INTRODUCTION
The International Telecommunications Union (ITU) defines cognitive radio (CR) as a "radio or system that detects and is aware of its surroundings and can be adjusted dynamically and autonomously according to its radio operation parameters" (Hernández, Pedraza, Páez, & Rodriguez-Colina, 2015). The solution in cognitive radio against the inefficient use of the radioelectric spectrum is none other than the dynamic access to the spectrum (DSA), allowing the opportunistic use of the available frequencies by secondary users (SU) without causing any interference to the primary users (PU) (Hernández, Pedraza, et al., 2015; R. López & Montejo Sánchez, 2015). This is materialized through the process known as a cognitive cycle (Játiva, 2017; Romero, 2015).
The spectral decision function plays a very important role for the optimal performance of the cognitive radio network (CRN) within the cognitive cycle (Lala, Balkhi, & Mir, 2017) since the proper move at a timely instant within the radioelectric spectrum guarantees that the PUs do not suffer from any interference during the use of licensed channels, and that the SUs have uninterrupted communications without delays that cause information loss. Hence, most of the research work has focused on improving and establishing more assertive strategies for optimal development (Das, Ghosh, Das, & Barman, 2017; Hoque, Azmal, & Arif, 2017; Kyryk & Yanyshyn, 2017).
To achieve this there is a need for artificial intelligence algorithms and techniques (Bayrakdar & Çalhan, 2018), as well as machine learning (A. M., Matyjas, Hu, & Kumar, 2017; Koushik, Hu, & Kumar, 2018), and multi-criteria decisionmaking methods (Hernández, Giral, & Santa, 2015; Salgado, Hernandez, Molina, & Beltran-Molina, 2016), among others. Some examples include particle swarm optimization (Agrawal, Tyagi, & Singh, 2018; Alhammadi, Roslee, & Alias, 2017) , real time spectrum transfer (Chakraborty & Misra, 2015), and fuzzy logic (Javed & Naeem, 2018) . When it is uncertain which frequency of the spectrum is available during the transmission time required by the SU, it is important to consider the behavior pattern of PUs. Most of the algorithms used in CR manage to model and even predict the behavior of spectrum usage by Pus based on past spectral information such as spectral occupancy (Harold Vásquez Suarez, 2017; Hernández & Luis Fernando Pedraza Martínez, 2016; D. A. López, Trujillo, & Gualdron, 2015).
To model or predict with enough accuracy it is necessary to use a large amount of historical spectral information. However, as large amounts of past information can have a positive effect on prediction and modeling of the spectral occupancy pattern, they can also have a negative impact in the processing times of the algorithms, as well as significant delays during communication. Therefore, it is necessary to determine the lowest amount of spectral information needed to generate forecasts with accuracy and low delays. The present research aims to determine an optimal number of past data needed to carry out a proper modeling process of the behavior of spectral occupancy in a given frequency band as well as the maximum time in which the parameters of the algorithms must be adjusted, so as to reduce resource consumption such as the processing time, energy, hardware and software requirements, among others.
This work was carried out based on a series of spectral mobility simulations in the GSM frequency band with the CRN simulation software developed by (Hernández & Giral, 2015). Criteria Time and Time Range parameters were varied in this software which have maximum times of 1800 and 10800 units respectively (180 units correspond to 1 minute), while the other parameters remain constant.
RELATED WORK
One of the main problems faced by cognitive radio networks is the high consumption of energy and the stronger impact seen in the battery-supplied networks. Therefore, (Abdullah & Abed, 2016) carried out simulations to reduce the number of samples used in the PU detection stage. Reductions by 50%, 33%, and 25% are achieved in order to determine energy savings. This led to discover that the reduction in energy consumption when Eb/No is 10 dB is 50%, 60% and 63%, respectively, for the sample reductions mentioned above. However, not all detections were convenient, so the authors concluded that a reduction of up to 40% in energy consumption can be reached as long as half of the samples or more is used with an acceptable error margin in the detection of the primary user.
In (Syed & Safdar, 2017), the efficiency and demand in energy of the CR system are improved using the historical records for spectrum detection, which is none other than data acquired over time. This reduces the spectrum exploration frequency. In this assisted scheme, the database of the centralized analytical engine offers a wide historical record of the spectrum usage so it can be harnessed by SUs, which acquire said data and process it in order to ensure an effective detection, reduction in energy consumption by close to 50%.
In (Marino, Paura & Savoia, 2016), in order to optimize energy consumption in the spectrum detection process, a theoretical analysis is carried out on an arbitrary mobility network model to show that there is a unique detection time in which performance is increased for the detection of SU mobility. Afterwards, several simulations are performed to prove the existence of said value.
