Introduction
Gastric cancer (GC) is a significant public health concern. Globally, nearly one million new cases of GC were diagnosed in 2022, making it the fifth most common cause of cancer-related deaths, with 660,175 fatalities recorded in the same period1. In Colombia, according to GLOBOCAN 2022, there were an estimated 8938 new cases of GC, representing the fourth most frequent cancer (7.6%) and the leading cause of cancer mortality, with 6901 deaths (12.2%)1. This places the average mortality rate for GC in Colombia at approximately 11.5 per 100,000 inhabitants, with even higher rates in the Andean region2,3. The high incidence and the fact that most cases are detected at advanced stages make GC a critical public health issue.
Endoscopic technology has advanced significantly in recent decades and is now widely used for the diagnosis of early gastric cancer (EGC)4. Esophagogastro-duodenoscopy (EGD), or upper gastrointestinal endoscopy, is the preferred procedure for examining the stomach for premalignant lesions or cancer. However, it has been reported that between 11% and 20% of EGC cases are not diagnosed during an EGD5. This high error rate is primarily due to human factors that can affect the efficacy of endoscopy in the early detection of gastric lesions6.
These factors include the presence of mucus and saliva on the gastric mucosa, conducting the examination too quickly, and inadequate training of the endoscopist, who may not evaluate all areas of the stomach, leaving some blind spots7. Therefore, avoiding blind spots is a fundamental prerequisite for the efficacy of endoscopy in detecting early-stage gastric cancer8,9. In general, it is very challenging for a trainee physician to acquire the necessary skills to maneuver the endoscopic device and interpret the observed anatomy; this training requires hundreds of supervised procedures10. In this context, various gastroenterology associations worldwide have developed protocols to improve the efficiency and quality of the procedure11-13. Systematic photography of specific anatomical areas of the stomach is recommended as a quality indicator for endoscopic procedures by the British Society of Gastroenterology (BSG)14 and the European Society of Gastrointestinal Endoscopy (ESGE)15. They recommend acquiring images in eight specific locations within the stomach. The ESGE suggests an image capture rate (photodocumentation) of over 90% of all anatomical markers as an indicator of a complete examination. Meanwhile, the Japan Gastroenterological Endoscopy Society (JGES) implemented a more extensive systematic screening protocol of the stomach (SSS)16, initially described by Professor Kenshi Yao, consisting of a series of 22 endoscopic photographs capturing four areas that cover 100% of the gastric surface: the gastric antrum, distal body, middle, and upper part of the gastric body8.
Recently, the World Endoscopy Organization (WEO) provided practical guidelines for professionals to conduct comprehensive photodocumentation of endoscopic procedures. This document detailed 28 distinct endoluminal areas, covering the entire inner lining of the upper gastrointestinal tract, from the hypopharynx to the second portion of the duodenum17. Our study focuses on the gastric cavity; therefore, we used Dr. Yao’s guidelines as a reference for our research.
Despite the high agreement among experts, these protocols have not been widely disseminated, and many endoscopists neither visually document nor likely observe these regions15. This situation poses a challenge to ensuring a thorough inspection of the stomach, which could partly explain the difference in early-stage tumor detection rates between Japan and Colombia. In Japan, approximately 70% of cases are detected at this curable stage. In contrast, in Colombia, this percentage is minimally representative, as over 90% of diagnosed cases are in advanced stages, when the disease is potentially fatal19-23. Therefore, it would be desirable for endoscopists to practice a systematic inspection of the stomach, documenting these 22 specific areas to eliminate blind spots or unevaluated regions. However, to validate that this is routinely done, a monitoring system would be useful to verify the evaluation of all areas or alert the endoscopist about regions that may have been missed during the examination.
In this context, integrating computational methods into medical practice can provide substantial support to gastroenterologists, enabling them to routinely perform comprehensive and high-quality endoscopies. These methods can act as a second reader for the anatomical regions that need analysis by the specialist. This second reader could identify areas that have not been examined, have been incompletely evaluated, or are blind spots in the entire gastric cavity, potentially reducing the omission of precancerous and malignant lesions. By verifying whether all anatomical landmarks in Dr. Yao’s protocol have been inspected, the computational approach can play an essential role. If the system detects an incomplete examination, it can function as an alert, notifying the specialist that a specific anatomical region has yet to be evaluated. This is crucial, as early-stage gastric cancer can go unnoticed, often presenting as small or subtle lesions that could be overlooked if a specific area is not thoroughly examined.
