were generated via Excel.
Analysis of co-occurrence of DCh and similarities between wetlands
The co-occurrence of DCh was determined to identify the most reported types of DCh in the articles. To this effect, a multivariate grouping analysis (similarity dendrogram or cluster analysis) was carried out via the UPGMA method. A matrix was prepared with the DCh influences per wetland, which were grouped using Jaccard’s index (a measurement that uses influences to group components that occur together, i.e., DCh in this case). Afterwards, the cophenetic correlation coefficient was calculated. The same procedure was followed to evaluate the similarity between wetlands according to their identified DCh. This evaluation was performed in the PAST V.3.15 software (Hammer et al., 2001).
Elaborating the conceptual model
The CM was elaborated via a modified technique previously used by Sarkar et al. (2020). Water and soil quality were considered as a single ecological process in this study. In addition, the energy flow was included, and the independent effects were evaluated for each DCh. The list of the DCh collected in each work was used, as well as the number of times each of them was mentioned next to another one. With all that, the relationships between the DCh and their effects on the most important ecological processes (EP) were established. Hydrology (H), the development of biodiversity (DB), soil and water quality (SWQ), and energy flow (EF) were obtained in order to determine the conditions of the wetland ecosystem (Sarkar et al., 2020). A matrix was elaborated which included the effects caused by DCh on ecological processes, in addition to an additional matrix with the interactions between DCh. Then, these interactions were plotted, and the corresponding frequencies of each interaction were added. The design of the CM was carried out in PowerPoint.
RESULTS
177 scientific articles were reviewed, 66 of which (37.28 %) had recorded DCh via direct observations. Based on the analysis, 22 DCh were identified (Supplementary Material 2). The most frequent (≥ 50 %) direct DCh were livestock and shepherding (58 %), agriculture (50 %), the accumulation of debris and/or garbage (50 %), and the introduction of exotic species (50 %). Governance (38 %) was the most frequent indirect DCh. The wetlands that showed the highest number of DCh (≥ 10), were Los Pantanos de Villa (15), Paraíso (12), San Pedro Mangrove (11), Villa María (10), and Ventanilla (10) (Table 1).
Table 1 List of DCh identified for the Peruvian coast wetlands. Mangroves of Tumbes (MT), San Pedro (MSP), Eten (ETEN) Salaverry (SL), Choc Choc (CHCH), Campo Nuevo (CN), Cerro Negro (CG), Tres Palos (TP), Balsares de Huanchaco (BH), Villa María (VM), La Encantada (ENC), Medio Mundo (MM), Carquín (CAR), Paraíso (PAR), Santa Rosa (SR), Ventanilla (VEN), Arenilla (ARE), Pantanos de Villa (PAN), Puerto Viejo (PV), Tambo Mora (TM), Caucato (CAU), Las Salinas de Chilca (SCH), Paracas National Reserve (RNP), and Wetland of Ite (ITE).
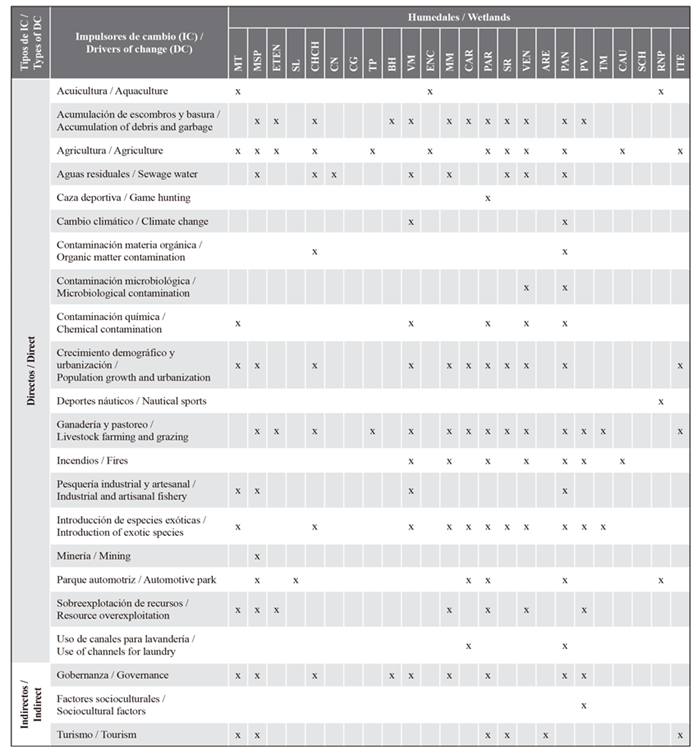
Regarding the geographic distribution of the DCh along the Peruvian coast, a high variability was observed in the north and central zones, in wetlands with large (> 10) and small (< 10) numbers of DCh, unlike the south zone, where wetlands with a small number of reported DCh were found (1-3 DCh) (Figure 2; Supplementary Material 2). A direct correlation (p < 0.05) between the number of publications and the number of DCh (rs = 0.77) was identified. Likewise, a direct correlation (p < 0.95) between the number of publications with reported DCh and the number of publications was observed (rs = 0.95) (Figure 3; Supplementary Material 3).