METHODOLOGY
The simulation software for CRN used in this research was developed in the project "Development of an adaptive algorithm for spectral handoff focused on increasing the performance and efficiency of mobile networks," funded by the Center for Research and Scientific Development from the District University Francisco José de Caldas (Hernández & Giral, 2015). That software allows to parameterize different variables such as:
Type of technology: Wi-Fi or GSM.
Simulation time: SU transmission, between 1 and 10 minutes.
Handoff models: AHP RT, AHP BE, FAHP RT, FAHP BE, FFAHP RT, FFAHP BE, SAW RT, SAW BE, MEW RT, MEW BE, TOPSIS RT, TOPSIS BE, VIKOR RT, VIKOR BE, GRA, or RANDOM, among others.
Traffic level: high, medium, or low traffic.
Threshold: Power threshold that can determine the presence or absence of a PU in a determined channel.
Noise Floor: Average noise floor of the spectrum analyzer during the metering campaign.
BW Fixed: Fixed bandwidth for each channel.
Criteria time: Periodic time in which the values of the decision criteria are recalculated and updated during the execution of the algorithm. It can take a maximum value of 1800 time instants (10 minutes).
Time Range: Time in which the initial values of the decision criteria are calculated, before the assessment. It can take a maximum value of 10,800 (60 minutes).
Multichannel: Number of continuous channels that the algorithm can use to perform multi-channel transmission.
Type of modulation: 16QAM, 64QAM, QPSK y
BFSK.
Weights: In this section, the weights must be defined for the four decision criteria: Available Average (AA), Bandwidth (BW), Signal-to-Noise Ratio plus interference (SINR), and Estimated Availability Time (EAT).
The software works under three traffic levels: High, Medium, and Low, both for the training phase (used to set the initial parameters of the algorithms) and the assessment phase (used to assess the performance of the algorithms). This distribution corresponds to six databases for each type of technology (GSM and Wi-Fi), thus to 12 databases (see Table 1).
Table 1 Selected data
Tecnology | Traffic | Traffic level | Time lapses | Rows | Columns |
---|---|---|---|---|---|
GSM | Evaluation | High | 1800 (10 min) | 1800 | 551 |
Low | |||||
Medium | |||||
Training | High | 10800 (60 min) | 10800 | 551 | |
Low | |||||
Medium | |||||
Wi-Fi | Evaluation | High | 1800 (10 min) | 1800 | 461 |
Bajo | |||||
Medium | |||||
Training | High | 10800 (60 min) | 10800 | 461 | |
Low | |||||
Medium |
Source: Taken from (Hernández & Giral, 2015).
The simulation software uses real spectral occupancy data gathered during a previous metering campaign in Bogota city, over a one-week period. Tables 2 and 3 show the amount of data gathered for each technology (Hernández & Giral, 2015). The column represents the frequency channels, and the rows represent time (1/3 of a second approximately for each row).
Table 2 Acquired data
Tecnology | Quantity of Acquired Data | ||
---|---|---|---|
Rows | Columns | Total Data | |
GSM | 1.145.700 | 551 | 631.280.700 |
Wi-Fi | 2.490.000 | 461 | 1.147.890.000 |
Source: Taken from (Hernández & Giral, 2015).
Table 3 Data quantity for training and evaluation
Tecnology | Process | Data |
---|---|---|
GSM | Evaluación | 991.800 |
Entrenamiento | 5.950.800 | |
Wi-Fi | Evaluación | 829.800 |
Entrenamiento | 4.978.800 |
Source: Taken from (Hernández & Giral, 2015).
The software also allows to load some data by default. The data loaded for this research included the weight of Available Average (AA), Bandwidth Average (BW), PSINR, Estimated Availability Time (EAT), and the parameters Threshold, Noise Floor, and Fixed BW. The system was parameterized with 4 channels in the criteria (Multichannel) and the GSM technology.
Simulations were carried out for the decision-making algorithm Fuzzy Feedback Analytic Hierarchy Process (FFAHP) due to its notable performance in CRN (Hernandez-Suarez, Pedraza-Martinez, & de la Colina, 2016). Four different types of scenarios were considered: (1) Real-time approach with high traffic (RT-HT); (2) Real-time approach with low traffic (RT-LT); (3) Better effort with high traffic (BE-HT); and (4) Better effort with low traffic (BE-LT). The parameters were modified in each scenario while the criteria time remained constant. The opposite case was also assessed.
Figure 1 shows the parameterization of the previously described variables in the graphical software interface for FFAHP with RT-HT.
