1. Introduction
The diagnosis of an ophthalmologic disease is done through different kinds of clinical exams. Exams may be non-invasive such as slit-lamp exam, visual acuity, eye fundus image (EFI), ultrasound, optical coherence tomography (OCT); or invasive exams as fluorescein angiography [1]. Non-invasive clinical exams are easier to take, have no contraindications and do not affect the eye's natural response to external factors in comparison to invasive exams. Therefore, EFI and OCT exams are high-patient compliant, quick and simple techniques, with the main advantages that images can be easily saved to be analyzed at a later time, and the prognosis, diagnosis and follow-up of diseases can be monitored over time.
Automatic analysis of EFIs and OCTs as a tool to support medical diagnosis has become an engineering challenge in terms of achieving the best performance, the lowest computational cost and lowest runtime among the different algorithms [2-6]. Thus, the choice of the best method to represent, analyze and make a diagnosis using ocular images is a complex computational problem [7-11]. On the other hand, deep learning techniques have been applied with some success to several eye conditions using as evidence individual sources of information [12-14].
Some researchers have studied how to support the diagnosis with different methodologies. Vandarkuhali and Ravichandran [2] detected the retinal blood vessels with an extreme learning machine approach and probabilistic neural networks, Gurudath et al. [12] worked with machine learning identification from fundus images with a three-layered artificial neural network and a support vector machine to classify retinal images, and Priyadarshini et al. studied clustering and classifications with data mining to give some useful prediction applied to diabetic retinopathy diagnosis [3]. Despite good results, the main problem with these works is that datasets are small and the need for labels is expensive and cumbersome work.
Deep learning (DL) offers some advantages such as the processing of lots of images with the use of graphic processing units (GPU) and tensor processing units (TPU); and the ability to automatically learn data representation from raw data. Tanks to these features, DL has been able to outperform traditional methods in several computer vision and image analysis tasks. Tis success has motivated its application to medical image analysis including, of course, ophthalmology images.
Tis article focuses on the review and analysis of deep learning methods applied to ocular images for the diagnosis of diabetic retinopathy (DR), glaucoma, diabetic macular edema (DME) and age-related macular degeneration (AMD). Tese diseases are related with diabetes as one of the four major types of chronic noncommunicable disease and they are the leading cause of blindness worldwide in productive age (20-69 years), with the main problem that 25 % of diabetics worldwide will have visual problems along diabetes, and without a preventive diagnosis and treatment promptly, these subjects will suffer irreversible blindness [15-20].
Te paper is organized as follows: Section 2 contains an overview of ocular diseases medical background with their corresponding information sources. Section 3 summarizes free public-available ocular datasets. Section 4 summarizes the most common performance metrics used by deep learning methods. In addition, Section 5 reports on the main deep learning methods for each source of medical information. Finally, Section 6 discusses the main results, limitations, and future works.
2. Medical background
2.1 Ocular diseases
2.1.1 Diabetic retinopathy
Diabetic retinopathy is caused by a diabetes side-effect which reduces blood supply to the retina, including lesions appearing on the retinal surface [21]. DR-related lesions can be categorized into i) red lesions such as microaneurysms and hemorrhages, and ii) bright lesions such as exudates and cotton-wool spots [22], as shown in Figure 1.
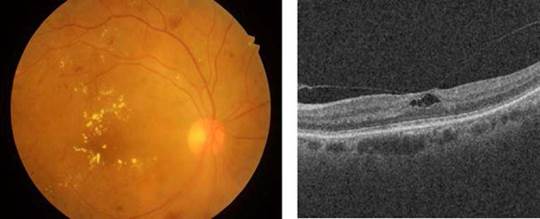
Source: Taken from [24].
Fig. 1 [Left] A color eye fundus image showing multiple microaneurysms, intraretinal hemorrhages, and exudation affecting the fovea in a patient with severe non-proliferative diabetic retinopathy with severe diabetic macular edema, and [Right] A b-scan OCT showing vitreomacular traction affecting the foveal depression.
