Introduction
Aging is a complex phenotype that develops through the intervention of various cellular and molecular processes 1. Some of these processes are related to the reaction to the damage accumulation in the DNA, senescent cells number increase, mitochondrial dysfunction, stem cells depletion, epigenetic modifications, alterations in the cellular communication, and proteostasis loss 1. One of the tissues most vulnerable to the effects of aging is the central nervous system (CNS).
The CNS is particularly susceptible to the decline in the function over the years, since its recovery is limited by the presence of post-mitotic cells. Structural and functional modifications associated with aging have been described in the CNS. Images obtained through magnetic resonance evidence a decrease of the gray matter 2. Histologic analyses report, beside dendritic regression and signs of neuronal death, especially in the prefrontal cortex (PFC), also demyelination of the white substance, and DNA oxidative damage in the oligodendrocytes 3 . In general, neurons undergo cell death due to energy deprivation, mitochondrial dysfunction, calcium overload and the impact of disproportionate inflammatory processes 4 . With advancing age, DNA oxidative damage and increase of its activation state in glia cells have been identified 5. All this leads to a decline of the CNS functions and to an increase in the vulnerability to suffer neurodegenerative diseases 1. Not all brain regions age in the same way; it has been observed that the PFC is more susceptible to exhibit characteristics associated with aging, for this reason, it has been subject of various researches in order to identify which processes are altered by aging in this zone 6.
With aging, the speed of the information processing and of the capacity of short-term and long-term memory decreases; besides, there is an alteration in the impulse control, all these functions depend as much on the modification of the impulse control, as on the integrity of the PFC, the hippocampus and the post-rolandic cortex too 7-11. Transcriptomics studies of aged PFC have consistently reported decrease of the synapsis function and activation of the neuroglia cells 12-15. Recently, modifications in the splicing mechanisms associated with the transcriptome in the PFC have been found 16. However, it is not clear which are the initial causes or the molecular mechanisms underlying these alterations.
One step forward in understanding the molecular processes involved in aging is the identification of the hub genes in the transcriptional deregulation associated to the process. To accomplish this, a weighted correlation network analysis (WGCNA) was conducted. This is a data mining procedure used in analyzing biological networks based on paired correlations between variables 17. For this specific research, WGCNA was used to identify central genes in the modification of the aging transcriptome, thus comparing the transcriptomes of 45 persons aged between 60 and 80 years and the transcriptomes of 38 persons aged between 20 and 40 years. Five genes with high topologic characteristics were identified.
Four of them -HPCA, CACNG3, CA10, PLPPR4- were repressed and one overexpressed CRYAB. The four repressed genes are expressed preferentially in neurons and regulate the synaptic function and the neuronal plasticity, while the overexpressed gene is characteristic for neuroglia cells, its expression is activated as a response to neuronal damage and facili tates myelinization and regeneration. Identifying these hub genes underlines the role of the synaptic dysfunction in brain aging and offers potential biomarkers and therapeutic targets for modifying the normal and pathological aging processes.
Materials and Methods
Data Selection: A search in the transcriptomics database GEO OMNIBUS (https://www.ncbi.nlm.nih.gov/geo/) on researches studying the expression of prefrontal cortex genes in humans throughout life and with a high number of biological replies. For this purpose, key words were used such as human prefrontal cortex, aging, lifespan. Selection criteria for obtaining a data set were original data availability (raw data with no previous processing), and that the study had at least 30 biological replies in each interest age group: 20 to 40 years old and 60 to 80 years old. Samples should be taken from healthy people, with no decreased mental status nor neurological diseases at the moment of death.
Quality Control: For each microarray a percentage of probesets with fluorescence, background fluorescence, RNA degradation profiles, intensities density histogram; and establishment of standard errors failures were identified. Microarrays with negative outcomes in at least one of the measured parameters were not included in the subsequent analysis. Quality control was conducted through the qc.affy function of the sympleaffy package used in R (https://www.r-project.org/) 18.
