Services on Demand
Journal
Article
Indicators
-
Cited by SciELO
-
Access statistics
Related links
-
Cited by Google
-
Similars in SciELO
-
Similars in Google
Share
Revista Facultad Nacional de Agronomía Medellín
Print version ISSN 0304-2847
Rev. Fac. Nac. Agron. Medellín vol.66 no.1 Medellín Jan./June 2013
Prediction Models for Total Milk Yield and Fat Percentage Using Partial Samples
Modelos de Predicción para Producción de Leche Total y Porcentaje de Grasa a Partir de Muestreos Parciales
Juan Diego Rodríguez Neira1; Guillermo Antonio Correa Londoño2 and José Julián Echeverri Zuluaga3
1 Zootechnician. Universidad Nacional de Colombia - Sede Medellín - Facultad de Ciencias Agrarias - Grupo BIOGEM. A.A. 1779, Medellín, Colombia. <yojuandiego@gmail.com>
2 Associate Professor. Universidad Nacional de Colombia - Sede Medellín - Facultad de Ciencias Agrarias - Departamento de Ciencias Agronómicas. A.A. 1779, Medellín, Colombia. <gcorrea@unal.edu.co>
3 Assistant Professor. Universidad Nacional de Colombia - Sede Medellín - Facultad de Ciencias Agrarias - Departamento de Producción Animal. A.A. 1779, Medellín Colombia. <jjecheve@unal.edu.com.co>
Received: February 28, 2012; Accepted: September 18, 2012.
Abstract
This research aimed to present prediction models for daily total milk yield and percentage of total fat from the partial productions in the morning (a.m.) and the afternoon (p.m.). This research was carried out with three dairy herds in Antioquia. 182 Holstein dairy cows milked twice a day were sampled monthly over a year. Recorded data included: information on entering time, milk yield, fat percentage, parity, days in milk, calving season and milking intervals. Predictive models were created from multiple regression analysis. The effects of duration of days in milk, milking interval, birth number, fat percentage and the quadratic terms of the previously mentioned effects were significant for the percentage of fat per day and the total milk production per day. Fat percentage and total milk production are affected by different environmental factors. In order to have more accurate estimates of production, it is necessary to consider adjustment factors for the known effects.
Key words: Environmental effects, mathematical models, adjustment factors, genetic improvement.
Resumen
En esta investigación se pretenden plantear modelos de predicción para la producción de leche total y el porcentaje de grasa total día, a partir de las producciones parciales de la mañana (a.m.) y de la tarde (p.m.) La investigación se llevó a cabo en tres hatos lecheros del departamento de Antioquia. Se realizó muestreo de leche mensual a 182 vacas Holstein en dos ordeños durante un año. Se capturó información de hora de entrada al ordeño, producción de leche, porcentaje de grasa, número de partos, días en lactancia, época de parto e intervalo entre ordeños. Los modelos de predicción fueron realizados a partir de un análisis de regresión múltiple. Los efectos de días en lactancia, intervalo entre ordeños, número de partos, porcentaje de grasa y la distribución cuadrática de los efectos mencionados anteriormente fueron significativos sobre el porcentaje de grasa día y la producción total día. El porcentaje de grasa y la producción total de leche están afectados por diferentes factores ambientales y para tener estimativas más precisas de producción es necesario considerar factores de ajuste sobre los efectos conocidos.
Palabras clave: Efectos ambientales, modelos matemáticos, factores de ajuste, mejoramiento genético.
Milk production in Colombia has focused on increasing milk volume, neglecting the study of other important traits (Rodríguez et al., 2009). The new requirements of the market have led to increased development, searching for high standards of quality through breeding strategies (Quijano and Echeverri, 2007).
Studying milk and its components is essential for management and genetic improvement in dairy cattle. Use of genetic parameters such as heritability, repeatability and breeding values are of great importance in selection, because they ensure some progress per generation (Rodriguez et al., 2009).
Milk yield and fat percentage are affected by various environmental effects such as parity, duration of lactation, lactation stage (Stoop et al., 2009), length of pregnancy (Karijord et al., 1982; Syrstad et al., 1982; Palmquist et al., 1993) and others (Kay et al., 2005; Garnsworthy et al., 2006). According to Loker et al. (2009), milk fat decreases with the advancement of the pregnancy stage.
These characteristics, as well as all quantitative features, have an environmental component and a genetic one. Being able to isolate them is the key to the estimation of breeding values of animals.
