1. INTRODUCTION
Credit risk is defined as the classification or evaluation that is assigned to someone after having applied for a loan from a bank. The main task of Credit Risk Assessment (CAR) systems is to solve the problem of classifying customers based on their behavior or credit history [1]. Nevertheless, their results vary depending on the variables that are considered in the process, such as unpaid bills, balance in other loans, characteristics of personal accounts, monthly income, and demographic data (e.g., age and marital status) [2]. This problem has been present for a long time. However, recently, and due to the COVID-19 pandemic, it has become worse because the number of loan applications has increased significantly, and many borrowers had a poor credit history or requested amounts higher than they could pay back. As a result, their default risk assessments were deficient, and a massive number of loan defaulters resulted in financial losses for banking institutions. In spite of the gradual return to normality, the number of defaulters keeps rising. Therefore, different and more efficient solutions should be developed to minimize these losses [3].
This is a brief description of the problem in CRA. Hence, new and affordable technologies should be adopted in CRA to support the loan granting decisions of banking institutions [4]. This is where CRA systems emerge as a viable alternative to improve credit assessment processes for loan granting. Different systems of this kind have been increasingly used, applying many current technologies. Several banks are developing their own systems based on their own selection criteria to reduce the probability of losses in loans requested by customers or firms [5]. Some of the most widely used CRA systems are based on Artificial Intelligence (AI) and Machine Learning (ML) techniques that analyze and pinpoint trends in potential debtors [6]. These solutions have proven to be efficient, but their performance depends on different factors, such as required data and standards. Many banks, especially microfinance institutions, do not have access to these data, and the latter do not include demographic characteristics, which would improve the system performance. In addition, currently, there are no standards in place to define this type of data treatment as a “solution.” Nevertheless, these solutions aim to improve the process of CRA and thus reduce financial losses for financial institutions [7].
CRA can use technology-based methods, models, and algorithms to make predictions and select borrowers [8]. In this type of assessment, banks create computer models to represent the process of selecting customers for loan granting so that the approach is clearer and produces better results [9]. The operation of these tools is based on the application of several statistical models depending on the complexity of the assessment.
Among the statistical models most commonly used for data treatment in CRA systems, the most popular is the Synthetic Minority Over-sampling Technique Evaluation (SMOTE) [10]-[12]. The SMOTE applies algorithms and mathematical processes to artificially generate synthetic samples, that is, artificial samples are created based on the characteristics of original ones in the classes under evaluation. Therefore, it can generate more general and balanced samples to be treated [13]. Besides this model, the efficiency of each kind of statistical model depends on the approach and the type of model adopted by the authors, who define their own efficiency measurements to evaluate the aspects that they deem convenient [14]. In addition, CRA can change depending on the financial institution and the financial model they use. This is because the set of variables that institutions use to measure risk can vary depending on the approach they need [15].
In CRA systems, AI techniques have been especially used to process and classify data-substituting human analysis to improve the accuracy and speed of the assessment. Based on predictions, these systems classify the risk level of a loan by evaluating different variables: economic, social, demographic, financial factors, etc. [16].
CRA systems appeared at the beginning of the 2000s, with the development of new technologies and financial institutions’ need for information systems that could conduct CRA. The first CRA systems implemented algorithms and statistical techniques based on financial models to determine potential debtors [17]. Later, ML-based models appeared and evolved into AI models that use big data techniques to process big databases, which is necessary to improve the results of the assessment [18].
CRA systems also involve the use of different financial models, which should be compared and validated with suitable theoretical foundations to provide reliable solutions. In addition, these systems include statistical models to treat vast amounts of data, which is necessary to continuously improve them [19].
In these systems, the literature recommends the implementation of ML techniques, especially algorithms based on Random Forest, thanks to their accuracy considering several factors. It also recommends AI techniques. Among them, the most popular are solutions based on Neural Networks, which combine statistical algorithms and algorithmic processes. The literature also suggests to take into consideration the hardware that is used for CRA, which should be adequate to run the algorithms [20].
CRA systems need models that determine the variables to be assessed. However, only a few studies analyzed in this literature review describe the logic behind their solutions for CRA. In addition, there is little information on the limitations of CRA systems.
