INTRODUCTION
Electricity transmission and distribution systems are exposed to failures caused not only by nature but also by disruptive agents [1]. The effects of the latter kind of attacks have been historically evaluated using security analysis techniques, among which contingency analysis is a subset [2]. The traditional approach of security studies of power systems is based on N-1 or N-2 criteria and consists of ensuring that the system can continue operating normally, even if one or two elements are out of service [3].
Nevertheless, this approach cannot be applied to the modeling of simultaneous attacks of k elements because, in real systems, the number of combinations to be evaluated would be prohibitive [4]. Furthermore, current reliability policy and security standards for electric power systems around the world are limited to the analysis of a small set of events [5], and traditional reliability indices are not adequate to effectively plan for emerging dangers.
As a result, developing resilience indicators can help network planners to adequately budget the maintenance of the network and investments to improve its functionality in case of low-probability high-consequence risks [6].
In the technical literature, intentional attacks in which the disruptive agent deliberately tries to maximize its damage to the system have been analyzed using multilevel interdiction models. A model is defined here as a set of equations, functions, and mathematical formulas that represent a particular phenomenon, in this case, the interdiction problem. Interdiction models generally implement a simplified representation of transmission networks. Such simplification, known as the DC model, is achieved using linear equations that represent the relationship between power injections and flows in the network. However, a few interdiction multilevel studies have used a more realistic representation of the network, i.e., an AC model. This is because using a DC model of the network facilitates the use of exact methods to solve the interdiction problem, while the AC model limits the solution methods to heuristic or meta-heuristic strategies.
Network operators can adopt different strategies in the face of an attack. The most common of them is redispatch [1], [7], but other possibilities are modifying the topology of the network [8] or exploiting distributed generation (DG) to operate in isolation [9], which is the most widely used in distribution networks.
However, multiple reliability metrics that are generally accepted often exclude important interruptions caused by unexpected events; for instance, the Customer Average Interruption Frequency Index (Caifi) and Customer Average Interruption Duration Index (Caidi). As a consequence, according to the existing reliability metrics, a highly reliable power system may not necessarily be resilient. The objective of resilience is not only to withstand all possible disaster scenarios but also to have quick and efficient recovery measures [10].
The number of studies regarding the vulnerability of electric power systems has presented an important upward trend in recent years. A bibliometric analysis using the keywords power system vulnerability and power system security in the databases Science Direct, Scopus, and Web of Science produced the results in Figure 1 and Figure 2.
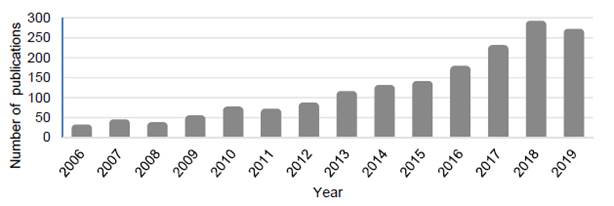
Source: own elaboration.
Figure 1 Number of publications that include the term power system vulnerability (2006-2019).
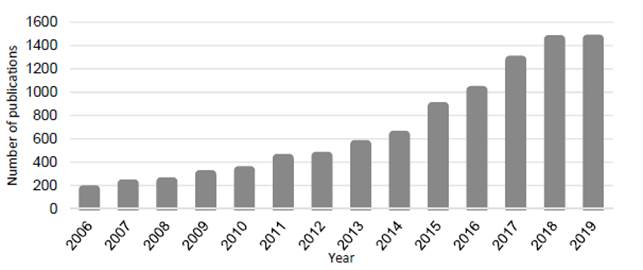
Source: own elaboration.
Figure 2 Number of publications that include the term power system security (2006-2019).
Although Figure 1 and Figure 2 present a similar trend of both keywords, the second case includes a much higher number of publications. For instance, in 2018, the number of vulnerability studies of power systems reached a total of 282 publications, while there were 1,451 security studies. It should be clarified that although there have been many vulnerability and security studies of power systems (as proven by our preliminary search in the databases above), only a small subset of them addresses the topic of interest in this article. This literature review focuses on vulnerability studies of power systems, more specifically, those that include deliberate attacks and are modeled using multilevel programming.