2.1.2 Diabetic macular edema
The diabetes macular edema is a complication of DR that occurs when the vessels of the central part of the retina (macula) are affected by the accumulation of fluid and exudate formation in different parts of the eye [25], as depicted in Figure 2.
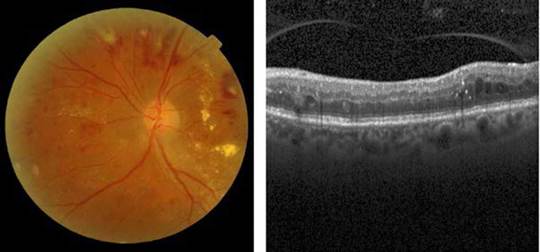
Source: Taken from [26] and [27].
Fig. 2 [Left] A color eye fundus image showing multiple dot and flame hemorrhages, cotton wool spots and macular exudation in a patient with severe non-proliferative diabetic retinopathy with diabetic macular edema, and [Right] A b-scan OCT showing multiple intraretinal hyperreflective dots and pseudo-cystic spaces in the middle retinal layers in a patient with diabetic macular edema.
2.1.3 Glaucoma
The glaucoma is related to the progressive degeneration of optic nerve fibers and structural changes of the optic nerve head [21]. Although glaucoma cannot be cured, its progression can be slowed down through treatment. Therefore, the timely diagnosis of this disease is vital to avoid blindness [28-29]. Glaucoma diagnosis detection is based on manual assessment of the Optic Disc (OD) through ophthalmoscopy, looking morphological parameters for the central bright zone called the optic cup and a peripheral region called the neuro-retinal rim [30], as reported in Figure 3.
2.1.4 Age-related macular degeneration
The age-related macular degeneration (AMD) causes vision loss at the central region and distortion at the peripheral region [21]. The main symptom and clinical indicator of dry AMD are drusen. The major symptom of wet AMD is the presence of exudates [33], as presented in Figure 4.
2.2 Medical information sources
There are different types of clinical exams for the diagnosis of ocular disease. Some researchers documented eye digital signal and image processing techniques such as electrooculogram (EOC) [36], electroretinogram (ERG) [37-38], visual evoked potentials [39-42], dynamic pupillometry [43-44], among other methods [45].
The two non-invasive techniques widely used by the ophthalmologist to diagnose the ocular condition are EFIs and OCT. On the one hand, the eye fundus is represented as a 2D image of the eye that allows checking faster and easily parts of the eyes (i.e. optic disc, blood vessels, and others), but also some retinal abnormalities (i.e. microaneurysms, exudates, among others). On the other hand, the OCT uses near-infrared light based on low coherence interferometry principles to record the set of retinal layers. The OCT depicts the information in a 3D volume with a resolution of a cross-sectional area with a defined number of scans as shown in Figure 5. In the two cases, the diagnosis performed by experts depends crucially on the clinical findings located during the exam.
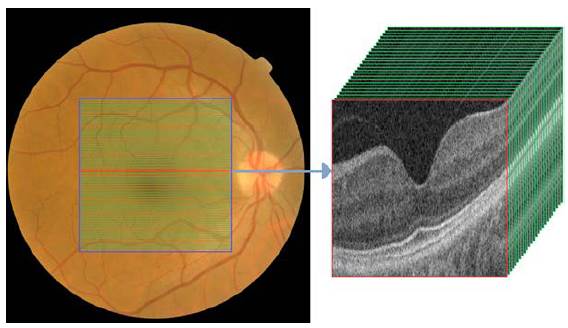
Source: Taken from [46].
Fig. 5 EFI and OCT volume containing cross-sectional b-scans from a healthy subject.
3. Ocular image datasets
In recent years, the detection of clinical signs and the grading of ocular diseases have been considered engineering challenging tasks. In addition, worldwide researchers have published their methods and a set of EFIs and OCTs databases with different ocular conditions, population, acquisition devices and image resolution. The available ocular datasets for each ocular disease, the type of ocular image and the study population are presented in Table 1.
Table 1 A summary of free public ocular datasets with ocular diseases graded by experts, dataset names and da-taset descriptions.