Correction of the Batch Effect: In studies in which a large number of samples is analyzed and, furthermore, samples are difficult to collect, as in the case of human brain tissue, it is common that samples are processed in different days. Affymetrix microarrays are very sensitive to variations of the conditions under which hybridization is conducted, therefore, this possible confusion factor should always be considered. Identifying the batch effect was carried out by recovering the hybridization date of each microarray through the pdata command of the affydata package implemented in R program for statistical analysis (https://www.r-project.org/) 19. Once the different microarray hybridization dates (batches) have been identified, this non-biological variability source was corrected by using a methodology based on nonparametric Bayesian statistics implemented in ComBat 20. The extent of the batch effect correction was assessed by a main component analysis (MCA). MCA is a technique for dimensionality decline that allows identifying unbiasedly the natural relation between the samples composed of different variables. Thus, it is expected that, if the effect of a confusion factor (the same batch) produces important modifications in the transcriptome, the samples that share the same batch in a natural way should be closer to each other than even to other that share the same experimental group, in the case of this research, age.
Identifying Differentially Expressed Genes: Microarrays were assigned to two different experimental groups: elderly (aged 60-80 years) and young (aged 20-40 years). Besides, all were simultaneously normalized using RMA. RMA algorithm was also used for determining the expression levels of each gene in the microarray. In order to detect differential expression genes (DEG) in the two experimental groups, the limma statistics package (lineal models for microarray data) was used 21. With this methodology the expression change was calculated and the statistical significance adjusted for multiple comparisons (FDR - false discovery rate). It was a priori established that a gene is differentially expressed if an expression change is higher than |1.2| and a fdr value lower than 0.05.
Biological Interpretation of the Genes: An overrepresentation analysis of the biological pathways was performed using the DAVID tool with the database of biological process pathways of gene ontology (GO) 22. Repressed and overexpressed GED were analyzed independently in order to have an intuitive interpretation of the deregulation direction of the altered pathway.
Gene Coexpression Networks Analysis (WGCNA): Coexpression networks were developed based on the GED between older and young. The similarity matrix was calculated, by identifying the Pearson correlation coefficients of the expression levels along all samples for all possible gene pairs. The similarity threshold was then identified, it was calculated with the adjacency function that is established according to the unique characteristics of each similarity matrix 23. In order to select the threshold, the adaptable method developed by Elo was used 24. The method compares the grouping coefficient of the network (Co) with the grouping coefficient expected for a random network (Cr) by different tao values. Finally, the adjacency matrix of the network was established, this is a 2x2 matrix that allows the representation of binary relationships, in this case it represents if a pair of genes do coexpress (1) or do not (0). All processes for the WGCNA were performed in an R environment (https://www.r-project.org/) using statistical functions embedded in the same environment.
Visualizing and Analyzing Coexpression Networks: For the visualization of the coexpression networks Cytoscape open code program was used, and also for ulterior studies on this matter different algorithms implemented in the same program have been used 25. The topological characteristics of all genes were established according to ten different algorithms: six of them are global descriptors (bottleneck, closeness, grouping coefficient, eccentricity, radiality, stress), and the remaining four are local descriptors (intercalation, degree, DMNC, MNC) 26. A gene was identified in the network as being a hub if it was among the first ten genes with the highest values for at least seven of the ten studied topologic characteristics. It was decided that seven measures will be the cutting point, since six are global descriptors, therefore, if a gene is among the ten best measures of at least seven descriptors, this gene is important within the network, globally as well as locally.
Validation of Hub Genes for Coexpression Networks: Genes identified as hub genes in the coexpression networks were validated against the results of a meta-analysis of four independent transcriptomics studies on human PFC in which the transcriptomes of persons between 60 and 80 years old with persons between 20 and 40 years old were compared for DEG identification 15.
Results
Quality Control and Differential Expression Genes Identification in Elderly and Young People
The dataset with the highest number of biological replies within the ages of interest (60 to 80 years old and 20 to 40 years old) in GEO OMNIBUS was GSE71620 10. In this study, the expression levels of all PCF genes, Brodmann 11 (BA11) were analyzed. All persons included in the study were neurologically healthy at the time when the brain tissue was collected. Measuring the gene expression level was performed with the Affimetrix "Human Gene 1.1 ST Array" microarray. After quality control, four microarrays were removed (three of the group of elderly and one of the group of young) because they had expression levels very different to the remaining data. It was also identified that the dataset had been hybridized in 19 different dates, this potential variation source was adjusted with ComBat 20. Figure 2 shows the non-biased distribution of the samples under study in this research: 45 samples of the group of elderly and 38 samples of the group of young.