Materials and methods
This study explores the automatic classification of different regions of the stomach that must be reviewed during endoscopic procedures using deep learning image classification methods. Figure 1 shows the layout of the regions of interest in the stomach, accompanied by sample images, and illustrates the proposed methodology. The strategy used is transfer learning, which leverages a deep learning model previously trained with millions of natural images to capture low-level visual features (e.g., edges), adapt the model’s high-level features to endoscopic images, and use them as input for a classification module.
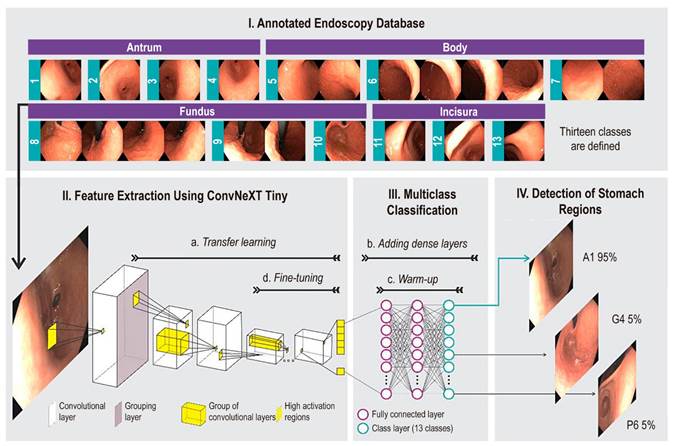
Source: Author’s own research.
Figure 1 Flow of the proposed method to automatically identify 13 gastric regions. In (I), the endoscopic image database is consolidated and categorized into anatomical regions, divided into training, validation, and test partitions. In (II), each training frame feeds a convolutional neural network-based model. This model (II-a) is pre-trained with millions of natural images. In (III-b), a densely connected layer is added to the neural network, and (III-c) it is pre-trained. In (II-d), neuron layers responsible for information extraction are unfrozen to learn contextual relationships. The trained network is evaluated in (IV) to classify each frame into one of the 13 regions of the stomach.
The first stage of the process, feature extraction, involves a Convolutional Neural Network (CNN) that has been pre-trained on a large dataset of natural images. The second stage, classification, involves a set of dense layers trained using the specific image set for the problem. The model’s output is a softmax layer with 13 neurons corresponding to the 13 different classes of stomach regions, assigning a probability to each class and highlighting the highest as the definitive classification for the region in question. For the feature extraction stage, a state-of-the-art CNN architecture, such as ConvNext Tiny24, was used. This architecture was selected for its superior performance in a previous study compared to 22 other evaluated networks25.
Acquisition and Preprocessing Protocol
Esophagogastroduodenoscopy and image capture are performed by a highly experienced gastroenterologist with over 20 years of practice in endoscopy and a history of over 50,000 procedures. This specialist works at Hospital Universitario Nacional de Colombia and follows the systematic endoscopy protocol developed by Dr. K. Yao8. The process unfolds as follows: when a patient is scheduled for an upper digestive endoscopy, they sign informed consent forms for the procedure, sedation, research protocol, and image capture before entering the room. They are then given a preparation consisting of 10 mL of a solution containing 400 mg of N-acetylcysteine and 200 mg of simethicone approximately half an hour before the procedure. The patient is instructed to lie in the left lateral decubitus position for 5 minutes, followed by a wait of 20 to 30 minutes before entering the procedure room. In the room, a cannula is placed in the patient’s right arm, and a certified anesthesiologist administers intravenous sedation with propofol. Once the patient is sedated, an Olympus series 190 endoscope is introduced.