Ocular disease | Dataset | Dataset description |
---|---|---|
DR | [47] | 40 eye fundus images with a resolution of images are 768 x 584 pixels. The dataset contains 7 images graded by experts as mild DR and 33 images as normal. |
[48] | 130 eye fundus images with 110 DR and 20 normal images. The images labeled as DR contain the segmentation of clinical signs: hard exudates, soft exudates, microaneurysms, hemorrhages, and neovascularization. | |
[49] | 89 eye fundus images where 84 images have mil DR and 5 images labeled as normal. | |
[50] | 100 digital color fundus images with microaneurysms in all the images. This dataset was randomly split into training and test datasets with 50 images. | |
[51] | 28 eye fundus images with two blood vessel segments performed by experts. | |
[52] | Two subsets: a set of 47 eye fundus images with the segmentation of exudates and 35 images without lesions labeled as normal. The second set has 148 images with microaneurysms and 233 images labeled as normal. | |
[53] | Two subsets: the training set has 35126 and the test set has 53576. The images were labeled as normal, mild, moderate, severe and proliferative DR. | |
[54] | 13000 images with normal, mild, moderate, severe and proliferative DR. | |
DR, Glaucoma | [55] | 49 eye fundus images with the optic head segmentation and the grading of DR and glaucoma. |
[56] | 45 eye fundus images with 15 healthy, 15 DR and 15 glaucomatous subjects. The images have the detection and segmentation of clinical signs provided by experts. | |
DR, DME | [26] | 1200 eye fundus images with DR and DME labels performed by an expert. |
[23] | 516 images with a resolution of 4288x2848 pixels with the grading of DME and DR performed by experts. | |
DR, AMD | [57-58] | 400 eye fundus images and 400 black and white masks with blood vessel annotations. |
[59-60] | 143 color fundus images with a resolution of 768x576 pixels. The images were grading as 23 AMD, 59 DR, and 61 normal images. | |
[61] | 500 OCTs with normal, macula hole, AMD, central serous retinopathy and DR. | |
Glaucoma | [62] | 110 color fundus images with optic nerve head segmentation. The images were labeled as 26 glaucomatous and 84 with eye hypertension. |
[63] | 650 eye fundus images with the classification of glaucoma condition. | |
[64] | 40 color images with the blood vessels, optic disc, and arterio-venous reference. | |
[31] | 783 images with glaucomatous, suspicious of glaucoma and normal conditions. | |
[65] | 258 eye fundus images with 144 normal and 114 glaucomatous subjects. | |
[66-67] | 101 images with optic disc and optic cup segmentation and glaucoma condition. | |
[68] | 760 retinal fundus images with glaucoma labels. | |
[69] | 1200 eye fundus images with optic disc and cup segmentation with normal and glaucoma conditions. | |
[32] | 1110 scans where 263 were diagnosed as healthy and 847 with primary open-angle glaucoma (POAG). | |
AMD | [70] | 206500 eye fundus images with AMD and non-AMD conditions. |
[34] | 1200 eye fundus images with early AMD and non-AMD conditions. | |
[35] | 385 OCTs with 269 AMD and 115 normal subjects. Each OCT volume has 100 B-scan with a resolution of 512x1000 pixels. | |
[71] | 15 OCT volumes with the retinal layer segmentation performed by an expert. The database was labeled with AMD condition. | |
DME | [72] | 169 eye fundus images with mild, moderate and severe DME. |
DME, AMD | [27] | 45 OCTs with 15 AMD, 15 DME, and 15 normal subjects. Each OCT volume has 100 B-scan with a resolution of 512x1000 pixels. |
[73] | 148 OCTs as follows: 50 DME, 50 normal and 48 AMD subjects. | |
[74] | 109309 scans of subjects with DME, drusen, choroidal neovascularization and normal conditions. | |
DME, AMD, DR | [24] | 75 OCTs labeled as 16 normal, 20 DME and 39 DR-DME. The OCT volume contains 128 B-scans with a resolution of 512x1024 pixels. |
DR, AMD, Glaucoma | [46] | 231806 OCTs and eye fundus images with the labels of glaucoma, DR and AMD. |
Source: Compiled by the authors.