Figure 1 shows the two principal components (PC) containing the greatest variation of the dataset, as a whole they represent 18 % of variability.
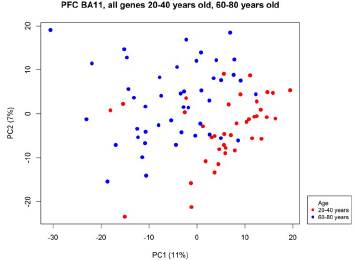
Figure 1 Principal component analysis (pca) of 45 samples of the group of elderly and 38 of the group of young
PC1 represents the largest part of the separation of the samples of the group of the elderly contrasted with the group of the young. Although there is no categorical separation between groups, the sample distribution shows that there are differences in the gene expression in the PFC with regard to age. Young tend to be located towards the positive side of the PC1, while elderly towards the negative side of the MC1. Samples mixed with the opposite group also show the variability in individual aging processes and that there are also differences between chronological and biological age.
When comparing transcriptomes of the elderly with those of the young, 276 repressed genes and 166 overexpressed genes were identified, amounting to a total of 442 DEG out of the 18840 genes in the microarray. With these genes, with different expressions between the study groups, the subsequent analyses were conducted.
In order to establish the biological relevance of the DEG, a pathway overrepresentation analysis was performed using DAVID, repressed genes and overexpressed genes were analyzed separately, thus making it possible to identify the direction of change of the altered biological pathway 27. Table 1 represents the ten pathways with more significant p values. In the group of the repressed genes it was found that some genes took part in biological processes characteristic of the neurons, such as synaptic transmission, ion transport, glutamate signaling pathway, and some biological processes related to conduct and perception, such as sensory pain perception and memory (table 1A). In the group of overexpressed genes, pathways related to the development of astrocytes, myelinization and inflammation were found as being overrepresented (table 1B).
A. First ten pathways overrepresented in the list of repressed genes. B. First ten pathways overrepresented in the list of overexpressed genes. The biological pathways correspond to the database of GO biological processes. The Counting column shows the DEG number in the pathway, Enrichment indicates how many times there are more genes than the randomly expected.
Development of the Weighted Coexpression Networks
With the expression levels of the 442 DEG in the 83 samples a correlation matrix was developed, then the similarity threshold was identified and the adjacency matrix was computed. The similarity threshold was calculated through the maximum local method, and it was found that a Pearson correlation coefficient equal or higher than 0.75 differentiates the distributions of the correlations between the study genes and those of a random gene population 23. This threshold allowed to separate genes with low correlation coefficients from genes with the highest correlation coefficient, 251 genes out of 442 DEG were selected.
With the 251 highly correlated genes, an adjacency matrix was developed and, on this base, the coexpression networks were visualized in Cytoscape 25. Figure 2 shows the coexpression networks for the DEG between elderly and young. Three networks were pro duced, the largest one (figure 2A) is composed of 158 repressed genes. In the second largest network, composed of 89 overexpressed genes (figure 2B), two subnetworks are identified: one that is highly connected and composed of 68 genes, and another smaller network, with a lower connection level, composed by 21 genes. Additionally, there is a network of four overexpressed genes connected to one another (figure 2C). Five DEG did not connect to any of the networks described before.
Visualization of the coexpression networ in Cytoscape. A. Coexpression network of repressed genes. B. Coexpression network of overexpressed genes. C. Network of four overexpressed genes. The nodes are represented as hexagons, each node correspond to one gene, the lines connecting a pair of nodes represent a Pearson correlation coefficient higher than 0.75. The repressed genes are represented in green and the overexpressed in red. In each network the hub genes are represented for their high topological level by bigger hexagons, colored in blue the repressed genes, and in orange the overexpressed genes.