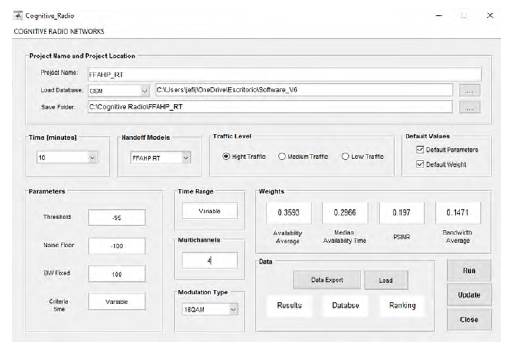
Source: MATLAB main software screen
Figure 1 Parameters of the graphical interface of the software for FFAHP for RT-HT scenario.
Simulations
To determine the minimum amount of information needed, three sets of simulations were developed. Initially, the parameter "Criteria_Time" kept a constant value of 1800 (maximum value), and the parameter "Time_Range" was modified for all types of scenarios RT-HT, RT-LT, BE-HT and BE-LT. Table 4 shows the variation ranges of each parameter.
Table 4 Time_Range Variation for FFAHP
FFAHP RT-HT, RT-LT, BE-HT, BE-LT | Criteria Time | Time Range |
1800 | 180 | |
360 | ||
540 | ||
720 | ||
900 | ||
1080 | ||
1260 | ||
1440 | ||
1620 | ||
1800 | ||
3600 | ||
5400 | ||
7200 | ||
9000 | ||
10800 |
Source: Authors
A similar procedure was also carried out in which the parameter "Time_Range" had a constant value of 10.800 (maximum value), while the parameter "Criteria_Time" was set in each of four scenarios RT-HT, RT-LT, BE-HT and BE-LT. Table 5 shows the variation ranges for each parameter.
Table 5 Criteria_Time Variation for FFAHP
FFAHP RT-HT, RT-LT, BE-RT, BE-LT | Criteria Time | Time Range |
180 | 10800 | |
360 | ||
540 | ||
720 | ||
900 | ||
1080 | ||
1260 | ||
1440 | ||
1620 | ||
1800 |
Source: Authors
Finally, joint simulations were carried out using the best results from the previous experiments in order to determine the optimal behavior. For each experiment, 10 simulations were performed and the average values were stored in order to deliver more significant data in terms of statistics.
The present research was based mainly on the previously mentioned metering campaigns, that lead to the charts corresponding to the spectral handoff with real spectral occupancy databases, with a SU transmission time of 10 minutes.
RESULTS
The results are organized into three sections: (1) Time_Range, (2) Criteria_Time, and (3) Joint Results. In the first section, figures 2 to 5 show the simulation results when the "Time_Range" remained constant with a value of 10800 and the "Criteria_ Time" varied. In the second section, figures 6 to 9 describe the simulation results when the "Criteria_Time" remained constant with a value of 1800 and the "Time_Range" varied. In both sections, the figures describe the results obtained in each simulation scenario described in the methodology: RT-HT, RT-LT, BE-HT, and BE-LT. In the third section, figures 6 to 9 show the information corresponding to the third simulation campaign, in which the parameter values corresponding to the best behaviors during initial simulations were considered.
Figures 2 to 5 lead to some conclusions. Firstly, when the update time of the decision parameters is increased, the variations in the handoff rate for low traffic scenarios (RT-LT and BE-LT) are more significant than in their high traffic counterparts (RT-HT and BE-HT). Secondly, in most cases the Criteria Time variable delivers similar results; thus, it is not necessary to update the decision-making parameters so frequently, even when an inflection point is detected in the charts. Hence, three of the highest values of the Criteria Time were chosen (900, 1260, and 1800) to perform a joint analysis in the third subsection of the results section to determine with more objectivity the best value of the Criteria Time. However, when the Criteria Time equals 720 (4 minutes), there is an abrupt negative change in 3 out of 4 scenarios (RT-LT, BE-HT, and BE-LT) followed by a stabilization when it reaches the value of 900 (5 minutes). As a result, the value of 720 is classified as an atypical value.
As a preliminary statement, it could be assumed that it is unnecessary to update (or recalculate) the decision parameters in such short periods, rendering the Criteria Time equal to 1800 (10 minutes), which is the best option among the analyzed values, yet establishing that the best option may be a superior value.