4. Performance methods
Deep learning approaches have shown astonishing results in problem domains like recognition system, natural language processing, medical sciences, and in many other fields. Google, Facebook, Twitter, Instagram, and other big companies use deep learning in order to provide better pplications and services to their customers [75]. Deep learning approaches have active applicatio s using Deep Convolutional Neural Networks (DCNN) in object recognition [76-79], speech recognition [80-82], natural language processing [83], theoretical science [84], medical science [85-86], etc. In the medical field, some researchers apply deep learning to solve different medical problems like diabetic retinopathy [86], detection of cancer cells in the human body [87], spine imaging [88] and many others [89-90]. Although unsupervised learning is applicable in the field of medical science where sufficient labeled datasets for a particular type of the disease are not available. In particular, the state-of-the-art methods in ocular images are based on supervised learning techniques.
4.1 Performance metrics in deep learning models
The performance comparison of deep learning methods in classification tasks is performed by the calculation of statistical metrics. These metrics assess the agreement and disagreement between the expert and the proposed method to grade an ocular disease [35,62,74]. The performance metrics used in state-of-the-art works are presented in Equations (1 - 7) as follows:
where,
TP = True positive (the ground-truth and predicted are non-control class)
TN = True Negative (the ground-truth and predicted are control class)
FP = False Positive (predicted as a non-control class but the ground-truth is control class)
FN = False Negative (predicted as control class but the ground-truth is non-control class)
po = Probability of agreement or correct classification among raters.
pe = Probability of chance agreement among raters.
5. Deep learning methods for diagnosis support
5.1 Dl methods using eye fundus images
The state-of-the-art DL methods to classify ocular diseases using EFIs are focused on conventional or vanilla CNN and multi-stage CNN. The most common vanilla CNN used with EFIs are the pre-trained inception-V1 and V3 models on the ImageNet database (http://www.image-net.org/). The inception-V1 is a CNN that contains different sizes of convolutions for the same input to be stacked as a unique output. Another difference with normal CNN is that the inclusion of convolutional layers with kernel size of 1x1 at the middle and global average pooling at the final of its architecture [79]. On the other hand, inception-V3 is an improved version batch normalization and label smoothing strategies to prevent overfitting [91].
[94] used the U-Net model proposed by [92] to segment the retinal vessel from EFIs. Then, two new datasets were created with and without the vessels to be used as inputs in the inception-V1. This method obtained an AUC of 0.9772 in the detection of DR in the DRIU dataset. [96] and [98] proposed a patch-based model composed of pre-trained inception-V3 to detect DR in the EyePAC dataset. [98] used a private dataset with segmentations of clinical signs to classify an EFI into normal or referable DR with a sensitivity of 93.4 % and specificity of 93.9 %. The ensembled of four inception-V3 CNN by [96] reached an accuracy of 88.72 %, a precision of 95.77 % and a recall of 94.84 %.
The multistage CNN is centered first on the detection of clinical signs to sequentially grade the ocular disease. [95] located different types of lesions to integrate an imbalanced weighting map to focus the model attention in the local signs to classify DR obtaining an AUC of 0.9590. [97] used a similar approach to generate heat maps with the detected lesion as an attention model to grade in an image-level the DR with an AUC of 0.954. [99] uses a four-layers CNN as a patches-based model to segment exudates and the generated exudate mask was used to diagnose DME reporting an accuracy of 82.5 % and a Kappa coefficient of 0.6. Then, [104] proposes a three-stage DL model: optic and cup segmentation, morphometric features estimation and glaucoma grading, with an accuracy of 89.4 %, a sensitivity of 89.5 % and a specificity of 88.9 %. Finally, [101] proposed a model to segment optic disc and cup and calculate a normalized cup-discratio to discriminate healthy and glaucomatous optic nerve of EFIs. Table 2 presents a brief summary of DL methods in eye fundus images used to support an ocular diagnosis.
Table 2 An overview of the main state-of-the-art DL methods to ocular diagnosis using EFIs. Dataset and method used in the study are included with method performance.