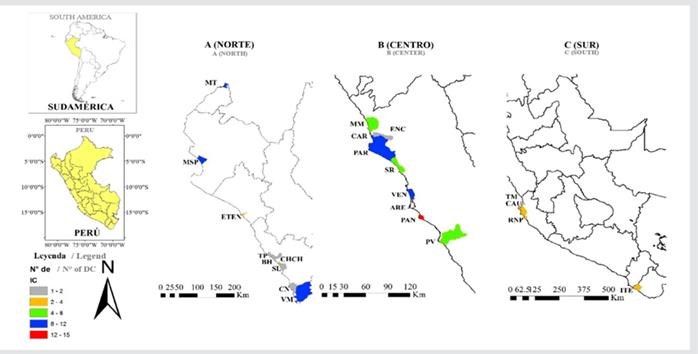
Figure 2 Distribution map of DCh for the wetlands of the Peruvian coast per district. Mangroves of Tumbes (MT), San Pedro (MSP), Eten (ETEN) Salaverry (SL), Choc Choc (CHCH), Campo Nuevo (CN), Cerro Negro (CG), Tres Palos (TP), Balsares de Huanchaco (BH), Villa María (VM), La Encantada (ENC), Medio Mundo (MM), Carquín (CAR), Paraíso (PAR), Santa Rosa (SR), Ventanilla (VEN), Arenilla (ARE), Pantanos de Villa (PAN), Puerto Viejo (PV), Tambo Mora (TM), Caucato (CAU), Las Salinas de Chilca (SCH), Paracas National Reserve (RNP), and Wetland of Ite (ITE).
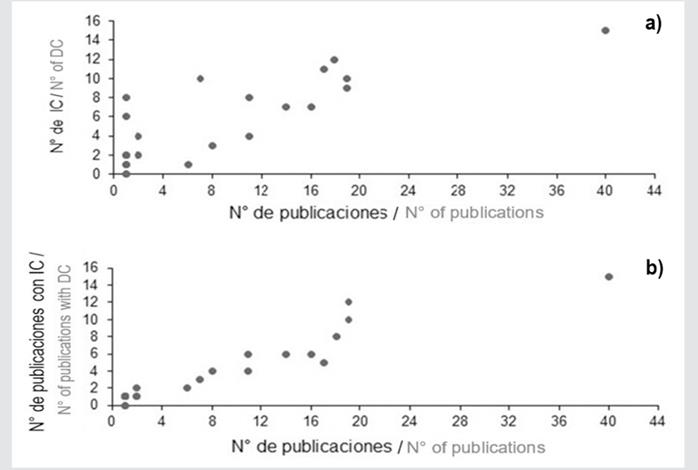
Figure 3 (a) Relationship between the number of publications and the number of DCh with a 1.38E-05 significance (p < 0.05) and a 0.77 correlation coefficient. (b) Relationship between the number of publications and the number of publications with reported DCh with a 3.93E-13 significance (p < 0.05) and a correlation coefficient of 0.95.
The DCh co-occurrence analysis evidenced two groups (similarity ≥ 50 %) represented by a) the fishing industry and chemical pollution; b) demographic growth and urbanization, the accumulation of debris or garbage, livestock and shepherding, and the introduction of exotic species (Figure 4). The graphical representation of these relationships was reliable (cophenetic correlation coefficient = 0.88).