This Systematic Literature Review (SLR) proposes four research questions, which will be detailed later. In particular, this SLR aims to determine what methods, models, and algorithms have been employed in CRA systems; their individual efficiency; the logic behind their assessment models; and their limitations.
This paper describes and analyzes the most efficient solutions used in CRA systems, as well as their limitations and problems.
This SLR followed the PRISMA statement, which offers guidelines to orderly and systematically review published documents about a research topic [21].
As a result, this SLR found 41 common solutions in CRA systems. Ten of them are techniques based on financial models. This review also determined three limitations or problems that could arise when CRA systems are implemented-one of them is particularly significant and should be considered after the implementation process.
This paper is structured as follows. Section 1 introduces the study and presents the state of the art. Section 2 details the methodology of this SLR and reports the results of the statistical analysis. Section 3 provides answers to the research questions. Finally, Section 4 draws the conclusions of this work.
2. METHODOLOGY
This SLR implemented the PRISMA statement to investigate the available knowledge and studies about the topic addressed here. This statement provides researchers with a framework to adequately carry out SLRs that are accepted by the entire scientific community. It also details the necessary steps for an SLR, including establishing goals, eligibility criteria, results, and conclusions [21]. The protocol of this SLR followed the 17 steps in the PRISMA statement.
In addition, the research objectives of this SLR were clearly established in order to cover and obtain all the necessary information. Table 1 details the four research objectives and four research questions in this SLR based on the PICO (Problem, Intervention, Comparison, and Outcome) model provided by the PRISMA statement. The PICO model is used to formulate research questions [21]. The first research objective in this SLR is quantitative because it aims to identify and count the total number of methods, models, or algorithms employed in CRA systems. The other three objectives are qualitative because they intend to determine the most efficient models, methods, and algorithms, as well as the problems or limitations that may arise during the implementation of CRA systems. The four questions were formulated to achieve the four research objectives, respectively.
Subsequently, a search string was defined considering three groups of terms that were identified. The first group contained terms related to the implementation of CRA systems, i.e., methods, models, algorithms, and technologies. The second group included terms related to the topic of this review, i.e., credit risk or credit risk assessment. The last group referred to solutions: system, implementation, and software. Considering these three groups of terms, the following search string was formulated: (methods OR models OR algorithms OR technologies) AND (“credit risk” OR “credit risk assessment”) AND (system OR implementation OR software).
In addition, six inclusion criteria were established. First, this SLR included only documents in English as it is the most widely accepted language in this scientific area. Second, to study recent literature, the articles should have been published between 2018 and 2022. Third, to ensure the legitimacy of the material, the articles should be final published versions. Fourth, they should be indexed in research databases. Fifth, the articles should be about Credit Risk Assessment (CRA) systems. Sixth, articles about the implementation of said systems were also included. Table 2 details these criteria.
After the search string and the inclusion and exclusion criteria were defined, the search was conducted in three databases: Scopus, IEEExplore, and ResearchGate. These three databases were selected because we had access to the articles indexed in them. The search was performed on September 13, 2022, and only articles indexed in those databases were selected.
A preliminary search found 2,285 articles in these three databases. Among them, 88 were excluded because they were duplicates. Then, 1,860 records were excluded as they did not meet the pre-established inclusion criteria. At that point, there were 337 articles: 82 in Scopus, 117 in IEEExplore, and 38 in ResearchGate. Afterward, the titles and abstracts of the documents were analyzed to determine if they latter were about topics closely related to the implementation of CRA systems. After this process, there were 104 articles: 71 in Scopus, 17 in IEEExplore, and 16 in ResearchGate. Subsequently, the full text of these articles was examined to evaluate their connection to the research topic addressed of this study. Thus, other 35 records were excluded, for 69 articles in the final sample. This process is described in detail in Figure 1.
In this methodology, due to the criteria established and the limited access to articles behind paywalls, the results could present some bias. Because of funding reasons, only articles we had access to were reviewed. In addition, time and language limitations influenced the course of this study.
The list of all the articles that were selected for this review was used to generate a series of figures to illustrate the characteristics of these documents.