This article is structured as follows. The first section introduces and defines concepts of vulnerability studies of power systems. The second section classifies these studies paying special attention to multilevel models. The third section describes future challenges, which consist of incorporating new defense options for network operators. Finally, the fourth section presents the conclusions.
1. THE VULNERABILITY PROBLEM
In [11], the authors define vulnerability as the decrease in the performance of an electrical network in the face of a disturbance and present a correlation analysis of different vulnerability metrics. In [12], the authors reviewed the literature on the resilience of power systems in order to face high-probability high-risk events. They associate the vulnerability problem of power systems with a specific triggering event. Therefore, defining the triggering event is the first step in a vulnerability analysis.
Vulnerability studies can be grouped by triggering events: random failures, natural threats, and malicious attacks [13].
Random failures are a series of triggering events that exhibit wide variations and uncertainty [14]. These failures can be modeled by (a) randomly eliminating a given part or number of components of a system; (b) assigning a failure probability to each component and then comparing that probability with a random number between zero and one uniformly distributed to assess the state of the component [15]; or (c) selecting, first, the number of defective components based on a given distribution and, then, randomly eliminating a set of components with the selected number [16].
The second type of vulnerability analysis considers natural threats such as earthquakes, hurricanes, floods, and thunderstorms [17]. These dangers can cause the components of a system distributed over an area of influence to fail simultaneously. The impact of these natural hazards on the components of a system is generally modeled using fragility curves. The latter show the probability of exceeding a certain threshold of damage, which is constrained by a selected danger intensity measurement, such as maximum acceleration of the terrain, maximum soil speed, permanent deformation of the ground for seismic dangers [18] or gust wind speed for hurricane dangers [19].
The third type is the analysis of vulnerability in the face of malicious attacks. In this case, on the one hand, a disruptive agent tries to maximize the damage to the system by intentionally attacking some of its components [20]. On the other hand, the network operator minimizes the damage by redispatching or making topological changes to the network. Due to its action-reaction nature, the vulnerability to disruptive attacks is analyzed using multilevel optimization models [1].
Figure 3 presents a classification of events in electric power systems. Although the events due to internal failures were the most frequent (47.86 %), those caused by natural disasters, vandalism, and cyber attacks still resulted in 37.85 % of the total power cuts around the world from 1965 to 2012 [10]. This highlights the importance of studies into the vulnerability of electric power systems to intentional attacks.
2. CLASSIFICATION OF VULNERABILITY ASSESSMENT APPROACHES
The specialized literature presents different approaches to analyze vulnerability. This section describes the conventional vulnerability analysis models and those that involve multilevel programming.
2.1 Conventional vulnerability models
The conventional models of the vulnerability of electric power systems do not usually consider intentional attacks. Such models are focused on finding a set of critical elements that, if out of service, would cause the biggest problems to the system, whether in terms of load shedding, overloading of other elements, or technical aspects such as voltage stability issues [21]. In order to identify critical lines in a power system, the network can be modeled as a graph with links (transmission lines, transformers, etc.) and nodes (busbars, substations, etc.). This approach was proposed in [3] for a vulnerability analysis using what the authors call an improved maximum power flow. Similar studies [2], [22] rank critical lines in a context of safe and economical electricity dispatch.
In addition to power lines and transformers, substations and generators can also be critical elements in electric power systems. In [23] the authors considered substation failures that can occur sequentially and non-synchronously. In particular, they found that this kind of attacks generates many combinations, and these failures may cause large-size blackouts. Additionally, they proposed a metric called the sequential attack graph and a strategy based on such metric.
The vulnerability metrics in [24] are useful to implement guidelines to plan, design, invest in, and operate electricity networks. In countries such as China, studies have identified critical attack areas with three types of vulnerabilities, and a comparative analysis was conducted using vulnerability metrics under different tolerance parameters [25]. Likewise, a multidimensional vulnerability analysis was carried out based on given metrics and failure scenarios in order to identify critical areas from the topological and functional standpoints [26].
Another approach [4] combines traditional metrics based on the physical and operating characteristics of the network with two newly proposed metrics: entropic degree and network capacity. This approach can be used to evaluate the structural vulnerabilities of electric power systems.