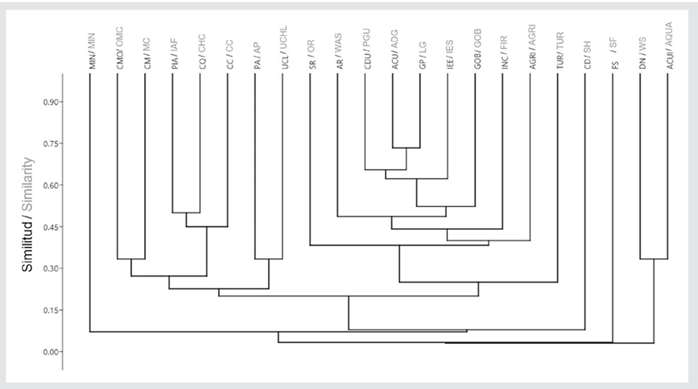
Figure 4 Similarity dendrogram for the DCh for the wetlands of the Peruvian coast. Aquaculture (ACUI), accumulation of debris and/or garbage (ACU), agriculture (AGRI), wastewater (AR), sport hunting (CD), pollution with organic material (CMO), microbiological pollution (CM), chemical pollution (CQ), demographic growth and urbanization (CDU), water sports (DN), livestock and shepherding (GP), fire (INC), industrial/artisan fishery (PIA), exotic species introduction (IEE), mining (MIN), automobile fleet (PA), overexploitation of resources (SR), use of channels for laundry (UCL), governance (GOB), climate change (CC), sociocultural factors (FS), and tourism (TUR). The coefficient of cophenetic correlation is 0.88.
Four groups of wetlands were identified, with similarities equal to or greater than 50 %. The groupings comprised a) Eten and Tres Palos, b) Choc Choc and Santa Rosa, c) Medio Mundo and Puerto Viejo, and d) Villa María, Pantanos de Villa, Paraíso, and Ventanilla (Figure 5). The graphical representation of these relationships was reliable (cophenetic correlation coefficient = 0.92).
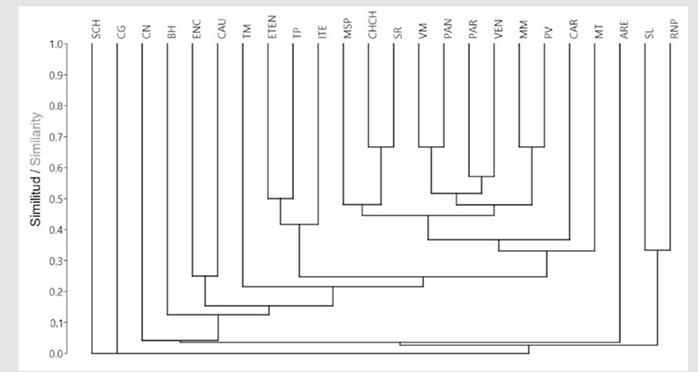
Figure 5 Similarity dendrogram for the wetlands of the Peruvian coast. Mangroves of Tumbes (MT), San Pedro (MSP), Eten (ETEN) Salaverry (SL), Choc Choc (CHCH), Campo Nuevo (CN), Cerro Negro (CG), Tres Palos (TP), Balsares de Huanchaco (BH), Villa María (VM), La Encantada (ENC), Medio Mundo (MM), Carquín (CAR), Paraíso (PAR), Santa Rosa (SR), Ventanilla (VEN), Arenilla (ARE), Pantanos de Villa (PAN), Puerto Viejo (PV), Tambo Mora (TM), Caucato (CAU), Las Salinas de Chilca (SCH), Paracas National Reserve (RNP), and Wetland of Ite (ITE). Cophenetic correlation coefficient = 0.92.
From the CM, four DCh were identified which affect the aforementioned ecological processes with a frequency higher than 10 % (i.e., demographic growth and urbanization with 23.52 %, uncontrolled tourism with 13.72 %, the automobile fleet with 13.72 %, and agriculture with 13.72 %). Four DCh with more interactions with other DCh were identified: demographic growth and urbanization, the accumulation of debris and/or garbage, fire, pollution with organic, microbiological, and chemical material, and poor wastewater disposal. It was observed that demographic growth and urbanization are the DCh most related to the introduction of exotic species and the accumulation of debris or garbage. Poor wastewater disposal entails issues associated with the pollution of water bodies. On the other hand, poor governance, the accumulation of debris and/or garbage, and demographic growth are related to fire events, while tourism and aquaculture are linked to the overexploitation of resources. Regarding ecological processes, the development of biodiversity is the most affected by DCh, while the energy flow is the least affected (Figure 6; Supplementary Material 4).
DISCUSSION
The varied distribution of DCh in the study area (with locations containing much information or no information) could indicate the unbiased effort to conduct scientific work in Peruvian coastal wetlands. This variability in scientific production could be due to three causes: (i) little interest in mentioning or reporting the effects of DCh in studies; (ii) little effort to directly study DCh, or (iii) economic aspects that do not allow studying DCh (Seifollahi-Aghmiuni et al., 2019). The results showed another possible factor: because these areas are protected, a more significant effort is required to report DCh. This, makes a tremendous difference in researchers’ interest in increasing the evidence of the impacts that deteriorate protected wetlands, as is the case of MT, MSP, PAR, VEN, and PAN, which represent more than 11 scientific articles with reported DCh in the 2000-2020 period. Thus, it is necessary to increase the efforts to study DCh in unprotected areas, where they could be occurring more frequently. It is important to consider that a systematic search for specialized literature (as the one conducted in this research) is an auxiliary tool to identify DCh; there are other direct methods or tools to estimate these impacts, such as interviews (Shalizi et al., 2020), satellite images (Lahai et al., 2022), and observational notes (Obubu et al., 2022).