Upon entering the gastric cavity, the endoscope performs aspiration of residues from the gastric contents, distends the cavity, and proceeds to locate itself in the duodenum. After inspecting the duodenum, the pylorus is identified, and the endoscope is withdrawn 5 cm to begin photodocumentation of the antrum. This process starts from the greater curvature and proceeds clockwise, capturing four overlapping photos as follows: greater curvature (photo 1-G1); anterior wall (photo 2-A1); lesser curvature (photo 3-L1); and posterior wall (photo 4-P1). The endoscope is then withdrawn 15 cm and positioned in the distal gastric body, where photos are taken in the same clockwise manner: greater curvature (photo 5-G2); anterior wall (photo 6-A2); lesser curvature (photo 7-L2); and posterior wall (photo 8-P2). Next, the endoscope is withdrawn an additional 15 cm and positioned in the upper-middle gastric body to continue the recording in a clockwise direction: greater curvature (photo 9-G3); anterior wall (photo 10-A3); lesser curvature (photo 11-L3); and posterior wall (photo 12-P3). Subsequently, the gastroscope is advanced to the corpoantral junction, where retroflexion is performed to visualize the cardia and gastric fundus. From this position, photodocumentation continues as follows: greater curvature (photo 13-G4); anterior wall (photo 14-A4); lesser curvature (photo 15-L3); and posterior wall (photo 16-P4). With the endoscope in retroversion, it is advanced along the lesser curvature by 5 cm to fully visualize it and obtain the following three photos: anterior wall (photo 17-A5); lesser curvature (photo 18-L5); and posterior wall (photo 19-P5). Finally, the tip of the endoscope is positioned at the incisura and visualized in its entirety to obtain the last set of photos: anterior wall (photo 20-A6); lesser curvature (photo 21-L6); and posterior wall (photo 22-P6). The results of this documentation are presented in Figure 2.
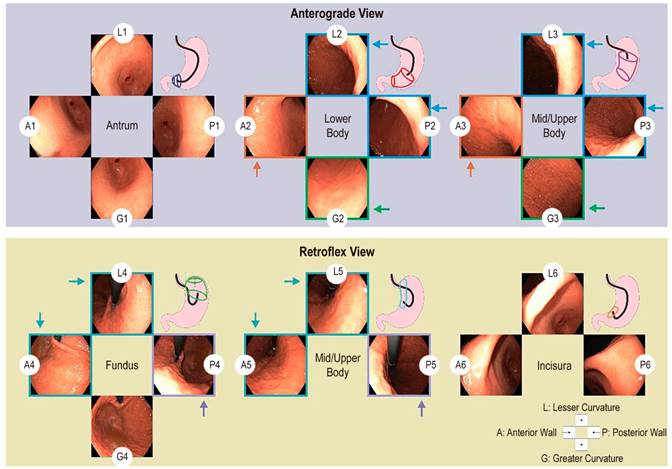
Source: Author’s own research.
Figure 2 Protocol for photographic documentation of the stomach, which begins as soon as the endoscope is inserted into the gastric antrum. Using the anterograde view, endoscopic photographs are taken of four quadrants of the gastric antrum, lower, middle, and upper body. Then, using the retroflex view, endoscopic photographs are taken of four quadrants of the cardia fundus and three quadrants of the upper middle body and gastric incisura. In total, the SSS series comprises 22 endoscopic photographs. The arrows represent the unification of labels to obtain 13 categories. A: anterior wall; G: greater curvature; L: lesser curvature; P: posterior wall; Q: quadrant.