In order to identify the hub genes in the coexpression networks, a topological characterization of these by cytoHubba was performed 26. The two networks with the highest number of genes (figure 2A and table 2A) were topologically characterized by ten different algorithms (table 2), the genes with higher outcomes for at least seven of the ten measures are selected as hub genes. Four repressed genes in the coexpression network were identified (figure 2A and table 2A), and one in the overexpressed genes network (figure 2B and table 2B).
A. Hub genes selected in the repressed gene coexpression network. B. Hub genes selected in the overexpressed gene coexpression network. The Average column shows the average of the measure for all genes in the network as reference point. The Top 10 Total column represents the number of measures in which the selected gene was among the ten highest scores for the ten topological measures.
As an external validation of the results, the expression change direction of the hub genes was compared with the results of a meta-analysis in which four independent studies different to the one used in the present research were integrated, these characterized the transcriptomic response to aging in the PFC 15. Of the five identified hub genes, three were analyzed in the meta-analysis -CA10, PLPPR4, CRYAB- and all of them presented the same direction of change found in this study, the first two repressed, and the third one, overexpressed. These results suggest that the identified genes are reliable and that the result could be generalized to the aging process of the PFC in humans. The remaining two genes that were not studied in the meta-analysis - HPCA and CACNG3- had the best values in the topological measures in the coexpression network of repressed genes. Due to the high consistency to the genes that were studied in the meta-analysis, the latter were conserved although it was not possible to confirm their direction of change with the external study.
Finally, based on the detected values in the microarrays for each hub gene in all the studied samples, a two-ways heatmap was developed (figure 3); this in order to determine the capacity of the hub genes to differentiate older from young and to know the distribution of the expression levels in each analyzed person.
The heatmap was developed by clustering genes by similarity in the expression levels through the samples (vertical clustering), and grouping the samples by similarity in the gene expression levels (horizontal clustering). The group of the elderly is represented in orange and the group of the young in blue; the numbers of the right vertical bar correspond to the ages of the people in the study. The intensity level of each gene was normalized with a Z transformation (left superior bar); the blue color represents the low expression level, while the red color represents the high expression.
In the heatmap the repressed genes were organized in a cluster, the only overexpressed gene (CRYAB) remained outside this cluster. This gene has the most homogeneous expression levels among the samples of the same experimental group.
Samples were organized in two clusters, cluster 1 was mainly composed of the samples of the young persons (34 of this experimental group), and seven of elderly. This cluster, in turn, is organized in two clusters, a larger one where the distribution of the hub gene intensities is homogeneous, and another smaller cluster, marked with a red arrow in Figure 3, composed of nine samples of young and five of elderly. In that cluster, although the behavior of the hub genes is similar to almost all samples of the young, in one or two genes by sample the expression change direction is different to almost all samples of its age group. Cluster 2 is predominantly composed of 38 samples of the group of elderly and of three samples of the group of young.
If cluster 1 is of the samples of the young individuals and cluster 2 of the samples of the elderly, in sum there are eleven samples in the opposite group, these correspond to 13.3 % of the analyzed persons. This way, combining the expression of the five hub genes allows the correct classification of 86.7 % of people, according to the experimental group.
Discussion
Aging appears in heterogeneous ways in humans, since it is associated with genetic, environmental and random factors that cannot be totally controlled in this kind of researches 14,28-30. In PCA (figure 1) this heterogeneity was evidenced since, when unbiasedly identifying the natural relations within the transcriptome in samples of persons of the two groups at the opposite ends of life expectancy, it was found that, although a high percentage of young persons can clearly be differentiated from the older, it is not possible to totally separate both experimental groups. There is a transition zone shared by young and elderly, even further, some young people mix with older persons and vice versa. It is tempting to propose that the persons in the group of the young who are nearer to the negative PC1 zone (figure 1) are people whose biological age is higher than the chronological age, while the persons of the group of the elderly located in positive PC1 regions were in the opposite situation: a biological age lower than their effective chronological age. Unfortunately, the experimental design of our study does not allow the verification of this hypothesis since the samples were obtained from post mortem brains and it is therefore not possible to track each one of them.