Figure 2 details the number of documents published every year in the selected period. The year 2021 presented the highest number of publications (26), followed by 2022 (15). This figure shows the publication trend in this field during that period.
It also includes the distribution of those articles into the quartiles of the journals where they were published. The website Scimago was used to classify the journals into quartiles (from Q1 to Q4). The results show that 33 of the articles were published in Q1 journals; 19, in Q2; 13, in Q3; and 4, in Q4. This chart provides information about the quality and impact of the sources where the articles were published.
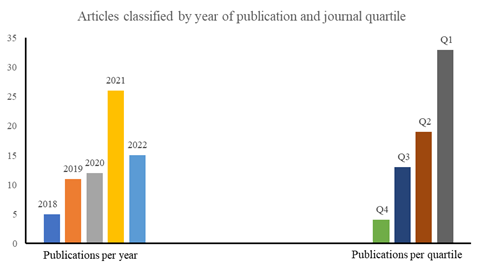
Source: Own work.
Figure 2 Number of articles published every year in the selected period and classified by quartile of their journal
In Figure 3, the articles are classified based on the nationality of their main author. Most of them are from China (36), followed by India (5), the US (4), and the UK (4).
Table 3 lists the top 15 articles in number of citations, showing the most influential papers in this research field.
In turn, Figure 4 shows the countries where the articles included in this SLR were published. Again, most of them were published in China (33), followed by India (5).
Table 4 details all the keywords retrieved from the selected documents. They were grouped into three themes: technologies, supply chain, and financial processes. The first theme includes 32 keywords related to technologies that are employed to implement CRA systems. The second one features three terms associated with the supply chain. The third theme has 22 keywords related to financial processes in CRA.
Following the PRISMA statement, the next step was to extract key information from the selected documents. This included methods, results obtained, and any other variables that might have been relevant for the objectives of this review. The data was extracted systematically and following a pre-established protocol.
Subsequently, the results of this data extraction were summarized to identify patterns and trends and draw conclusions about the reviewed literature. This enabled us to address the research objectives and answer the research questions.
Simultaneously, the quality of the studies was assessed. The PRISMA statement encourages researchers to conduct a critical assessment of the methodological quality of the selected studies. This includes factors such as study design, sample size, validity of the results, and presence of possible bias. The quality of the selected studies was taken into account in the interpretation of the results. When the selected studies presented comparable and sufficiently homogenous results, a meta-analysis was conducted to combine the data and obtain global estimations of the effects. This contributed to a more accurate assessment of the available information. Finally, the heterogeneity and bias in the included studies were continuously evaluated. Thanks to this continuous evaluation, it was possible to identify possible sources of heterogeneity and bias as the review progressed, which contributed to a more accurate interpretation of the results.
3. RESULTS AND DISCUSSION
3.1 Research question 1: What solutions are used in CRA systems?
In different studies, diverse computational solutions have been implemented in CRA systems. These solutions can be classified into three types: methods, models, and algorithms. Authors in this field adopt a particular solution in accordance with the topic they are investigating or their purpose. Table 5 details the solutions identified in the reviewed studies (31 references).
3.2 Research question 2: What are the most efficient solutions to implement a CRA system?
The efficiency of the solutions found in the selected articles was evaluated based on the accuracy percentage of the systems developed and described by the authors. There are three efficiency levels: inefficient, efficient, and very efficient. Table 6 details the solutions and their efficiency levels.
3.3 Research question 3: What credit risk models are used by banking institutions?
This review also identified financial models that banking institutions use for CRA. A credit assessment model can assign weights to qualitative and quantitative variables to evaluate customer credit quality [54]. These models enable banking institutions to measure (based on their own variables and specifications) the risk level that represents granting a loan to a borrower. Table 7 details the credit risk models identified in this review.
A total of 10 models were found in the selected documents. They define the necessary variables for CRA and are adapted according to specific requirements. Many of these models include the demographic characteristics of the applicants to improve the accuracy of the assessment.