After capturing images of the 22 regions and completing the process of assigning labels in an information system, the creation of the artificial intelligence model, named GastroUNAL in honor of our university, begins. We observed that some areas are anatomically very similar and often overlap, making differentiation difficult. Therefore, in collaboration with the team, we decided to regroup the 22 zones into 13 by merging those that inspect similar areas. The grouped regions are described as follows:
Lower body region in L2 and P2, and upper middle region in L3 and P3
Lower body region in A2 and upper middle region in A3
Lower body region in G2 and upper middle region in G3
In retroflex view, cardia fundus region in L4, A4, and upper middle region in L5, A5
In retroflex view, cardia fundus region in P4 and upper middle region in P5
Case Collection and Region Labeling System
The storage, organization, and labeling of endoscopic videos and images corresponding to the 22 regions of the stomach were carried out using a prototype information system developed specifically for this study. The system was designed based on the functional requirements gathered with the guidance of two gastroenterologists. These requirements encompass the clinical information needed to document an endoscopy study, the strategies for visualizing endoscopic videos and images, and the tools necessary for labeling these videos and images. The non-functional requirements were established collaboratively with a team of three engineers experienced in clinical prototypes. They focused on scalability, security, interoperability, and usability to ensure stable operation and secure handling of sensitive data. This system was developed using the Django web development framework in conjunction with the PostgreSQL database management system. This combination provides a secure and reliable environment for capturing sensitive information, such as clinical data. Django incorporates advanced security strategies for transmitting data via the web, which PostgreSQL then encrypts and stores. According to the requirements, the system includes the following modules:
Authentication: Controls user registration and access.
Patient Management: Allows users to view, create, and edit information of patients involved in the study.
Endoscopic Procedure Management: Assigns one or more procedures to a specific patient, capturing the associated clinical information, the video of the procedure, and the informed consent.
Procedure Visualization and Labeling: The system displays the video of a procedure in a web player (Figure 3-I) along with the specific frame sequence for the current moment of the video (Figure 3-II). When the user, in this case, a gastroenterologist, identifies one of the 22 gastric regions while viewing the video, they can select and label an image or a sequence of images representing that region (Figure 3-III).
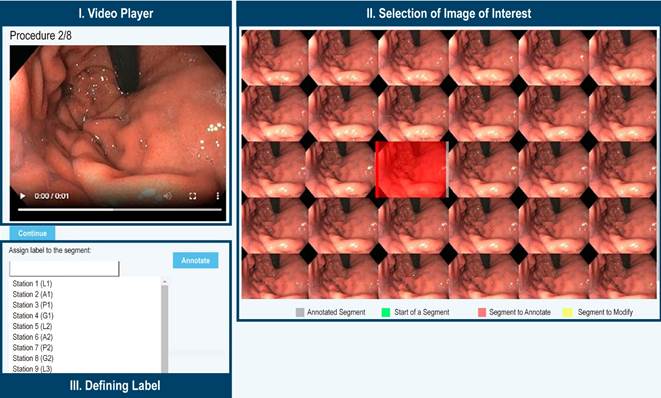
Source: Author’s own research.
Figure 3 Anatomical Region Labeling System. The video of the procedure is displayed alongside the images associated with the current moment of the video, allowing the annotation of the image that best represents the region. Once the image is selected, it is marked in red, and an option to assign a label to the image is enabled.
Architecture of the Convolutional Neural Network for Describing Endoscopic Images
A Convolutional Neural Network (CNN) constructs a hierarchical representation of distinctive gastric patterns based on appearance-such as color and texture-and anatomical structures from various perspectives, including gastric folds, the lower esophageal sphincter, pylorus, and incisura, among others. A CNN is organized as a set of neurons grouped into layers, which are sequentially connected from input to output, as illustrated in Figure 1 (II-III). The initial stages of these layers take an image as input and decompose it into primitives, which are extracted by applying convolutions or sets of filters designed to capture local patterns. This initial transformation of the image is processed sequentially through consecutive layers of additional convolutions, progressively selecting relevant information. Throughout this process, layers are commonly introduced to reduce dimensionality or group information, along with other layers that normalize the output of each neuron using activation functions to mitigate signal attenuation from sequential processing. This relatively simple process is repeated layer by layer, transforming the image into increasingly complex, non-linear patterns with fewer statistical dependencies.
The architecture of the network is defined by the number of filters, layers, and neurons, which in turn determine the number of parameters in the model, typically in the order of millions. For this study, we selected the ConvNext Tiny architecture24, a convolutional neural network that learns spatial relationships at different scales while maintaining the simplicity and efficiency of traditional convolutional networks. The ConvNeXt architecture consists of a sequence of blocks called Inverted Bottleneck Blocks. Each block includes the following components: depthwise separable convolution, self-attention mechanism, traditional convolution, layer normalization, and GeLU (Gaussian Error Linear Units) activation. This architecture incorporates direct connections between non-contiguous layers, known as residual blocks, which help mitigate the gradient attenuation problem. The model’s architecture comprises 27 million parameters, of which 11,000 correspond to the fully connected layers of the neural network, with the remainder assigned to the weights of the convolutional layers.