Estimation of breeding values is essential to making decisions regarding the selection of superior animals with the estimated breeding value (EBV) (VanRaden et al., 2008). The problem is that environmental effects that may mask the true genetic value of individuals should be controlled in order to obtain an unbiased EBV.
Setting environmental factors causes a reduction in environmental variance, which allows for reliably comparing individuals under different environmental conditions and selecting the best (Searle, 1962; Miller, 1973).
Under the conditions of Colombian livestock, where there are no milk production control programs, being able to predict daily milk yield and fat percentage from only one milking sample is necessary for the establishment of any breeding program.
This work aimed to develop predictive models for daily total milk production and the percentage of total fat from partial samples of milk in the morning (a.m.) or afternoon (p.m.). In addition, other environmental effects were analyzed that may affect these productive parameters, generating adjusted expressions, simple and easy to apply for use in genetic evaluations and animal breeding programs.
Materials and methods
Description and location of the research area. The research was conducted on three specialized dairy farms that stand out for their high productive and genetic levels. The nutrition management conditions in the selected herds were very similar, taking care that the herd effect did not impact the fit of the models. The farms are located in the municipalities of Santa Elena and San Pedro, in the department of Antioquia, Colombia. According to Espinal (1985) and IAVH (1997), Santa Elena is located in the Eastern Highlands, in a lower montane moist forest (mf-LM) ecological life zone, with a height of 2,600 masl, average temperature of 12.5 °C and average rainfall of 2,500 mm annually. The municipality of San Pedro is located in the Northern Highlands, in a lower montane rain forest (rf-LM) ecological zone, with an average height of 2,745 masl, and an average temperature of 14 °C.
Sampling and evaluation. We measured milk production in milking by direct readings and we took a representative sample from the proportional step gauges, Waikato®. These samples were sealed and transported to the laboratory for physicochemical analysis. Each container had some preservative (Bronopol®) to maintain the sample without risk of loss in the compositional state and free from the proliferation of microorganisms. The procedure was carried out during 14 months on 182 Holstein cows distributed within the herds as follows: Santa Elena (117); San Pedro: rural area El Despiste (47) and Alto Medina (18). We recorded information on milk production, parity number, calving season (1: first semester, 2: second semester), days in milk and the milking interval. For each cow, information on at least one full lactation between December 2008 and February 2010 was accumulated. The samples were subjected to physicochemical analysis in a Milkoscan FT120®, using infrared light which measures the percentage of fat and milk protein.
Statistical analysis. This research was carried out using as a basis the regression models previously described (Delorenzo and Wiggans, 1986; Cassandro et al., 1995; Liu et al., 2000). The first step was to determine the environmental effects that significantly affected each of the characteristics in the study. We fitted a multiple regression model for the sources of variation that we wished to study. Assumptions on homogeneity of variances, normality and independence of residuals were validated.
The general model for the percentage of fat was as follows:
γijklmn = β0 +β1γi +β2θj +β3 ηk+β4εl +β5δm +β6λn + eijklmn
Where:
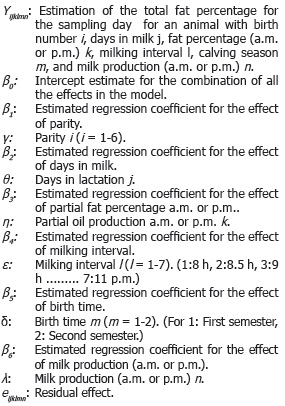
Two final prediction models were fitted, one based on the percentage of fat in the morning (a.m.) and another on the percentage of fat in the afternoon (p.m.). Only the significant effects were included in the model.
We also explored quadratic and interaction effects for each of the sources of variation and their significance levels to define the final prediction model. In addition, for the significance of the effects, the coefficient of determination (r2) was taken into account for the choice of the final prediction model.
A partial regression coefficient (β) was estimated for each of the significant variables in the definitive model, in order to predict the daily average percentage of fat of the samplings from the fat obtained in the milking in the morning or in the afternoon and the total daily milk production of the samplings. For all the statistical analyses, we used the software: Statgraphics (Statistical Graphics Corp. Rockville, MD, USA).
Results
Descriptive analysis. Milk production in the morning had an average of 9.26 ± 3.85 L, while milk production in the evening was 7.81 ± 3.18 L. The percentage of milk fat in the morning and afternoon was 3.38 ± 0.80 and 3.57 ± 0.69, respectively. The mean, standard deviation and coefficient of variation for all the characteristics included in the initial models are presented in Table 1.