The tolerance of electric power networks to contingencies has been analyzed in the context of complex network theory [27] by comparing the efficiency of the system with a newly defined parameter such as network capacity. The efficiency and capacity of the network are compared by estimating its vulnerability in terms of both metrics.
The effects of uncertain repair times and recovery resources after the interruption to the critical infrastructure are investigated in [28]. In that case, a restoration framework is applied to the British electric power system, and the results demonstrate the added value of using a stochastic model instead of a deterministic one.
Because electric power systems should reestablish their operation in the face of contingencies, the concept of resilience and their dimensions in distribution networks have also been studied. The methodology proposed in [29] is based on mixed-integer linear programming and an adequate evaluation of the resilience of smart distribution systems. Such model examines the formation of dynamic microgrids, their service areas, and the optimal management of different technologies, such as energy storage systems, demand management programs and distributed generation.
In [30], the authors proposed an improved model to study the reconfiguration of a distribution network based on a refined genetic algorithm. Their objective was to minimize the energy losses of the system.
Another defense strategy based on planning is assigning resources to strengthen the electric power network in order to maximize its immunity against malicious attacks [31], not only by increasing the protection of the power system components but also by building redundant schemes [32].
Other authors have proposed simple critical interdiction strategies for transmission systems [33], [34]. Their method is based on a greedy algorithm in which the transmission line with the highest load is interdicted at each iteration.
The effectiveness of protecting transmission lines identified as promising candidates for interdiction has also been investigated. In [35], two typical models to evaluate the vulnerability of an electric power network were used under intentional attacks: a purely topological model and an interrelation-based model. Afterwards, their results were compared with a DC power flow model.
The inclusion of renewable energy sources on a large scale in distribution networks and its impact on vulnerability was modeled in [36] using a complex network based on a bidirectional flow that considered the change in topology and the power flow pattern in the smart grid.
2.2 Bilevel models
Multilevel models are based on the hypothesis that, on the one hand, there is an aggressor whose objective is to cause maximum damage to the system; and, on the other hand, an agent reacts to the disruptive actions, modifying its operating scheme in order to minimize the damages caused by the attack. This attack-defense relationship can be modeled using a bilevel programming structure (see Figure 4.).
In Figure 4, the aggressor should determine an attack scheme that maximizes the damage to the network (measured in load shedding). For that purpose, it should consider the fact it has limited resources and anticipate the system operator’s response. From the viewpoint of game theory, this dynamic represents a Stackelberg competition [38]. In this type of games, there are a leader and a follower who have conflicting objectives. The leader should determine its game strategy by anticipating the possible reaction of the follower [39]. In addition, the objective function of the leader depends on the decision made by the follower. In the vulnerability problem, the leader is the aggressor and the value of its objective function (load shedding) depends on the network operator’s strategy.
The vulnerability problem under a bilevel programming scheme, also known as the terrorist threat problem, was first proposed in [7]. Later, the model in [7] was generalized in [1], thus allowing users to define different objective functions for both agents. In [40], the authors proposed minimum and maximum vulnerability models. The former aims to find a minimum number of elements to attack in order to cause a previously defined load shedding, while the latter aims to cause as much shedding as possible with a predetermined number of elements to attack.
Given the non-convexity intrinsic in multilevel programming models, some authors have adopted metaheuristics to address the vulnerability problem. In [41], the authors proposed a genetic algorithm (GA) to solve the interdiction problem considering the topological modification of the system an additional strategy for the network operator to minimize load shedding.
A hybrid GA that involves a local search was used in [42] to address the vulnerability problem in different demand and generation scenarios. In [43], the authors implemented an iterated local search (ILS) to solve the vulnerability problem modeling attacks to lines and generators. In [44], other authors compared different metaheuristics (including GA, ILS, taboo search, and Grasp) applied to the vulnerability problem of electric power systems. Benders decomposition, another numerical tool, has also been employed to deal with interdiction problems, as described in [45].
The vulnerability of power systems can also be analyzed using a specific time horizon. In [46], the authors presented a vulnerability analysis model that can be used to determine where and when (over a given horizon) a power system will be more vulnerable to intentional attacks. In this case, at the upper level of the optimization, the disruptive agent should determine an attack plan that includes the elements to attack and the best time for such attack.