Classification of Endoscopic Images Using Transfer Learning
The performance of a convolutional neural network (CNN) in classification tasks significantly depends on the volume of data used; training it with a small dataset is ineffective. This study implemented transfer learning on the ConvNeXt architecture, utilizing the extensive ImageNet database as the initial information source, which includes more than 14 million natural images distributed across nearly 20,000 categories. The ConvNeXt has been rigorously trained and validated with these images, leveraging the large volume of examples in ImageNet26,27. Similar to how the human visual system constructs a representation of the world using visual primitives up to the primary cortex28-30, and learns specific domain associations in higher regions, these networks capture the informative units of an image. This learned information can then be adapted for this specific problem. This process, known as transfer learning, involves using the weights of filters learned from millions of natural image examples while adapting some layers, usually the last ones, to learn from upper gastrointestinal endoscopy images. This training consists of three stages:
Frozen convolutional layers: These are layers whose parameters are not updated with endoscopic images.
Unfrozen layers: These layers have their parameters adjusted by training the network with endoscopic images (fine-tuning), resulting in a feature vector output.
Classification layers: These fully connected layers are trained for the specific classification problem presented in this study (Figure 1). The number of neurons in the output layer is determined by the number of classes. This final layer also provides a confidence score, indicating the probability that an image belongs to a specific class (Figure 1-IV). The learning process of the network is iterative and adaptive, using a relatively low learning rate over several iterations (epochs). In each epoch, data batches are trained until all samples in the training set have been processed. The number of epochs is determined by the complexity of the classification problem. Finally, the training concludes when the classification error on the validation data is below a specified threshold.
Databases
The database comprises 96 patient cases who underwent EGD procedures. From the white-light videos recorded, 2054 images were obtained, with their anatomical locations established as one of the 22 possible regions in the Kenshi Yao protocol (see the Label column in Table 1)8, later unified into 13 regions. These annotations were made by consensus among the group of residents and the head of gastroenterology, who has over 20 years of experience at Hospital Universitario Nacional. Each frame was captured with a spatial resolution of 1350 x 1080 pixels. This study was conducted in accordance with the principles of the Declaration of Helsinki and received approval from the Ethics Committee of Hospital Universitario Nacional de Colombia (approval number CEI-2019-06-10). A detailed description of the database is presented in Table 1.
Table 1 Distribution of the EGD Database in Images for the Validation Scheme
Name | Label* | Training (n = 58) | Validation (n = 9) | Test (n = 29) | Total (n = 96) |
---|---|---|---|---|---|
Antrum A1 | 1 | 48 | 7 | 29 | 84 |
Antrum L1 | 2 | 48 | 5 | 37 | 90 |
Antrum P1 | 3 | 45 | 7 | 30 | 82 |
Antrum G1 | 4 | 56 | 7 | 33 | 96 |
Body A2-3 | 5 | 114 | 18 | 58 | 190 |
Body L2-3, P2-3 | 6 | 223 | 39 | 121 | 383 |
Body G2-3 | 7 | 114 | 19 | 58 | 191 |
Cardia-Fundus A4-5, L4-5 | 8 | 231 | 38 | 120 | 389 |
Cardia-Fundus P4-5 | 9 | 106 | 18 | 58 | 182 |
Cardia-Fundus G4 | 10 | 54 | 8 | 33 | 95 |
Incisura A6 | 11 | 46 | 12 | 31 | 89 |
Incisura L6 | 12 | 56 | 10 | 34 | 100 |
Incisura P6 | 13 | 44 | 10 | 29 | 83 |
n: number of patients. *Label: corresponds to the station or grouping. Labels 1-7 are for anterograde view, and labels 8-13 are for retroflex view.
Source: Author’s own research.
Ethical considerations
This study was conducted in accordance with Resolution 008430 of 1993, which establishes the scientific, technical, and administrative standards for research involving humans, as specified in Article 11. This project is classified as minimal-risk research since it only involves the use of digital images generated from anonymized endoscopic videos. Therefore, there is no way to identify the subjects included in the study.