When the transcriptomes of the group of the elderly and those of the younger were contrasted, 442 DEG were found. When taking age as a dichotomic variable (older or young) the identified genes represent the transcriptional deregulation common to most persons analyzed between ages 60 and 80 years. In order to identify the central processes secondary to these modifications, the coexpression networks analysis was performed. 251 (56 °%) of the 442 DEG had a coexpression level higher than the level to be expected randomly, hence, almost half of the DEG presented expression patterns with low correlation to other DEG, this, again, can be explained by the heterogeneity of aging and by the stochastic nature of some processes associated with it, such as the accumulation of DNA mutations over the years that would produce expression patterns not consistent among different samples, for the PFC of each person will have its own random mutation accumulation history 31,32.
Of the 251 coexpressed DEG, 246 genes were included in three coexpression networks, indicating that this gene group has a similar behavior along the analyzed samples, and that they could represent a common transcriptional response in PFC aging. All repressed genes were located in only one network (figure 2A) while most overexpressed genes were located in two connected networks and in a network composed of four genes (figures 2B and 2C).
The four genes network (figure 2C) was composed of the genes that codify for the glial fibrillary acid protein (GFAP), aquaporin 1 (AQP1), the acidic form of the complement factor 4 (C4A), and the apelin receptor (APLNR). The first two genes are recognized markers of the activation of astrocytes in response to nervous tissue damage. For instance, GFAP is overexpressed in astrocytes in response to trauma or ischemia and in neurodegenerative diseases, additionally, an increase in its expression during aging has been consistently reported since the 90's 33,34. Something similar occurs with the AQP1 gene that is expressed during the astrocyte polarization in response to brain damage, and in neurodegenerative diseases such as Parkinson's 35-37. Another gene of the network, C4a, is part of the humoral immune response; it has been proved that microglia cells express it during the formation of amyloid plaques in Alzheimer's disease 38. Besides, there is a clear increase in its expression associated with a higher age 39. Regarding the last gene in the network, APLNR, it has been recently reported that it acts as an important maintenance regulator mediated by the endothelium of glioblastoma derived cells; and in the same sense, in mice it was found that APLNR is crucial for astrocyte maturation through endothelial cell signaling action 40-42. In the overrepresented pathways in the group of overexpressed aging genes, astrocyte development and inflammatory pathways (table 1B) were found, both are connected to the four genes of this network. Three of the four genes take direct part on astrocyte activation, one of the best known and defined processes of brain aging 12,43. This indicates that the approach used in the present study is useful for finding relevant processes for the phenotype of interest and can identify relationships between molecular processes that interact as PFC aging determinants.
In the two coexpression networks with the highest number of genes (figure 2A and Figure 2B) a search for hub genes was conducted. The rationality behind identifying this type of genes is that it is probable that, having high topological characteristics, these genes control important points in the modulation of a coexpression network and, therefore, they will be important for the studied biological process 44,45. In the repressed genes network (figure 4A) four hub genes were identified: hypocalcin (HPCA), calcium voltage-gated channel auxiliary subunit gamma 3 (CACNG3), carbonic anhydrase-related protein 10 (CA10), and phospholipid phosphatase related 4 protein (PLPPR4). Hypocalcin is part of the family of specific neuron proteins that bind to calcium, their function is related to the regulation of the voltage dependent calcium channels 46. Another protein found in the network was CACNG3, which is specifically expressed in the brain, it is assumed that its function is to stabilize inactive calcium channels 47. It has been described that polymorphisms in this gene are associated with events of absence seizures in childhood 48. In the hippocampus of rats it was found that with aging there is a decrease of the regulating protein expression levels of the neurotransmission networks, among them HPCA and CACNG3 49. Our outcomes underline the importance of the decline of the synaptic function with aging and the central role of the calcium regulation in the transcriptomic modifications of the PFC over time. In the overrepresented pathways of the repressed genes group, synaptic transmission, signaling of glutamate receptor and memory were identified (table 1A), all these pathways in relation to the processes mediated by HPCA and CACNG3M proteins.