The interdiction model presented in [44] identifies the attacks that have the biggest impact on the power network. The contribution of this model lies in the fact that it incorporates the impacts of the possible attack in the short term (seconds to minutes) and the long term (minutes to days). The medium-term impacts are examined using a DC optimal power flow (DCOPF) model. The short-term impacts are addressed by means of an interruption cascade analysis model that uses a set of verifiers sequentially applied.
A common characteristic among most vulnerability studies is the representation of the transmission network using a DC model. This simplifies the modeling of the problem and, as a result, a bilevel model can be transformed into a single-level equivalent. However, DC models do not consider the effect of reactive power and network voltage limits.
In turn, the interdiction model in [43] and [47] uses an AC model of the transmission network. Such model can produce more realistic results because it considers voltage limits, the contribution of the reactive power, and the losses at the transmission network elements. Another vulnerability model [48] implemented an AC model of the network and quantified the impact of the attacks to said network in different ways, including the deviation of the voltages at the busbars and the minimum load that should be eliminated in order to restore the network to a stable operation.
The integration of information technologies into power systems makes the latter vulnerable to cyber attacks. This type of attacks can even affect the service provision or the information of the control and protection systems by injecting fake data [49]. Possible scenarios of coordinated attacks can be found in the specialized literature. The power grid is becoming more vulnerable to various kinds of cyber and physical attacks. Coordination between the attacks could bring higher impacts on the power system, as evidenced by the 2015 Ukrainian power system cyberattack. There is limited study in existing literature about possible coordinated attack scenarios and the detailed mathematical modeling of them. To prevent future coordinated attacks against power systems, in this paper the cyber-physical security of the power system is analyzed and probable coordinated attack scenarios are proposed. In [50], the authors studied in detail two typical examples of attack coordination: (1) between the load redistribution (LR) attack and the attackers; and (2) between the LR attack and the attacked lines. Operators could be misled to develop an uninformed energy dispatch strategy and, as a result, load reduction could be maximized.
2.3 Trilevel models
Trilevel vulnerability models contain the attack-defense bilevel scheme mentioned above and involve a third agent at the upper level of the optimization. The new agent is the network planner, who should make decisions about the construction of new reinforcements in order to minimize the impacts of eventual attacks. The attacker and system operator are on the second and third level, respectively. Figure 5. presents a scheme of the interaction between these agents. In this case, the system planner receives information about the lower-level problems based on which it makes decisions regarding network reinforcements.
In other studies [6], [9], trilevel vulnerability models have been applied to distribution systems. In [6], the objective function was formulated as the load shedding cost, in addition to the operation cost of the generator. Furthermore, the trilevel problem can be broken down into two stages: master and slave problem. Dual transformation has been proposed [51], [52] the authors numerically validate and analyse the tri-level transmission expansion planning (TTEP) to turn the trilevel model into a single-level one, which ensures a global optimum. In [53], the objective function was the load shedding cost, and the authors proposed the conversion to a bilevel problem using the duality principle. Finally, they utilized Benders decomposition to solve the problem.
In [54], the model was focused on protecting the network against cyber-physical attacks from the viewpoint of the allotment of defense resources. A coordinated attack means a physical short circuit of the transmission lines after hijacking the communication network of the safety relays. A systematic review of interaction models and solution methods in studies of the interaction between cyber-networks and power systems was presented in [49]. In turn, unidentifiable attacks produced by cyber attacks (wrong readings of meters) were considered in the reliability evaluation of power systems in [55]. More cyber technologies are being deployed in the modern cyberphysical power systems. However, as a result higher cyberattack risks will be brought about. Unidentifiable attacks, which are a type of emerging false data injection attacks, could affect the outcomes of State Estimation (SE).
In [56], a trilevel vulnerability model was implemented using an algorithm that generates columns and restrictions. The results showed that the protection using the optimal solution of the defender-attacker-defender model always enabled the network to survive in case of contingencies. Some defense strategies studied in the literature include the formation of DG islands and the reconfiguration of the network [9]. The model in [57] introduced multiple sets of uncertainty to characterize possible interruptions caused by attackers, and the probabilities of the multiple sets of uncertainty were estimated by means of an analytic hierarchy process.