Figures 6 to 9 enable different analyses. Firstly, in a similar case to Criteria Time when the number of previous samples is reduced, the variations in the handoff rate for low traffic scenarios (RT-LT and BE-LT) are more significant than in high traffic scenarios (RT-HT and BE-HT). There is an exception in high traffic where the Time Range value is 360 (2 minutes) and leads to atypical results in the spectral handoff rate, so the value is not considered for the corresponding values. Secondly, in most cases, the Time Range offers similar results, showing that it is not necessary to set the initial decision-making parameters with an excessive amount of past data. In contrast to Criteria Time, some inflection points were determined for each scenario. In the RT-HT case with 720 samples (4 minutes) and onward, the results are fairly similar. However, when the number of past samples is below 540 (3 minutes), the performance of the handoff rate is reduced by 25.87%. In the BE-HT case, the inflection point is reached at 360 (2 minutes). Similar results occur when the samples surpass a total of 540 (3 minutes) and when there are less than 360 samples (2 minutes): the performance of the handoff rate is reduced by 14.28%. In the case of RT-LT, the inflection point stands at 3600 (20 minutes) and for 5400 samples (30 minutes), and onward, where the results are fairly similar. When there are less than 3600 samples (20 minutes), the performance of the spectral handoff rate is reduced by 27.77%. The BE-LT case has the same behavior than BE-HT.
For high traffic (HT), it is concluded that close to 720 samples (4 minutes) are needed. This is significantly lower than the 5400 samples (30 minutes) required for low traffic and could be explained by the fact that high traffic translates into a more dynamic and frequent behavior, making it easier to model a behavior pattern in terms of spectral occupancy. It is important to point out that the previous explanation makes sense for spectral occupancy of the present work within the GSM band.
Given that the purpose is to determine the minimum value of required past samples, and based on the previous analysis, the Time Range values of 720, 1260, 1800, 3600, and 5400 were chosen to perform a joint analysis.
Joint results
Since the values of Criteria Time and Time Range that offered the best independent results were determined in previous analyses, the combinations of said values are assessed to determine the couple that offers the best results. The values of Criteria Time are: 720, 1260, 1800, 3600, and 5400; and for Time Range the values are: 900, 1260, and 1800. The analysis is based on the previously described scenarios RT-HT, RT-LT, BE-HT, and BE-LT, whose results can be seen in Tables 6, 7, 8, and 9, respectively.
Table 6 Handoffs for joint values of Criteria Time and Time Range, for RT-HT
Time Range | ||||||
---|---|---|---|---|---|---|
720 | 1260 | 1800 | 3600 | 5400 | ||
Criteria Time | 900 | 228 | 228 | 224 | 227 | 227 |
1260 | 227 | 229 | 225 | 230 | 230 | |
1800 | 228 | 228 | 224 | 231 | 231 |
Sources: Authors
Table 7 Handoffs for joint values of Criteria Time and Time Range, for RT-LT
Time Range | ||||||
---|---|---|---|---|---|---|
720 | 1260 | 1800 | 3600 | 5400 | ||
Criteria Time | 900 | 179 | 178 | 179 | 169 | 144 |
1260 | 177 | 172 | 165 | 186 | 144 | |
1800 | 178 | 172 | 174 | 184 | 144 |
Sources: Authors
Tabla 8 Handoffs for joint values of Criteria Time and Time Range, for BE-HT
Time Range | ||||||
---|---|---|---|---|---|---|
720 | 1260 | 1800 | 3600 | 5400 | ||
Criteria Time | 900 | 291 | 279 | 272 | 279 | 279 |
1260 | 279 | 277 | 279 | 297 | 296 | |
1800 | 288 | 291 | 270 | 291 | 290 |
Sources: Authors
Tabla 9 Handoffs for joint values of Criteria Time and Time Range, for BE-LT
Time Range | ||||||
---|---|---|---|---|---|---|
720 | 1260 | 1800 | 3600 | 5400 | ||
Criteria Time | 900 | 174 | 174 | 175 | 167 | 144 |
1260 | 174 | 173 | 166 | 186 | 144 | |
1800 | 174 | 174 | 175 | 185 | 145 |
Sources: Authors
The analysis in Tables 6 to 9 shows that the best handoff rates (the smallest ones) take place for the following combinations, respectively: for RT-HT, 1800 and 1800; for RT-LT, 1800 and 5400; for BE-HT; 1800 and 5400; and for BE-LT, 1800 and 5400.
In conclusion, it is sufficient to take 1800 samples in high traffic to calculate the initial value of the parameters and update them every 10 minutes (1800). In low traffic, it is sufficient to take 5400 samples to calculate the parameters and update them every 10 minutes. This translates into a reduction of energy consumption and computational effort for network and users by 10 times for Criteria Time and by 6 times for Time Range; this means that the computational cost was approximately reduced by 60 times.
CONCLUSIONS
Based on the previous results, it is not mandatory to have a high number of previous samples to determine the initial value of the decision parameters and deliver a good performance in handoff rates, nor is it necessary to update the values for traffic under the GSM frequency band. This allowed to significantly cut energy consumption as well as costs and delays in the information processing stages, thereby optimizing the resources of the cognitive radio network.