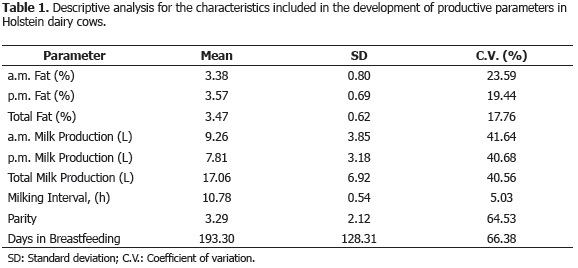
Prediction model for total fat percentage based on the morning percentage of fat (a.m.). For the first prediction model, we used the total percentage of fat per day as the dependent variable; and the a.m. percentage of fat and the other effects as independent variables. In this case, the partial production of milk (a.m. or p.m.), calving season, parity and milking interval had no significant effects (P>0.05); but the days in breastfeeding, a.m. fat percentage and the quadratic effect of a.m. fat percentage were highly significant (P<0.01). We fitted a multiple regression model with these effects (Table 2).
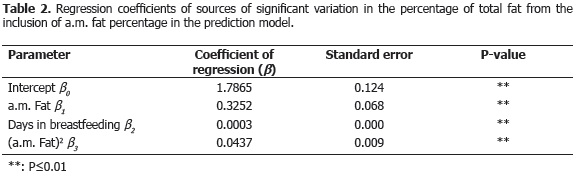
The prediction model chosen from the significance of the effects and the coefficient of determination was as
follows:
γ =β0 +β1η+ β2θ+ β3η2
Where:
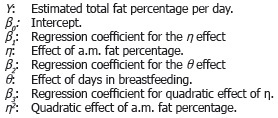
For the direct application of this model, we replaced the values for the regression coefficients of the significant effects, as shown in the following equation:
γ = 1.7861+ 0.3252 (η) + 0.0003 (θ) + 0.0437 (η2)
The reliability of the prediction model is given by the coefficient of determination (r2), which was 0.716, indicating that the effects included in the model explain 71.6% of the variability in the percentage of total fat per day; 28.35% is due to sources of variation not included in the model. The correlation coefficient (r) between actual and estimated values from the model's prediction was 0.842, which indicates a medium-high reliability for this model in the estimation of this parameter.
Prediction model for total fat percentage based on the p.m. percentage of fat. We used the percentage of total fat per day as the dependent variable; the p.m. percentage of fat and other effects were used as independent variables. In this case, the partial production of milk (a.m. or p.m.), calving season and the percentage of a.m. fat had no significant effects (P>0.05); however, parity number, days in milk, milking interval, p.m. fat percentage, effect of p.m. fat percentage and quadratic effect of parity were highly significant (P<0.01). Using the significant effects, we fitted a regression model (Table 3).
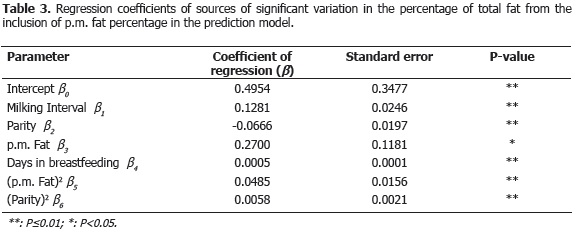
The prediction model from the model with the best Where: fit was:
γ =β0 +β1ε+ β2γ+ β3η+ β4θ+ β5η2 +β6γ2
Were:
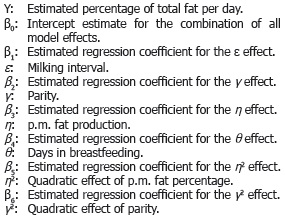
For direct application of this model, we replaced the values for the regression coefficients in the following equation:
γ = 0.4954+ 0.1281(ε) - 0.0666(γ) + 0.2700(η) + 0.0005(θ) + 0.0485(η2) + 0.0058(γ2)
The reliability of the prediction model is given by the coefficient of determination (r2), which was 0.652, indicating that the effects included in the model explained 65.25% of the variability in the average fat percentage of the sampling day; the remaining 34.75% was due to sources of variation not included in the model. The correlation coefficient (r) between actual and predicted data from the model's prediction was 0.807, which indicates that this model has a medium-high reliability for the estimation of this parameter.