Another perspective on vulnerability evaluation [13] aims to mitigate the vulnerability of the power system to the worst attacks located in space. These attacks are defined as the failure of a group of system components distributed over an area due to natural dangers or malicious attacks, while other components outside that area do not fail directly.
Regarding the allotment of resources for the defense or reinforcement of an electric power network, other authors [37] have proposed a two-stage solution approach that achieves optimization with a moderate computational effort. The original trilevel program is transformed, first, into an equivalent bilevel program, which is later solved using an implicit enumeration algorithm.
Table 1 presents a classification of the literature reviewed in this paper specifying the type of network model, solution method and defense strategies modeled for the network operator.
Table 1 Multilevel programming studies applied to the vulnerability problem of electric power systems.
Ref. | Multilevel prob. | Type of system | Model | Solution method | Defense strategies | ||||
Bilevel | Trilevel | Dist. | Transm. | AC | DC | Exact | Heuristic | ||
[1] | X | X | X | X | Redispatch | ||||
[5] | X | X | X | X | Redispatch | ||||
[6] | X | X | X | X | Planning | ||||
[7] | X | X | X | X | Planning | ||||
[8] | X | X | X | X | Redispatch | ||||
[9] | X | X | X | X | Reconfiguration/Islanding | ||||
[37] | X | X | X | Planning | |||||
[40] | X | X | X | X | Redispatch | ||||
[41] | X | X | X | X | Reconfiguration | ||||
[42] | X | X | X | X | Planning | ||||
[43] | X | X | X | X | Redispatch | ||||
[44] | X | X | X | X | Redispatch | ||||
[45] | X | X | X | X | Planning | ||||
[47] | X | X | X | X | Planning | ||||
[48] | X | X | X | X | Reconfiguration | ||||
[51] | X | X | X | X | Planning | ||||
[52] | X | X | X | X | Planning | ||||
[53] | X | X | X | X | Planning | ||||
[54] | X | X | X | X | Planning | ||||
[57] | X | X | X | X | Reconfiguration | ||||
[58] | X | X | X | X | Planning |
Source: own elaboration.
2.4 Future research
Future studies to solve the interdiction problem are connected to multiple fields. They include new formulations of mixed-integer linear programming models that represent the interdiction problem and integrate primary control actions in a single level, as proposed in [1], or incorporate uncertainty into terrorist objectives [7]. Other proposals introduce alternative solution techniques based on heuristics [8], [41].
Nevertheless, multilevel models need greater detail in the modeling of power systems, which involves more complex solution methods that could be considered in future work; for instance, the use of genetic operators in the metaheuristic applications [5]; stochastic programming to model the load, generation, and topology of the network [6]; and linear approximations of the min-max model, as proposed in [7]. Other studies seek to optimize computational calculation times [5], [9]. Finally, some defense strategies are the connection or disconnection of fast-acting generating units [8], [41], the mitigation of consequences of attacks (cascading outages, temporary stability, etc.) [7], and network reinforcement based on planning and considering stochasticity and distributed generation [9].
3. CONCLUSIONS
This paper presented a review of the literature on the vulnerability problem in power systems. Such review was mainly focused on the description of biand trilevel programming models. Bilevel models represent attack-defense dynamics between a disruptive agent and the network operator, while their trilevel counterparts involve the network planner and represent defense-attack-defense dynamics.
The studies we retrieved were classified based on their solution method, network model, and type of system. This review found that most vulnerability or interdiction studies were focused on the analysis of transmission systems. That is because attacks to distribution systems have less serious consequences regarding the load level and number of affected users. Most authors adopted a simplified network model, i.e., a DC model, to represent the transmission network, which enabled them to solve the problem using exact techniques based on mixed-integer linear programming. In addition, the studies that implemented an AC model of the network adopted heuristic methods to solve the vulnerability problem. The defense strategies in bilevel problems were redispatch or network reconfiguration. In turn, trilevel models considered network reinforcements to be the core defense strategy against eventual attacks.
This literature review also revealed future research lines to analyze the vulnerability of power systems. They include new mathematical formulations to achieve more accurate representations of the network; the introduction of uncertainty to model the system load and generation; new (heuristic and exact) solution techniques and novel defense strategies for network operators and planners that incorporate the effects of demand response, distributed generation and energy storage programs.