3.4. Research question 4: What problems or limitations may arise in the implementation of CRA systems?
Finally, in the selected articles, this review found three limitations or problems that may arise in the implementation of CRA systems. A limitation or problem is a factor that can negatively influence research, creating obstacles for authors. Table 8 details the problems and limitations found in the selected research papers. It is concluded that, nevertheless, these limitations or problems do not significantly affect the implementation of CRA systems.
Three limitations were found in this SLR. First, the fact that logistic regression is the standard approach in the sector (due to explainability regulations) means that there should be a balance between innovation and transparency in credit decision-making. Second, the “No Free Lunch” theorem indicates that algorithms should be adapted to variations in credit data, acknowledging that there is no “one-size-fits-all” approach. Third, default prediction performance in imbalanced classification situations shows that we should not only focus on absolute high accuracy, but on achieving good global performance and generalization ability.
The limitations identified here do not significantly hinder the implementation of CRA systems. However, these challenges should be strategically addressed to further improve the quality and efficiency of CRA in a constantly evolving environment.
This review found CRA solutions in 31 of the selected documents. AI-based algorithms and techniques are present in most of the implementations reported in the articles. Another widely used solution is Neural Networks. Many authors claim that the latter can process data efficiently, be adapted, and combined with other algorithms to improve their performance. Recently, decision trees have become more popular due to their predictive power based on features. Although these solutions have produced good results in implementations of CRA systems, many of them have been validated and tested using simulated databases based on information collected by several banks around the world. These simulated databases can be used to demonstrate the functionality of the systems, but their results do not represent the reality of some organizations.
Among the 31 articles that described solutions for CRA, 16 of them (i.e., 52 % in this sample) reported accuracies above 90 %. Therefore, their solutions were classified as “Very efficient.” In particular, AI techniques showed great efficiency. Although these studies stress the high performance of their systems, some of them may be biased as they reported 100 % accuracies, which is very unlikely.
CRA systems need to have models that determine the variables to be assessed. However, only a few studies analyzed in this review describe the logic behind their solutions for CRA.
Finally, only three articles in this review described the limitations or problems that should be considered in the implementation of their CRA solutions.
4. CONCLUSIONS
Currently, CRA systems are developed all over the world, mainly in China, India, and the US. These countries are particularly interested in constantly innovating in the implementation of CRA systems.
The methods, models, and algorithms reviewed here make a great contribution to these systems. However, their functionality should be analyzed using real (not simulated) databases to ensure that the results represent the reality of many organizations. Among these methods, models, and algorithms, AI was employed in most studies. In particular. Neural Networks and Random Forest were present in most articles. They have been combined with different algorithms to guarantee specific results according to the implementation.
All the solutions analyzed here have shown outstanding efficiency, with their own particularities. Neural Networks-in particular, deep and convolutional Neural Networks-have demonstrated high efficiency due to their ability to predict outcomes based on feature recognition. Their accuracy in the implemented systems is above average. These networks can identify and thoroughly analyze features and patterns in data, which results in accurate, reliable predictions. Likewise, Random Forest-based algorithms exhibited an outstanding predictive power in CRA. These algorithms exploit the diversity and predictive power of a collection of individual trees to offer reliable and consistent predictions, which results in more robust and generalizable models. Nevertheless, this can mean a possible deficiency in cases of large volumes of data or variables, which can increase the computational complexity and require significant processing and storage resources. Although these technologies presented remarkable efficiency, most of the other solutions achieved satisfactory results as well. Still, as some studies referred to margins of error, the methods they employed should be continuously improved and refined.
To develop CRA systems, it is important to describe the logic behind the variables that are involved in the implementation. This logic is not described in many of the articles reviewed here. Therefore, future studies should focus on this specific area to further improve CRA systems. Also, they could examine aspects such as the optimization of AI algorithms more thoroughly to achieve ever greater accuracy in CRA. They should use real data (instead of simulations) to identify and address possible challenges and limitations in the practical implementation of CRA systems. Furthermore, they can include demographic factors in CRA, which is a key opportunity. They can explore how to effectively incorporate these factors and how to adapt models to different populations. Finally, they should investigate the impact that current and future research in this field can have on the financial sector and decision-making regarding credit risk. These studies should benefit financial institutions and consumers in general.