We fitted two prediction models, one from the partial production in the morning and the other from the partial production in the afternoon. Only significant effects in the initial model were included in the final models.
Prediction model for the total milk production per day based on a.m. milk production. We used the total milk production as the dependent variable; whereas a.m. milk production and the other effects were used as independent variables. In this case, calving season, percentage of fat (a.m. or p.m.), quadratic and cubic effects and interactions between effects were not significant (P>0.05); however, parity, days in milk, milking interval and a.m.milk production were highly significant (P<0.01). We fitted a model with the significant effects (Table 4).
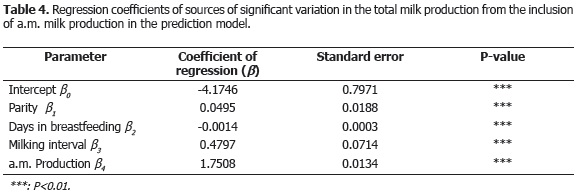
The prediction model from the model with the best fit due to the levels of significance and the coefficient of determination was as follows:
γ =β0 +β1 γ+ β2θ+ β3 ε +β4 η
Where:
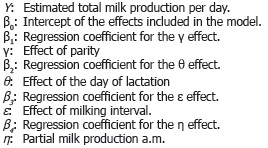
For direct application of this model, we replaced the values for the regression coefficients in the following model:
γ = -4.1746 + 0.0495(γ) - 0.0014(θ) + 0.4797(ε) + 1.7508(η)
The reliability of the prediction model is given by the coefficient of determination (r2), which was 0.977, indicating that the effects included in the model explained 97.77% of the variability of total milk production per day. The correlation coefficient (r) between actual and predicted data from the model's prediction was 0.988, which means that this model has a high reliability for the estimation of this parameter.
Prediction model for the total milk production per day based on p.m. milk production. This model was used with total milk production as the dependent variable; whereas p.m. milk production and the other effects were used as predictor variables. In this case, the calving season had no significant effect (P>0.05); however, parity, days in milk, milking interval, p.m. milk production, quadratic effect of parity, quadratic effect of days in breastfeeding, quadratic effect of p.m. milk production and the interaction effect between parity and days in breastfeeding were highly significant (P<0.01). We fitted a model with the significant effects (Table 5). The prediction model from the model with the best fit was as follows:
γ =β0 +β1 γ+ β2θ+ β3 ε +β4 η + β5
+β6 θ2 + β7 γ*θ +β8 η2
Where:
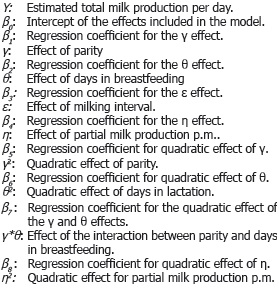
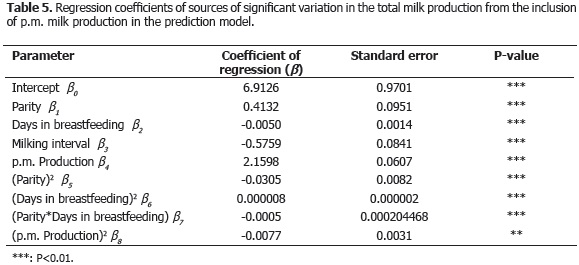
For direct application of this model, we replaced the values for the regression coefficients in the following model:
γ = 6.9126+ 0.4132(γ] 0.0050(θ] 0.5759(ε) + 2.1598(η) - 0.0305(γ2) + 0.0000008(θ2) - 0.0005(γ) (θ) - 0.0077(η2).
The reliability of the prediction model is given by the coefficient of determination (r2), which was 0.963, indicating that the effects included in the model explained 96.93% of the variability of total milk production in the day of sampling. The correlation between actual and predicted data from the model's prediction was 0.984, which means that this model has a high reliability for the estimation of the parameters studied.
Discussion
The trends of the predictive models estimated in this paper agree with those estimated by Liu et al. (2000), who evaluated factors that impact important characteristics, focusing on the daily performance of each individual and the variability in the composition of milk, demonstrating the effect between milking interval, days in milk, parity number and partial production. This research showed the effect of these factors on the percentage of fat and milk production.
The proposed model for prediction of fat percentage from sampling in the morning didn't show a significant effect from the milking interval; however, for fat percentage in the afternoon, the milking interval had a high level of significance.