Grouping wetlands with similar DCh shows the common lack of handling generated by the occurrence of some DCh (Figure 5). Thus, it can be suggested that management strategies are similar for these wetlands. Despite the different geographical locations of wetlands in the same group, it is possible to implement multiple flow policies between municipal and regional institutions which are able to identify problems, define measures, and evaluate the risk of the actions taken for the sake of better management and DCh mitigation (Floress et al.,2017). It should be noted that poor governance could indirectly affect the efforts to implement these new norms, prolonging the synergic impacts, as suggested by the CM elaborated in this study.
The analysis of the similarity between DCh allowed the identification of two groups (Figure 4). The first one comprises industrial or artisanal fishery as a driver that encompasses the marine environment and, at the same time, influences coastal ecosystems. This activity is related to the co-occurrence of chemical pollution, which could be related to a poor management of hydrocarbons in the fishing sector (Shi et al., 2022). Therefore, monitoring these impacts to counteract this DCh block is essential. The second group is composed of demographic growth and urbanization. These DCh influence other frequent impacts reported in previous works for the central coastal wetlands, such as waste disposal and species introduction (Aponte et al., 2020). The latter stresses the need for proper territorial planning. Coastal wetlands are generally surrounded by urbanization, which, in many cases, alters sedimentation and nutrient dynamics (Faulkner, 2004), which is associated with adverse effects on the hydrological regime and the deposit of chemical pollutants due to poor wastewater handling (Rodríguez et al., 2017; Camas-Guardamino and Mamani-Sinche, 2022). Considering the urban expansion surrounding the Peruvian coast’s wetlands is essential to articulating an effective management plan for these environments, as most of the DCh identified are caused by the human population. The Peruvian coast has the particularity of harboring most of the country’s population (58 % according to INEI-CENSO-2017 data), which is still growing and thus demanding more area to build housing, commercial areas, and corporate buildings. Wetlands such as Quilcay, Ventanilla, and Pantanos de Villa have these kinds of conflicts in their buffer or direct influence zones. Therefore, monitoring the urban expansion processes over time is fundamental to understanding what is happening to wetlands in this region.
Agriculture and livestock favor the reduction of coastal wetland cover, turning these areas into farming and livestock lands (Feka and Ajonina, 2011; Moreno-Casasola et al., 2014). In this sense, this reduction may also be happening in a generalized way in the wetlands of the Peruvian coast, as a loss of vegetation cover due to these DCh has been reported (Mendoza-Carbajal, 2020), which entails habitat fragmentation (Ruiz-Santillán et al., 2020). Conducting studies that directly evaluate these variables is necessary to confirm this hypothesis.
The information shown by the CM is a tool that scientists and managers can use to explain the interaction between DCh and their effects on the conditions of coastal wetlands (Euliss et al., 2004; Davis et al., 2005; Sarkar et al., 2020). Our CM allows identifying the relationships between DCh and those that deserve more attention for being the most frequent (the introduction of species, accumulation of debris, agriculture, and livestock). We believe that decision-makers must effect norms such as the Supreme Decree DS 006-2021-MINAM (which favors joint work between the Peruvian institutions in charge of wetland management) and include efficient projects aimed at mitigating the main DCh and reducing their adverse effects on the studied wetlands’ biota.
CONCLUSIONS
In the Peruvian coastal wetlands, 22 DCh were identified from the existent literature for the 2000-2020 period. Most of these drivers were found in the central and northern zones of the Peruvian coast. The number of published works allowed for the identification of DCh, in addition to the co-occurrence of drivers that generate synergic effects and wetlands with similar DCh,
The DCh with the most interactions were demographic growth and urbanization, the accumulation of debris and/or garbage, fire, pollution with organic, microbiological, and chemical material, and poor wastewater disposal. At the same time, the ecological process most affected by the DCh is the development of biodiversity.
The management of these wetlands is a joint task between decision-makers and society. This research lays the groundwork for other studies that seek to improve the schema of DCh occurring in other wetlands of Peru. It is paramount to preserve the biological diversity of these ecosystems, which, despite the reported DCh, continue to provide us with ecosystems valuable for our survival.