Ocular disease | Dataset used | Authors | Methods | Performance |
---|---|---|---|---|
DR | DRIVE | [93] | Gaussian Mixture Model with an ensemble classifier | AUC: 0.94 |
[94] | Pre-trained Inception V1 | AUC: 0.9772 | ||
EyePACS | [95] | DCNN with two stages | AUC: 0.9590. | |
[81] | [96] | An ensemble of 4 pre-trained Inception V3 | Acc.: 88.72 %; Precision: 95.77 %; Recall: 94.84 % | |
EyePACS & E-OPHTHA | [97] | Two linked DCNN | AUC: 0.954 and AUC: 0.949 respectively. | |
DR | EYEPACS & MESSIDOR & Private dataset | [98] | A pre-trained Inception V3 | Sensitivity: 93.4 %; Specificity: 93.9 %. |
DME | MESSIDOR & OPHTHA | [99-100] | DCNN with two stages | Acc.: 82.5 % Kappa: 0.6 |
Glaucoma | DRISHTI-GS & REFUGE | [101] | DCNN with two stages | AUC: 0.8583 |
DRISHTI-GS & RIM-ONE | [102] | Classical filters and an active disc formulation with a local energy function | Acc.: 0.8380 and 0.8456. | |
[102-103] | DCNN with three stages | Accuracy: 89.4 %; Sensitivity: 89.5 %; Specificity: 88.9 %; Kappa: 0.82 | ||
AMD | AREDS | [104] | DCNN | Acc.: 75.7 % |
Source: Compiled by the authors.
5.2. Dl methods using optical coherence tomography
The most representative DL methods to detect abnormalities in OCT obtained an outstanding performance using vanilla CNN models as reported with ResNet [35,106], VGG-16 [111] and Inception-V3 [110]. VGG-16 CNN contains five blocks of convolutional layers and max-pooling to perform feature extraction [78]. The final block is composed of three fully connected layers to discriminate among a number of classes. The ResNet model contains a chain of interlaced layers that adds the information from previous layers to future layers to learn residuals errors [112].
[106] used a pre-trained ResNet to differentiate healthy OCT volumes from DR with an accuracy of 97.55 %, a precision of 94.49 %, and a recall of 94.33 %. [24] combined the Inception and the ResNet model into a model termed as inception-ResNet-V2. This model was able to classify DME scans with an accuracy of 100 % using the SERI dataset.
On the other hand, the best DCNN model using OCT volumes as input are customized models with two or three stages. In particular, these DL models used two or more datasets reported in Table 1 to perform feature extraction of local signs, added to a classification stage for grading the ocular diseases as reported for OCTs in [105-107].
[110] defined a two-stage DL method to segment abnormalities from the OCT volume into a 3D representation. The generated segmentation was stacked with the 43 most representative cross-sectional scans from an OCT volume. This model obtained an AUC of 0.9921 to determine the grade of AMD in private datasets. Finally, [109] proposed a customized DL method called OctNet. This CNN is based in four blocks of convolutional and max-pooling layers, and a final block with two dense layers and a dropout layer to avoid overfitting during training. In addition, the proposed model classifies in scan and volume levels, delivering highlighted images with the most relevant areas for the model. The model was assessed for DR and DME detection with a precision of 93 %, an AUC of 0.86 and a Kappa agreement coefficient of 0.71. The proposed model presented a sensitivity of 99 % and an AUC of 0.99 for the classification task of OCT volumes as healthy and AMD. Table 3 reports an overview of the most prominent works used to support the diagnosis of ocular conditions using OCTs.
Table 3 An overview of the main state-of-the-art DL methods to ocular diagnosis using OCTs. Datasets and the methods used in the study with methods performance.