Study participants provided informed consent, clarifying that the information obtained during their medical care could be used for educational and research purposes. This agreement ensures that such data, including anonymized images and videos, will be handled with the highest standards of confidentiality, security, and custody, in compliance with applicable regulations for this type of research.
Results
This section evaluates the performance of the proposed methodology by comparing the predictions of a convolutional neural network with the annotations made by a highly qualified expert. The following details the experimental setup and the quantitative validation scheme applied to the proposed approach.
Experimental Setup
Our study involved 96 patients with an average age of 62 ± 15.5 years. Among these participants, 50.6% were women and 49.4% were men. The developed model was evaluated using a 70-30 split scheme: 70% of the cases for training and validation (67 cases, 1383 images) and the remaining 30% for testing (29 cases, 671 images). Cross-entropy with class weights was used as the loss function to balance the number of samples relative to the predominant class (Table 1). This unequal proportion was maintained in the test dataset.
The CNN was trained in two stages: a pre-training of the classification layers (warm-up) with a constant learning rate over 10 epochs, and fine-tuning of the last 20% of layers over 100 epochs with an early stopping criterion of 15 epochs without an increase in the macro F1-score metric. The details of the CNN and training configuration are as follows:
Pretrained weights: ImageNet
Optimizer: Adam
Loss function: cross-entropy
Dense layers: one dense layer followed by a batch normalization layer, a dropout layer, and finally two dense layers with 13 categories
The warm-up and fine-tuning stages were included in a hyperparameter optimization with 200 trials, monitoring the F1 metric to find the optimal batch size, initial learning rate, and learning rate schedule (gamma and step size). The values during the optimization were as follows:
Learning rate for pre-training: 0.001 with gamma: 0.1
Range of hyperparameter values during optimization: batch size (8-128), gamma (0.1-0.5), step size (5-10), and learning rate (1e -3 to 1e -5)
After the optimization process, the hyperparameters that maximized the F1 score were found in validation trial 132/200, with a batch size of 8, a learning rate of 0.00071, a step size of 7, and a gamma of 0.30 for the learning rate schedule.
Quantitative Evaluation
The convolutional neural network (CNN) is designed to estimate one of 13 possible anatomical locations for each image, as illustrated in Figure 4. These categories correspond to different anatomical regions: the antrum (4 classes), the gastric body (3 classes), the cardia fundus (3 classes), and the incisura (3 classes). The network’s performance is evaluated by comparing its predictions with expert annotations using a confusion matrix that records true positives (TP), true negatives (TN), false positives (FP), and false negatives (FN). Specific metrics for each class, as well as an overall average of these metrics, are calculated from this matrix, as presented in Table 2. The results demonstrate that the proposed method effectively classifies the 13 classes, achieving an average accuracy of 86%.
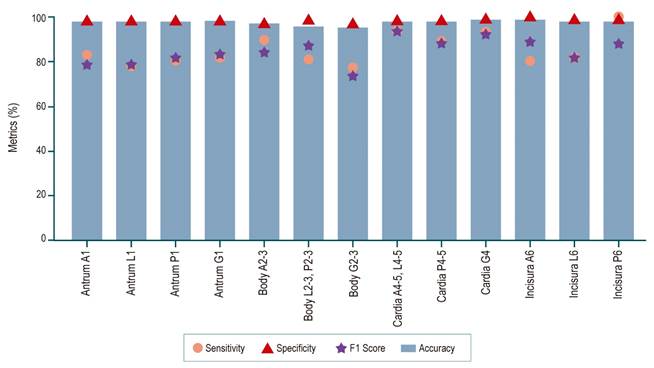
Source: Author’s own research.
Figure 4 Sensitivity, specificity, and accuracy metrics of the CNN for classifying stomach regions in EGD images into 13 locations.