The results of this research agree with those obtained by Soyeurt et al. (2006), who found a significant effect from the milking interval on the percentage of fat, when milking intervals are short. Similarly, Stelwagen et al. (2008) reported a significant effect from the milking interval on milk quality. Furthermore, Ouweltjes (1998) reported the effects of parity and days in milk on milk production as significant; along with some compositional characteristics of milk and fat. Lee and Wardrop (1984) found a significant effect from the season on the percentage of fat. In this research, this effect was not significant, perhaps due to the varying climatic conditions in the places where these studies were carried out.
Studies by Quist et al. (2008) suggest that there is an effect from the interaction between parity and season of calving on the percentage of fat; however, this relationship is governed by climatic conditions that differ from those of the tropics, and the effect obtained is of little influence. In this study, a significant relationship was only obtained between days in milk and parity on total milk production.
This research also found a significant effect from days in milk on a.m. and p.m. percentages of fat, and on milk production. It was also found that the quadratic effect of parity, days in milk and p.m. milk production were significant, contrasting with the reports of other authors who found no significance in these quadratic effects.
Similar results to the ones achieved in this research were reported in other studies (Camargo, 1994; Gonçalves et al., 1999; ), which state that the days in milk have a significant effect on milk production, but their results are different, showing that the season of calving was significant. Similarly, Ng-Kwai-Hang et al. (1984) reported the importance of days in breastfeeding and the effect of the time of year.
According to Pérez et al. (2007), the time of the year has no effect on fat percentage or milk production, which is consistent with the results of the present research.
Conclusions
Milk yield and fat percentage are affected by various environmental factors such as days in milk, parity and milking interval. The significance of these factors on the characteristics of importance allow for adjusting or correcting them, decreasing their variability, in order to obtain a good estimation of genetic parameters.
Predictive models will be useful in situations in which only one sample per day can be obtained. In our conditions, the milk production control programs only allow for one sampling of milk per day, so it is essential to use predictive models that reduce the effect of these sources of variation and allow for calculating daily milk productions and total fat, adjusting the variability of these effects. In this paper, we presented four prediction models for these important parameters. The coefficients of determination of each of the models are quite acceptable, considering the difficulties in the country in following-up on such cattle populations. These results constitute the first tool to be used by researchers in cases where environmental effects such as the ones described herein should be adjusted.
Since this is the first approach that has been carried out in Colombia for the analysis of different environmental factors on milk and fat production, further research is required because of the high impact of these effects. The best practice is to have a.m. and p.m. samplings, as well as increasing the sample size to increase the reliability of the estimated parameters. For now, these results will be useful for the research community and provide an initial approach, and are quite successful at generating predictive models used in animal breeding programs.
Bibliography
Camargo, A. 1994. Estudo de algumas características produtivas e reprodutivas de um rebanho mestiço Holandês-Zebu no Estado do Rio de Janeiro. Tese Mestrado em Zootecnia. Universidade Federal de Viçosa, Viçosa. 81 p. [ Links ]
Cassandro, M., P. Carnier, L. Gallo, R. Mantovni, B. Contiero, G. Bittante and G. Jansen. 1995. Bias and accuracy of single milk testing schemes to stimate daily and lactation milk yield. Journal of Dairy Science 78(12): 2884-2893. [ Links ]
Delorenzo, M. and G. Wiggans. 1986. Factors for estimating daily yield of milk, fat and protein from a single milking for a herds milked twice a day. Journal of DairyScience 69(9): 2386-2394. [ Links ]