The third hub gene of the coexpression network of repressed genes is CA10, this gene codifies for a protein that catalyzes the reversible hydration of carbon dioxide in various biological processes, among them the development of the brain 50. CA10 protein binds directly to neurexin 1 (NRXNI) and promotes the expression in the pre-synaptic membrane surface of the a and ß neurexins; this process is relevant for the establishment and specifica tion of synaptic connections 51. Interestingly, we did not find a significative decrease of the NRXNI expression levels, CA10 primary ligand, this could indicate that the pathway alteration depends on the decline of CA10 signaling and not on an upstream alteration of it, a fact that stresses the central and regulating role of CA10 in this neuronal aging mechanism.
The last repressed hub gene is PLPPR4, it is part of the phospholipid phosphatases whose function is to catalyze dephosphorylation of bioactive lipidic mediators regulating different cell functions 52. PLPPR4 is expressed in neurons and is found specifically in growing axons. It has been proved that it plays an important role during the development and formation of new axonal terminals, the formation of filopodia, the extension of neurites, and the axonal reorganization after neural damage 53,54.
Our analysis identified the repression of two hub genes related to the neuronal function, specifically to establishing new synapsis (CA10), and the neuronal regeneration (PLPPR4). The fact that they are repressed in the PFC of individuals older than 60 years old explains, at least partially, the reasons why the neuronal dysfunction and the loss of cognitive functions appear.
This, additionally, is supported on the fact that one repressed pathway of the DEG analysis was the development of the nervous system (table 1A).
In the overexpressed gene network (figure 2B) only one hub gene was identified: Alpha-crystallin B chain (CRYAB). CRYAB is part of the family of small heat shock proteins that act as molecular chaperones. These chaperones bind to misfolded proteins to prevent their aggregation, they can also inhibit apoptosis and contribute to intracellular architecture 55. CRYAB is expressed both in astrocytes and in oligodendrocytes and it has been found that the overexpression of this gene in astrocytes decreases the neurological deterioration in mouse models of Huntington disease through the decrease of the aggregation of mutant huntingtin protein 56. Also in an animal model of glaucoma, a degenerative disease of the optical nerve, a neuroprotective effect with CRYAB injection in the vitreous was observed. However, CRYAB has been also connected to the development of CNS pathology, since it has been reported that in substantia nigra of persons with Parkinson's it is overexpressed, indicating a possible participation in the dopaminergic neuronal degeneration 57,58. Likewise, it has been informed that CRYAB increases astrocyte mediated demyelination in multiple sclerosis and that it increases oxidation of the beta amyloid protein, thus incrementing its aggregating potential and therefore its neurotoxicity 59,60. Unlike the former result, with the induction of the CRYAB expression in astrocytes, the beta amyloid protein aggregation is inhibited 61. These contradictory results, where CRYAB acts sometimes as a neuroprotective protein and in other cases as neurotoxic could be explained by the post-translational regulation of the protein. CRYB can be phosphorylated in different amino acids; phosphorylation of 19, 45 and 59 serines does not confer citoprotection, while phosphorylation of 59 and 45 serines does 59,62-64. In the altered pathways in the overexpressed genes, astrocyte development and myelinization were found de/regulated, although we couldn't identify the status of CRYAB phosphorylation due to the experimental design of our study, the fact that the myelinization pathway is increased and that, as previously described, CRYAB is involved in favoring this process in the peripheral nervous system, could suggest that the increase of the CRYAB expression in the PFC exerts a cytoprotective effect 65,66. However, additional studies are needed to define the protein real function in normal aging.
In conclusion, in the present study, five hub genes were identified in the transcriptomic deregulation secondary to PFC aging. Four of these five genes dominate the coexpression ne twork of repressed genes and are involved mainly in the synaptic function and the neuronal plasticity. The only overexpressed hub gene could be exerting a neuroprotective function in response to the synaptic dysfunction.
With the implemented methodological design, it was intended that identifying the genes that presented differential expression when comparing the group of young with the group of elder would allow describing common cell and molecular events in the studied samples. The fact that, when grouping the samples according to the expression levels of the five hub genes, a good discrimination between older and young (figure 3) was allowed, indicates that the selected hub genes could be candidates to biomarkers of biological processes generalizable for PFC aging. These results need to be validated in animal models and other human cohorts with normal and pathological aging. Once they are validated, they could be used as a basis for evaluating potential biomarkers of biological age, as prevention and treatment targets of neurodegenerative diseases, and for evaluating anti-aging therapies