Table 2 Results for the Proposed Configuration
Metric (%) | 13 Stations |
---|---|
Accuracy | 85.99 |
Specificity | 98.82 |
Macro Sensitivity | 85.53 |
Macro Precision | 84.73 |
Macro F1 | 84.86 |
Weighted Sensitivity | 85.99 |
Weighted Precision | 86.64 |
Weighted F1 | 86.07 |
Source: Author’s own research.
Given that the primary objective of this study is the detection of anatomical regions, it is important to highlight that the method achieves the highest F1 score (94.1%) for the cardia region A4-5, L4-5 in the retroflex view (Figure 4). In contrast, the class with the lowest F1 score is the gastric body G2-3, with 73.7% (Figure 4). This is likely due to the fact that, in some cases, the gastric tissue does not present a distinctive pattern that allows for precise region localization, a limitation that could be mitigated with a larger training dataset. Nonetheless, it is important to emphasize that the proposed method demonstrates a strong capability to handle the high variability of the various anatomical structures and views.
Qualitative Evaluation
Using Grad-CAM (Gradient-Weighted Class Activation Mapping), we identified the areas in the images that the network considers most representative for distinguishing between different anatomical regions. Figure 5 illustrates the evaluation of a test case involving the 13 classes.
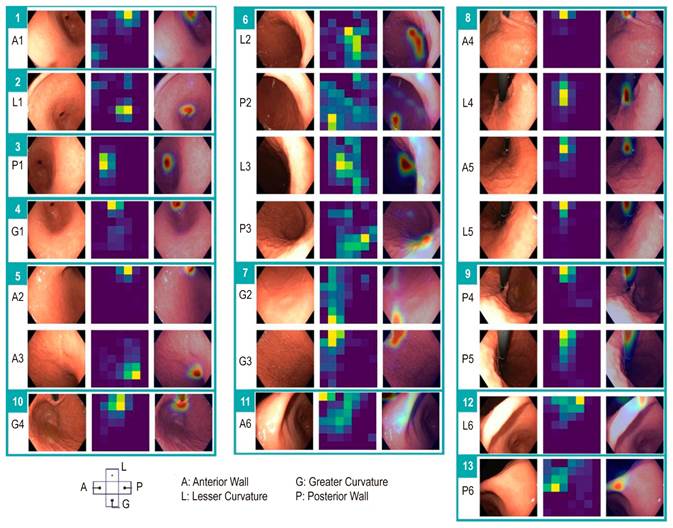
Source: Author’s own research.
Figure 5 Analysis of a convolutional neural network in a test case. The sequence includes an endoscopic image, a heatmap visualizing the importance of the areas of the image in the network’s prediction, and the superposition of this heatmap on the endoscopic image to highlight the relevant zones. In this case, 13 regions (marked with a green rectangle) were identified with a prediction confidence above 95%, matching the labels assigned by an expert. Within the rectangle, A1-P6 corresponds to the region grouping mechanism. A: anterior; G: greater curvature; L: lesser curvature; P: posterior.
For the regions of the antrum (A1, L1, P1, and G1), it is observed that the pattern of the pyloric sphincter location is crucial. For the gastric body regions, the network uses the quadrant of the gastric wall. Regarding the cardia fundus, the positioning and location of the endoscope determine the class structure. Finally, for the incisura (A6, L6, and P6), capturing the anterior, middle, and posterior portions is crucial for accurate identification.
Discussion
Upper gastrointestinal endoscopy, also known as esophagogastroduodenoscopy (EGD), is the preferred method for diagnosing gastric cancer and premalignant lesions. Identifying and monitoring these lesions is crucial to prevent this serious disease, necessitating high-quality endoscopic procedures. EGD is also used as a screening tool for early diagnosis of upper gastrointestinal cancer in high-risk areas18. In a study by Hamashima, Chisato, and colleagues31, the impact of endoscopic screening on reducing gastric cancer mortality was evaluated. The results indicated that gastric cancer mortality was significantly lower in the endoscopic screening group compared to the radiographic screening and photofluorography groups. The study concluded that endoscopic screening for gastric cancer is associated with a notable 57% reduction in mortality from this disease, highlighting its effectiveness in early detection and treatment.