Espinal, L.S. 1985. Geografía ecológica del Departamento de Antioquia (zonas de vida (formaciones vegetales) del Departamento de Antioquia). Revista Facultad Nacional de Agronomía Medellín 38(1): 1-106. [ Links ]
Garnsworthy, P., L. Masson, A. Lock and T. Mottram. 2006. Variation of milk citrate with stage of lactation and de novo fatty acid synthesis in dairy cows. Journal of Dairy Science 89(5): 1604-1612. [ Links ]
Gonçalves, T., M. Martínez, C. Rodríguez, R. Verneque e A. Gomes. 1999. Determinação de fatores multiplicativos para estimar a produção de leite no dia do controle leiteiro a partir da produção de leite da manhã ou da tarde. Revista Brasileira Zootecnia 28(5): 1000-1006. [ Links ]
Instituto Alexander von Humboldt, IAVH. 1997. Caracterización ecológica de cuatro remanentes de bosque seco tropical de la región Caribe colombiana. Grupo de Exploraciones Ecológicas Rápidas, IAVH, Villa de Leyva. 76 p. [ Links ]
Kay, J., W. Weber, C. Moore, D. Bauman, L. Hansen, H. Chester-Jones, B. Crooker and L. Baumgard. 2005. Effects of week of lactation and genetic selection for milk yield on milk fatty acid composition in Holstein cows. Journal of Dairy Science 88(11): 3886-3893. [ Links ]
Karijord, O., N. Standal and O. Syrstad. 1982. Sources of variation in composition of milk fat. Zeitschrift für Tierzüchtung und Züchtungsbiologie 99(1-4): 81-93. [ Links ]
Lee, A. and J. Wardrop. 1984. Predicting daily milk yield, fat percent, and protein percent from morning or afternoon tests. Journal of Dairy Science 67(2): 351-360. [ Links ]
Liu, Z., R. Reents, F. Reinhardt and K. Kuwan. 2000. Approaches to estimating daily yield from single milk testing schemes and use of a.m. - p.m. Records in test-day model genetic evaluation in dairy cattle. Journal of Dairy Science 83(11): 2672-2682. [ Links ]
Loker, S., F. Miglior, J. Bohmanova, J. Jamrozik and L.R. Schaeffer. 2009. Phenotypic analysis of pregnancy effect on milk, fat, and protein yields of Canadian Ayrshire, Jersey, Brown Swiss, and Guernsey breeds. Journal of Dairy Science 92(3): 1300-1312. [ Links ]
Miller, R. 1973. Recent study of age adjustment. Journal of Dairy Science 56(7): 952-959. [ Links ]
Ng-Kwai-Hang, K., J. Hayes, J. Moxley and H. Monardes. 1984. Variability of test-day milk production and composition and relation of somatic cell counts with yield and compositional changes of bovine milk. Journal of Dairy Science 67(2): 361-366. [ Links ]
Ouweltjes, W. 1998. The relationship between milk yield and milking interval in dairy cows. Livestock Production Science 56(3): 193-201. [ Links ]
Palmquist, D., A. Beaulieu and D. Barbano. 1993. Feed and animal factors influencing milk fat composition. Journal of Dairy Science 76(6): 1753-1771. [ Links ]
Pérez, L., R. Anrique and H. González. 2007. Non genetic factors affecting milk production and composition in a dairy herd with two calving seasons in Los Lagos Region, Chile. Agricultura Técnica 67(1): 39-48. [ Links ]
Quijano, J. y J. Echeverri. 2007. Estimación de algunos parámetros genéticos y ambientales para el porcentaje de proteína en leche. Revista Colombiana Ciencias Pecuarias 20(4): 582. [ Links ]
Quist, M., S. LeBlanc, K. Hand, D. Lazenby, F. Miglior and D. Kelton. 2008. Milking-to-milking variability for milk yield, fat and protein percentage, and somatic cell count. Journal of Dairy Science 91(9): 3412-3423. [ Links ]
Rodríguez, J.D., J.J. Echeverri y G. Correa. 2009. Efecto del intervalo entre ordeños y estimación de los factores de corrección para el porcentaje de grasa de la leche en vacas Holstein en condiciones tropicales. Revista Colombiana de Ciencias Pecuarias 22(3): 372. [ Links ]
Searle, S. 1962. Age and herd effects in New Zeland dairy cow records. Journal of Dairy Science 45(1): 82- 85. [ Links ]
Soyeurt, H., P. Dardenne, A. Gillon, C. Croquet, S. Vanderick, P. Mayeres, C. Bertozzi and N. Gengler. 2006. Variation in fatty acid contents of milk and milk fat within and across breeds. Journal of Dairy Science 89(12): 4858-4865. [ Links ]
Stoop, W., H. Bovenhuis, J.M. Heck and J.A. van Arendonk. 2009. Effect of lactation stage and energy status on milk fat composition of Holstein-Friesian cows. Journal of Dairy Science 92(4): 1469-1478. [ Links ]
Syrstad, O., N. Standal and O. Karijord. 1982. Concentration of various fatty acids in milk. Zeitschrift für Tierzüchtung und Züchtungsbiologie 99(1-4): 94-100. [ Links ]
Stelwagen, K., V. Farr, G. Nicholas, S. Davis and G. Colin. 2008. Effect of milking interval on milk yield and quality and rate of recovery during subsequent frequent milking. Livestock Science 114(2-3): 176-180. [ Links ]