Ocular disease | Dataset | Authors | Methods | Performance |
---|---|---|---|---|
DR | OCTID | [105] | Pre-trained ResNet model | Accuracy: 97.55; Precision: 94.49; Recall: 94.33. |
DME | SERI | [35] | Pretrained Inception-ResNet-V2 | Accuracy: 100 % |
SERI+CUHK | [106-107] | OCTNET with 16 layers, class activation maps and medical feedback | Precision: 93.0 %; Kappa: 0.71; AUC: 0.86 | |
Glaucoma | POAG | [62] | A 3D-DCNN with 6 layers | AUC: 0.89 |
AMD | A2A SD-OCT | [108] | HOG Feature Extraction and PCA, with SVM and Multi-Instance SVM classifiers | Accuracy: 94.4 %, Sensitivity: 96.8 % Specificity: 92.1 % |
[108-109] | OCTNET with 16 layers, class activation maps and medical feedback | Sensitivity: 99 %; AUC: 0.99 | ||
Private dataset | [110] | DCNN with two stages by Google | AUC: 0.9921 | |
[111] | Pretrained VGG-16 model | AUC: 0.9382 | ||
[74] | Pretrained Inception-V3 model | AUC:0.9745; Accuracy: 93.45 %. |
Source: Compiled by the authors.
6. Discussion
This review reports the deep learning state-off-the-art works applied to EFIs and OCT for ocular diagnosis as presented in Tables 2 and 3. The main DL methods in the detection of ocular diseases using EFIs are focused on the fine-tuning of pre-trained CNNs such as Inception V1 [94] and Inception V3 [96]. In addition, the pre-trained CNNs applied to OCT obtained an outstanding performance as reported with pre-trained ResNet [35,105], VGG-16 [111] and Inception V3 [74]. Thus, the feature extraction stage performed by CNNs using a non-medical domain dataset from ImageNet is enough to discriminate healthy and unhealthy patterns from ocular images. On the other hand, the best CNN models using OCT volumes as input are customized models with two or three stages. In particular, these DL models used two or more ocular medical datasets reported in Table 1 to perform the feature extraction of local signs, added to a classification stage for grading the ocular diseases as reported for EFIs in [95,97,99-103] and for OCTs in [106-109].
The number of free public available datasets contributes to the design of new DL methodologies to classify ocular conditions as reported in Table 1. However, the use of a private dataset limits the comparison among performance metrics reached by DL methods [74,98,110-111]. The replication of studies reported by [98] and [110] have been criticized for the lack of information related to the description of the method and the hyperparameters used [113]. The use of public repositories as GitHub (https://github.com/) to share datasets and codes is still a need.
Nowadays, the growing interest of big technology companies and medical centers to create open challenges has increased the number of ocular datasets such as the DR detection by Kaggle [53,84], the blindness detection by the Asia Pacific Tele-Ophthalmology Society (APTOS) [54] and iChallenge for AMD detection by Baidu [34]. These new datasets contain diverse information related to acquisition devices, image resolution, and worldwide population. Moreover, DL techniques are leveraging the new data to the design of new robust approaches with outstanding performances as reported in Tables 2 and 3.
The lack of validation of DCNN models with real-world scans or fundus images is still a problem. We found a couple of methods validated with ocular images from medical centers [96, 108-111]. However, the number of free public real-world ocular images is limited to five sets of images [31,46,53-54,74]. The clinical acceptance of the proposed DCNN models depends critically on the validation in clinical and nonclinical datasets.
Conclusions
Deep Learning methods are novel techniques that detect and classify different abnormalities in eye images with great potential to effectively ocular disease diagnosis. These methods take advantage of a large number of available datasets with different annotations of clinical signs and ocular diseases to perform the automatic feature extraction that supports medical decision making.
In the medical context, new devices such as Optical Coherence Tomography-Angiography (OCTA) require new models to represent and extract features that support the prognosis, diagnosis and follow-up of ocular diseases. Hence, the design of deep learning methods that use multi-modal information such as clinical reports, physiological data and other medical images is still an important issue. The validation of DL methods in the clinical environment with real-world data-sets and images acquired using low-cost devices could improve the social impact of the methods developed.
Despite the outstanding results, there are some open challenges with these methods related to the interpretability and the feedback of medical personnel to the models. In addition, the application of DL models in medical centers could potentially increase the number of subjects diagnosed with the consequent improvement in the quality of life of the population. Realizing the potential of these techniques requires a coordinate, interdisciplinary effort of engineers and ophthalmologists focused on the patient to optimize the medical diagnosis time and costs.