In clinical practice, a systematic approach by a gastroenterologist during endoscopy minimizes the likelihood of missing areas or having blind spots. However, there is generally no record of how a specialist performed the procedure, whether a minimum number of regions were observed, or the duration of observation for each region. Consequently, photodocumentation has become an important aspect of the EGD report. A recent study found a positive correlation between the number of endoscopic images and the detection rate of clinically significant gastric lesions (p < 0.001). In the clinical setting, a trained endoscopist can perform systematic and efficient photodocumentation, but this requires proper endoluminal orientation through extensive prior training and a clean mucosa to ensure optimal visualization. Inadequate mucosal cleaning can impact the quality and integrity of the procedure. Successful photographic documentation also demands adequate insufflation and equipment control. Additionally, patient movement and anatomical variability can influence this documentation. Finally, a significant barrier is the storage and analysis of images, which remains a common disadvantage of current endoscopic equipment17.
Currently, well-designed studies are needed to examine the relationship between the number of images, a specific photodocumentation protocol, and the neoplasm detection rate during EGD32. However, an Asian consensus on standards in upper gastrointestinal endoscopy suggests that methodical observation of the upper gastrointestinal tract can improve the detection of superficial neoplasms by minimizing unvisualized areas. Additionally, the expert panel concluded that current evidence supports the use of simethicone to enhance visual clarity during the examination33.
In this context, a second reader (artificial intelligence) should not only recognize the gastric anatomical regions of interest but also ensure they are observed for a minimum duration. Thorough inspection of the entire gastric cavity is a crucial step in the systematic search for early gastric cancer. Consequently, there is a global interest in applying artificial intelligence in this area, and some researchers have classified frames using convolutional neural network (CNN) architectures34. For example, Takiyama and colleagues35 used a GoogleNet architecture to accurately recognize four anatomical locations (larynx, esophagus, stomach, and duodenum), as well as three specific sub-classifications for stomach images. Wu and colleagues36 employed a VGG-16 network to classify gastric locations into 10 categories and then refined the classification into 26 anatomical parts (22 for the stomach, 2 for the esophagus, and 2 for the duodenum). Additionally, Chang and colleagues37 trained a ResNeSt architecture to classify EGD images into eight anatomical locations, with an additional location specifically for the pharynx. However, most proposed architectures have a large number of parameters, making real-time task resolution challenging, thus necessitating the training of smaller architectures. In a previous study25, the performance of 22 architectures was compared to identify six anatomical regions of the stomach, including state-of-the-art architectures, and the best performance was obtained with ConvNext Tiny. This network has around 27 million parameters, which would allow its implementation in a clinical setting.
In the present study, we developed an automated auditing system for the endoscopic exploration protocol of the stomach using artificial intelligence, enabling the identification of gastric areas. We achieved a macro sensitivity of 85.5% in interpreting the 13 specific areas previously described. These results demonstrate the potential effectiveness of artificial intelligence as a valuable tool to assist endoscopists in conducting thorough and meticulous examinations of the entire stomach.
Various experts and organizations have proposed guidelines regarding the number of images that should be captured during an EGD. An appropriate number of images encourages endoscopists to perform the procedure with greater attention, and this information can be used to train, validate, and improve the performance of artificial intelligence strategies. This factor is essential to overcome the challenge of effectively addressing this clinical problem with AI technology.
One of the major limitations of this type of study is the lack of a common validation framework, a frequent issue in the analysis of endoscopic images, which has limited the comparison between existing approaches. It is difficult to determine which of these methods could have a real advantage in clinical use.
In summary, this proposal presents a computerized quality monitoring system that identifies 13 anatomical gastric regions (covering the entire gastric cavity) using a convolutional neural network. The performance of this methodology could be improved and applied to more anatomical regions with an increase in annotated cases. This system is expected to become a valuable tool for the recognition of stomach regions, alerting to incomplete explorations of the gastrointestinal tract and, consequently, ensuring the quality of the procedure. Additional studies are currently being conducted to further validate and enhance the effectiveness of this system.
Finally, this pioneering study, which to our knowledge is the first in Latin America, can guide future research on the application of automatic classification to the 22 gastric regions recommended by K. Yao8 or other upper endoscopy photodocumentation protocols as presented in other studies14,15,17.